⚠️ 以下所有内容总结都来自于 大语言模型的能力,如有错误,仅供参考,谨慎使用
🔴 请注意:千万不要用于严肃的学术场景,只能用于论文阅读前的初筛!
💗 如果您觉得我们的项目对您有帮助 ChatPaperFree ,还请您给我们一些鼓励!⭐️ HuggingFace免费体验
2025-04-16 更新
GAF: Gaussian Avatar Reconstruction from Monocular Videos via Multi-view Diffusion
Authors:Jiapeng Tang, Davide Davoli, Tobias Kirschstein, Liam Schoneveld, Matthias Niessner
We propose a novel approach for reconstructing animatable 3D Gaussian avatars from monocular videos captured by commodity devices like smartphones. Photorealistic 3D head avatar reconstruction from such recordings is challenging due to limited observations, which leaves unobserved regions under-constrained and can lead to artifacts in novel views. To address this problem, we introduce a multi-view head diffusion model, leveraging its priors to fill in missing regions and ensure view consistency in Gaussian splatting renderings. To enable precise viewpoint control, we use normal maps rendered from FLAME-based head reconstruction, which provides pixel-aligned inductive biases. We also condition the diffusion model on VAE features extracted from the input image to preserve facial identity and appearance details. For Gaussian avatar reconstruction, we distill multi-view diffusion priors by using iteratively denoised images as pseudo-ground truths, effectively mitigating over-saturation issues. To further improve photorealism, we apply latent upsampling priors to refine the denoised latent before