⚠️ 以下所有内容总结都来自于 大语言模型的能力,如有错误,仅供参考,谨慎使用
🔴 请注意:千万不要用于严肃的学术场景,只能用于论文阅读前的初筛!
💗 如果您觉得我们的项目对您有帮助 ChatPaperFree ,还请您给我们一些鼓励!⭐️ HuggingFace免费体验
2025-04-17 更新
H3AE: High Compression, High Speed, and High Quality AutoEncoder for Video Diffusion Models
Authors:Yushu Wu, Yanyu Li, Ivan Skorokhodov, Anil Kag, Willi Menapace, Sharath Girish, Aliaksandr Siarohin, Yanzhi Wang, Sergey Tulyakov
Autoencoder (AE) is the key to the success of latent diffusion models for image and video generation, reducing the denoising resolution and improving efficiency. However, the power of AE has long been underexplored in terms of network design, compression ratio, and training strategy. In this work, we systematically examine the architecture design choices and optimize the computation distribution to obtain a series of efficient and high-compression video AEs that can decode in real time on mobile devices. We also unify the design of plain Autoencoder and image-conditioned I2V VAE, achieving multifunctionality in a single network. In addition, we find that the widely adopted discriminative losses, i.e., GAN, LPIPS, and DWT losses, provide no significant improvements when training AEs at scale. We propose a novel latent consistency loss that does not require complicated discriminator design or hyperparameter