⚠️ 以下所有内容总结都来自于 大语言模型的能力,如有错误,仅供参考,谨慎使用
🔴 请注意:千万不要用于严肃的学术场景,只能用于论文阅读前的初筛!
💗 如果您觉得我们的项目对您有帮助 ChatPaperFree ,还请您给我们一些鼓励!⭐️ HuggingFace免费体验
2025-04-15 更新
Generalized Multilingual Text-to-Speech Generation with Language-Aware Style Adaptation
Authors:Haowei Lou, Hye-young Paik, Sheng Li, Wen Hu, Lina Yao
Text-to-Speech (TTS) models can generate natural, human-like speech across multiple languages by transforming phonemes into waveforms. However, multilingual TTS remains challenging due to discrepancies in phoneme vocabularies and variations in prosody and speaking style across languages. Existing approaches either train separate models for each language, which achieve high performance at the cost of increased computational resources, or use a unified model for multiple languages that struggles to capture fine-grained, language-specific style variations. In this work, we propose LanStyleTTS, a non-autoregressive, language-aware style adaptive TTS framework that standardizes phoneme representations and enables fine-grained, phoneme-level style control across languages. This design supports a unified multilingual TTS model capable of producing accurate and high-quality speech without the need to train language-specific models. We evaluate LanStyleTTS by integrating it with several state-of-the-art non-autoregressive TTS architectures. Results show consistent performance improvements across different model backbones. Furthermore, we investigate a range of acoustic feature representations, including mel-spectrograms and autoencoder-derived latent features. Our experiments demonstrate that latent encodings can significantly reduce model size and computational cost while preserving high-quality speech generation.
