⚠️ 以下所有内容总结都来自于 大语言模型的能力,如有错误,仅供参考,谨慎使用
🔴 请注意:千万不要用于严肃的学术场景,只能用于论文阅读前的初筛!
💗 如果您觉得我们的项目对您有帮助 ChatPaperFree ,还请您给我们一些鼓励!⭐️ HuggingFace免费体验
2025-04-16 更新
VideoAds for Fast-Paced Video Understanding: Where Opensource Foundation Models Beat GPT-4o & Gemini-1.5 Pro
Authors:Zheyuan Zhang, Monica Dou, Linkai Peng, Hongyi Pan, Ulas Bagci, Boqing Gong
Advertisement videos serve as a rich and valuable source of purpose-driven information, encompassing high-quality visual, textual, and contextual cues designed to engage viewers. They are often more complex than general videos of similar duration due to their structured narratives and rapid scene transitions, posing significant challenges to multi-modal large language models (MLLMs). In this work, we introduce VideoAds, the first dataset tailored for benchmarking the performance of MLLMs on advertisement videos. VideoAds comprises well-curated advertisement videos with complex temporal structures, accompanied by \textbf{manually} annotated diverse questions across three core tasks: visual finding, video summary, and visual reasoning. We propose a quantitative measure to compare VideoAds against existing benchmarks in terms of video complexity. Through extensive experiments, we find that Qwen2.5-VL-72B, an opensource MLLM, achieves 73.35% accuracy on VideoAds, outperforming GPT-4o (66.82%) and Gemini-1.5 Pro (69.66%); the two proprietary models especially fall behind the opensource model in video summarization and reasoning, but perform the best in visual finding. Notably, human experts easily achieve a remarkable accuracy of 94.27%. These results underscore the necessity of advancing MLLMs’ temporal modeling capabilities and highlight VideoAds as a potentially pivotal benchmark for future research in understanding video that requires high FPS sampling. The dataset and evaluation code will be publicly available at https://videoadsbenchmark.netlify.app.
广告视频作为目的驱动信息的丰富且宝贵的来源,包含了为吸引观众而设计的高质量视觉、文本和上下文线索。由于它们具有结构化的叙事和快速的场景转换,往往比类似时长的普通视频更加复杂,这给多模态大型语言模型(MLLMs)带来了重大挑战。在这项工作中,我们推出了VideoAds,这是专门为广告视频评估MLLM性能而定制的第一个数据集。VideoAds包含了精心挑选的具有复杂时间结构的广告视频,以及针对三个核心任务的多样化手动注释问题:视觉寻找、视频摘要和视觉推理。我们提出了一种定量度量标准,将VideoAds与现有基准测试在视频复杂度方面进行比较。通过广泛的实验,我们发现开源MLLM Qwen2.5-VL-72B在VideoAds上达到了73.35%的准确率,超过了GPT-4o(66.82%)和Gemini-1.5 Pro(69.66%);这两个专有模型尤其在视频摘要和推理方面落后于开源模型,但在视觉寻找方面表现最佳。值得注意的是,人类专家很容易达到94.27%的准确率。这些结果强调了提高MLLMs的时间建模能力的必要性,并突出了VideoAds作为未来研究理解视频的高FPS采样关键基准测试的潜在重要性。数据集和评估代码将在https://videoadsbenchmark.netlify.app公开提供。
论文及项目相关链接
Summary:
广告视频作为目的驱动信息的丰富来源,包含高质量视觉、文本和上下文线索,旨在吸引观众。由于其结构化的叙事和快速的场景转换,广告视频对多模态大型语言模型(MLLMs)构成重大挑战。本研究推出VideoAds数据集,专为评估MLLMs在广告视频上的性能而设计。VideoAds包含精心挑选的广告视频,具有复杂的时序结构,并配有针对三项核心任务的手工标注多样化问题:视觉发现、视频摘要和视觉推理。我们提出一种定量度量标准,将VideoAds与现有基准进行比较,以评估视频复杂性。