⚠️ 以下所有内容总结都来自于 大语言模型的能力,如有错误,仅供参考,谨慎使用
🔴 请注意:千万不要用于严肃的学术场景,只能用于论文阅读前的初筛!
💗 如果您觉得我们的项目对您有帮助 ChatPaperFree ,还请您给我们一些鼓励!⭐️ HuggingFace免费体验
2025-04-16 更新
CliniChat: A Multi-Source Knowledge-Driven Framework for Clinical Interview Dialogue Reconstruction and Evaluation
Authors:Jing Chen, Zhihua Wei, Wei Zhang, Yingying Hu, Qiong Zhang
Large language models (LLMs) hold great promise for assisting clinical interviews due to their fluent interactive capabilities and extensive medical knowledge. However, the lack of high-quality interview dialogue data and widely accepted evaluation methods has significantly impeded this process. So we propose CliniChat, a framework that integrates multi-source knowledge to enable LLMs to simulate real-world clinical interviews. It consists of two modules: Clini-Recon and Clini-Eval, each responsible for reconstructing and evaluating interview dialogues, respectively. By incorporating three sources of knowledge, Clini-Recon transforms clinical notes into systematic, professional, and empathetic interview dialogues. Clini-Eval combines a comprehensive evaluation metric system with a two-phase automatic evaluation approach, enabling LLMs to assess interview performance like experts. We contribute MedQA-Dialog, a high-quality synthetic interview dialogue dataset, and CliniChatGLM, a model specialized for clinical interviews. Experimental results demonstrate that CliniChatGLM’s interview capabilities undergo a comprehensive upgrade, particularly in history-taking, achieving state-of-the-art performance.
大型语言模型(LLMs)由于其流畅的交互能力和丰富的医学知识,在辅助临床访谈方面具有巨大潜力。然而,缺乏高质量的访谈对话数据和广泛接受的评估方法,显著阻碍了这一进程。因此,我们提出了CliniChat框架,该框架整合了多源知识,使LLMs能够模拟现实世界的临床访谈。它包含两个模块:Clini-Recon和Clini-Eval,分别负责重建和评估访谈对话。Clini-Recon通过结合三种知识来源,将临床笔记转化为系统、专业且富有同情心的访谈对话。