decoding it into an image. We evaluate our method on the NeRSemble dataset, showing that GAF outperforms previous state-of-the-art methods in novel view synthesis. Furthermore, we demonstrate higher-fidelity avatar reconstructions from monocular videos captured on commodity devices.
我们提出了一种新的方法,可以从智能手机等普通设备捕获的单目视频中重建可动画的3D高斯化身。从这种记录中进行逼真的3D头部化身重建是一个挑战,因为观察有限,导致未观察到的区域约束不足,并且可能在新的视角中产生伪影。为了解决这个问题,我们引入了一个多视角头部扩散模型,利用先验知识来填充缺失的区域,并确保高斯平铺渲染中的视角一致性。为了实现精确的观点控制,我们使用基于FLAME的头部重建渲染的正态图,这提供了像素对齐的归纳偏见。我们还根据输入图像提取的VAE特征对扩散模型进行条件处理,以保留面部身份和外观细节。对于高斯化身重建,我们通过使用迭代去噪图像作为伪真实值来提炼多视角扩散先验知识,有效地减轻过饱和问题。为了进一步改善逼真度,我们应用潜在上采样先验知识来精细去噪潜在编码,然后将其解码为图像。我们在NeRSemble数据集上评估了我们的方法,结果表明GAF在新型视角合成方面优于以前的最先进方法。此外,我们还展示了从普通设备捕获的单目视频中的更高保真化身重建。