tuning, but provides stable improvements in reconstruction quality. Our AE achieves an ultra-high compression ratio and real-time decoding speed on mobile while outperforming prior art in terms of reconstruction metrics by a large margin. We finally validate our AE by training a DiT on its latent space and demonstrate fast, high-quality text-to-video generation capability.
自编码器(AE)是潜扩散模型在图像和视频生成中取得成功的关键,它能够降低降噪分辨率并提高效率。然而,关于自编码器的网络设计、压缩比和训练策略等方面的潜力长期以来一直被忽视。在这项工作中,我们系统地研究了架构设计选择,优化了计算分布,获得了一系列高效、高压缩的视频自编码器,可在移动设备上实时解码。我们还统一了普通自编码器和图像条件I2V VAE的设计,实现了单一网络的多功能性。此外,我们发现广泛采用的判别损失,即GAN、LPIPS和DWT损失,在训练规模较大的自编码器时并未提供显著改进。我们提出了一种新型潜在一致性损失,不需要复杂的判别器设计或超参数调整,但在重建质量方面提供了稳定的改进。我们的自编码器在移动设备上的压缩比超高,解码速度实时,同时在重建指标方面大大优于先前技术。最后,我们通过在其潜在空间上训练DiT来验证我们的自编码器,并展示了快速、高质量的文字到视频生成能力。
论文及项目相关链接
PDF 8 pages, 4 figures, 6 tables
Summary
该文本介绍了自动编码器(AE)在潜扩散模型中的关键作用,并对其进行系统研究,优化计算分布以获取高效、高压缩的视频AE,能在移动设备上实时解码。同时实现了普通自动编码器和图像条件I2V VAE的统一设计,具有多功能性。提出新的潜在一致性损失,无需复杂的鉴别器设计和超参数调整,但在重建质量上提供稳定的改进。此外,所构建的AE具有高压缩比和实时解码速度,在重建指标上大幅优于先前技术。最后,通过对潜在空间进行训练,验证了AE的快速、高质量文本到视频生成能力。
Key Takeaways
- 自动编码器(AE)在潜扩散模型中扮演关键角色,能够降低去噪分辨率并提高效率。
- 系统研究网络设计、压缩比和训练策略,优化计算分布以获取高效、高压缩的视频AE。
- 在移动设备上实现了实时解码能力的高压缩视频AE。
- 统一普通自动编码器和图像条件I2V VAE的设计,实现单一网络的多功能性。
- 提出新的潜在一致性损失,无需复杂的鉴别器设计和超参数调整,提升重建质量。
- AE具有高压缩比和实时解码速度,并在重建指标上显著优于先前技术。
点此查看论文截图
