⚠️ 以下所有内容总结都来自于 大语言模型的能力,如有错误,仅供参考,谨慎使用
🔴 请注意:千万不要用于严肃的学术场景,只能用于论文阅读前的初筛!
💗 如果您觉得我们的项目对您有帮助 ChatPaperFree ,还请您给我们一些鼓励!⭐️ HuggingFace免费体验
2025-04-17 更新
Big Brother is Watching: Proactive Deepfake Detection via Learnable Hidden Face
Authors:Hongbo Li, Shangchao Yang, Ruiyang Xia, Lin Yuan, Xinbo Gao
As deepfake technologies continue to advance, passive detection methods struggle to generalize with various forgery manipulations and datasets. Proactive defense techniques have been actively studied with the primary aim of preventing deepfake operation effectively working. In this paper, we aim to bridge the gap between passive detection and proactive defense, and seek to solve the detection problem utilizing a proactive methodology. Inspired by several watermarking-based forensic methods, we explore a novel detection framework based on the concept of hiding a learnable face within a face''. Specifically, relying on a semi-fragile invertible steganography network, a secret template image is embedded into a host image imperceptibly, acting as an indicator monitoring for any malicious image forgery when being restored by the inverse steganography process. Instead of being manually specified, the secret template is optimized during training to resemble a neutral facial appearance, just like a
big brother’’ hidden in the image to be protected. By incorporating a self-blending mechanism and robustness learning strategy with a simulative transmission channel, a robust detector is built to accurately distinguish if the steganographic image is maliciously tampered or benignly processed. Finally, extensive experiments conducted on multiple datasets demonstrate the superiority of the proposed approach over competing passive and proactive detection methods.
