⚠️ 以下所有内容总结都来自于 大语言模型的能力,如有错误,仅供参考,谨慎使用
🔴 请注意:千万不要用于严肃的学术场景,只能用于论文阅读前的初筛!
💗 如果您觉得我们的项目对您有帮助 ChatPaperFree ,还请您给我们一些鼓励!⭐️ HuggingFace免费体验
2025-04-16 更新
Progressive Transfer Learning for Multi-Pass Fundus Image Restoration
Authors:Uyen Phan, Ozer Can Devecioglu, Serkan Kiranyaz, Moncef Gabbouj
Diabetic retinopathy is a leading cause of vision impairment, making its early diagnosis through fundus imaging critical for effective treatment planning. However, the presence of poor quality fundus images caused by factors such as inadequate illumination, noise, blurring and other motion artifacts yields a significant challenge for accurate DR screening. In this study, we propose progressive transfer learning for multi pass restoration to iteratively enhance the quality of degraded fundus images, ensuring more reliable DR screening. Unlike previous methods that often focus on a single pass restoration, multi pass restoration via PTL can achieve a superior blind restoration performance that can even improve most of the good quality fundus images in the dataset. Initially, a Cycle GAN model is trained to restore low quality images, followed by PTL induced restoration passes over the latest restored outputs to improve overall quality in each pass. The proposed method can learn blind restoration without requiring any paired data while surpassing its limitations by leveraging progressive learning