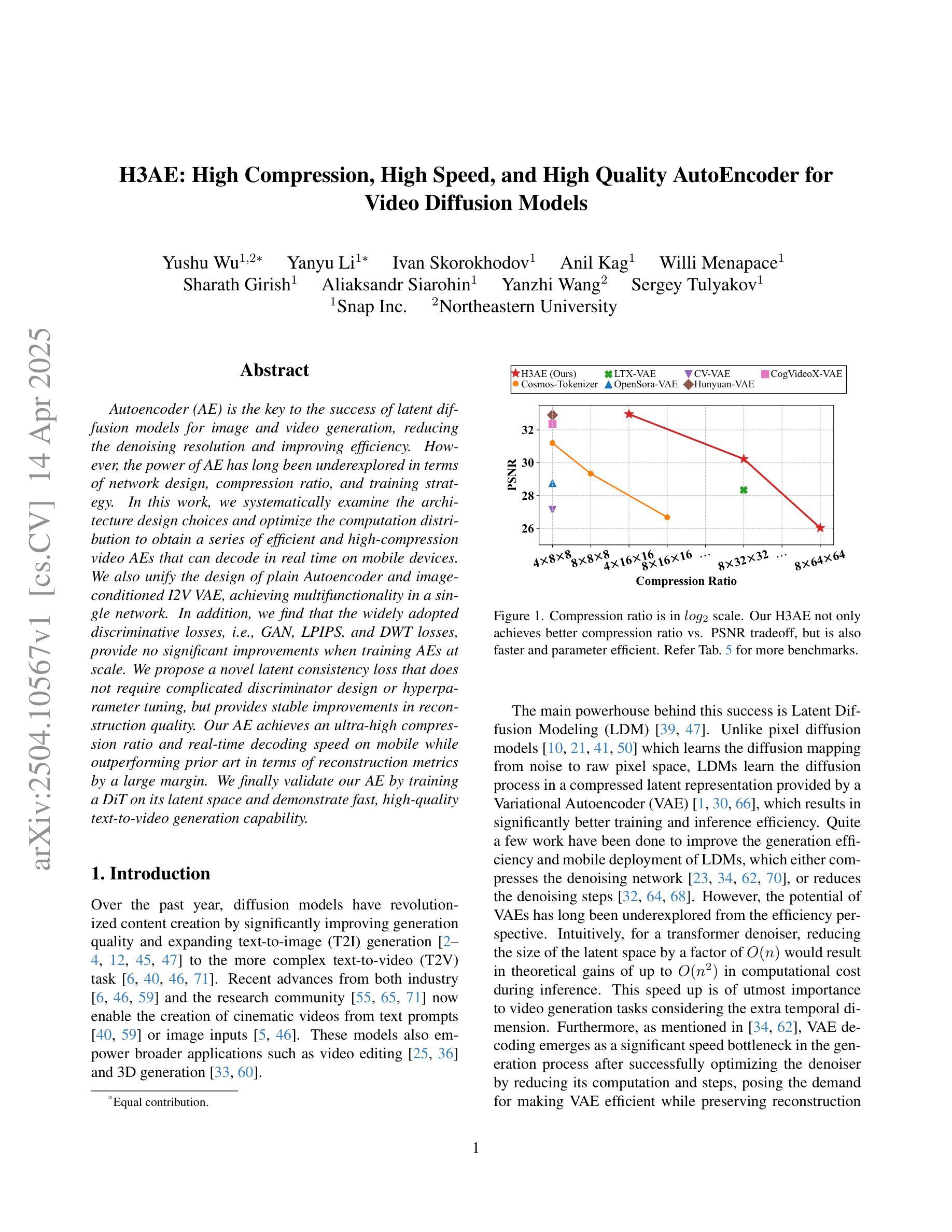
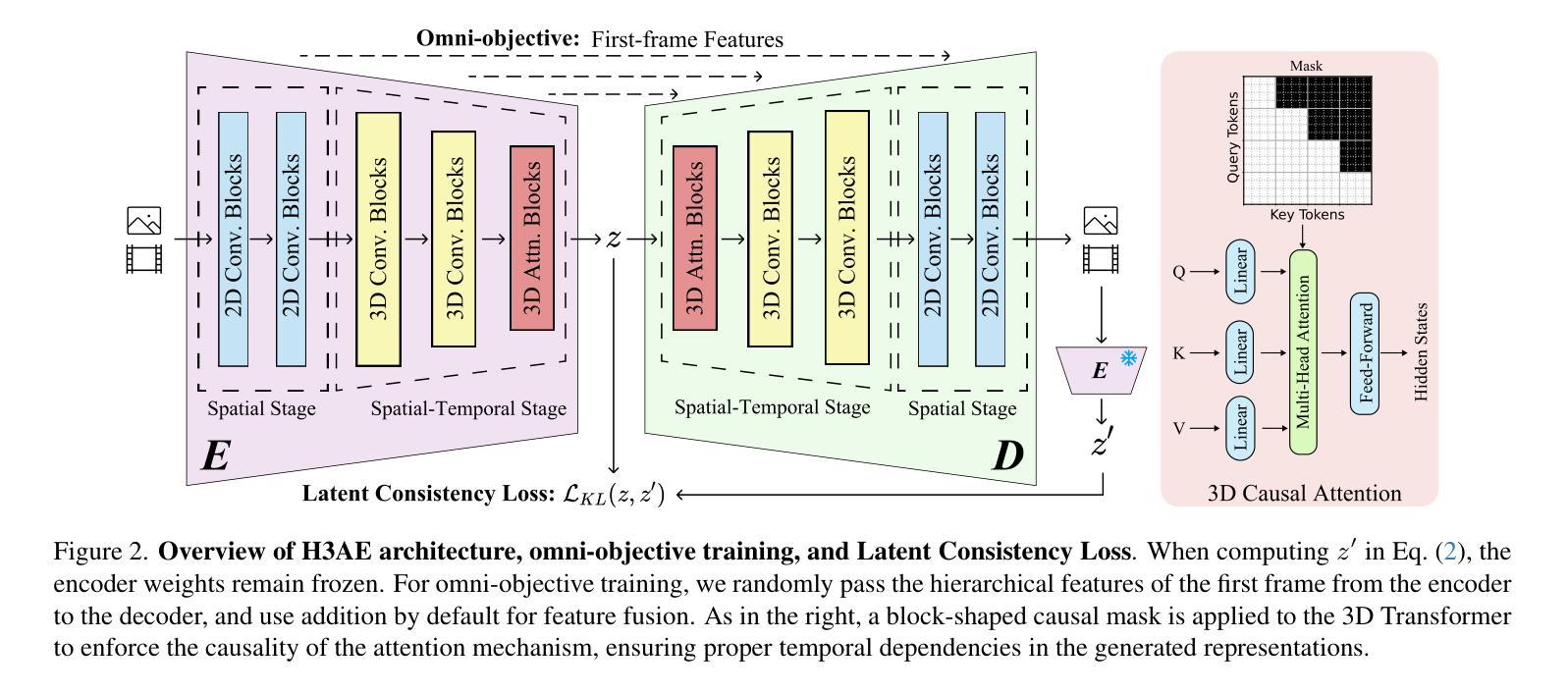
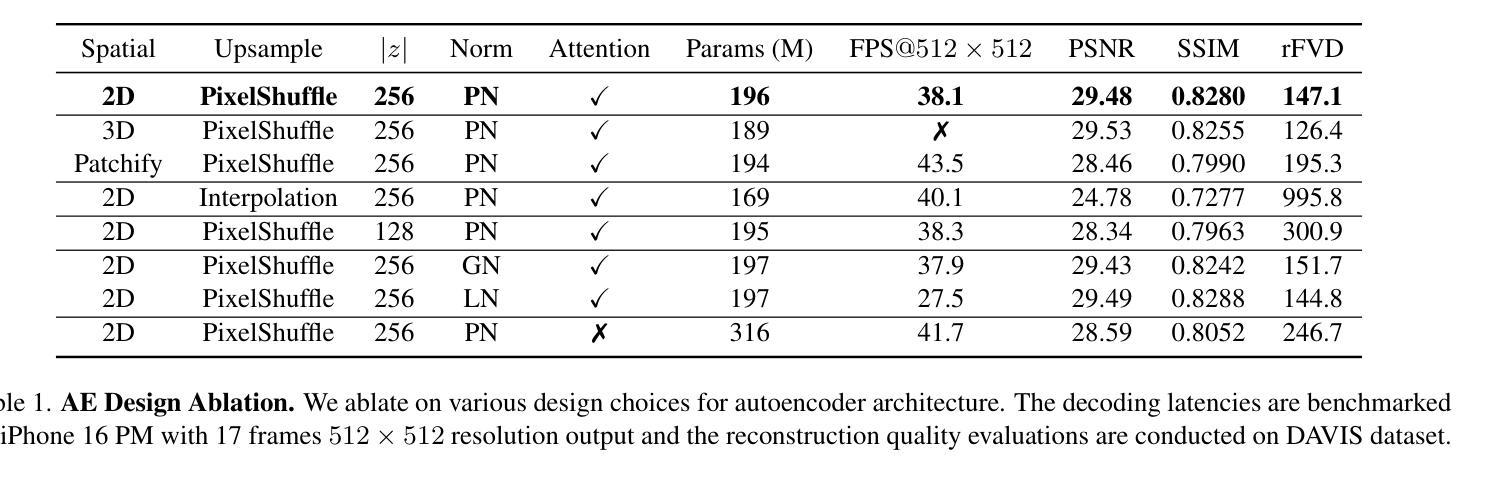
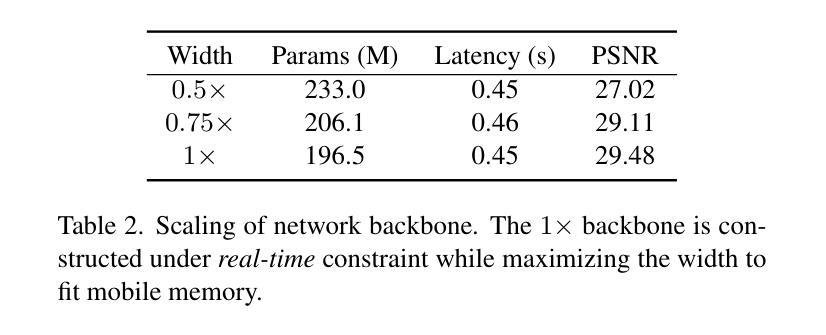
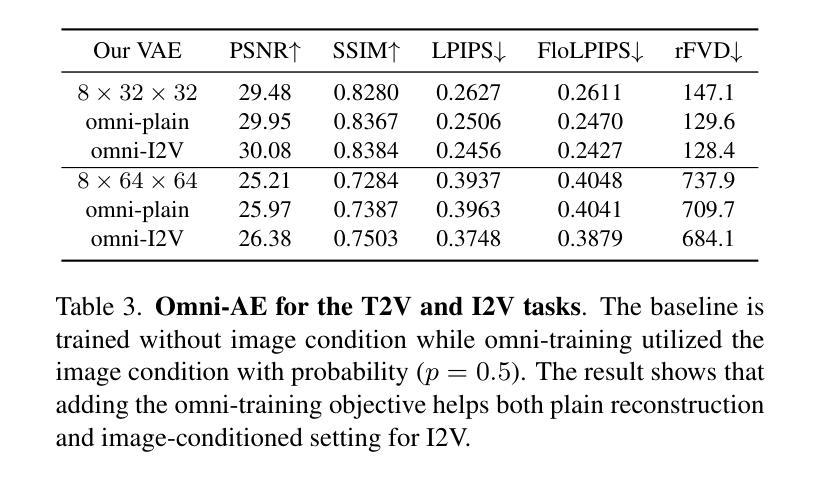
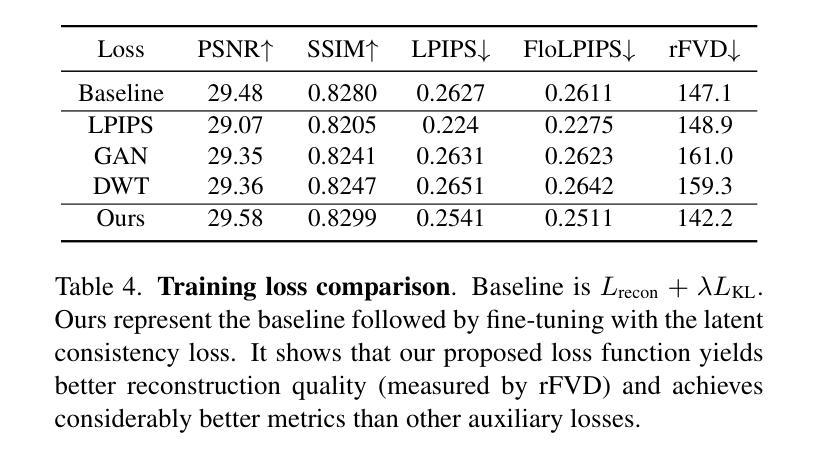
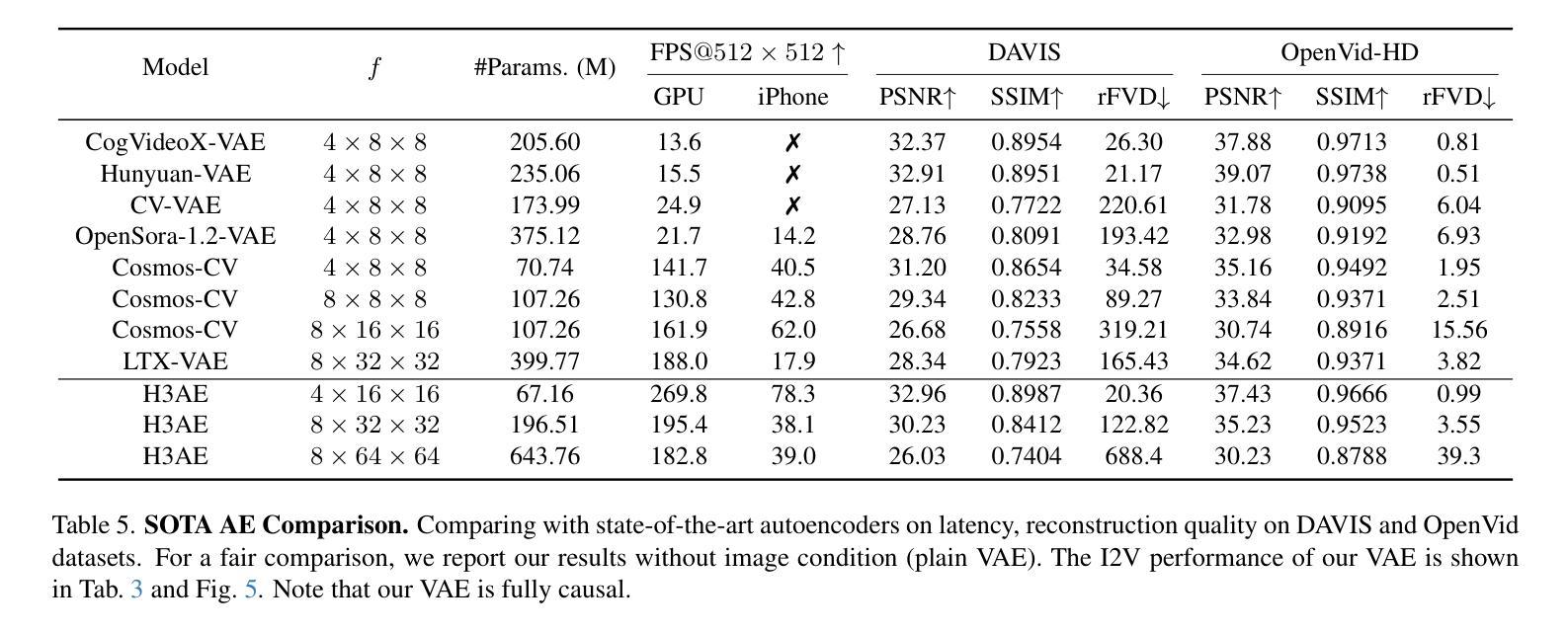
CyclePose – Leveraging Cycle-Consistency for Annotation-Free Nuclei Segmentation in Fluorescence Microscopy
Authors:Jonas Utz, Stefan Vocht, Anne Tjorven Buessen, Dennis Possart, Fabian Wagner, Mareike Thies, Mingxuan Gu, Stefan Uderhardt, Katharina Breininger
In recent years, numerous neural network architectures specifically designed for the instance segmentation of nuclei in microscopic images have been released. These models embed nuclei-specific priors to outperform generic architectures like U-Nets; however, they require large annotated datasets, which are often not available. Generative models (GANs, diffusion models) have been used to compensate for this by synthesizing training data. These two-stage approaches are computationally expensive, as first a generative model and then a segmentation model has to be trained. We propose CyclePose, a hybrid framework integrating synthetic data generation and segmentation training. CyclePose builds on a CycleGAN architecture, which allows unpaired translation