⚠️ 以下所有内容总结都来自于 大语言模型的能力,如有错误,仅供参考,谨慎使用
🔴 请注意:千万不要用于严肃的学术场景,只能用于论文阅读前的初筛!
💗 如果您觉得我们的项目对您有帮助 ChatPaperFree ,还请您给我们一些鼓励!⭐️ HuggingFace免费体验
2025-04-15 更新
EasyGenNet: An Efficient Framework for Audio-Driven Gesture Video Generation Based on Diffusion Model
Authors:Renda Li, Xiaohua Qi, Qiang Ling, Jun Yu, Ziyi Chen, Peng Chang, Mei HanJing Xiao
Audio-driven cospeech video generation typically involves two stages: speech-to-gesture and gesture-to-video. While significant advances have been made in speech-to-gesture generation, synthesizing natural expressions and gestures remains challenging in gesture-to-video systems. In order to improve the generation effect, previous works adopted complex input and training strategies and required a large amount of data sets for pre-training, which brought inconvenience to practical applications. We propose a simple one-stage training method and a temporal inference method based on a diffusion model to synthesize realistic and continuous gesture videos without the need for additional training of temporal modules.The entire model makes use of existing pre-trained weights, and only a few thousand frames of data are needed for each character at a time to complete fine-tuning. Built upon the video generator, we introduce a new audio-to-video pipeline to synthesize co-speech videos, using 2D human skeleton as the intermediate motion representation. Our experiments show that our method outperforms existing GAN-based and diffusion-based methods.
