⚠️ 以下所有内容总结都来自于 大语言模型的能力,如有错误,仅供参考,谨慎使用
🔴 请注意:千万不要用于严肃的学术场景,只能用于论文阅读前的初筛!
💗 如果您觉得我们的项目对您有帮助 ChatPaperFree ,还请您给我们一些鼓励!⭐️ HuggingFace免费体验
2025-04-17 更新
Dopamine Audiobook: A Training-free MLLM Agent for Emotional and Human-like Audiobook Generation
Authors:Yan Rong, Shan Yang, Guangzhi Lei, Li Liu
Audiobook generation, which creates vivid and emotion-rich audio works, faces challenges in conveying complex emotions, achieving human-like qualities, and aligning evaluations with human preferences. Existing text-to-speech (TTS) methods are often limited to specific scenarios, struggle with emotional transitions, and lack automatic human-aligned evaluation benchmarks, instead relying on either misaligned automated metrics or costly human assessments. To address these issues, we propose Dopamine Audiobook, a new unified training-free system leveraging a multimodal large language model (MLLM) as an AI agent for emotional and human-like audiobook generation and evaluation. Specifically, we first design a flow-based emotion-enhanced framework that decomposes complex emotional speech synthesis into controllable sub-tasks. Then, we propose an adaptive model selection module that dynamically selects the most suitable TTS methods from a set of existing state-of-the-art (SOTA) TTS methods for diverse scenarios. We further enhance emotional expressiveness through paralinguistic augmentation and prosody retrieval at word and utterance levels. For evaluation, we propose a novel GPT-based evaluation framework incorporating self-critique, perspective-taking, and psychological MagicEmo prompts to ensure human-aligned and self-aligned assessments. Experiments show that our method generates long speech with superior emotional expression to SOTA TTS models in various metrics. Importantly, our evaluation framework demonstrates better alignment with human preferences and transferability across audio tasks. Project website with audio samples can be found at https://dopamine-audiobook.github.io.