between microscopy images and segmentation masks. We embed a segmentation model into CycleGAN and leverage a cycle consistency loss for self-supervision. Without annotated data, CyclePose outperforms other weakly or unsupervised methods on two public datasets. Code is available at https://github.com/jonasutz/CyclePose
近年来,针对显微图像中的细胞核实例分割,已经发布了大量专门设计的神经网络架构。这些模型嵌入细胞核特异性先验知识,以超越通用架构(如U-Nets)的表现;然而,它们需要大量的标注数据集,而这些数据通常不可用。生成模型(GANs、扩散模型)已被用于通过合成训练数据来弥补这一缺陷。这两种阶段的方法计算成本高昂,因为首先需要训练一个生成模型,然后是一个分割模型。我们提出了CyclePose,这是一个混合框架,融合了合成数据生成和分割训练。CyclePose基于CycleGAN架构,实现了显微图像与分割掩膜之间的无配对转换。我们将分割模型嵌入到CycleGAN中,并利用循环一致性损失进行自监督。无需标注数据,CyclePose在两个公共数据集上的表现优于其他弱监督或无监督方法。代码可访问https://github.com/jonasutz/CyclePose。
论文及项目相关链接
PDF under review for MICCAI 2025
Summary
本文介绍了针对显微图像中的细胞核实例分割而设计的神经网络架构。为提高性能,已使用生成模型(如GANs和扩散模型)合成训练数据。提出了一种集成合成数据生成和分割训练的新型混合框架CyclePose,该框架基于CycleGAN架构,能够实现显微镜图像与分割掩膜之间的无配对转换。CyclePose嵌入分割模型,并利用循环一致性损失进行自监督学习,无需标注数据,在公共数据集上的表现优于其他弱监督或无监督方法。
Key Takeaways
- 近年针对显微图像中的细胞核实例分割设计了多种神经网络架构。
- 生成模型(如GANs、扩散模型)被用于合成训练数据以弥补标注数据的不足。
- 提出了新型混合框架CyclePose,集成合成数据生成和分割训练。
- CyclePose基于CycleGAN架构,实现显微镜图像与分割掩膜之间的无配对转换。
- CyclePose通过嵌入分割模型并利用循环一致性损失进行自监督学习。
- CyclePose在公共数据集上的表现优于其他弱监督或无监督方法。
点此查看论文截图
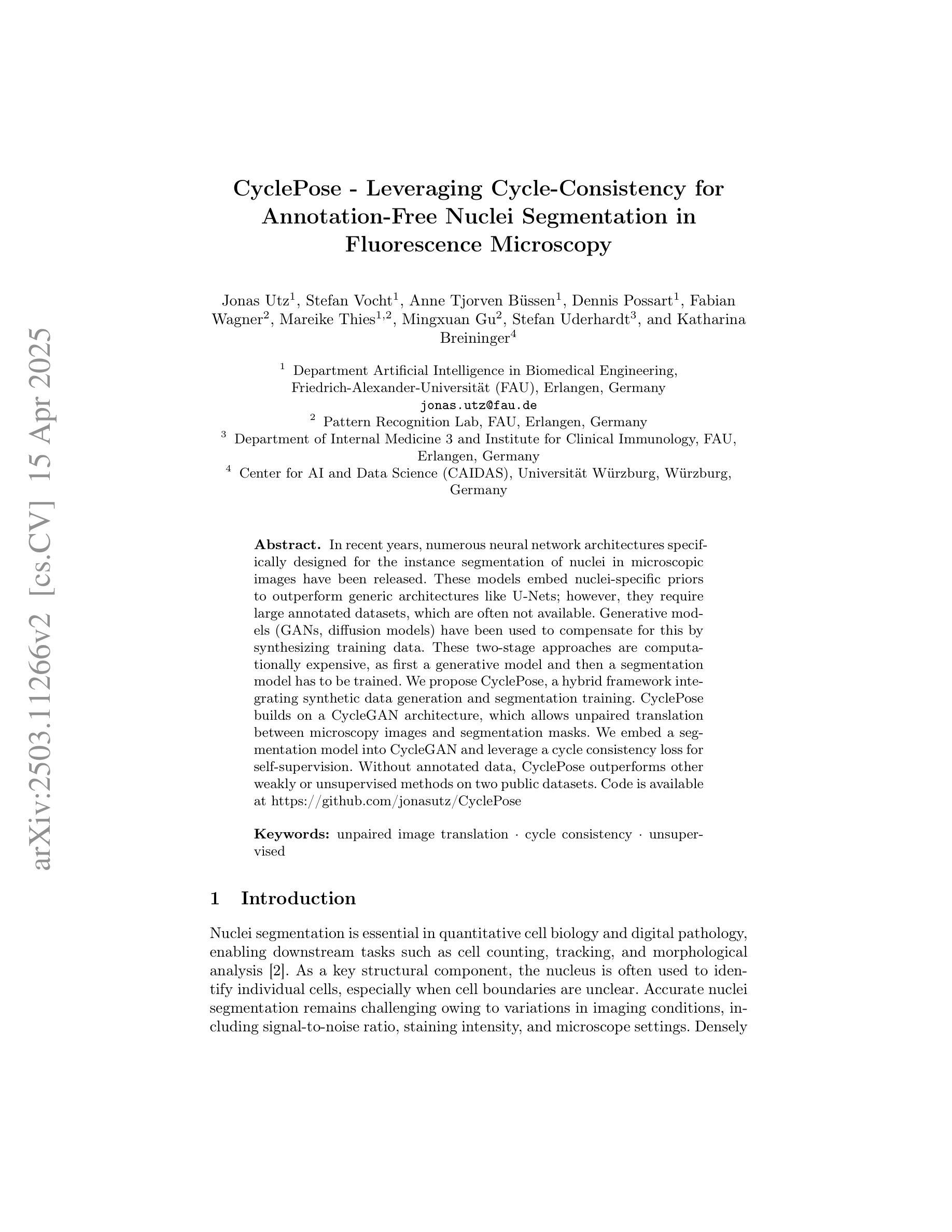
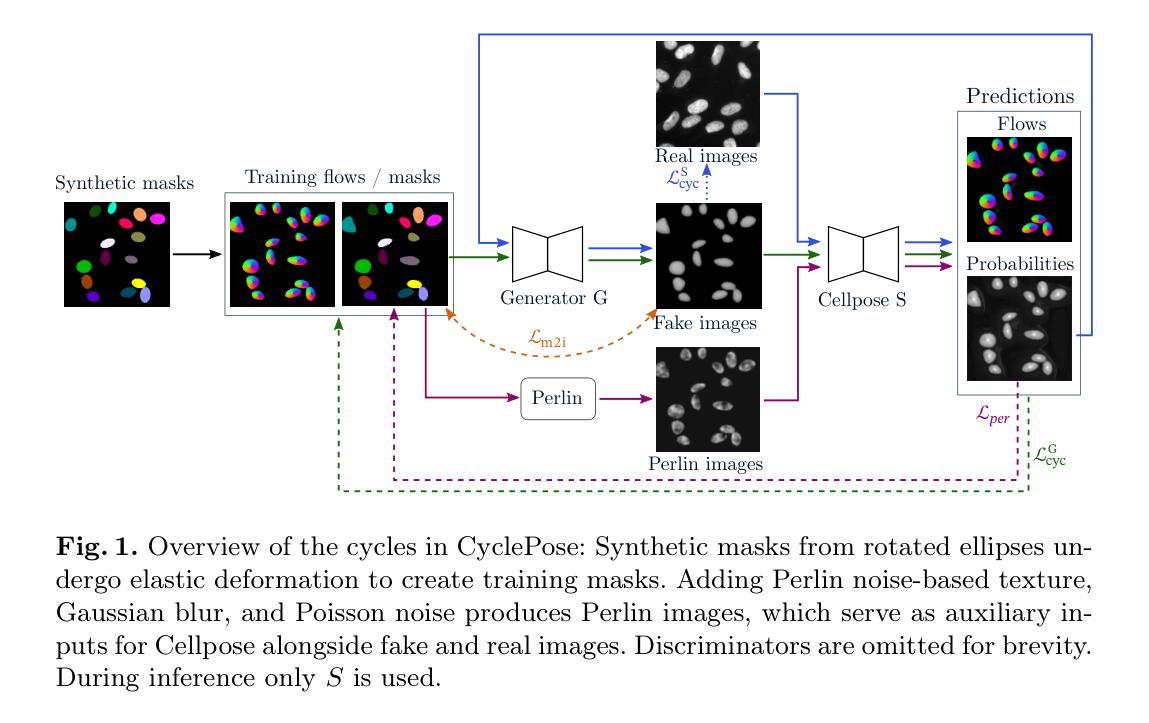
Reference-Based 3D-Aware Image Editing with Triplanes
Authors:Bahri Batuhan Bilecen, Yigit Yalin, Ning Yu, Aysegul Dundar
Generative Adversarial Networks (GANs) have emerged as powerful tools for high-quality image generation and real image editing by manipulating their latent spaces. Recent advancements in GANs include 3D-aware models such as EG3D, which feature efficient triplane-based architectures capable of reconstructing 3D geometry from single images. However, limited attention has been given to providing an integrated framework for 3D-aware, high-quality, reference-based image editing. This study addresses this gap by exploring and demonstrating the effectiveness of the triplane space for advanced reference-based edits. Our novel approach integrates encoding, automatic localization, spatial disentanglement of triplane features, and fusion learning to achieve the desired edits. We demonstrate how our approach excels across diverse domains, including human faces, 360-degree heads, animal faces, partially stylized edits like cartoon faces, full-body clothing edits, and edits on class-agnostic samples. Our method shows state-of-the-art performance over relevant latent direction, text, and image-guided 2D and 3D-aware diffusion and GAN methods, both qualitatively and quantitatively.