and fine tuning strategies to minimize distortions and preserve critical retinal features. To evaluate PTL’s effectiveness on multi pass restoration, we conducted experiments on DeepDRiD, a large scale fundus imaging dataset specifically curated for diabetic retinopathy detection. Our result demonstrates state of the art performance, showcasing PTL’s potential as a superior approach to iterative image quality restoration.
糖尿病视网膜病变是导致视力受损的主要原因,因此通过眼底成像进行早期诊断对于有效治疗计划至关重要。然而,由于照明不足、噪声、模糊以及其他运动伪影等因素导致的低质量眼底图像的存在,为准确的糖尿病视网膜病变(DR)筛查带来了重大挑战。在本研究中,我们提出了基于渐进式迁移学习的多通道修复方法,以迭代方式提高退化眼底图像的质量,确保更可靠的DR筛查。与之前经常专注于单通道修复的方法不同,通过渐进式迁移学习(PTL)的多通道修复可以实现出色的盲修复性能,甚至可以改进数据集中大多数高质量的眼底图像。首先,训练一个Cycle GAN模型来修复低质量的图像,然后对最新修复的输出来执行PTL引导的恢复通道,以在每次迭代中提高整体质量。所提出的方法可以学习盲修复,而无需任何配对的数据,同时通过利用渐进式学习和微调策略来超越其局限性,以最小化失真并保留关键的视网膜特征。为了评估PTL在多通道修复上的有效性,我们在DeepDRiD上进行了实验,这是一个专门为糖尿病视网膜病变检测整理的大规模眼底图像数据集。我们的结果展示了最先进的性能,展示了PTL作为迭代图像质量恢复的潜在方法的优势。
论文及项目相关链接
PDF 13 pages, 12 figures including appendix
Summary
本文提出一种基于渐进式迁移学习(PTL)的多通道恢复方法,用于增强退化眼底图像的质量,以提高糖尿病视网膜病变(DR)筛查的可靠性。通过训练Cycle GAN模型恢复低质量图像,并利用PTL进行多次恢复,改善整体质量。该方法可在无需配对数据的情况下实现盲恢复,并借助渐进学习和微调策略最小化失真,保留关键视网膜特征。在DeepDRiD数据集上的实验结果表明,该方法在眼底图像恢复上具有卓越性能。
Key Takeaways
- 糖尿病视网膜病变是导致视力受损的主要原因之一,早期通过眼底成像进行诊断对有效治疗至关重要。
- 眼底图像质量差给准确DR筛查带来挑战,本研究提出基于渐进式迁移学习(PTL)的多通道恢复方法。
- 该方法利用Cycle GAN模型初始恢复低质量图像,随后通过PTL进行多次恢复改善整体质量。
- 方法可在无需配对数据的情况下实现盲恢复。
- 利用渐进学习和微调策略以最小化图像恢复过程中的失真,并保持视网膜特征。
