⚠️ 以下所有内容总结都来自于 大语言模型的能力,如有错误,仅供参考,谨慎使用
🔴 请注意:千万不要用于严肃的学术场景,只能用于论文阅读前的初筛!
💗 如果您觉得我们的项目对您有帮助 ChatPaperFree ,还请您给我们一些鼓励!⭐️ HuggingFace免费体验
2025-04-17 更新
GaussianHead: High-fidelity Head Avatars with Learnable Gaussian Derivation
Authors:Jie Wang, Jiu-Cheng Xie, Xianyan Li, Feng Xu, Chi-Man Pun, Hao Gao
Constructing vivid 3D head avatars for given subjects and realizing a series of animations on them is valuable yet challenging. This paper presents GaussianHead, which models the actional human head with anisotropic 3D Gaussians. In our framework, a motion deformation field and multi-resolution tri-plane are constructed respectively to deal with the head’s dynamic geometry and complex texture. Notably, we impose an exclusive derivation scheme on each Gaussian, which generates its multiple doppelgangers through a set of learnable parameters for position transformation. With this design, we can compactly and accurately encode the appearance information of Gaussians, even those fitting the head’s particular components with sophisticated structures. In addition, an inherited derivation strategy for newly added Gaussians is adopted to facilitate training acceleration. Extensive experiments show that our method can produce high-fidelity renderings, outperforming state-of-the-art approaches in reconstruction, cross-identity reenactment, and novel view synthesis tasks. Our code is available at: https://github.com/chiehwangs/gaussian-head.
构建给定主题的生动三维头部虚拟角色并在其上实现一系列动画是有价值的且具有挑战性的。本文提出GaussianHead,其利用各向异性三维高斯模型模拟人类头部动作。在我们的框架中,分别构建了运动变形场和多分辨率三角平面,以处理头部的动态几何和复杂纹理。