生成对抗网络(GANs)通过操作潜在空间,已成为高质量图像生成和真实图像编辑的强大工具。最近的GAN进展包括三维感知模型,如EG3D,它采用基于高效三面架构的特性,能够从单幅图像重建三维几何。然而,对于基于三维感知、高质量、参考图像编辑的综合框架的关注仍然有限。本研究通过探索并展示三面空间在高级参考编辑中的有效性来解决这一差距。我们的新方法集成了编码、自动定位、三面特征的空间分离和融合学习,以实现所需的编辑。我们展示了我们的方法在包括人脸、360度头部、动物面部、部分风格化的编辑(如卡通脸)、全身服装编辑以及类别无关样本的编辑等多个领域的卓越表现。我们的方法在相关的潜在方向、文本、图像引导的二维和三维感知扩散以及GAN方法上均表现出最先进的性能,无论是定性还是定量。
论文及项目相关链接
PDF CVPR 2025 Highlight. Includes supplementary material
Summary
本文探讨了利用生成对抗网络(GANs)的潜在空间进行高质量图像生成和真实图像编辑的技术。针对当前缺乏一个集成的框架进行三维感知的参考式图像编辑问题,本文提出一种新型的编辑方法,利用triplane空间实现编码、自动定位、triplane特征的空间解耦和融合学习,展现出跨多个领域的优势表现,包括人脸、全景头部、动物面部等,甚至部分风格化的编辑如卡通脸、全身衣物编辑以及类别无关的样本编辑。相对于当前相关的潜在方向以及文本、图像引导的二维和三维扩散GAN方法,该方法在定性和定量上都展现出了卓越性能。
Key Takeaways
- GANs已被用于高质量图像生成和真实图像编辑。
- 当前缺乏一个集成的框架进行三维感知的参考式图像编辑。
- 本文提出了一种新型方法,利用triplane空间实现高效的三维感知参考式图像编辑。
- 方法结合了编码、自动定位、triplane特征的空间解耦和融合学习。