随着深度伪造技术不断发展,被动检测技术在面对各种伪造操作和数据集时难以实现普遍推广。主动防御技术已被广泛深入研究,其主要目标是有效防止深度伪造操作。本文旨在缩小被动检测和主动防御之间的差距,并寻求利用主动方法解决检测问题。受几种基于水印的取证方法的启发,我们探索了一种基于“在脸中隐藏可学习的脸”概念的新型检测框架。具体来说,我们依赖半脆弱可逆隐写网络,将秘密模板图像不可察觉地嵌入到宿主图像中,当通过隐写逆过程恢复时,可作为任何恶意图像伪造的监控指标。秘密模板不是手动指定的,而是在训练过程中进行优化,以模仿中性面部外观,就像隐藏在受保护图像中的“大兄弟”一样。通过结合自混合机制和鲁棒性学习策略以及模拟传输通道,构建了一个鲁棒的检测器,可以准确区分隐写图像是恶意篡改还是良性处理。最后,在多个数据集上进行的广泛实验表明,与竞争性的被动和主动检测方法相比,所提出的方法具有优越性。
论文及项目相关链接
Summary
深度伪造技术不断进化,被动检测技术难以应对多种伪造操作和数据集。研究人正积极探索主动防御技术以有效防止深度伪造操作。本文借鉴水印基于取证的方法,探索了一种新型的基于“在脸中隐藏可学习的脸”概念的检测框架。该框架利用半脆弱可逆隐写网络,将秘密模板图像不可察觉地嵌入到宿主图像中,在通过逆隐写过程恢复时,作为监测图像是否被恶意篡改的指标。