⚠️ 以下所有内容总结都来自于 大语言模型的能力,如有错误,仅供参考,谨慎使用
🔴 请注意:千万不要用于严肃的学术场景,只能用于论文阅读前的初筛!
💗 如果您觉得我们的项目对您有帮助 ChatPaperFree ,还请您给我们一些鼓励!⭐️ HuggingFace免费体验
2025-04-16 更新
MoLA: Motion Generation and Editing with Latent Diffusion Enhanced by Adversarial Training
Authors:Kengo Uchida, Takashi Shibuya, Yuhta Takida, Naoki Murata, Julian Tanke, Shusuke Takahashi, Yuki Mitsufuji
In text-to-motion generation, controllability as well as generation quality and speed has become increasingly critical. The controllability challenges include generating a motion of a length that matches the given textual description and editing the generated motions according to control signals, such as the start-end positions and the pelvis trajectory. In this paper, we propose MoLA, which provides fast, high-quality, variable-length motion generation and can also deal with multiple editing tasks in a single framework. Our approach revisits the motion representation used as inputs and outputs in the model, incorporating an activation variable to enable variable-length motion generation. Additionally, we integrate a variational autoencoder and a latent diffusion model, further enhanced through adversarial training, to achieve high-quality and fast generation. Moreover, we apply a training-free guided generation framework to achieve various editing tasks with motion control inputs. We quantitatively show the effectiveness of adversarial learning in text-to-motion generation, and demonstrate the applicability of our editing framework to multiple editing tasks in the motion domain.