实验表明,开源MLLM Qwen2.5-VL-72B在VideoAds上表现最佳,准确率为73.35%,优于GPT-4o(66.82%)和Gemini-1.5 Pro(69.66%)。人类专家的准确率高达94.27%。这突显了提升MLLMs时序建模能力的必要性,并强调VideoAds作为未来研究视频理解的重要基准,尤其需要高FPS采样。数据集和评估代码将公开提供在https://videoadsbenchmark.netlify.app。
Key Takeaways:
- 广告视频包含丰富视觉、文本和上下文信息,对语言模型提出挑战。
- VideoAds数据集专为评估MLLMs在广告视频上的性能而设计,包含复杂时序结构的广告视频及手工标注的问题。
- 定量度量标准用于评估视频复杂性。
- 开源MLLM Qwen2.5-VL-72B在VideoAds上表现最佳,准确率为73.35%。
- 人类专家准确率高达94.27%,突显提升MLLMs时序建模能力的必要性。
- VideoAds作为未来研究视频理解的重要基准,尤其需要高FPS采样。
点此查看论文截图
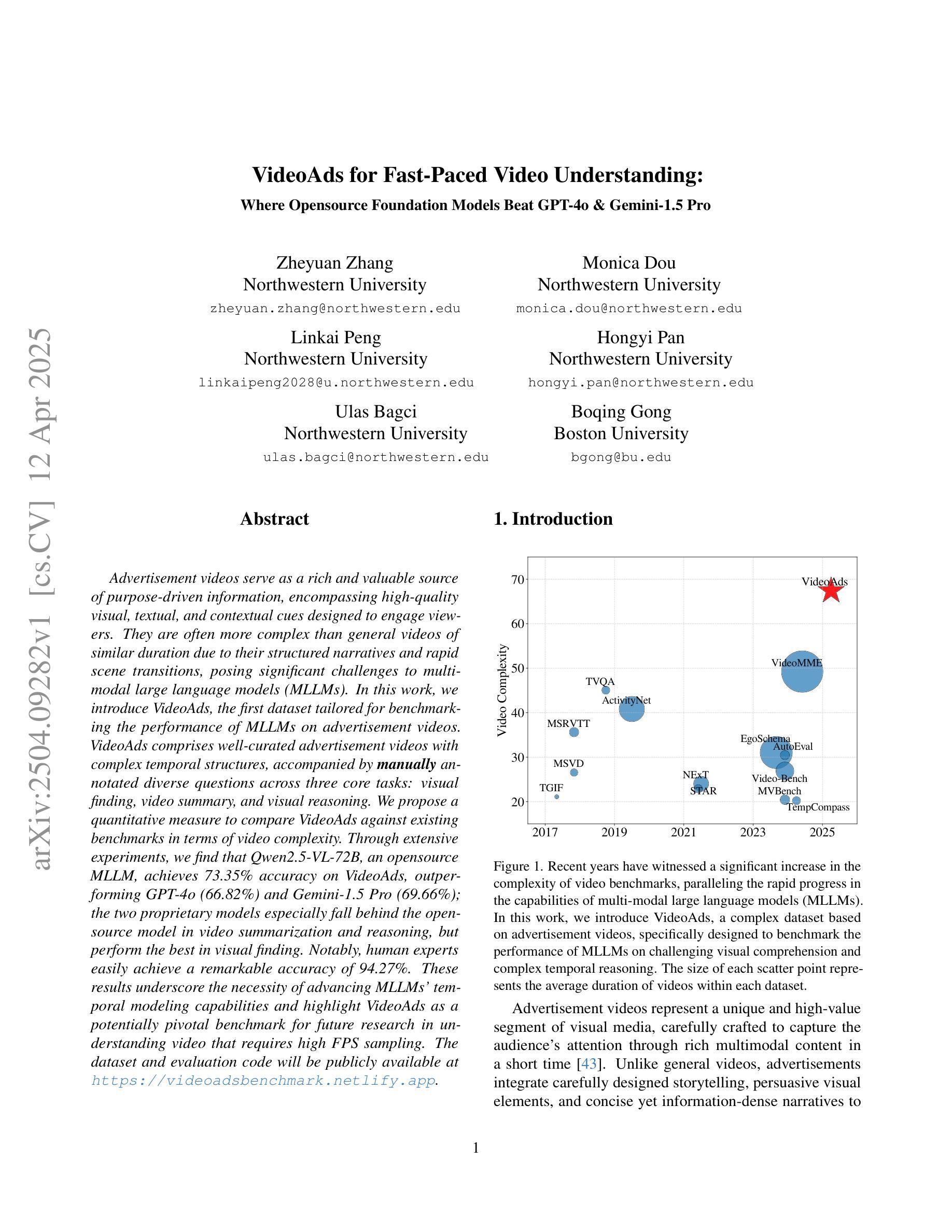
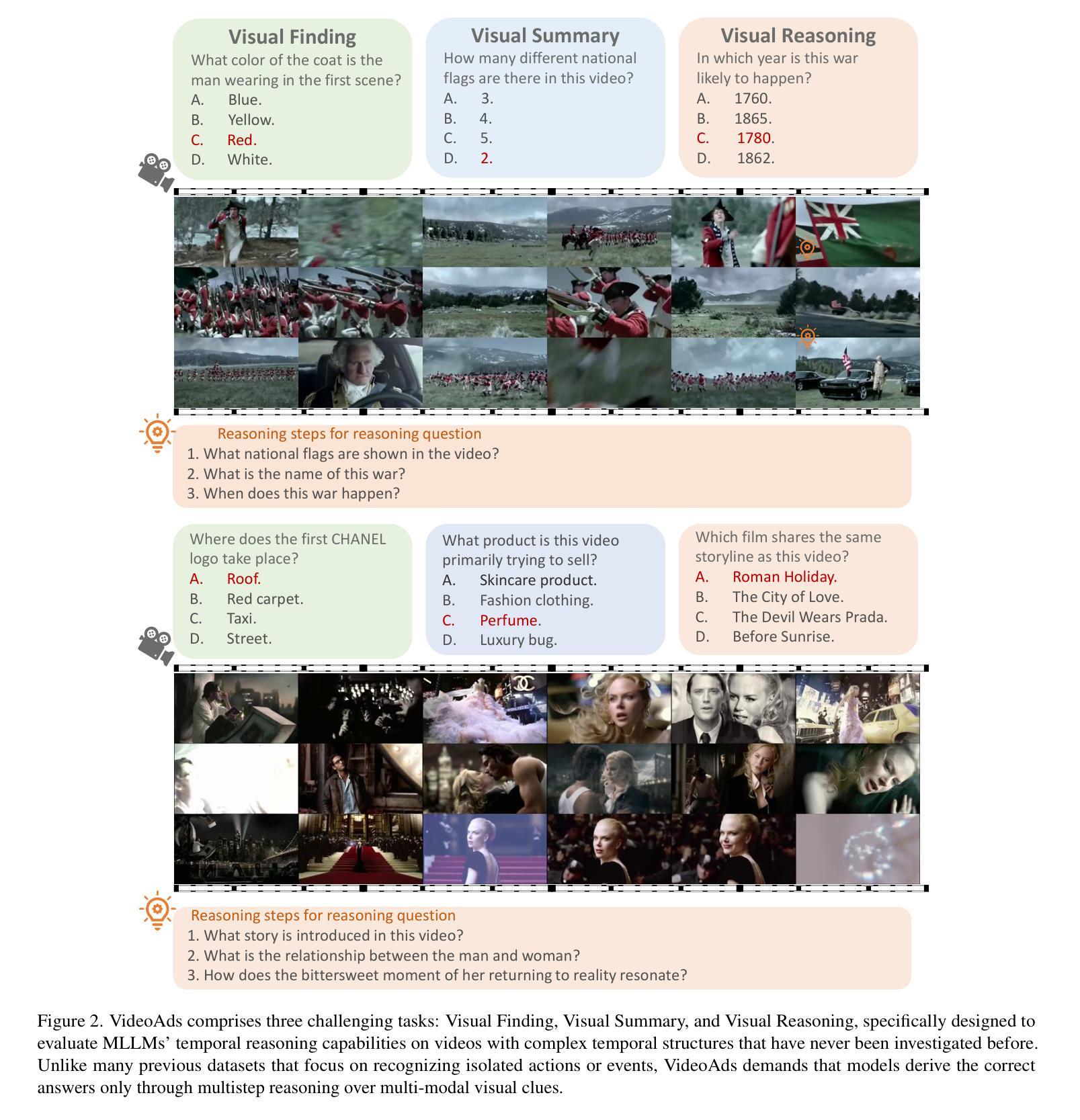
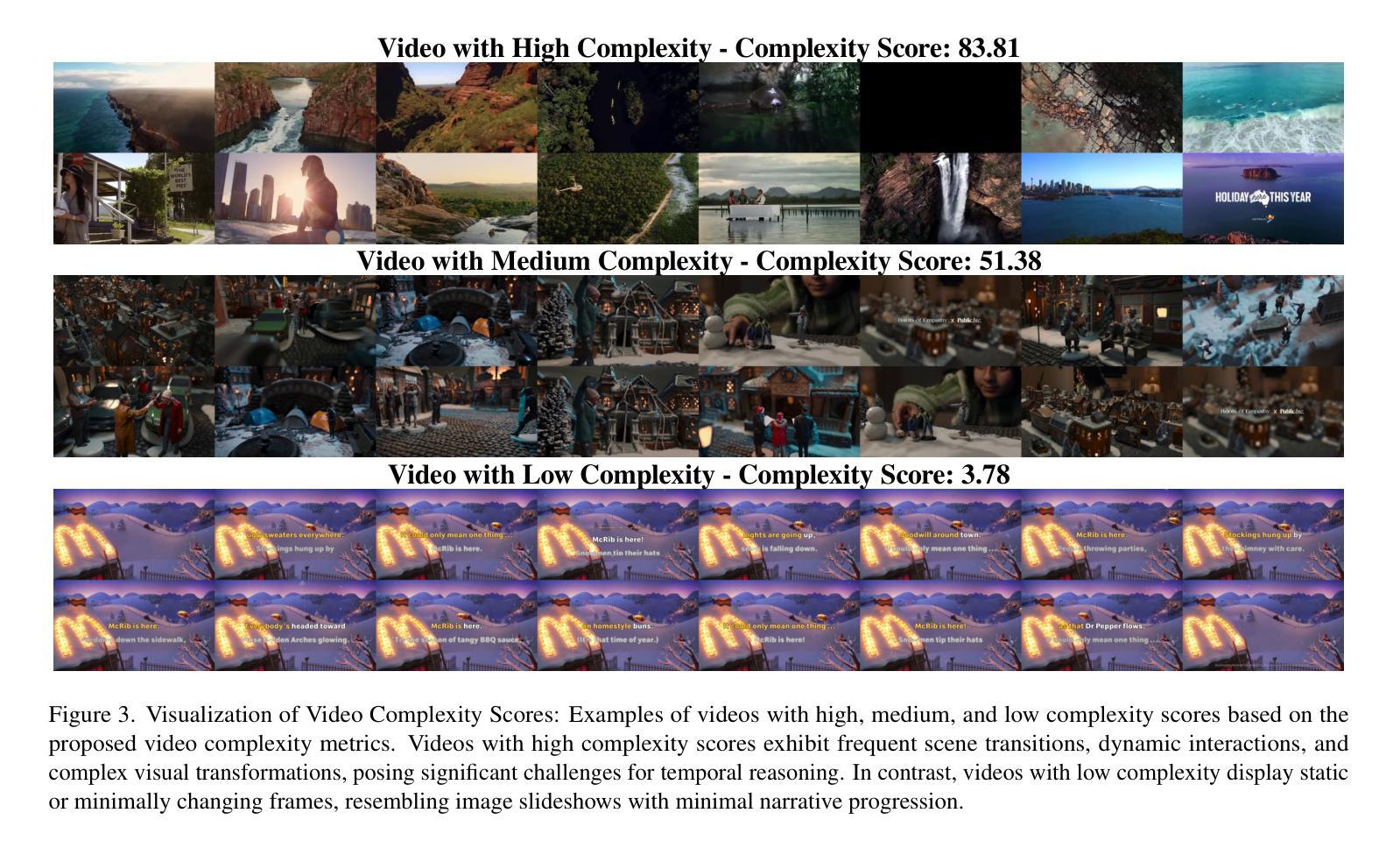
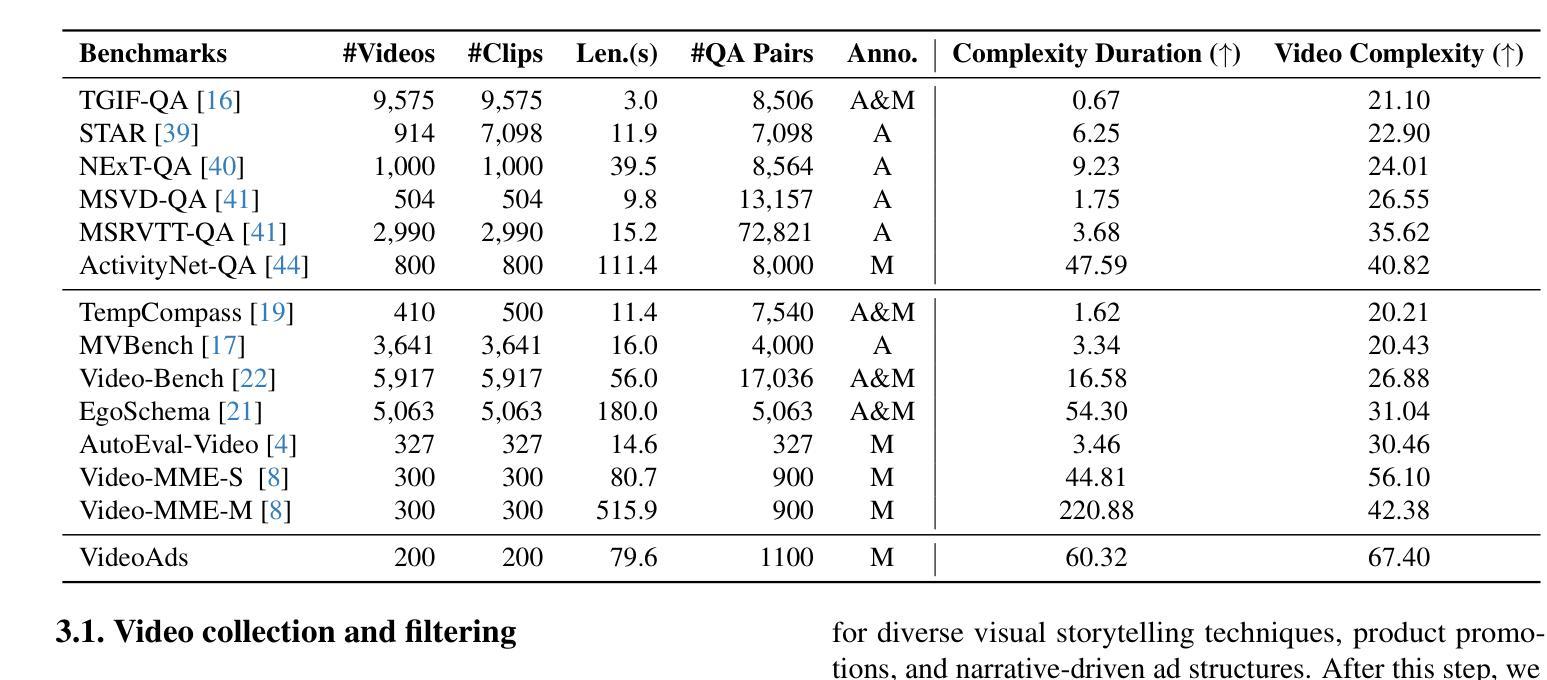
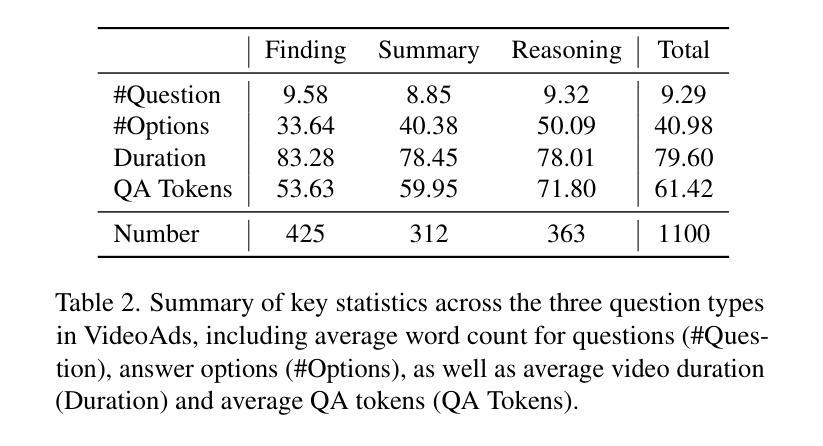