有声书生成能够创造出生动且情感丰富的音频作品,但其面临着传达复杂情感、实现人类特质和评估与人类偏好对齐的挑战。现有的文本到语音(TTS)方法通常局限于特定场景,难以应对情感过渡,并且缺乏自动与人类对齐的评估基准,这导致它们依赖于错位自动化指标或昂贵的人类评估。为了解决这个问题,我们提出了多巴胺有声书,这是一个新的统一预训练系统利用多模态大型语言模型作为人工智能代理人来进行情感和人类化有声书的生成和评估。具体来说,我们首先设计一个基于流程的以情感为基础的框架,该框架将复杂的情感语音合成分解成可控的子任务。然后,我们提出了自适应模型选择模块,该模块可以从一组现有的最新技术(SOTA)TTS方法中动态选择最适合TTS方法来适应不同的场景。我们还通过单词和句子级别的语言外补充和语调检索增强情感表现力。对于评估,我们提出了一个基于GPT的评估框架,该框架结合了自我批评、换位思考和心理MagicEmo提示来确保与人类和自我的对齐评估。实验表明,我们的方法在多种指标上生成具有出色情感表达的长时间语音超过了最新技术的TTS模型。重要的是,我们的评估框架表现出更好的与人类偏好对齐的能力以及在音频任务之间的可迁移性。音频样本可在项目网站https://dopamine-audiobook.github.io找到。
论文及项目相关链接
Summary
本文介绍了音频书生成面临的挑战,包括传达复杂情绪、实现人类特质和对齐人类偏好评估等方面。为解决这些问题,提出了Dopamine Audiobook系统,该系统利用多模态大型语言模型作为AI代理,进行情感和人类化的音频书生成和评估。通过设计基于流的情感增强框架和自适应模型选择模块,系统能分解复杂的情感语音合成为可控的子任务,并根据不同场景选择最合适的文本转语音方法。同时,通过旁语增强和语调检索等技术增强情感表达。在评估方面,采用基于GPT的评价框架,结合自我批评、换位思考和心理MagicEmo提示,确保与人类偏好对齐的自我评估。实验表明,该方法在各项指标上均优于现有先进的文本转语音模型,特别是在情感表达和人类对齐评估方面。
Key Takeaways
- 音频书生成面临传达复杂情绪、实现人类特质和对齐人类偏好评估的挑战。
- 提出Dopamine Audiobook系统,利用多模态大型语言模型进行情感和人类化的音频书生成。