Clini-Eval结合了一个全面的评估指标系统和一个两阶段的自动评估方法,使LLMs能够像专家一样评估访谈表现。我们贡献了MedQA-Dialog这一高质量合成访谈对话数据集和专门用于临床访谈的CliniChatGLM模型。实验结果表明,CliniChatGLM的访谈能力得到了全面升级,特别是在病史采集方面取得了最新性能。
论文及项目相关链接
Summary
大型语言模型(LLMs)在临床访谈中有巨大的应用潜力,其流畅的交互能力和丰富的医学知识使其成为理想的选择。然而,由于缺乏高质量的访谈对话数据和广泛接受的评估方法,限制了其应用。为此,我们提出CliniChat框架,它通过整合多源知识,使LLMs能够模拟真实世界的临床访谈。该框架包括Clini-Recon和Clini-Eval两个模块,分别负责重建和评估访谈对话。CliniChat还贡献了MedQA-Dialog高质量合成访谈对话数据集和专用于临床访谈的CliniChatGLM模型。实验结果表明,CliniChatGLM的访谈能力得到全面提升,尤其在病史采集方面达到先进水平。
Key Takeaways
- 大型语言模型在临床访谈中有潜力,但需解决数据缺乏和评估方法缺失的问题。
- CliniChat框架通过整合多源知识模拟真实世界临床访谈。
- CliniChat包括Clini-Recon和Clini-Eval两个模块,分别负责重建和评估访谈对话。
- CliniChat贡献了MedQA-Dialog数据集,为临床访谈提供高质量合成对话数据。
- CliniChatGLM模型专用于临床访谈,实验结果表明其访谈能力有所提升。
- CliniChatGLM在病史采集方面表现尤为出色,达到先进水平。
点此查看论文截图
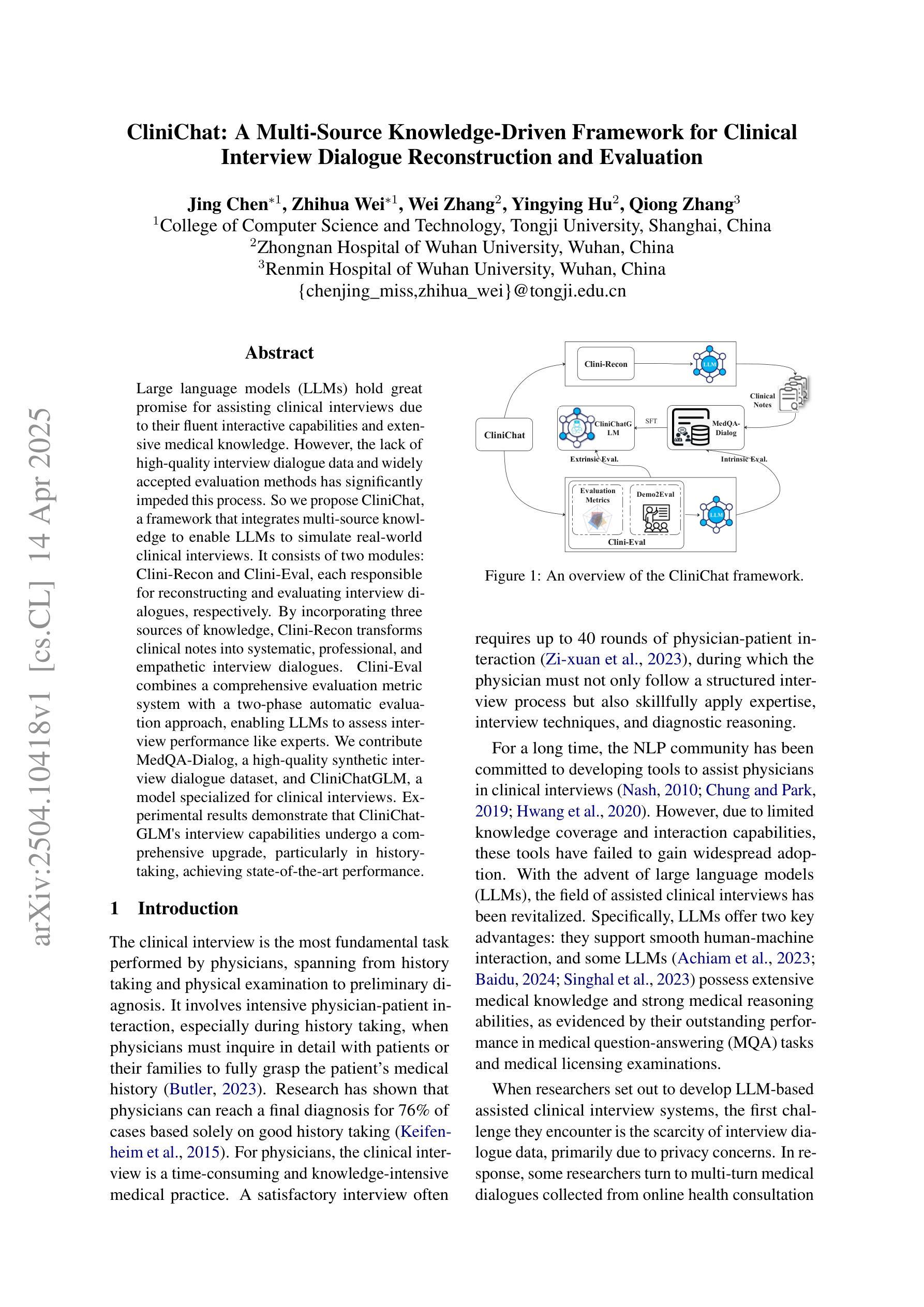
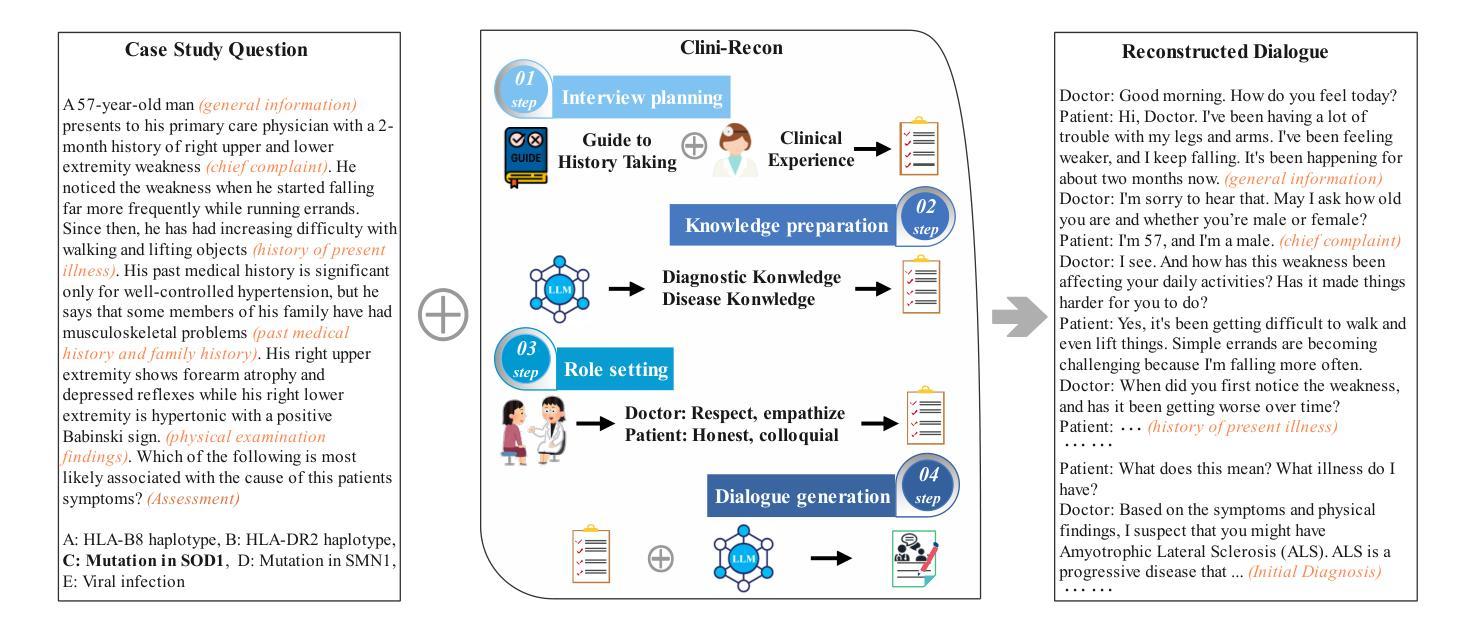
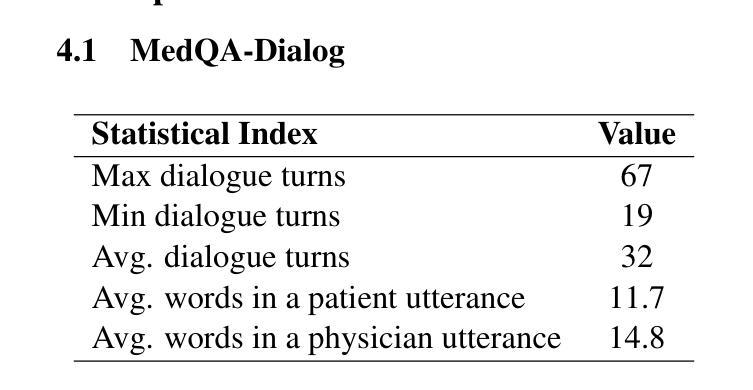