在文本到动作生成中,可控性以及生成质量和速度变得越来越关键。可控性挑战包括生成与给定文本描述相匹配的动作长度,并根据控制信号编辑生成的动作,例如起始和结束位置以及骨盆轨迹。在本文中,我们提出了MoLA,它提供了快速、高质量、可变长度的动作生成,并且可以在单个框架中处理多个编辑任务。我们的方法重新访问模型中用作输入和输出的动作表示,通过引入激活变量来实现可变长度的动作生成。此外,我们结合了变分自编码器和潜在扩散模型,通过对抗训练进一步增强,以实现高质量和快速的生成。而且,我们应用了一种无训练引导生成框架,以实现具有运动控制输入的多种编辑任务。我们定量展示了对抗学习在文本到动作生成中的有效性,并证明我们的编辑框架在动作领域的多个编辑任务中的适用性。
论文及项目相关链接
PDF CVPR 2025 HuMoGen Workshop
Summary
本文研究了文本到运动生成中的可控性、生成质量和速度问题。提出了MoLA方法,实现了快速、高质量的可变长度运动生成,并在同一框架内处理多个编辑任务。通过重新访问模型中的运动表示,引入激活变量实现可变长度运动生成。结合变分自编码器和潜在扩散模型,通过对抗训练进一步提高生成质量和速度。应用无训练引导生成框架实现多种运动控制输入下的编辑任务。
Key Takeaways
- 文本到运动生成中,可控性、生成质量和速度变得至关重要。
- MoLA方法提供了快速、高质量的可变长度运动生成。
- MoLA方法在同一框架内处理多个编辑任务。
- 通过引入激活变量重新访问运动表示,实现可变长度运动生成。
- 结合变分自编码器和潜在扩散模型,进一步提高生成质量和速度。
- 对抗训练在文本到运动生成中的有效性得到定量验证。
点此查看论文截图
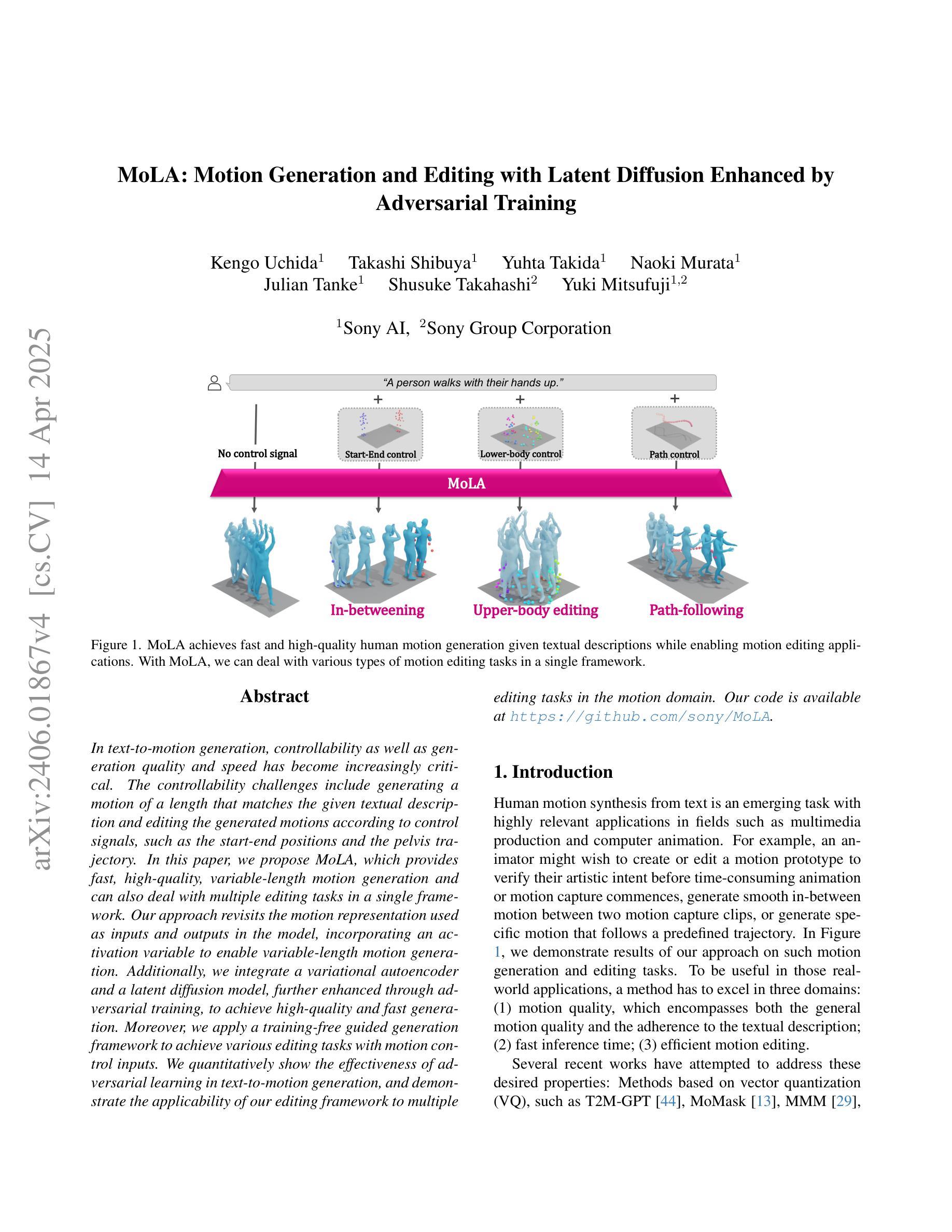
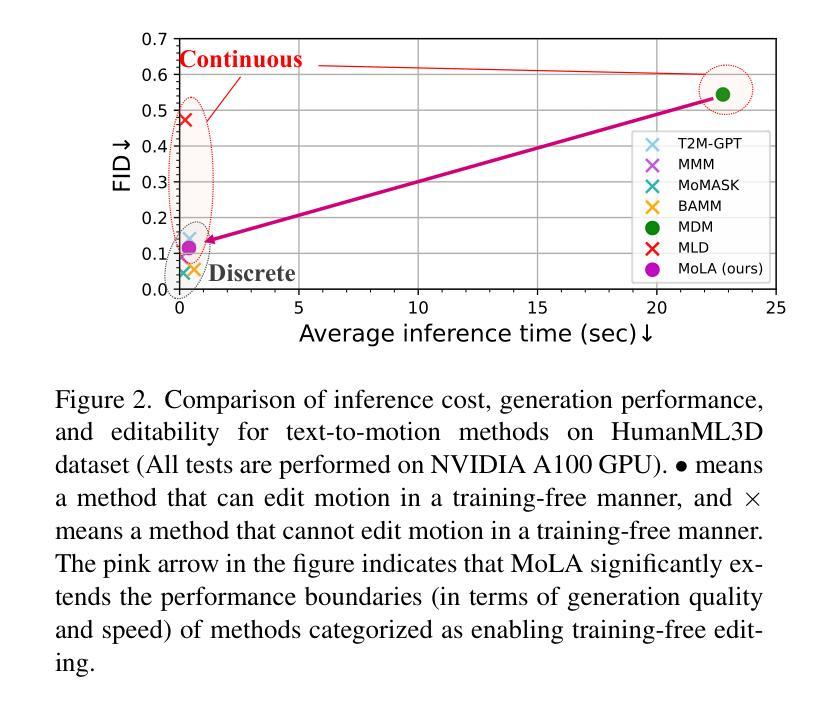
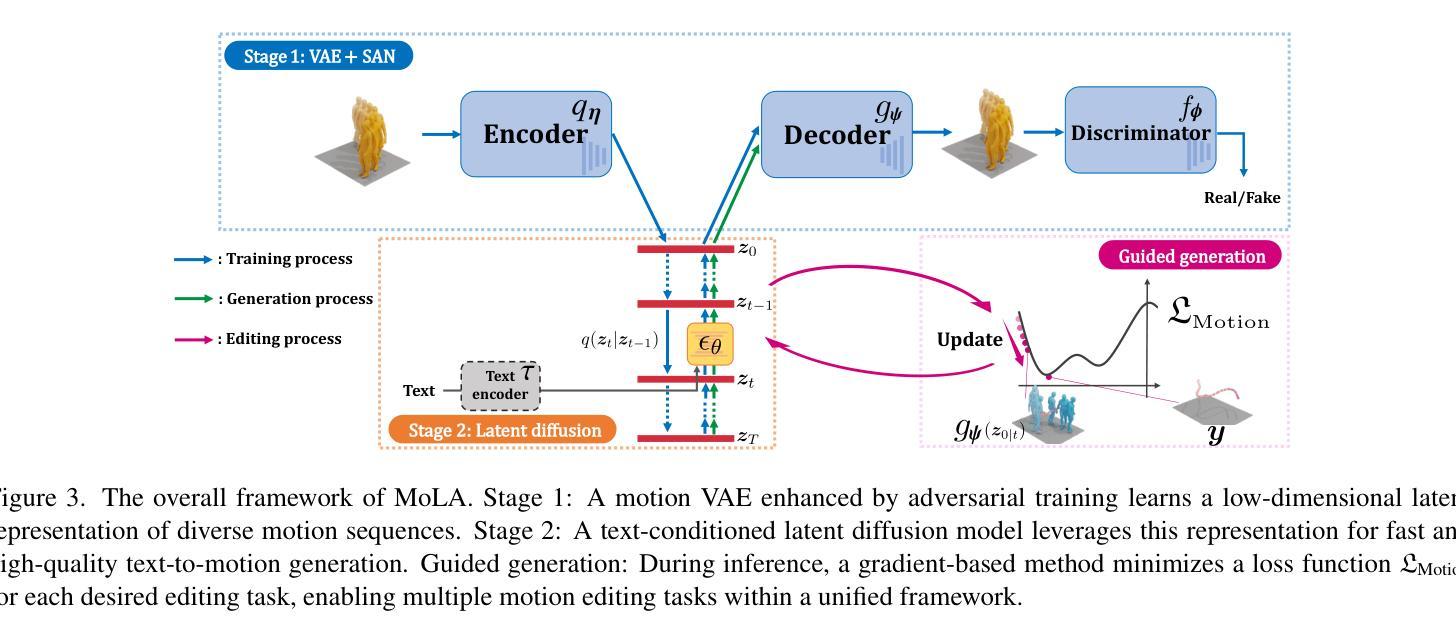
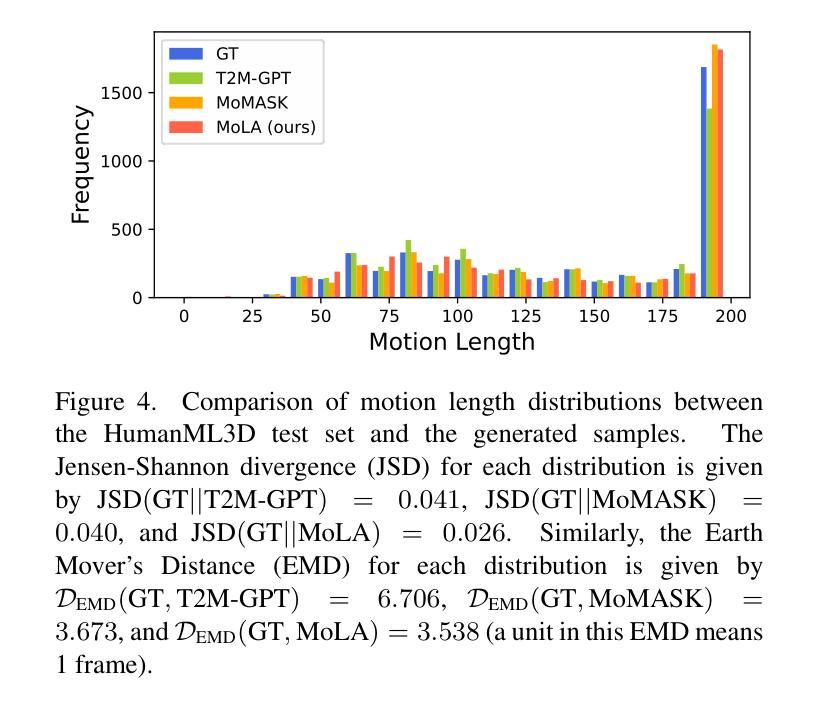