音频驱动的同步语音视频生成通常涉及两个阶段:语音到动作和动作到视频。虽然语音到动作的生成已经取得了重大进展,但在动作到视频系统中,合成自然表情和动作仍然具有挑战性。为了提高生成效果,早期作品采用了复杂的输入和训练策略,并需要大量数据集进行预训练,这给实际应用带来了不便。我们提出了一种简单的单阶段训练方法和一种基于扩散模型的时序推理方法,以合成逼真且连续的动作视频,而无需对时序模块进行额外的训练。整个模型利用现有的预训练权重,并且一次只需要几千帧数据即可完成对每个人物的微调。在视频生成器的基础上,我们引入了一个新的音频到视频的流水线,利用二维人体骨架作为中间运动表示来合成同步语音视频。我们的实验表明,我们的方法优于现有的基于GAN和扩散的方法。
论文及项目相关链接
Summary
文本提出了一种基于扩散模型的简单一阶训练方法和时序推理方法,用于合成真实且连续的手势视频,无需额外训练时序模块。该方法利用现有预训练权重,只需一次性对每字符数千帧数据进行微调,即可实现优质效果。此外,研究团队构建了一个基于视频生成器的新音频到视频管道,以合成共语视频,使用二维人体骨架作为中间运动表示。实验表明,该方法优于现有的基于GAN和扩散的方法。
Key Takeaways
- 音频驱动对话视频生成包含两个阶段:语音到动作和动作到视频。