- 在DeepDRiD数据集上的实验验证了该方法在眼底图像恢复上的卓越性能。
点此查看论文截图
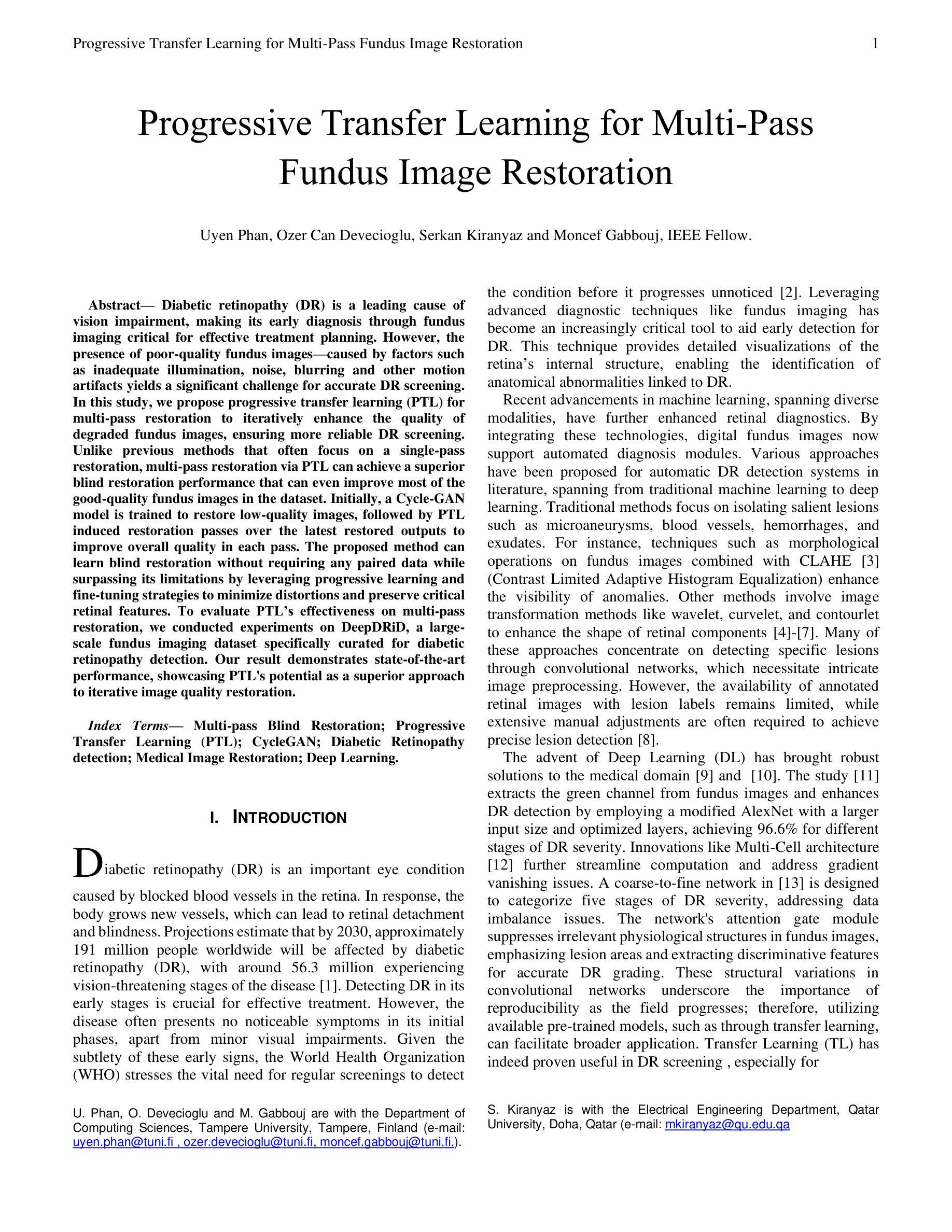
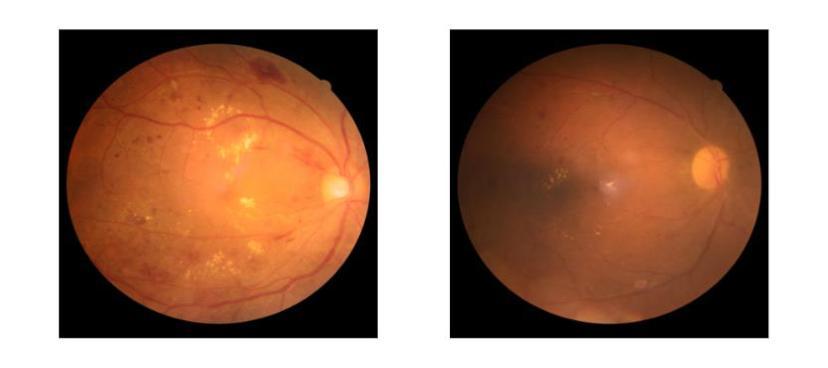
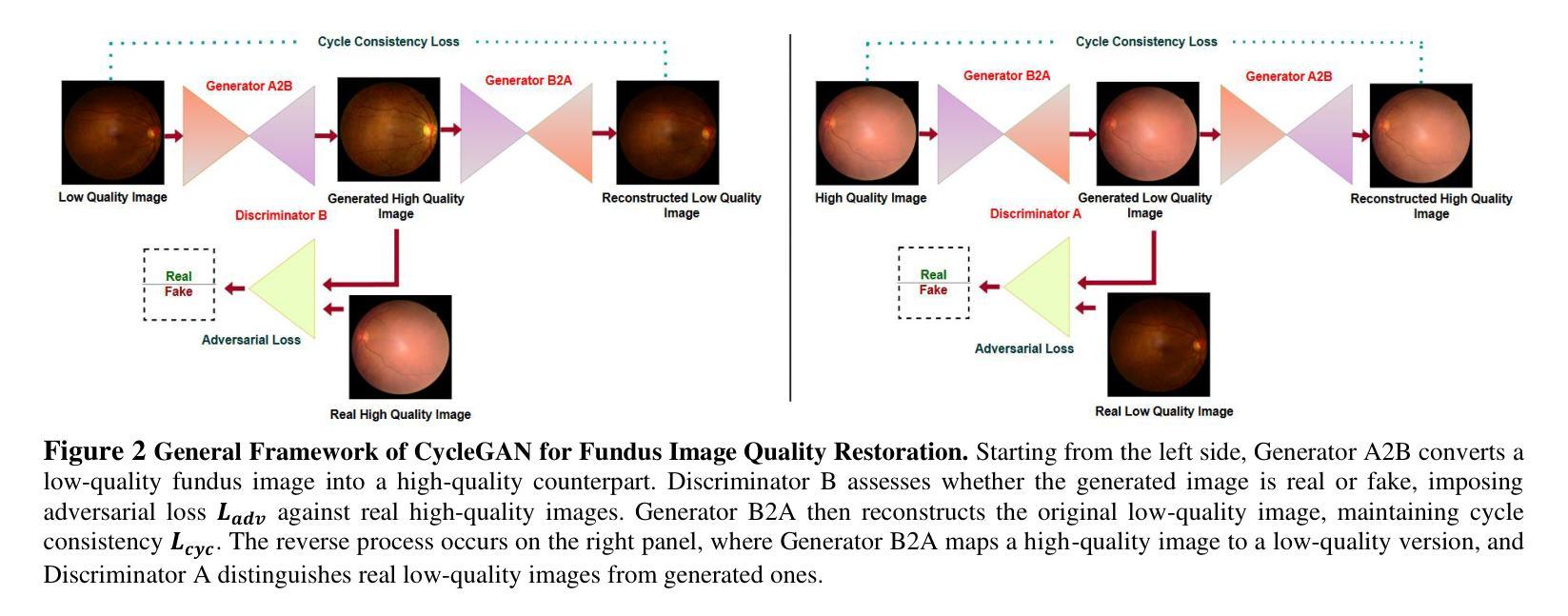
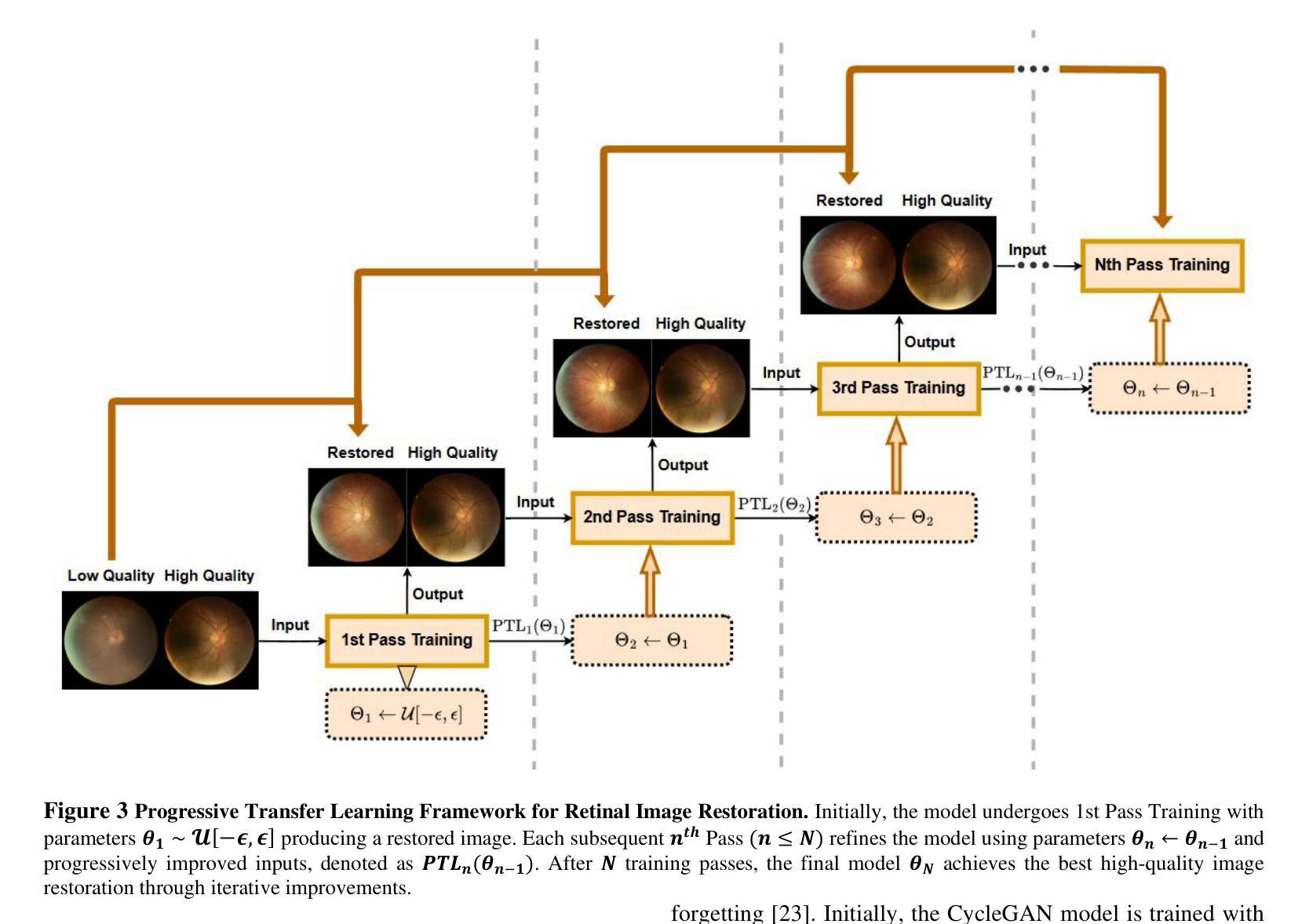
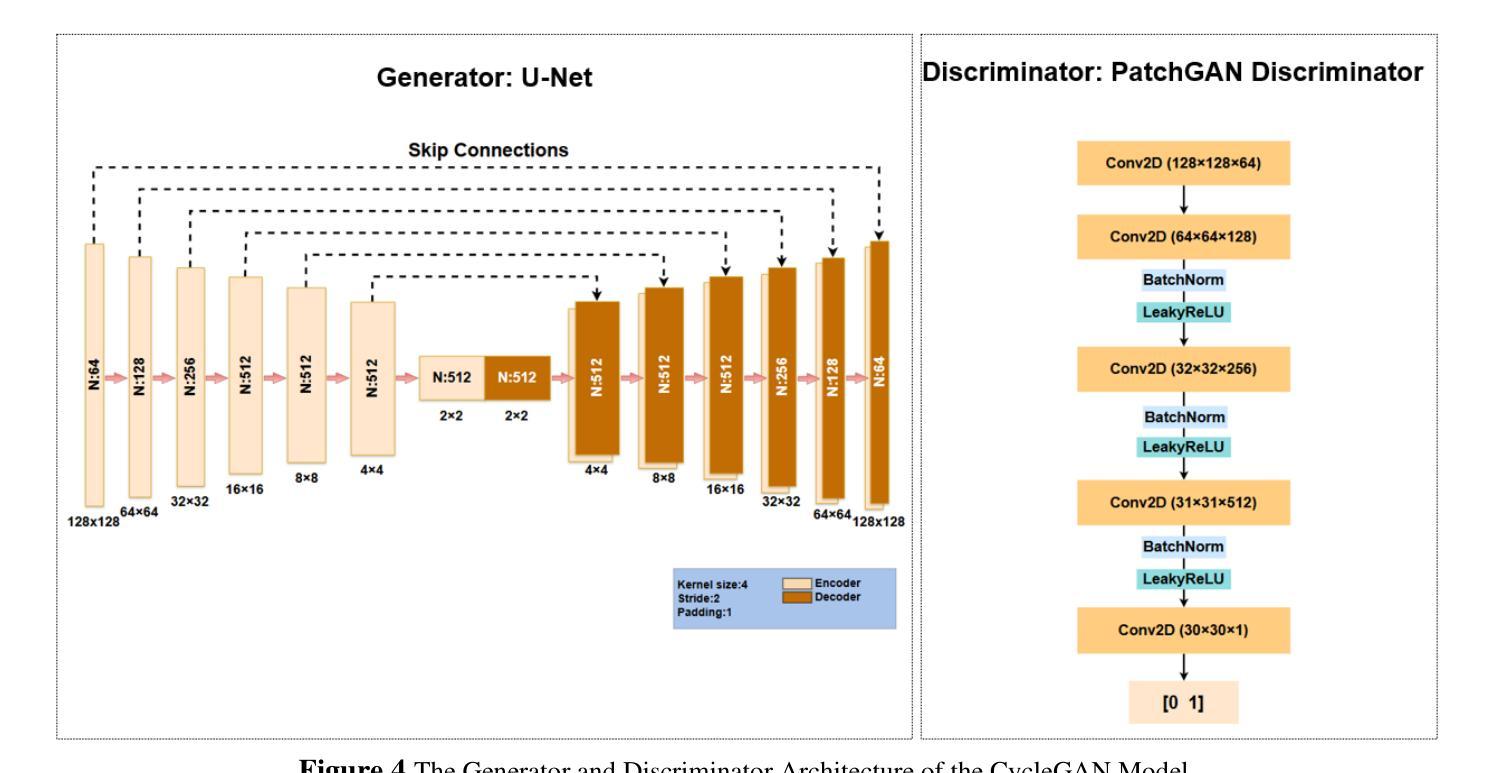
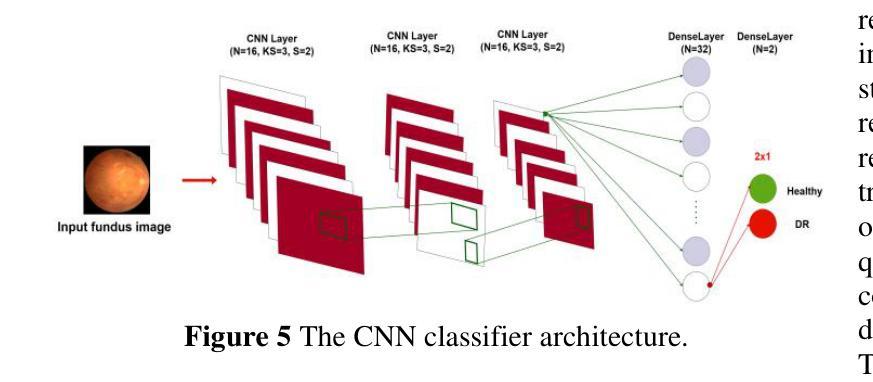
Distilling Textual Priors from LLM to Efficient Image Fusion
Authors:Ran Zhang, Xuanhua He, Ke Cao, Liu Liu, Li Zhang, Man Zhou, Jie Zhang
Multi-modality image fusion aims to synthesize a single, comprehensive image from multiple source inputs. Traditional approaches, such as CNNs and GANs, offer efficiency but struggle to handle low-quality or complex inputs. Recent advances in text-guided methods leverage large