文本转语音(TTS)模型可以通过将音素转换为波形来生成多种自然语言、类似人类的语音。然而,由于不同语言之间音素词汇的差异以及韵律和说话风格的差异,多语言TTS仍然具有挑战性。现有方法要么针对每种语言训练单独模型,虽然计算资源消耗较大但性能较高;要么使用统一的多语言模型,难以捕捉精细的语言特定风格变化。在这项工作中,我们提出了LanStyleTTS,这是一个非自回归、语言感知的风格自适应TTS框架,它标准化了音素表示,并启用了跨语言的精细音素级风格控制。这种设计支持统一的多语言TTS模型,无需训练特定语言的模型即可产生准确且高质量的语音。我们将LanStyleTTS与几种先进的非自回归TTS架构进行了集成。结果表明,在不同模型主干上均实现了性能改进。此外,我们研究了一系列声音特征表示,包括梅尔频谱和自动编码器衍生的潜在特征。我们的实验表明,潜在编码可以显著减小模型大小并降低计算成本,同时保持高质量语音生成。
论文及项目相关链接
Summary
本文提出了一种非自回归的、语言感知的风格自适应TTS框架——LanStyleTTS,该框架能标准化音素表示并支持跨语言的音素级风格控制。该设计实现了一个统一的多语言TTS模型,无需训练特定语言的模型即可产生准确、高质量的语音。
Key Takeaways
- TTS模型能将音素转化为波形,生成自然、类似人声的跨语言语音。
- 多语言TTS面临挑战,如音素词汇的差异和语音风格的变化。
- 现有方法包括为每种语言训练单独模型或使用统一模型,但前者计算资源消耗大,后者难以捕捉语言特定的细微风格变化。
- LanStyleTTS是一个非自回归、语言感知的风格自适应TTS框架,能标准化音素表示,实现跨语言的音素级风格控制。
- LanStyleTTS支持统一的多语言TTS模型,无需训练特定语言的模型,就能产生准确、高质量的语音。
- LanStyleTTS与多种先进的非自回归TTS架构相结合进行评估,证明其性能提升。
点此查看论文截图
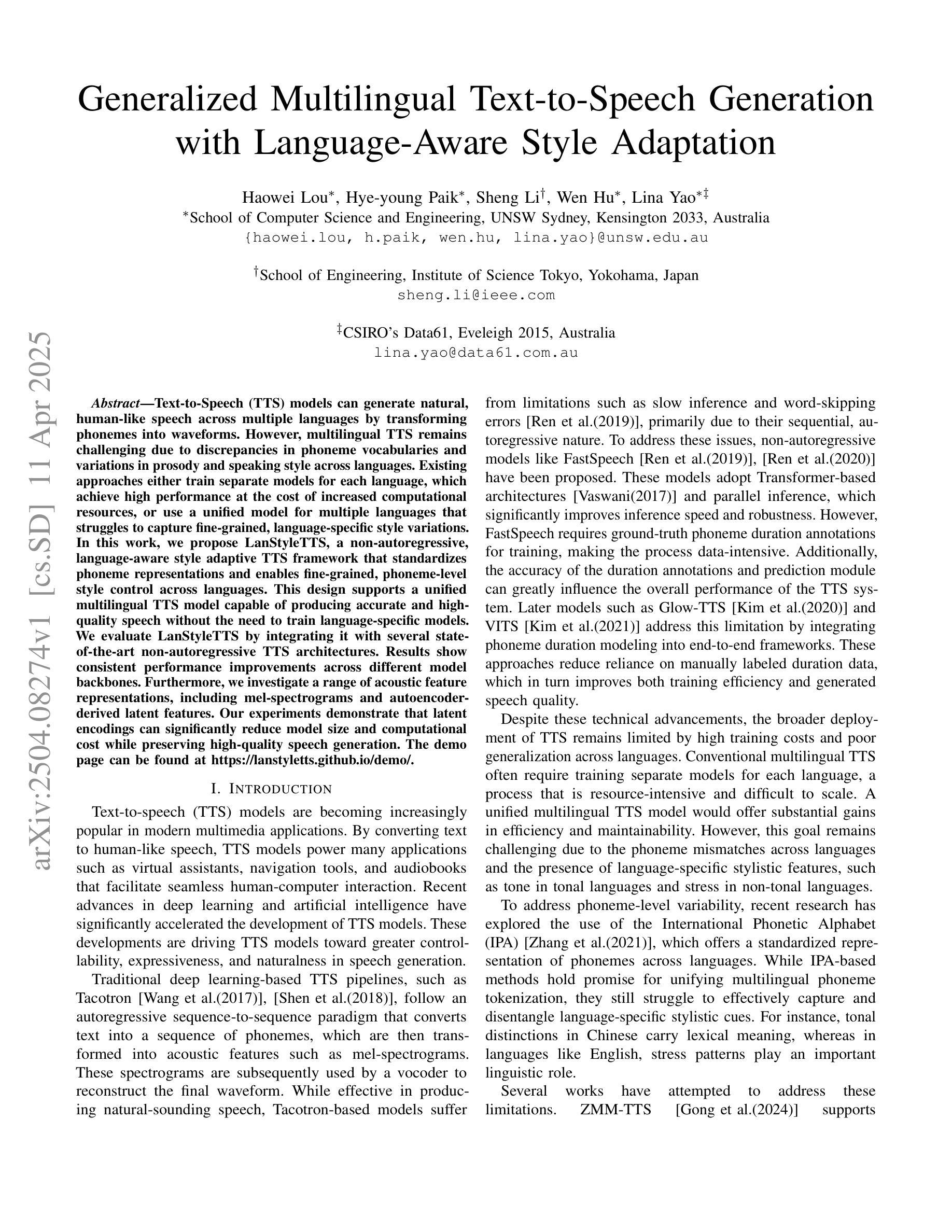
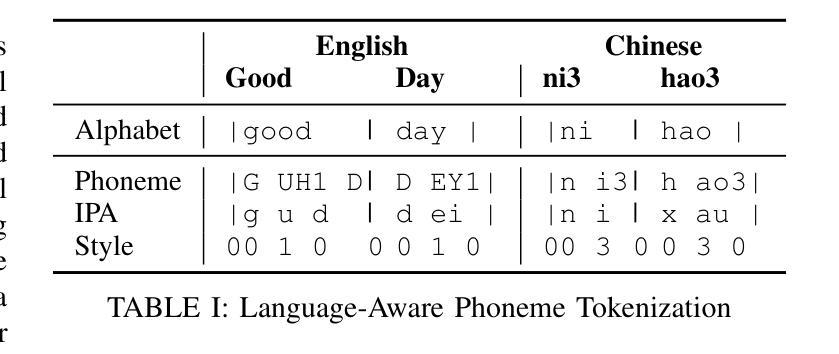
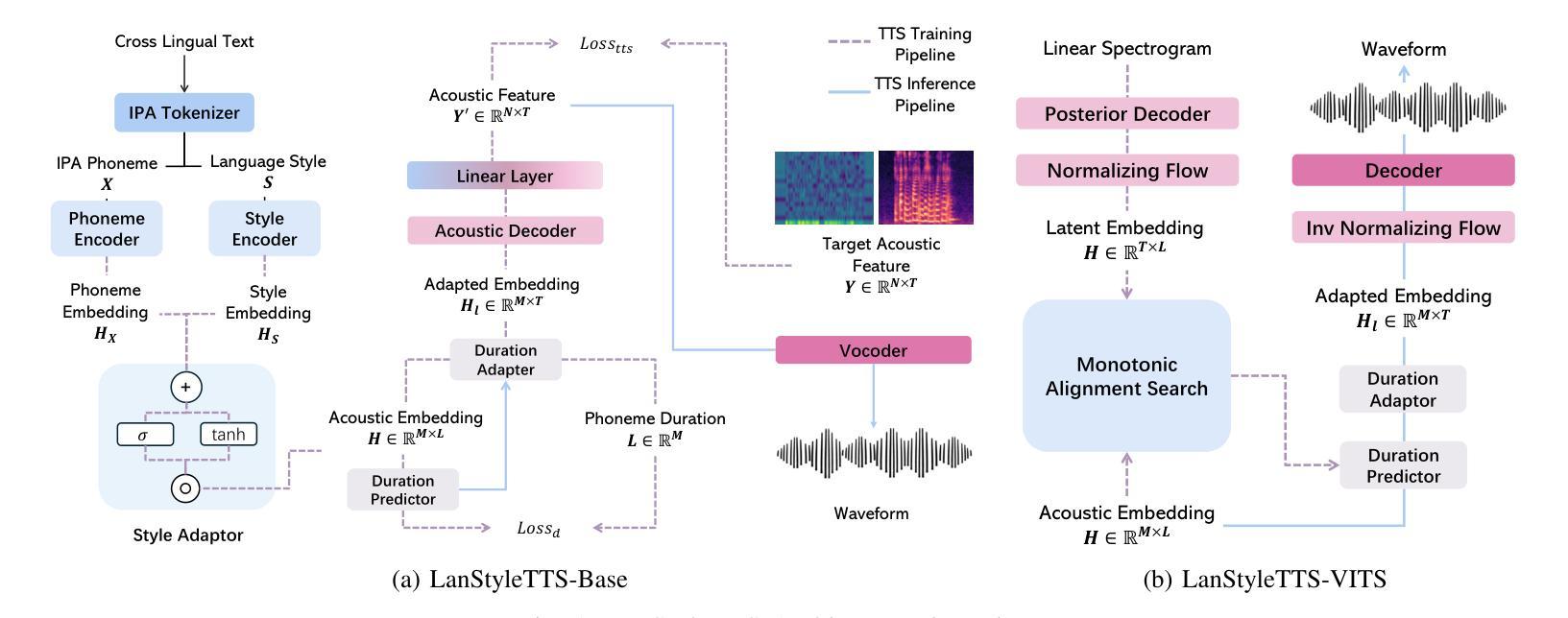
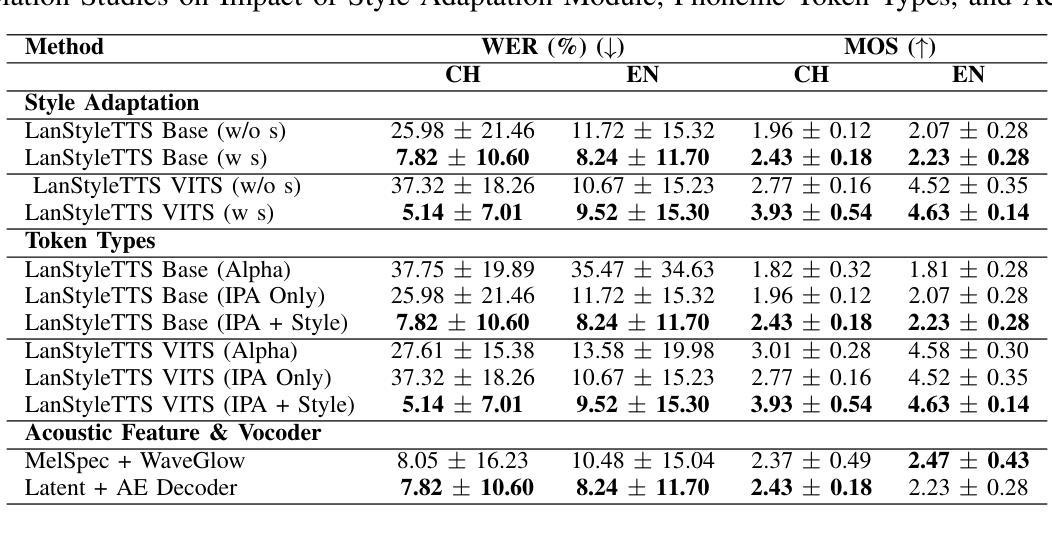
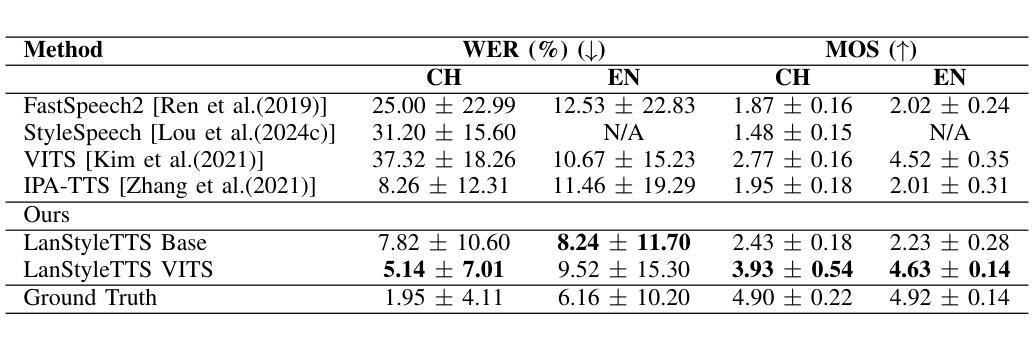