- 方法在多个领域展现出优势,包括人脸、全景头部、动物面部等。
- 该方法实现了部分风格化的编辑,如卡通脸、全身衣物编辑以及类别无关的样本编辑。
点此查看论文截图
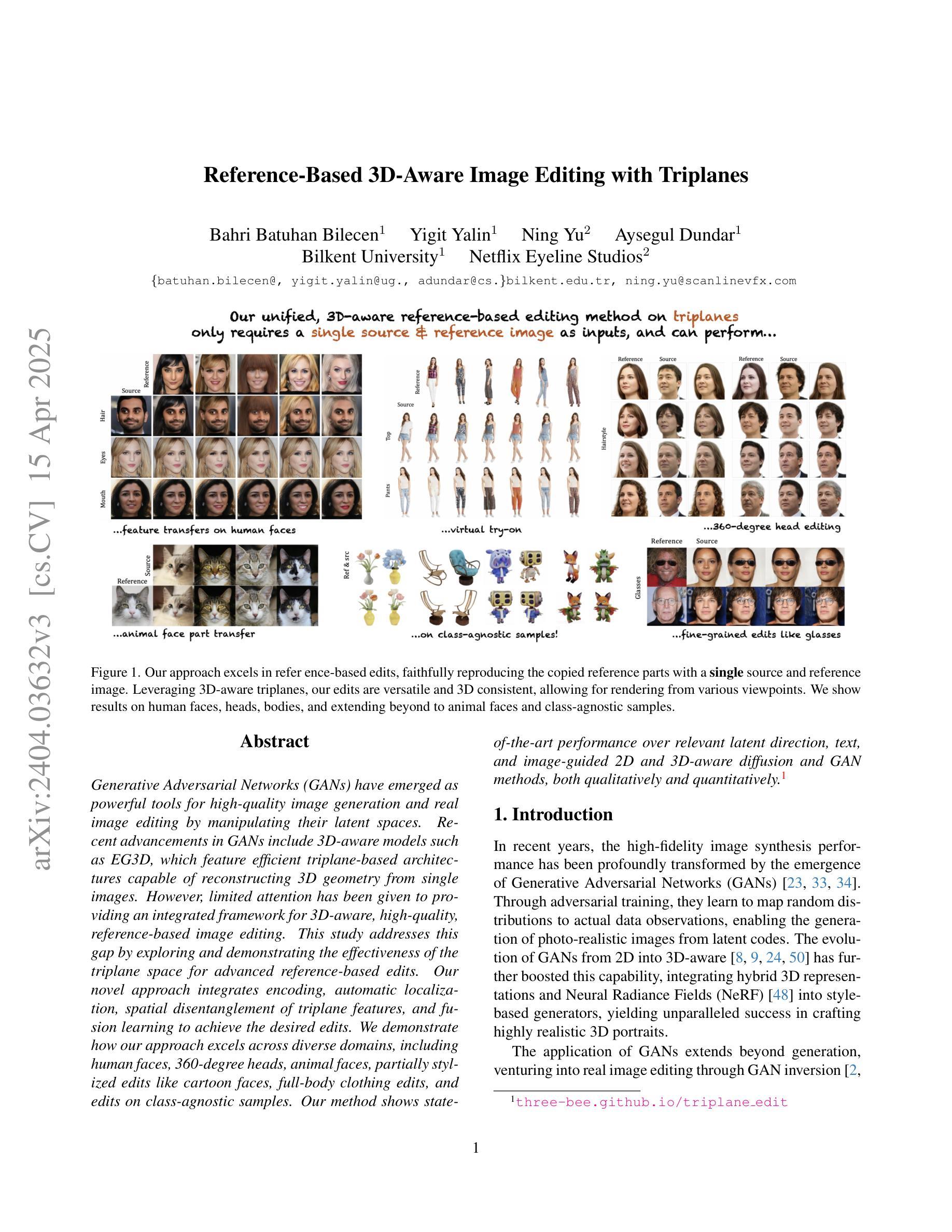
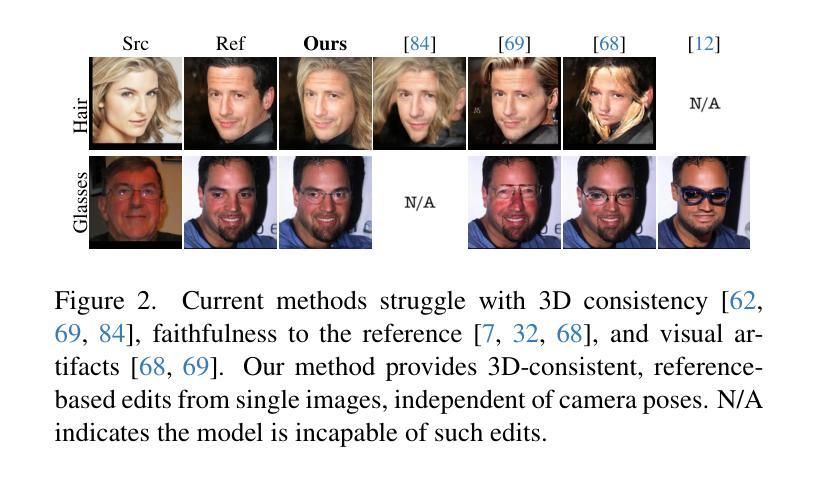
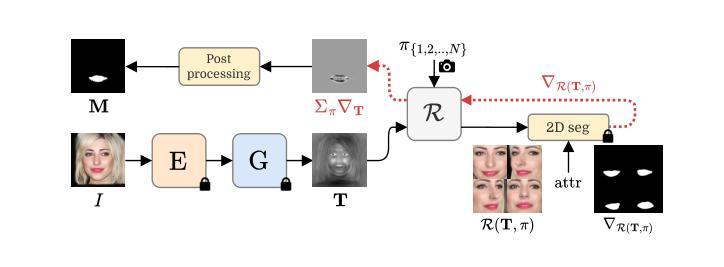
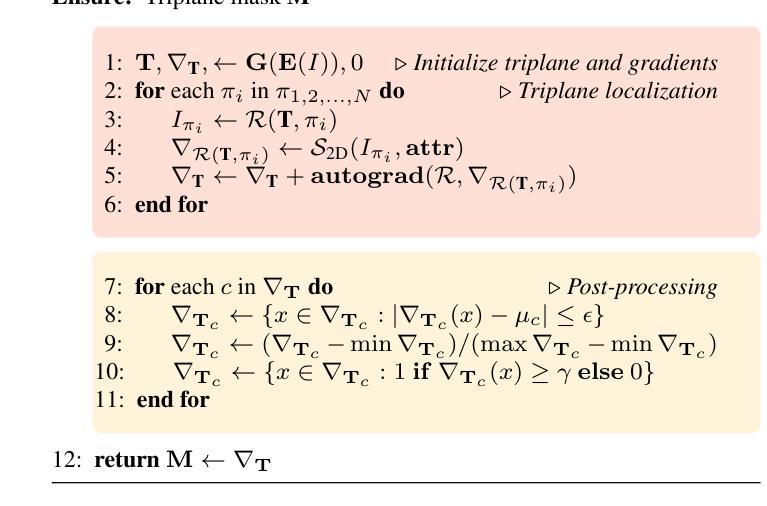
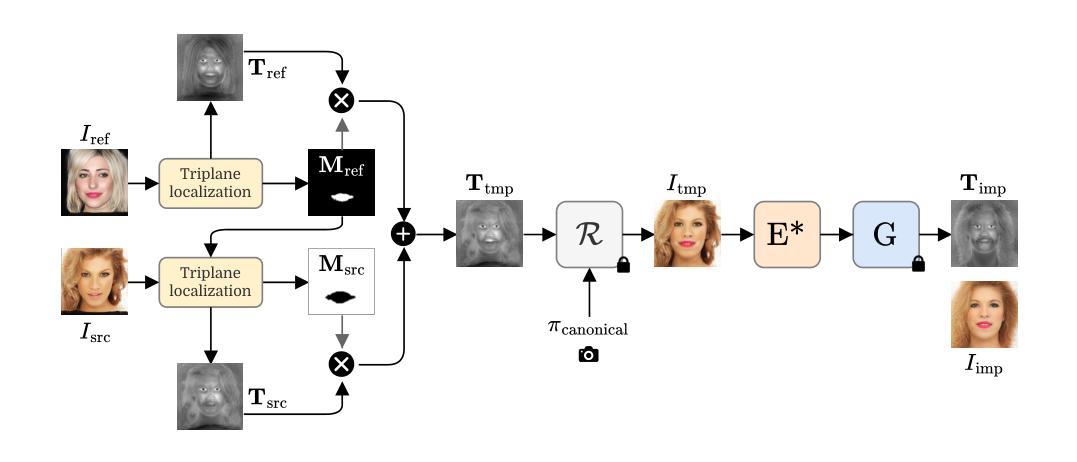
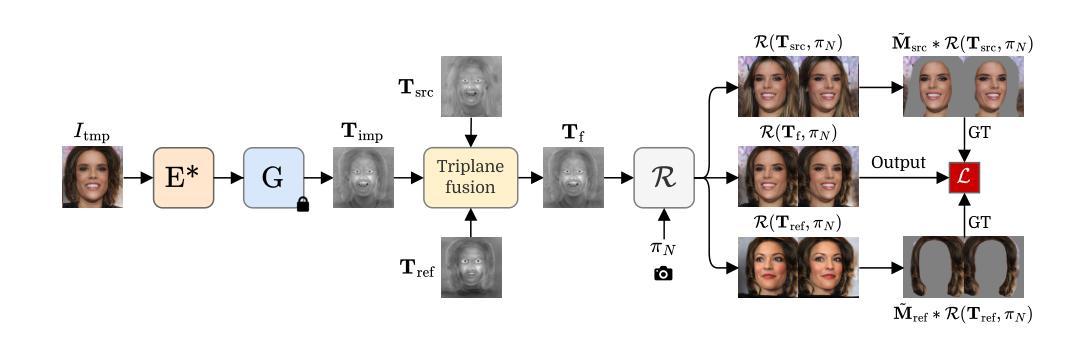
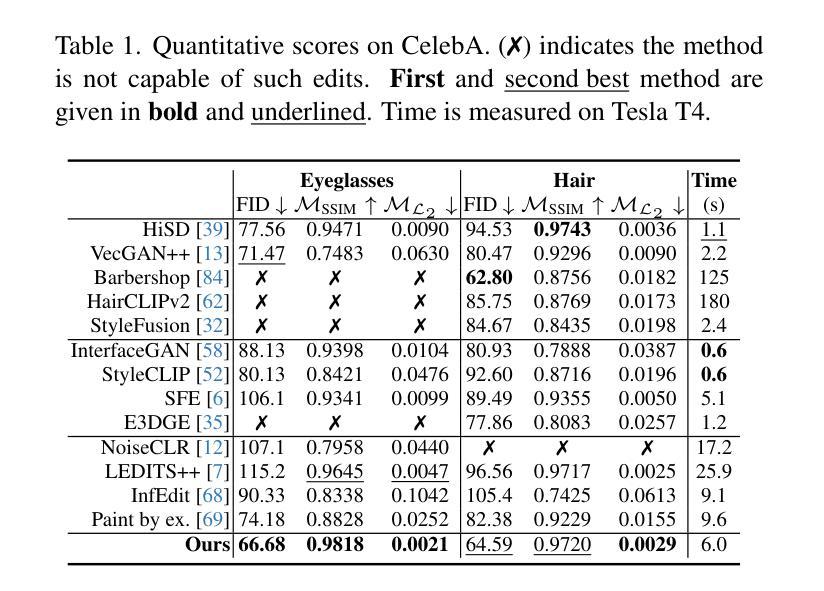
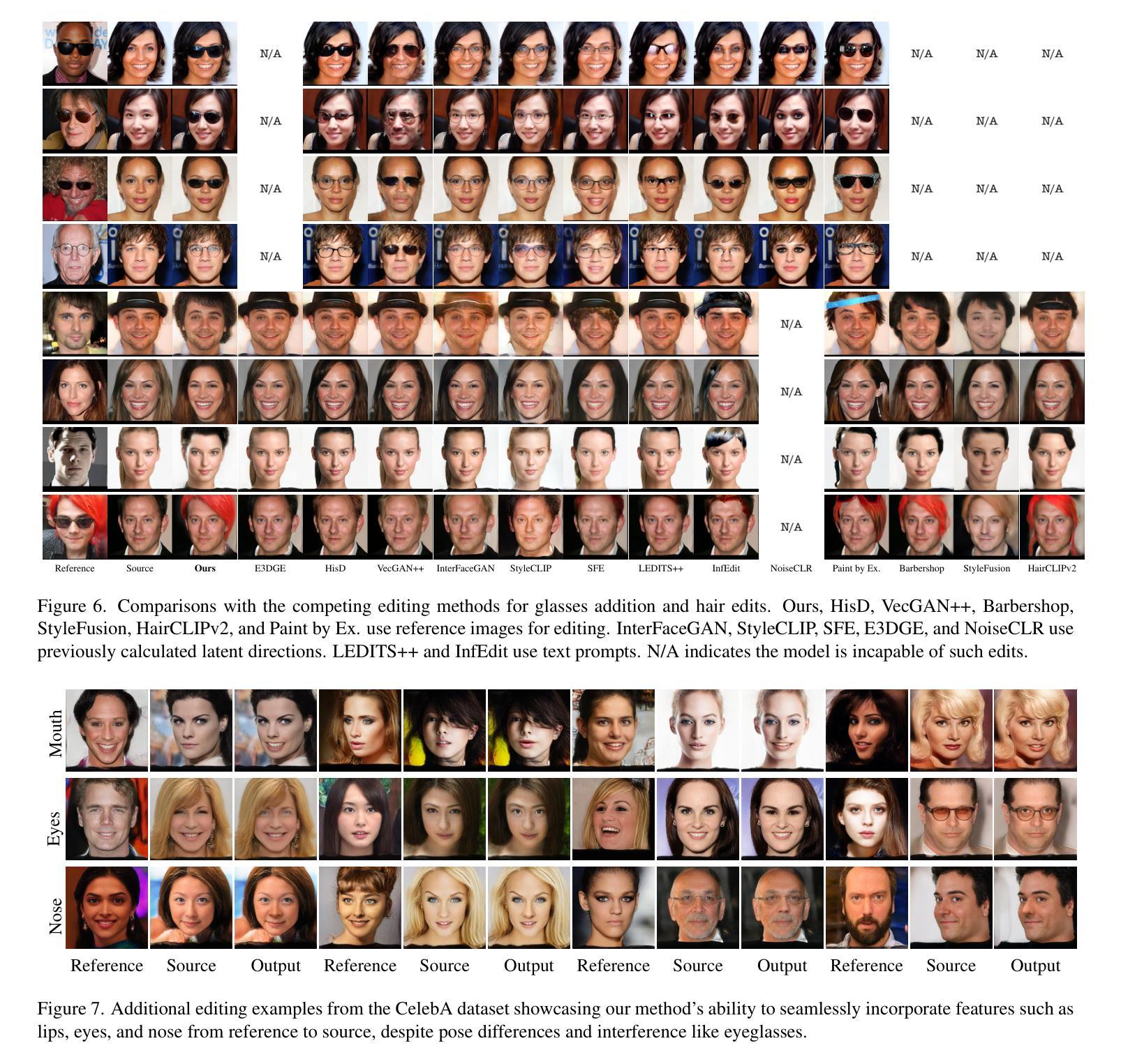