model priors to overcome these limitations, but at the cost of significant computational overhead, both in memory and inference time. To address this challenge, we propose a novel framework for distilling large model priors, eliminating the need for text guidance during inference while dramatically reducing model size. Our framework utilizes a teacher-student architecture, where the teacher network incorporates large model priors and transfers this knowledge to a smaller student network via a tailored distillation process. Additionally, we introduce spatial-channel cross-fusion module to enhance the model’s ability to leverage textual priors across both spatial and channel dimensions. Our method achieves a favorable trade-off between computational efficiency and fusion quality. The distilled network, requiring only 10% of the parameters and inference time of the teacher network, retains 90% of its performance and outperforms existing SOTA methods. Extensive experiments demonstrate the effectiveness of our approach. The implementation will be made publicly available as an open-source resource.
多模态图像融合旨在从多个源输入中合成一个单一、全面的图像。传统方法,如卷积神经网络和生成对抗网络,虽然效率高,但在处理低质量或复杂输入时遇到困难。最近的文本引导方法利用大型模型的先验知识来克服这些限制,但这需要内存和推理时间上的大量计算开销。为了应对这一挑战,我们提出了一种新型的大型模型先验知识提炼框架,该框架在推理过程中无需文本引导,同时能大幅度减小模型尺寸。我们的框架采用师徒架构,其中教师网络融入大型模型的先验知识,并通过定制提炼过程将这些知识转移到一个较小的学生网络。此外,我们引入了空间通道交叉融合模块,增强模型在空间和通道维度上利用文本先验知识的能力。我们的方法在计算效率和融合质量之间达到了有利的平衡。蒸馏网络仅需要教师网络10%的参数和推理时间,保留了90%的性能,并超越了现有的最先进方法。大量实验证明了我们的方法的有效性。该实现将作为开源资源公开发布。