值得注意的是,我们对每个高斯施加了一种独特的推导方案,该方案通过一组可学习参数生成其多个分身,用于位置变换。通过这种设计,我们可以紧凑且准确地编码高斯的外貌信息,即使对于拟合头部具有复杂结构的特定组件也是如此。此外,我们采用了一种针对新添加的高斯继承推导策略,以促进训练加速。大量实验表明,我们的方法能够产生高保真渲染效果,在重建、跨身份再现和新颖视图合成任务方面优于最先进的方法。我们的代码位于:https://github.com/chiehwangs/gaussian-head。
论文及项目相关链接
PDF 15 pages, 14 figures, published to TVCG
摘要
本文介绍了一种名为GaussianHead的方法,该方法使用各向异性三维高斯模型对动作人头进行建模。该方法通过构建运动变形场和多分辨率tri-plane,分别处理头部的动态几何和复杂纹理。通过一组可学习的参数对高斯进行位置变换,生成多个分身。此外,还采用了新添加的高斯继承推导策略,以加快训练速度。实验表明,该方法可以生成高保真度的渲染结果,并在重建、跨身份重构和新颖视图合成任务上优于现有技术。
要点
- GaussianHead方法利用各向异性三维高斯模型对动作人头进行建模。
- 通过运动变形场和多分辨率tri-plane处理头部的动态几何和复杂纹理。
- 使用可学习参数生成高斯分身,实现紧凑且准确编码外观信息。
- 新增高斯继承推导策略,加快训练速度。
- 该方法可以生成高保真度的渲染结果。
- 在重建、跨身份重构和新颖视图合成任务上优于现有技术。
点此查看论文截图
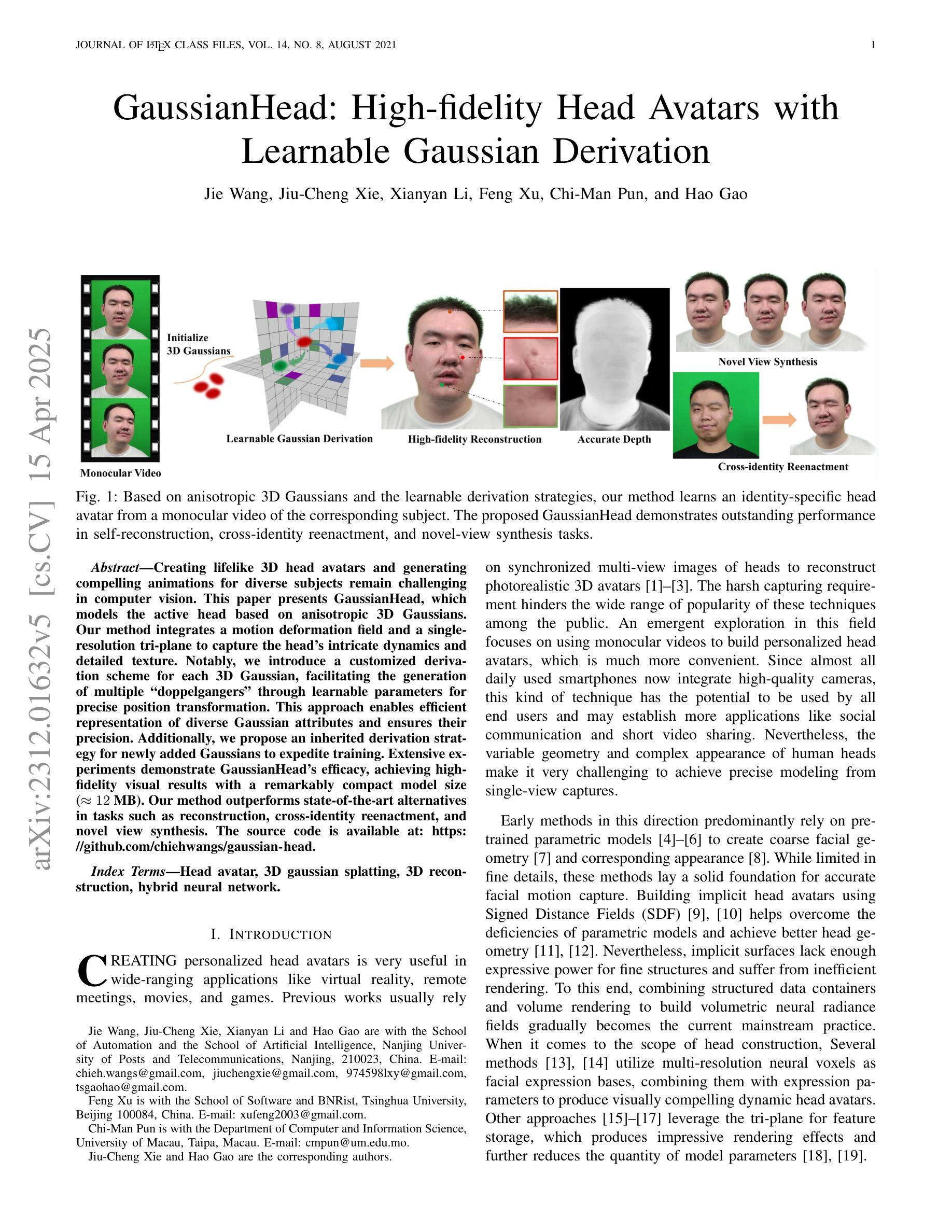
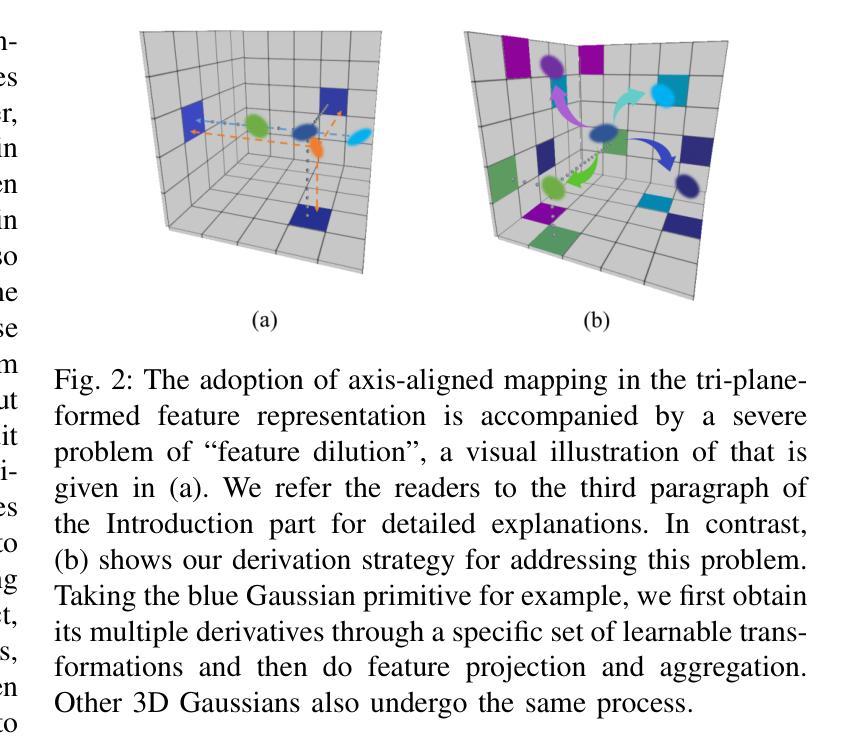
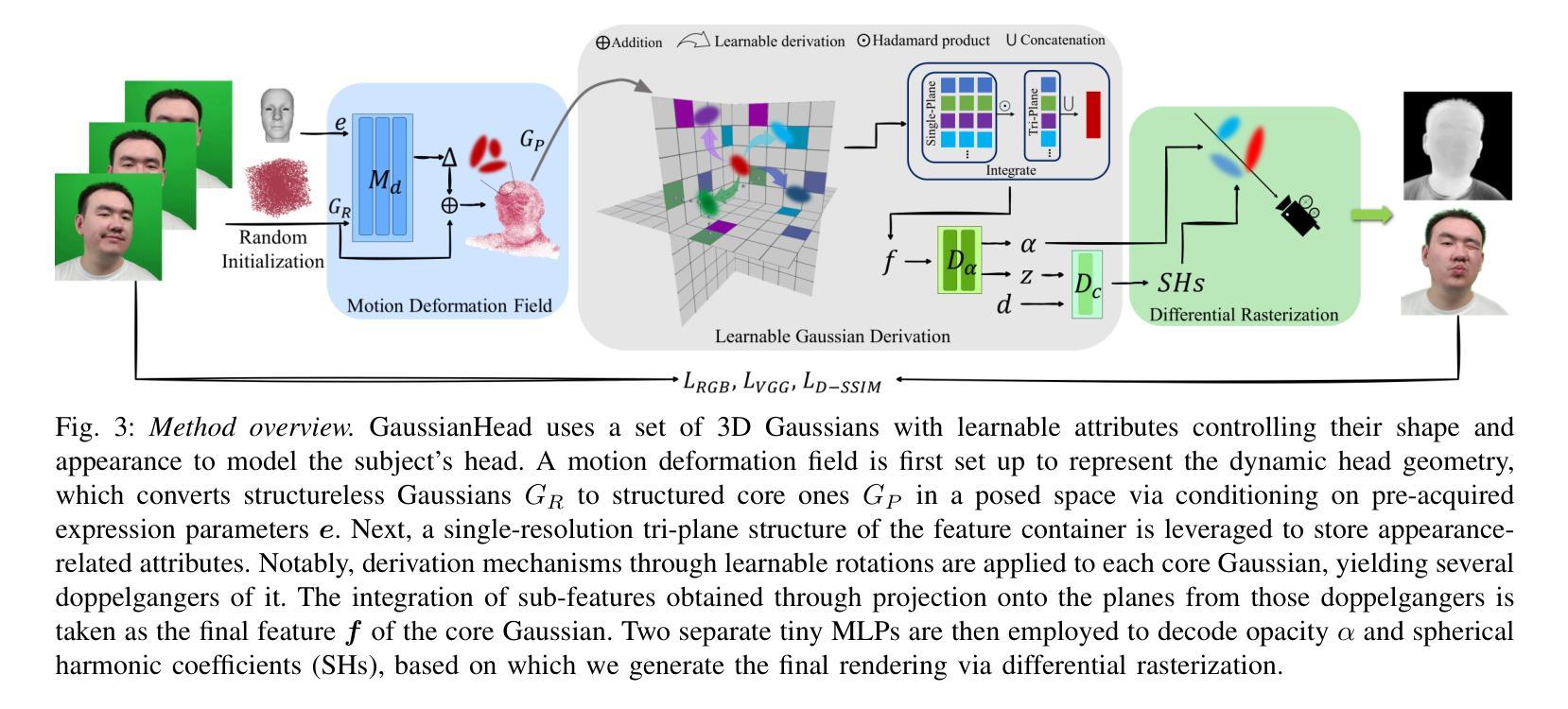