秘密模板在训练过程中进行优化,以模仿中性面部外观,就像隐藏在受保护图像中的“大兄兄”。结合自混合机制和鲁棒性学习策略以及模拟传输通道,构建了一个稳健的检测器,能够准确区分隐写图像是恶意篡改还是良性处理。在多个数据集上进行的广泛实验表明,该方法优于其他被动和主动检测方法。
Key Takeaways
- 深度伪造技术持续进步,被动检测技术面临挑战,需要探索新的方法。
- 本文提出了一种基于“在脸中隐藏可学习的脸”的检测框架,结合了主动防御的理念。
- 利用半脆弱可逆隐写网络,将秘密模板图像嵌入宿主图像中。
- 秘密模板在训练过程中优化,以模拟中性面部外观,作为监测图像是否被篡改的指标。
- 结合自混合机制和鲁棒性学习策略,以提高检测器的准确性和稳定性。
- 模拟传输通道被纳入考虑,以应对实际环境中的各种条件。
点此查看论文截图
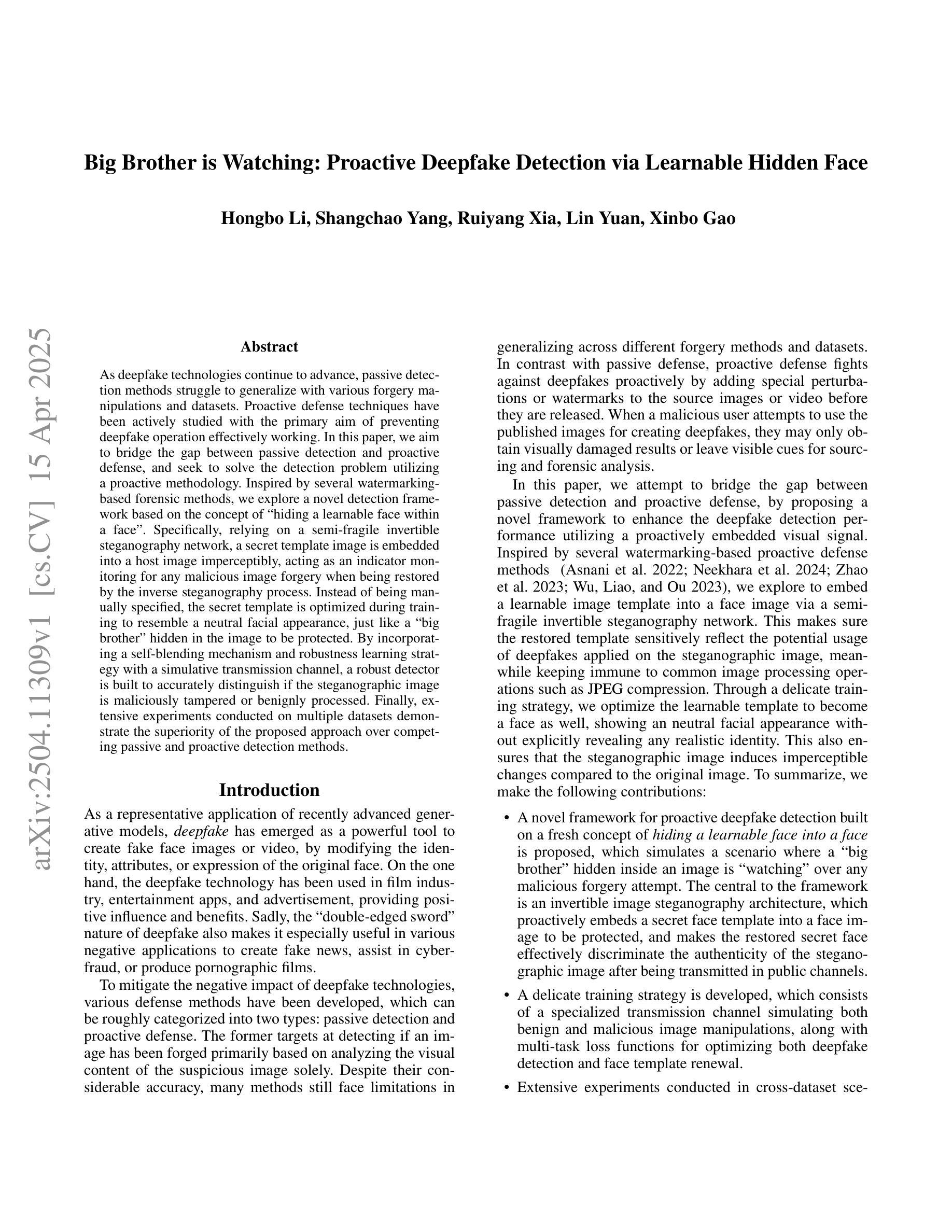
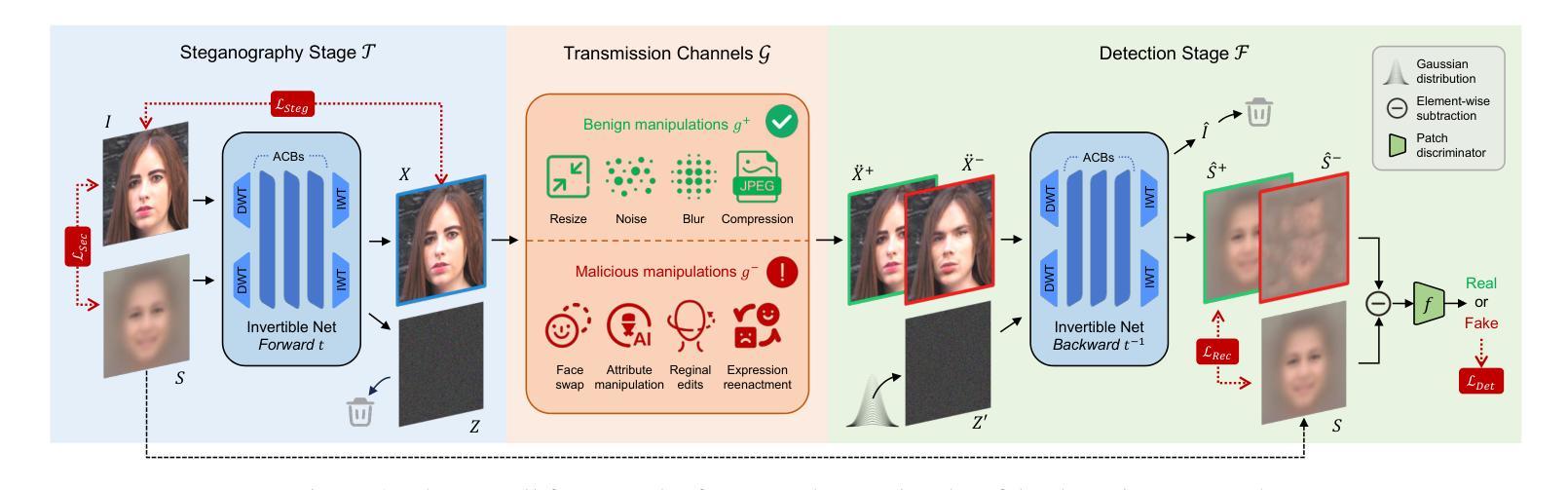
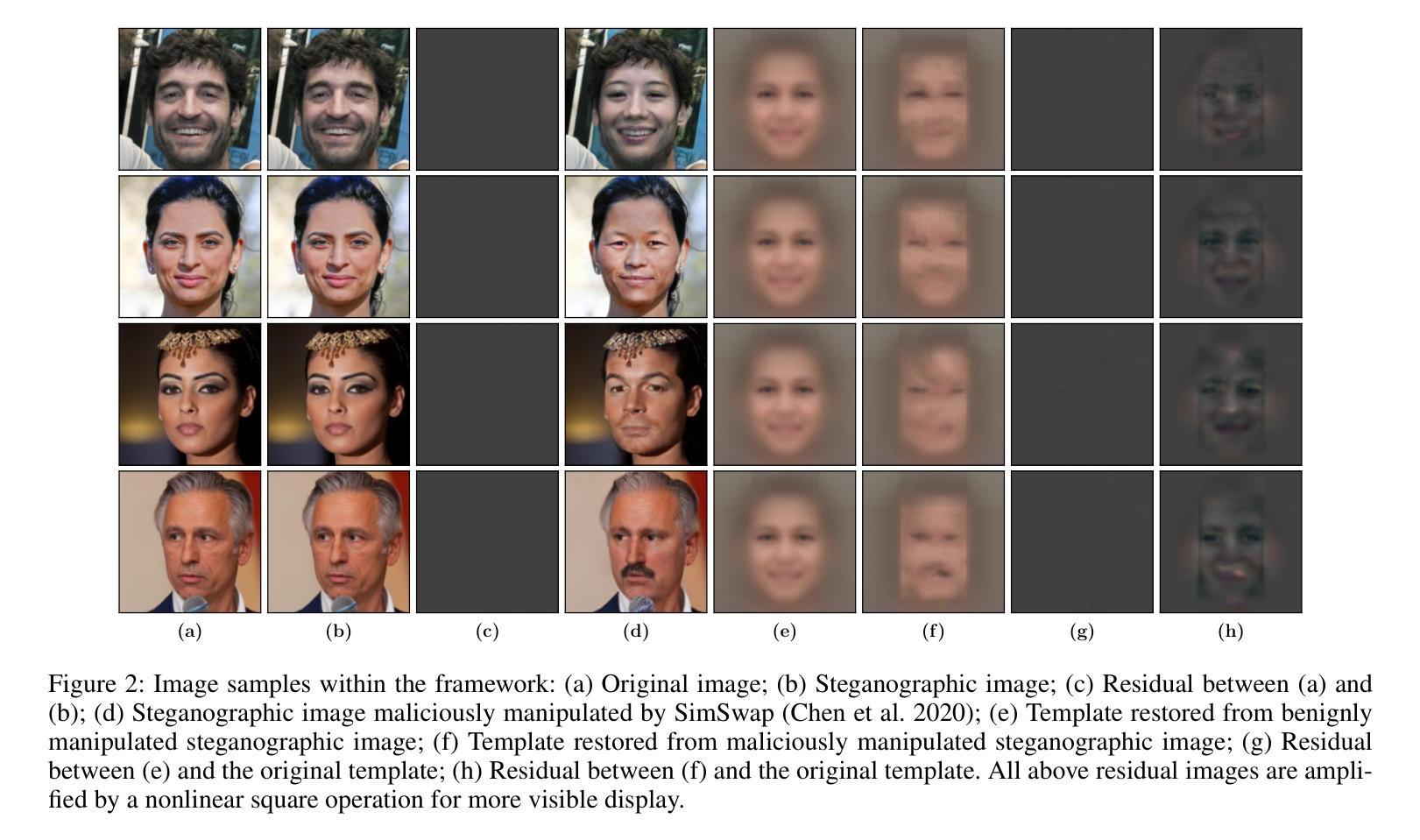
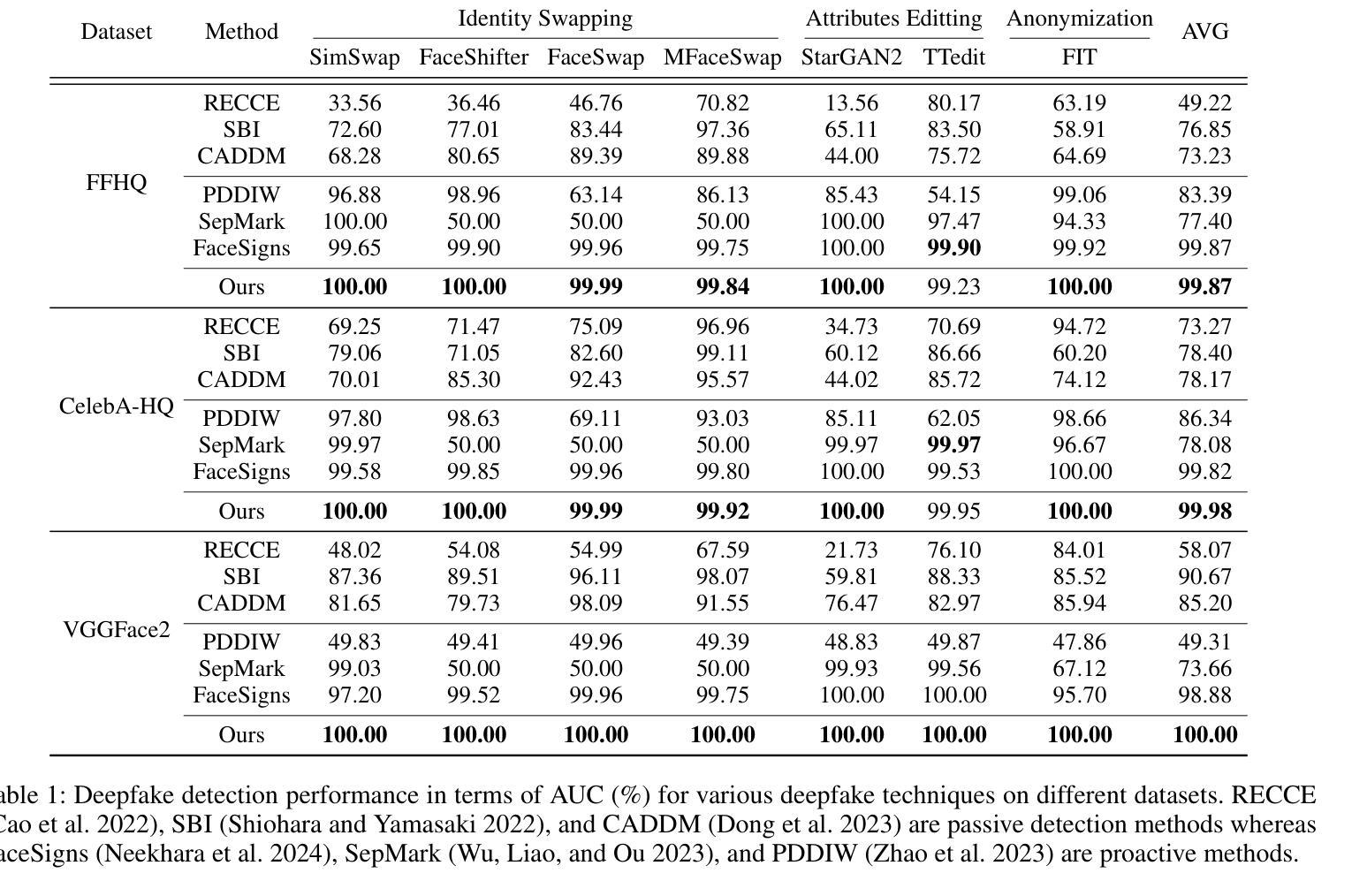
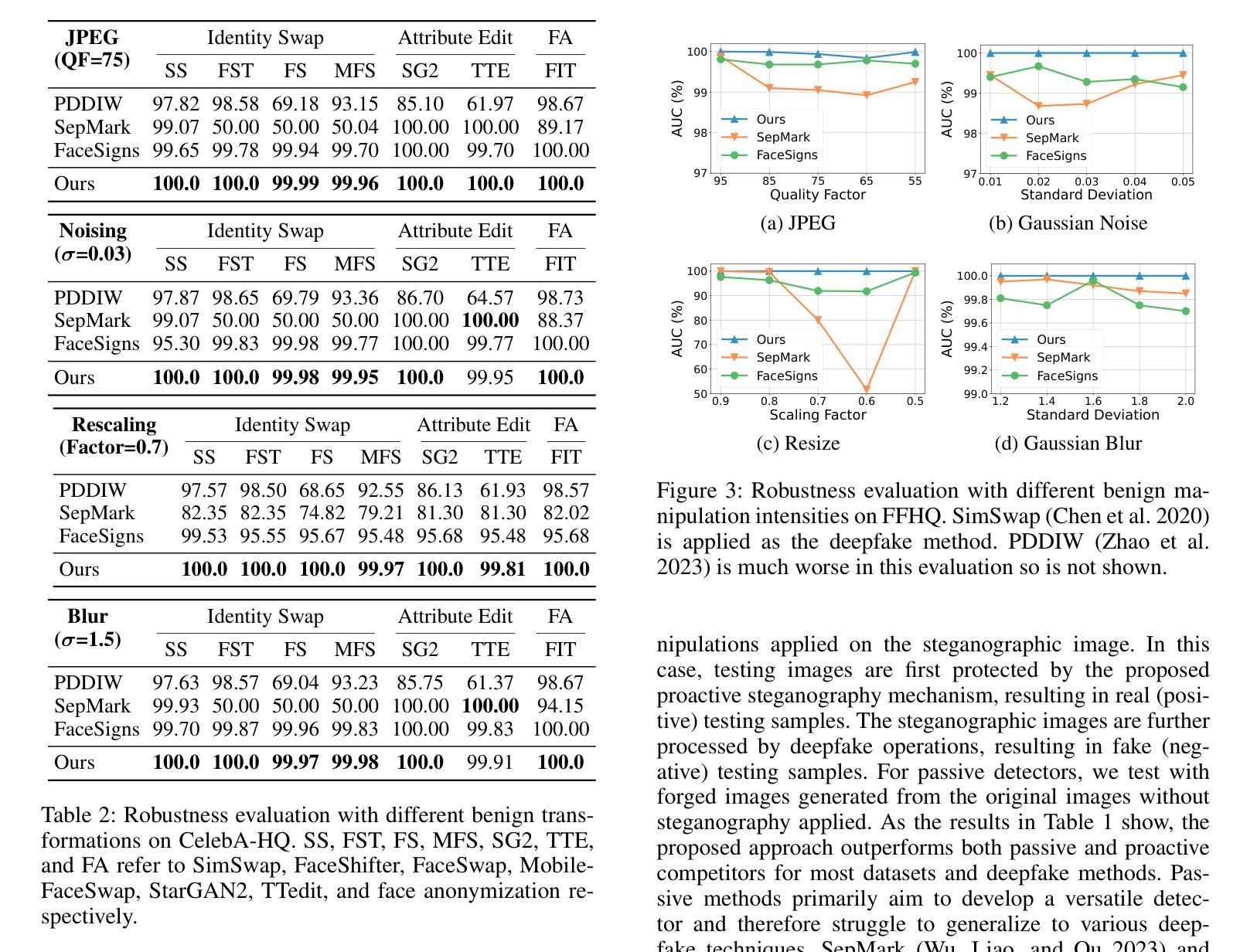