- 尽管语音到动作生成取得了显著进展,但动作到视频系统中的自然表情和手势合成仍然具有挑战性。
- 此前的研究采用了复杂的输入和训练策略,需要大量的数据集进行预训练,给实际应用带来了不便。
- 文本提出了一种基于扩散模型的简单一阶训练方法和时序推理方法,能合成真实且连续的手势视频,无需额外训练时序模块。
- 该方法利用现有预训练权重,只需少量数据即可完成微调。
- 研究团队构建了基于视频生成器的音频到视频管道,使用二维人体骨架作为中间运动表示。
点此查看论文截图
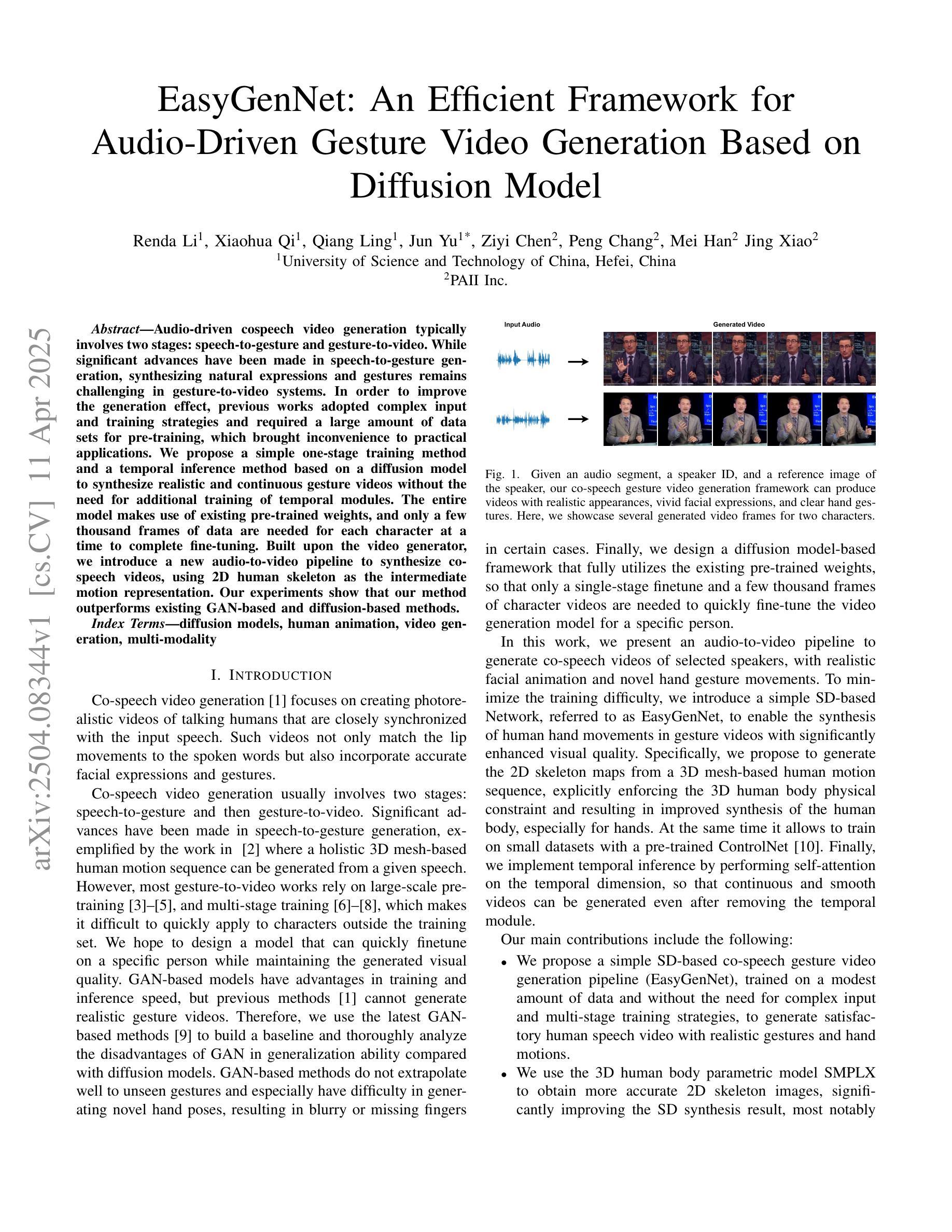
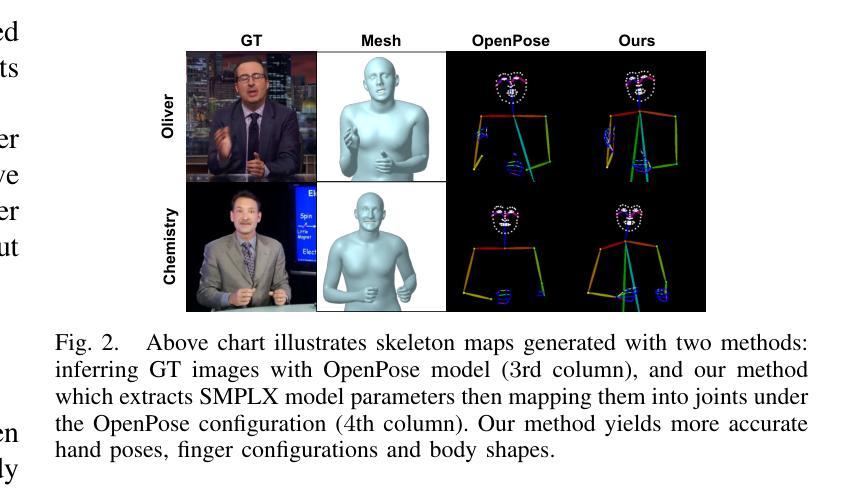
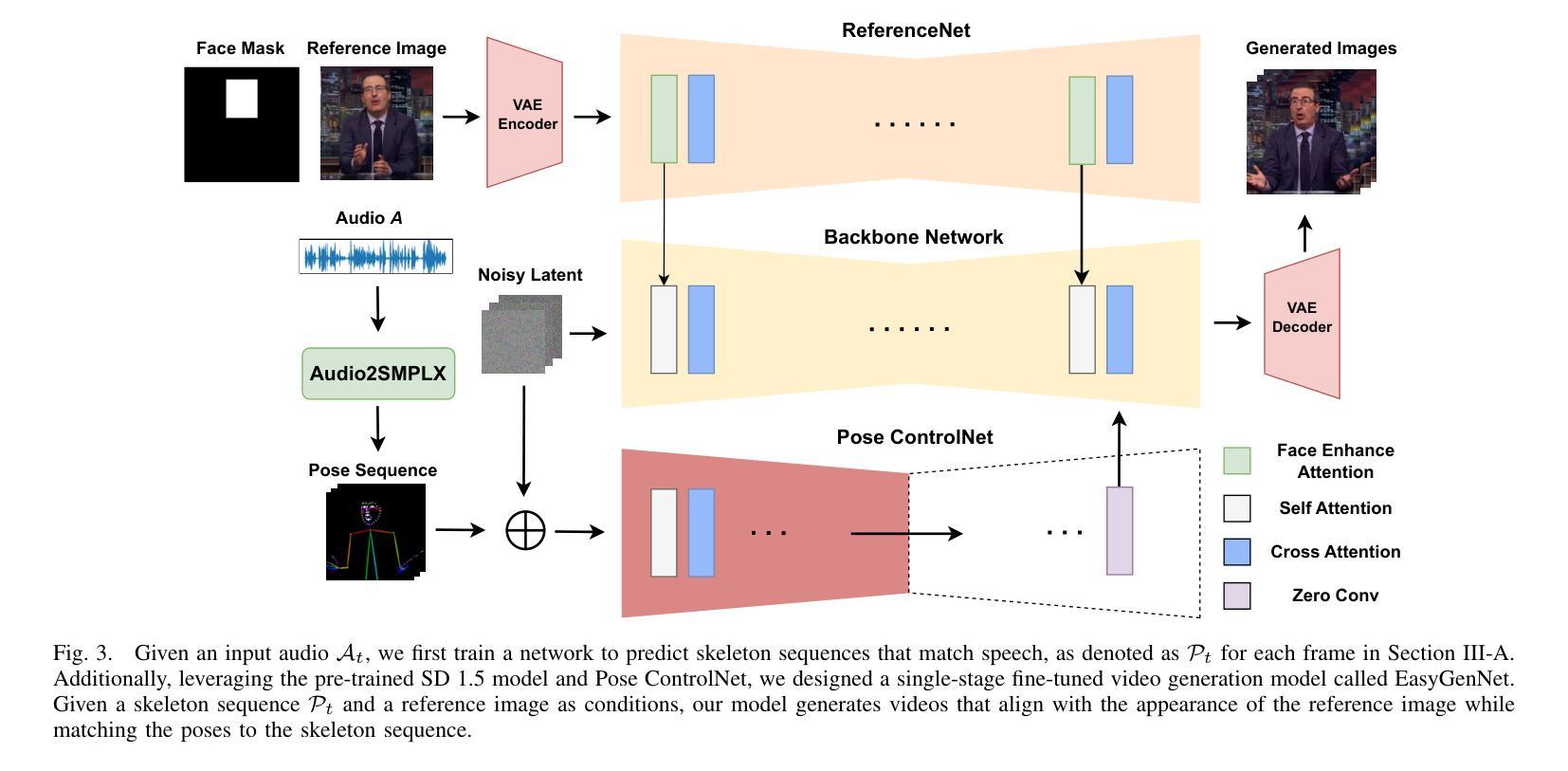
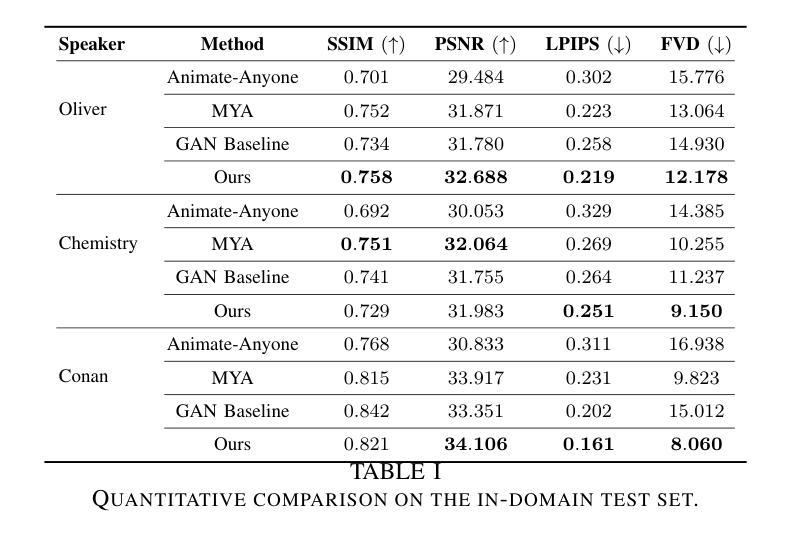
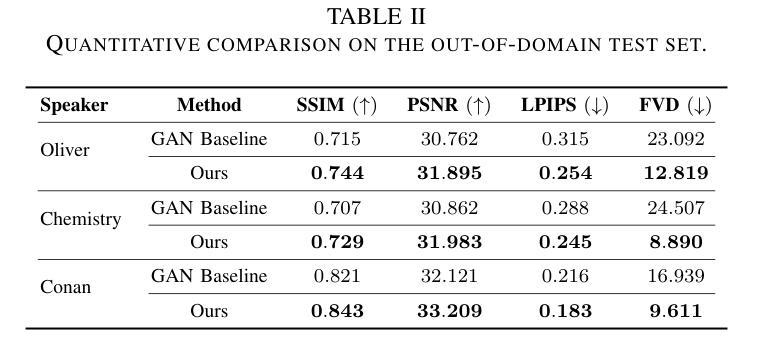
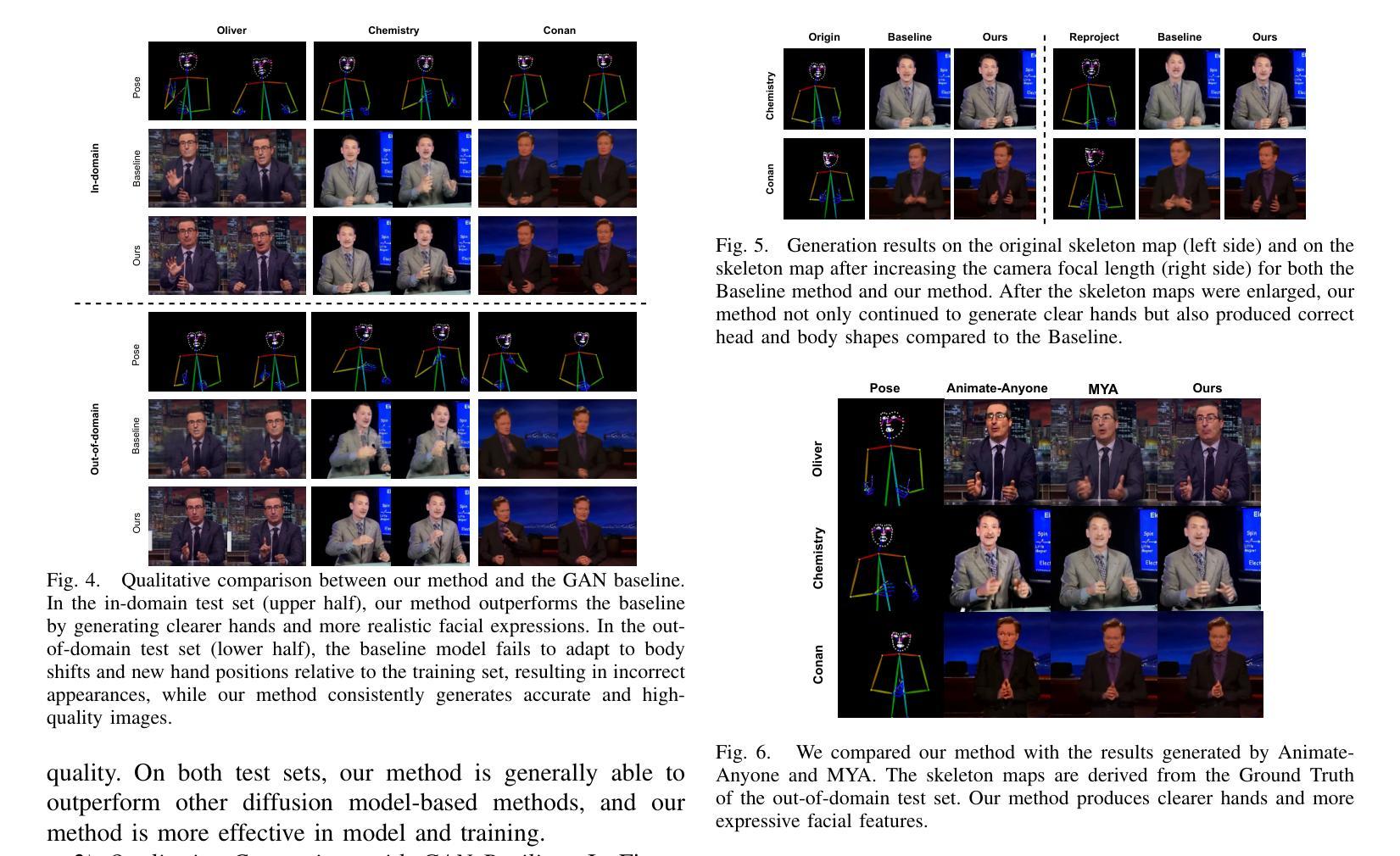
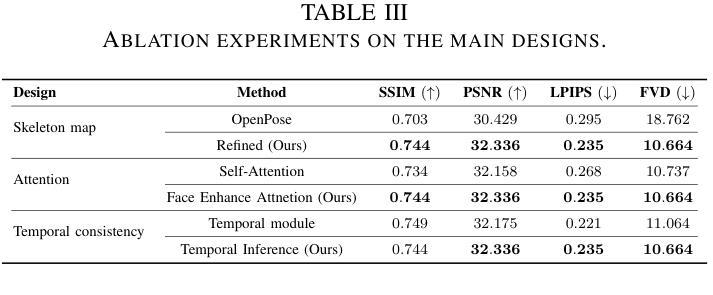