论文及项目相关链接
Summary
本文提出了一种新的多模态图像融合框架,通过蒸馏大型模型先验知识,在不需要文本指导的情况下,显著减少了模型大小。该框架采用教师-学生架构,教师网络融入大型模型先验知识,并通过定制蒸馏过程将这些知识传递给较小的学生网络。此外,引入了空间通道交叉融合模块,提高模型在空间和通道维度上利用文本先验的能力。该方法在计算效率和融合质量之间取得了良好的平衡。蒸馏网络仅需教师网络10%的参数和推理时间,就能保留90%的性能,并优于现有最先进的方法。
Key Takeaways
- 提出了一种新的多模态图像融合框架,能够合成单一、全面的图像。
- 通过蒸馏大型模型先验知识,减少了模型大小,并提高了计算效率。
- 教师-学生架构用于传递大型模型的先验知识到小型学生网络。
- 引入了空间通道交叉融合模块,提高了利用文本先验的能力。
- 蒸馏网络性能优异,仅需教师网络的10%参数和推理时间。
- 该方法实现了计算效率和融合质量的良好平衡。
点此查看论文截图
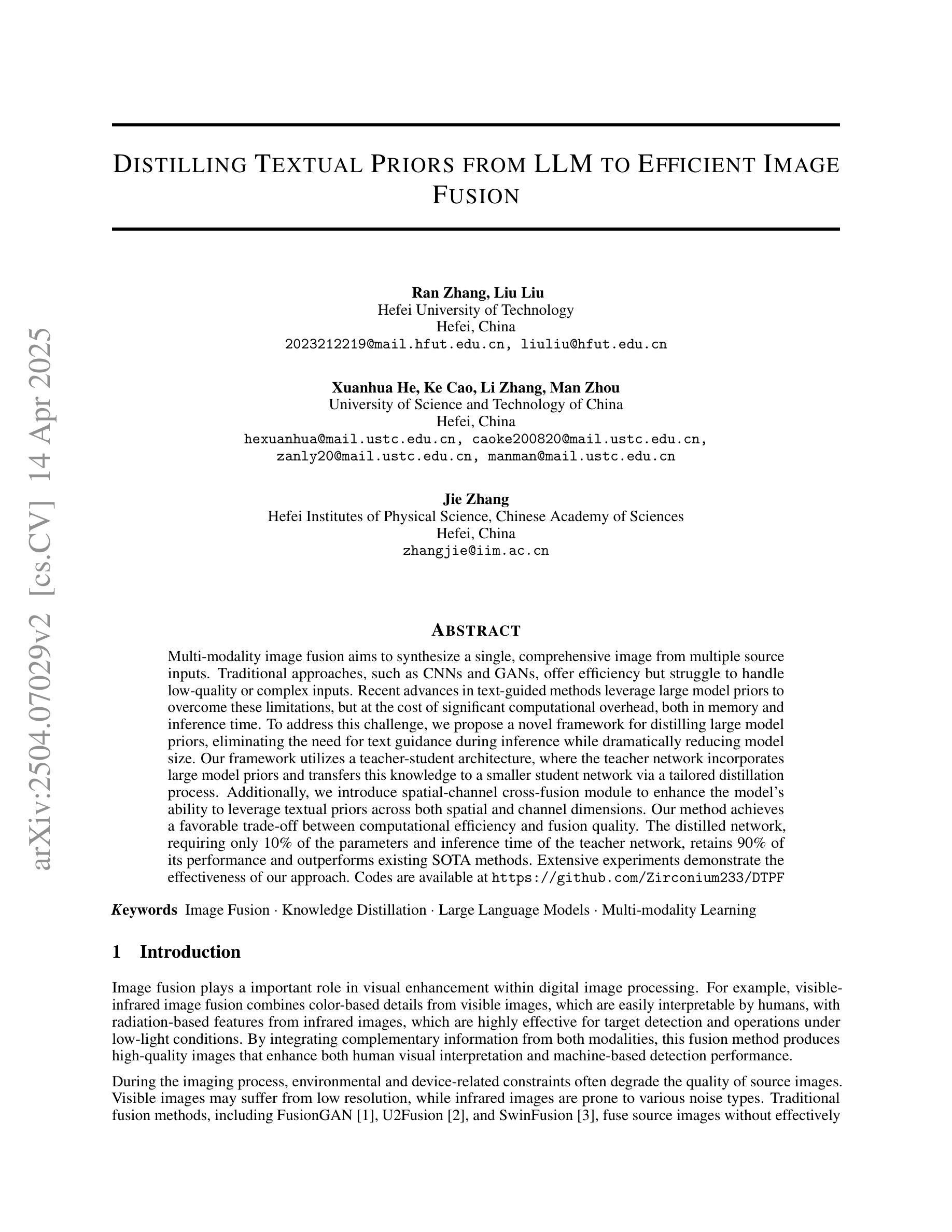
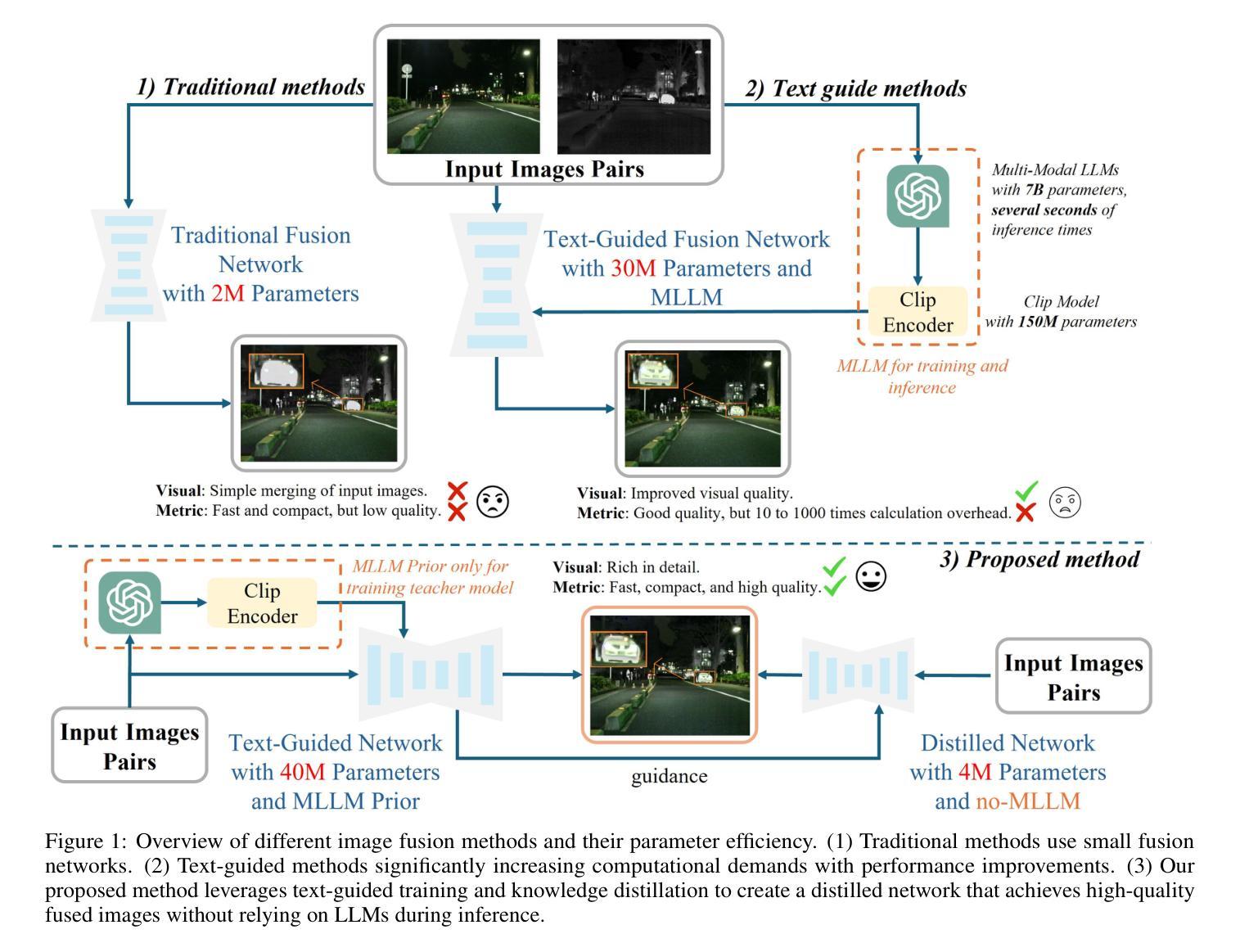
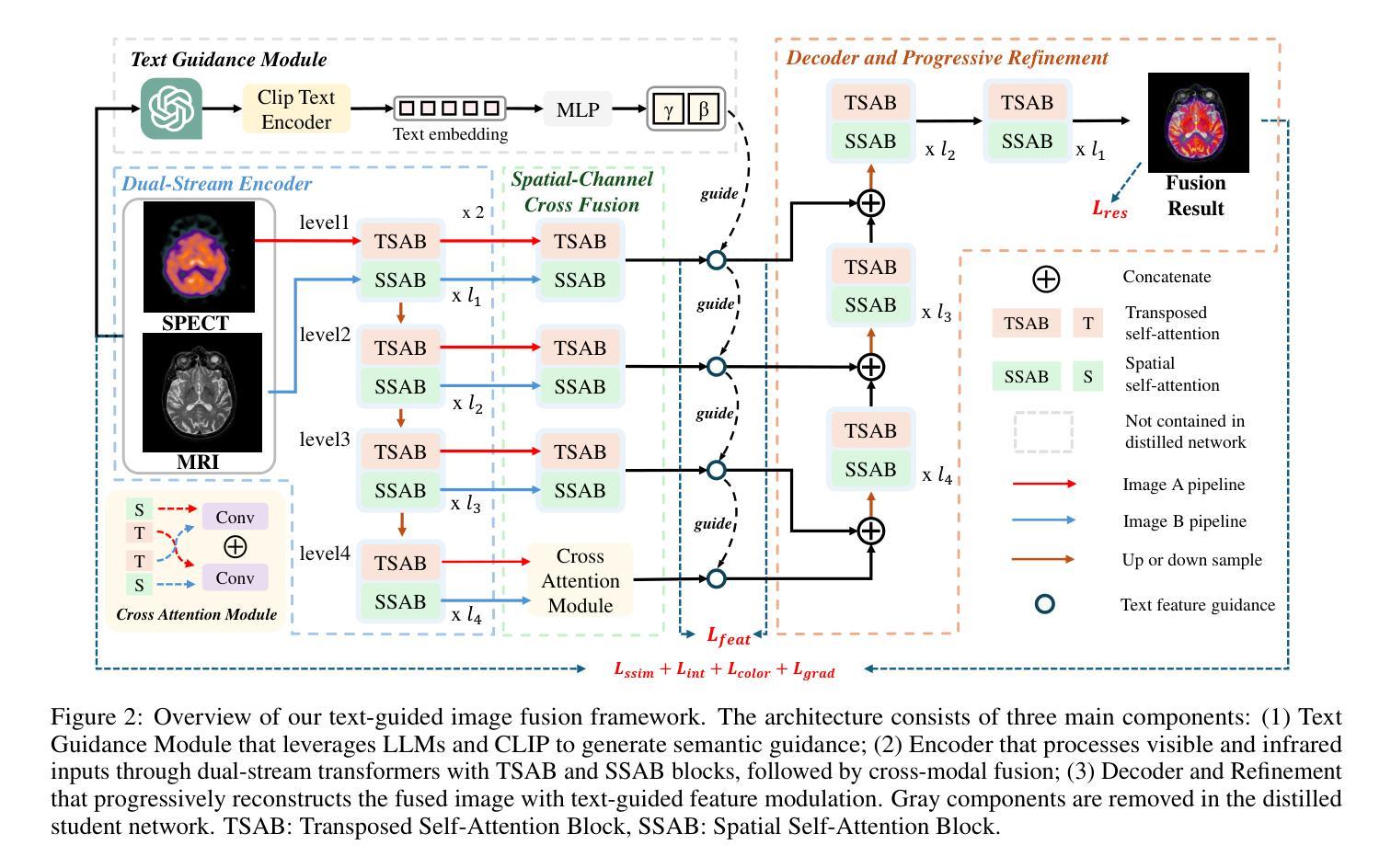
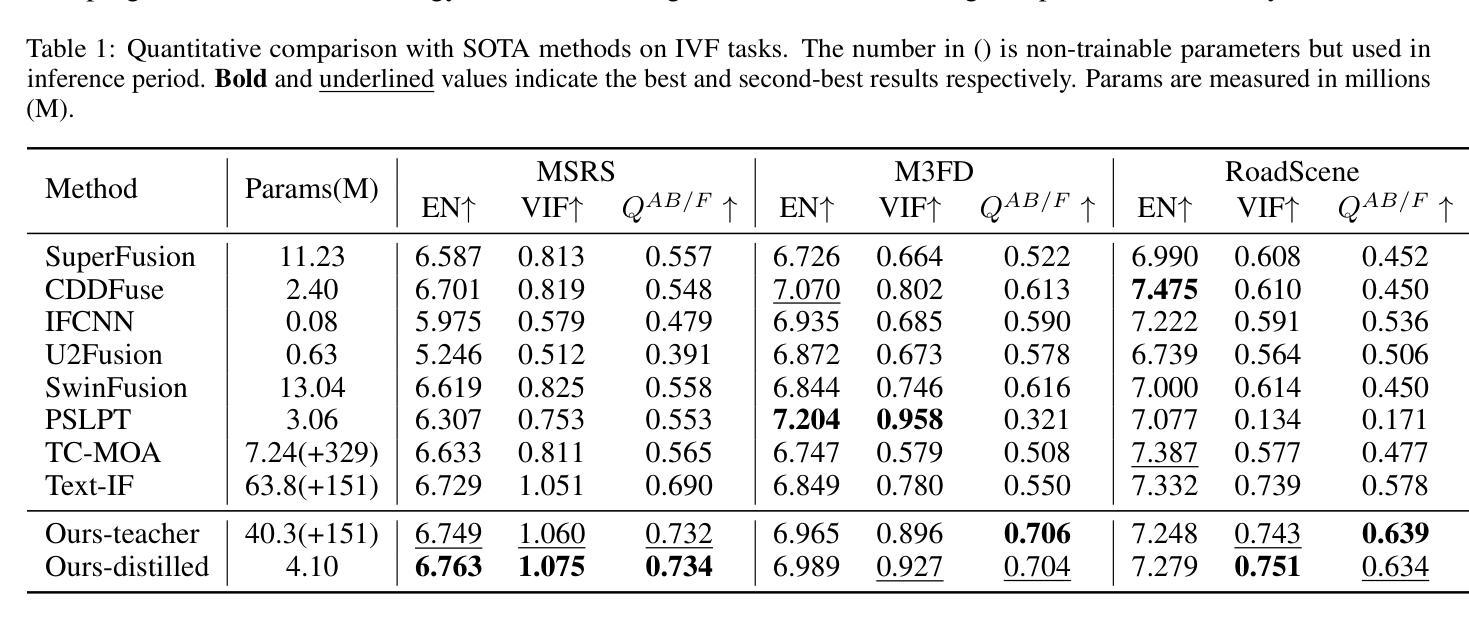