论文及项目相关链接
PDF Paper Video: https://youtu.be/QuIYTljvhyg Project Page: https://tangjiapeng.github.io/projects/GAF
Summary
本文提出一种新颖的方法,从普通设备如智能手机拍摄的单目视频中重建可动画的3D高斯头像。该方法通过引入多视角头部扩散模型解决从这种视频中重建逼真3D头像的挑战,确保在不同视角下的渲染一致性。此外,还利用基于FLAME的头部重建渲染的正常映射提供像素对齐的诱导偏见,以实现精确的视角控制。通过蒸馏多视角扩散先验,对迭代去噪图像作为伪真实值进行迭代优化,提高了高斯头像重建的光泽度。在NeRSemble数据集上的评估显示,GAF在新型视角合成方面优于先前的方法,并能从普通设备的单目视频中重建出更高质量的头像。
Key Takeaways
- 提出一种从单目视频重建3D高斯头像的新方法。
- 通过引入多视角头部扩散模型解决重建挑战。
- 利用正常映射实现精确的视角控制。
- 通过迭代去噪图像作为伪真实值进行多视角扩散先验蒸馏。
- 利用潜在上采样先验对去噪潜在进行细化,提高重建的光泽度。
- 在NeRSemble数据集上的评估显示GAF方法优于先前的方法。
点此查看论文截图
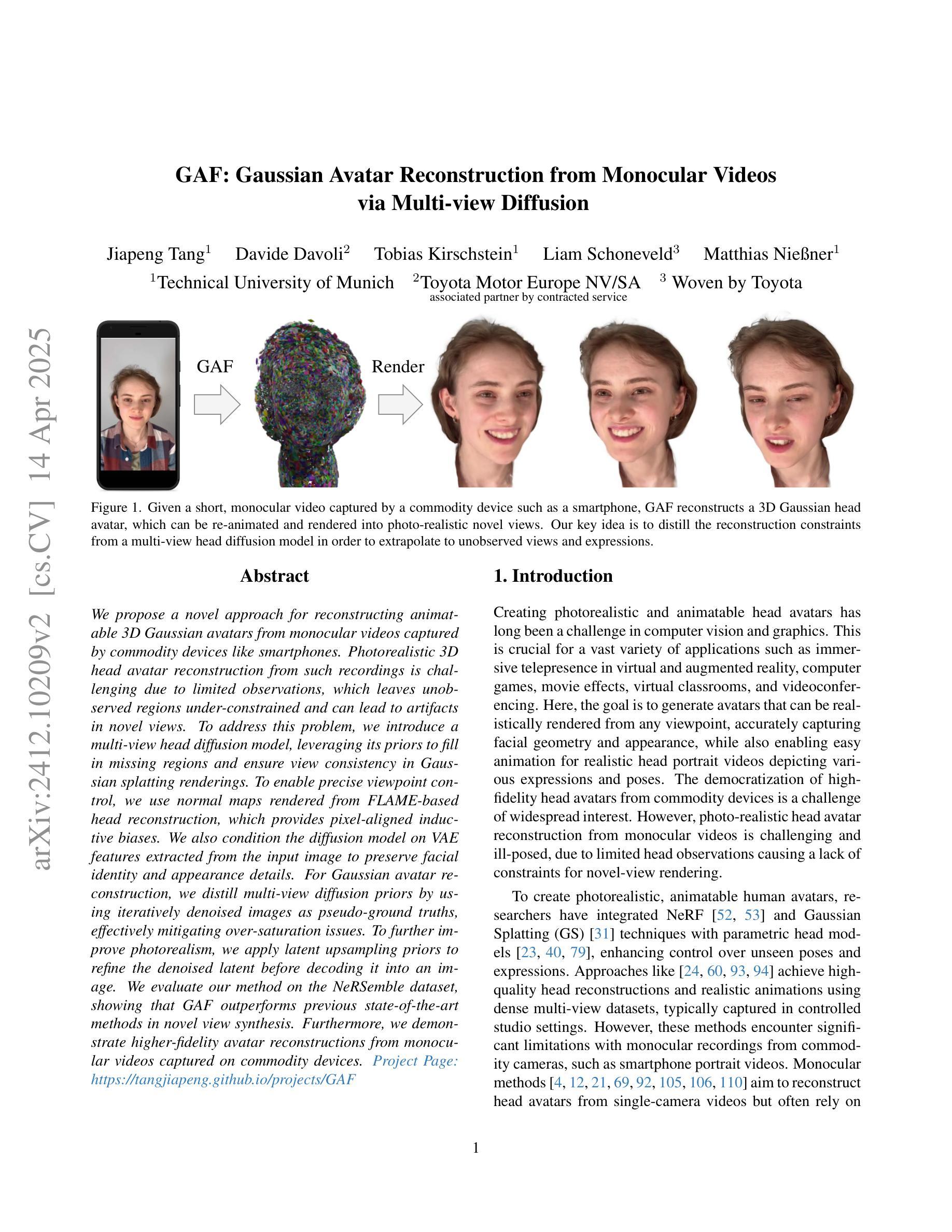
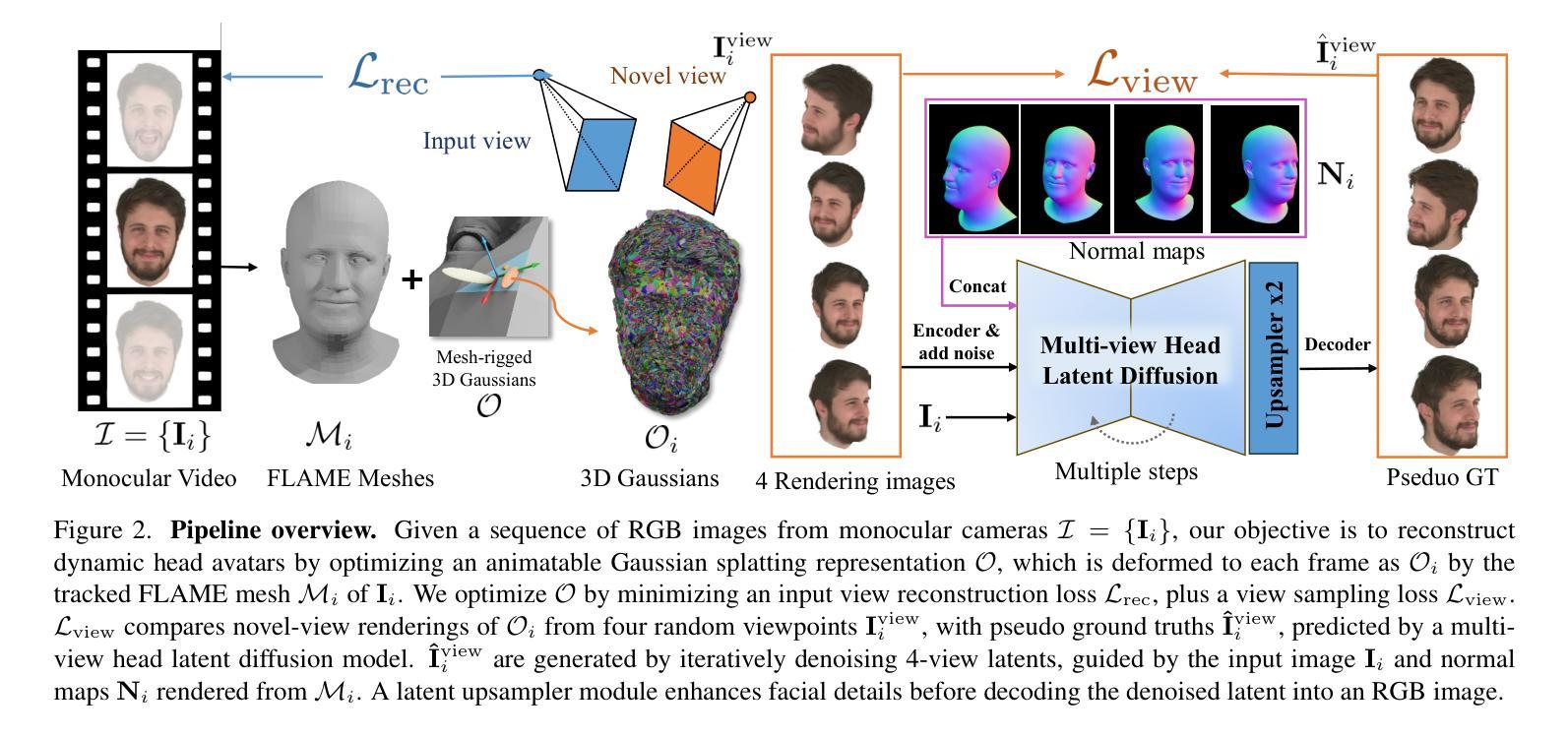
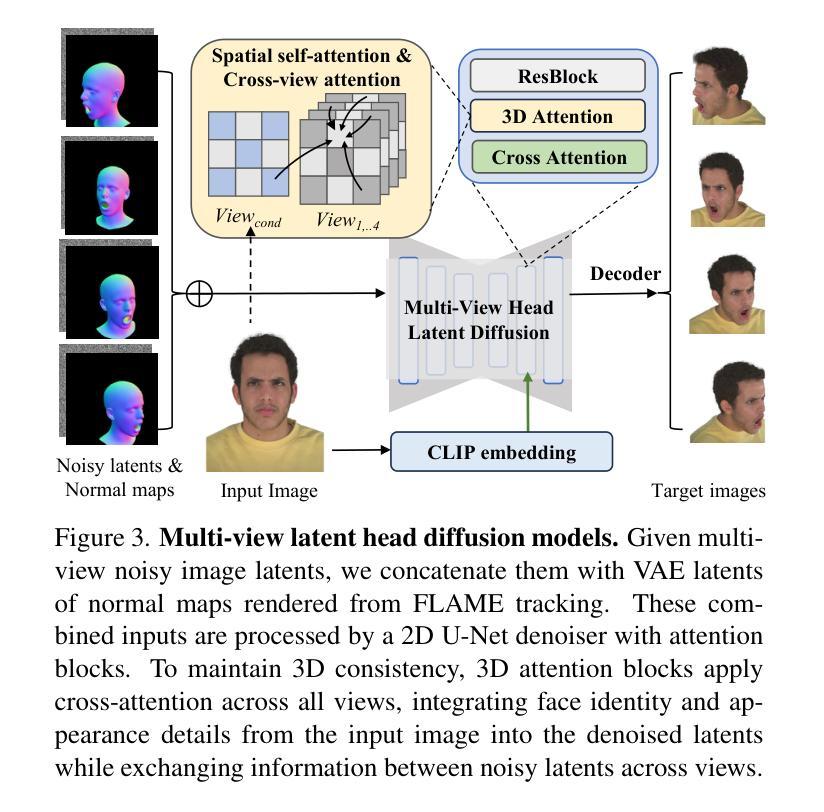
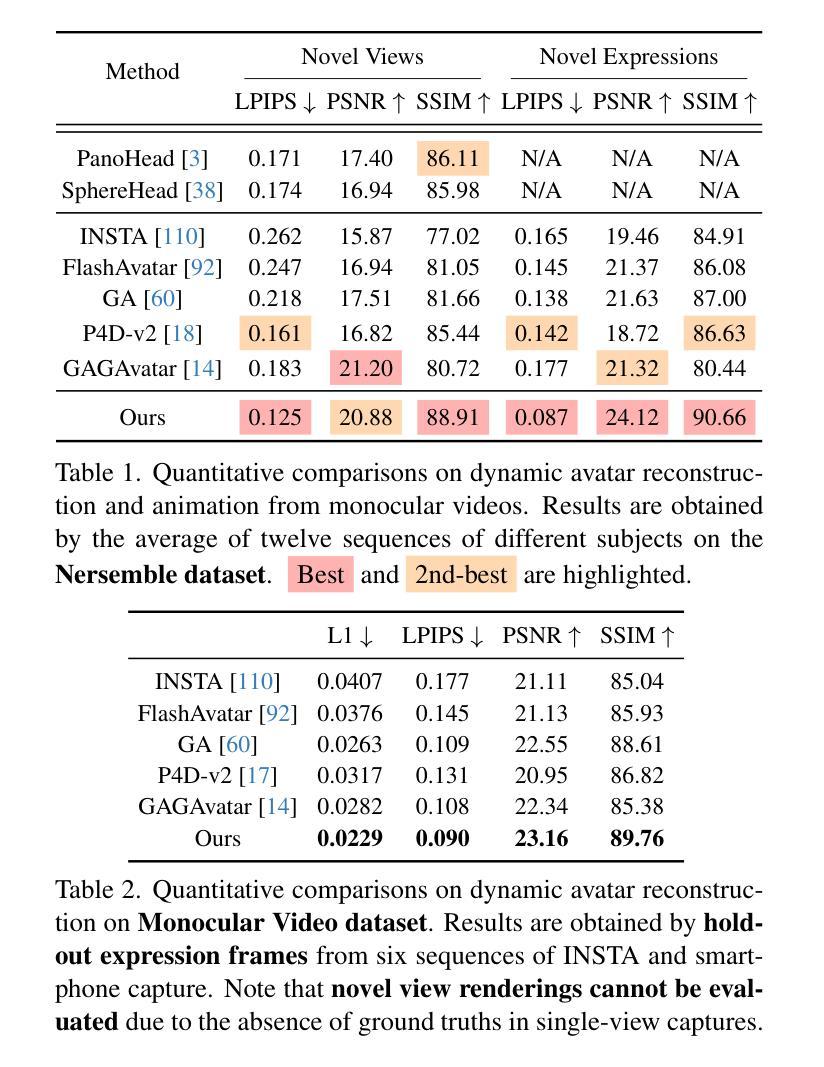