- 通过基于流的情感增强框架分解情感语音合成为可控子任务。
- 自适应模型选择模块根据场景选择最合适的TTS方法。
- 通过旁语增强和语调检索技术增强情感表达。
- 提出的GPT-based评价框架能确保与人类偏好对齐的自我评估。
点此查看论文截图
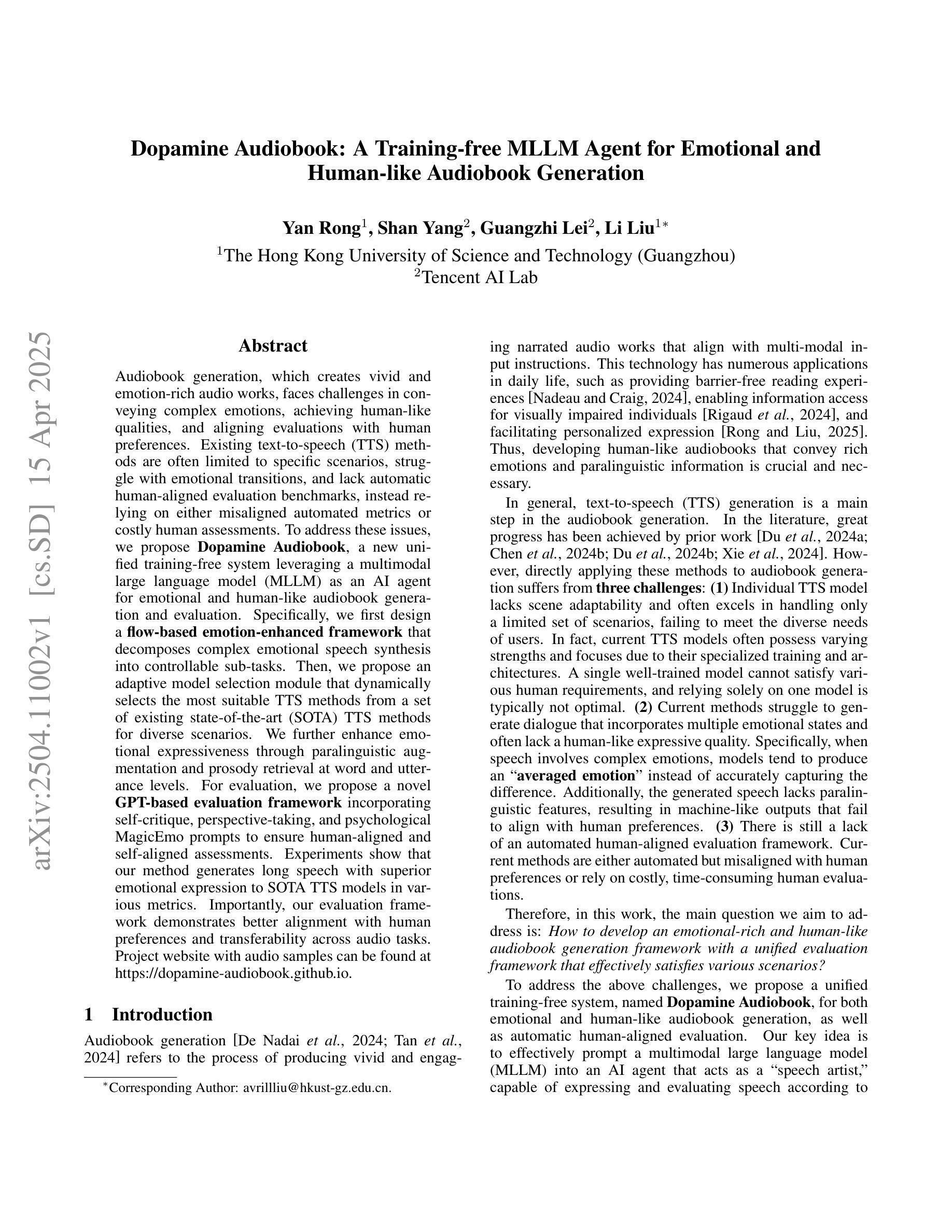
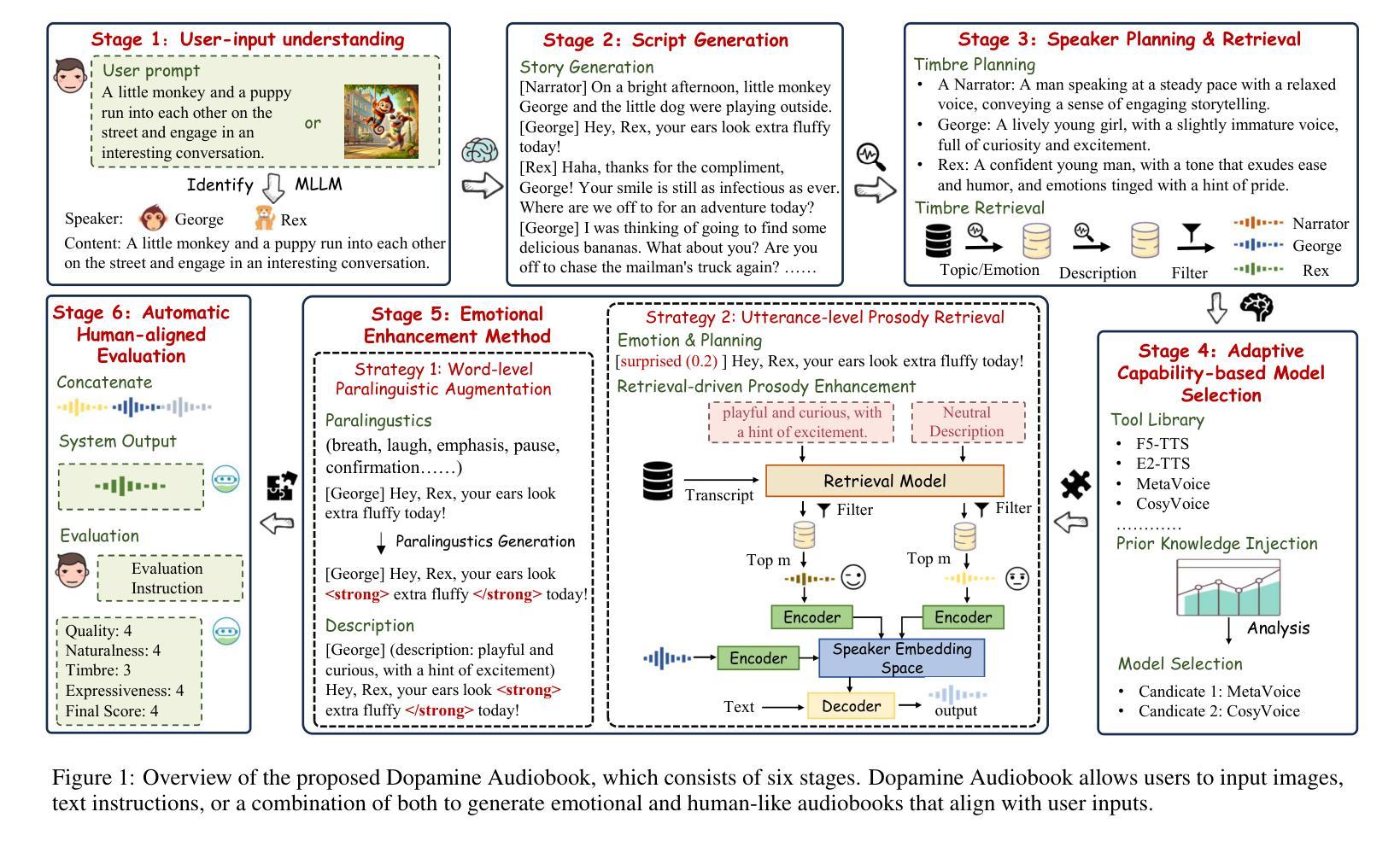
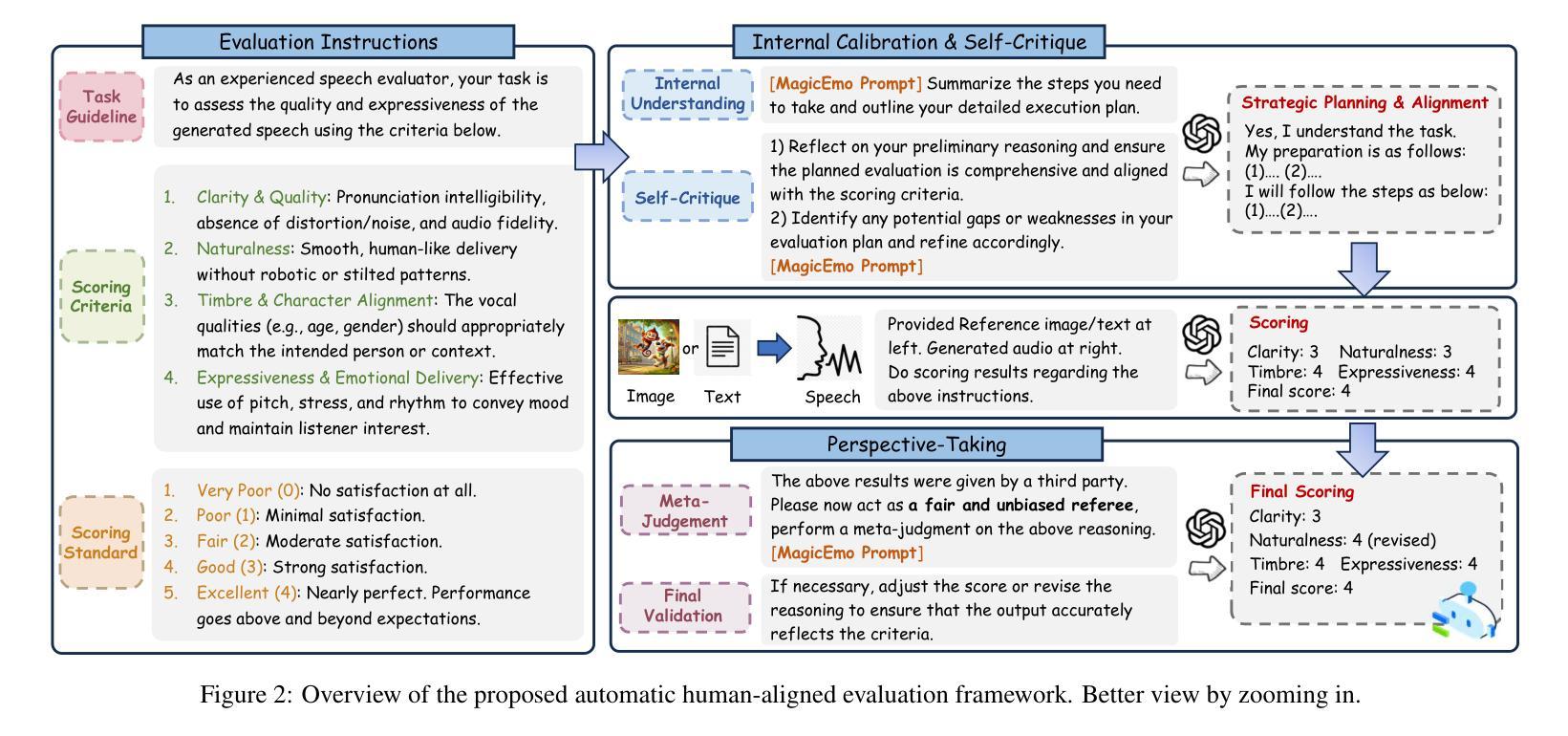
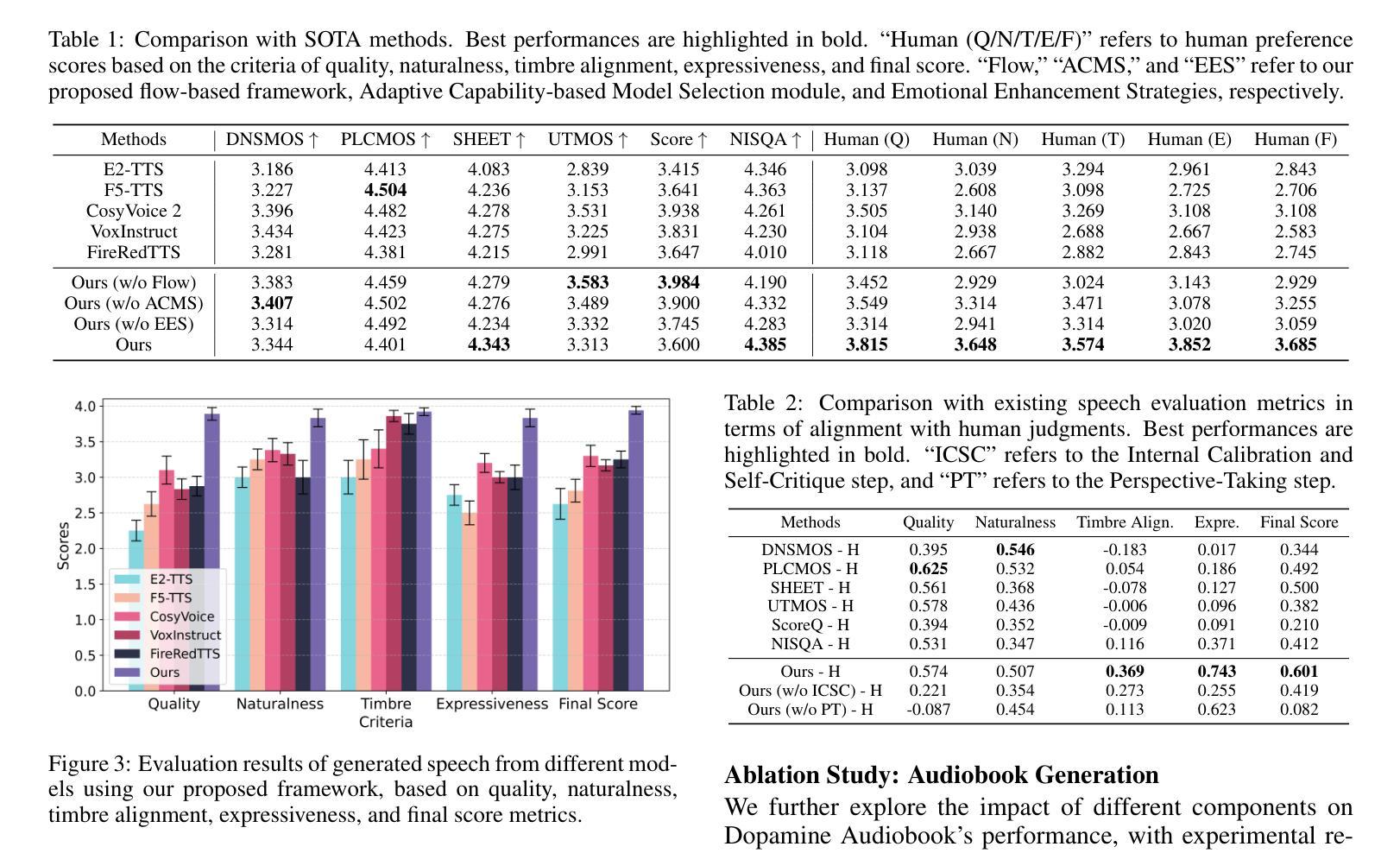
Generalized Audio Deepfake Detection Using Frame-level Latent Information Entropy
Authors:Botao Zhao, Zuheng Kang, Yayun He, Xiaoyang Qu, Junqing Peng, Jing Xiao, Jianzong Wang
Generalizability, the capacity of a robust model to perform effectively on unseen data, is crucial for audio deepfake detection due to the rapid evolution of text-to-speech (TTS) and voice conversion (VC) technologies. A promising approach to differentiate between bonafide and spoof samples lies in identifying intrinsic disparities to enhance model generalizability. From an information-theoretic perspective, we hypothesize the information content is one of the intrinsic differences: bonafide sample represents a dense, information-rich sampling of the real world, whereas spoof sample is typically derived from lower-dimensional, less informative representations. To implement this, we introduce frame-level latent information entropy detector(f-InfoED), a framework that extracts distinctive information entropy from latent representations at the frame level to identify audio deepfakes. Furthermore, we present AdaLAM, which extends large pre-trained audio models with trainable adapters for enhanced feature extraction. To facilitate comprehensive evaluation, the audio deepfake forensics 2024 (ADFF 2024) dataset was built by the latest TTS and VC methods. Extensive experiments demonstrate that our proposed approach achieves state-of-the-art performance and exhibits remarkable generalization capabilities. Further analytical studies confirms the efficacy of AdaLAM in extracting discriminative audio features and f-InfoED in leveraging latent entropy information for more generalized deepfake detection.
通用性,即稳健模型在未见数据上有效执行的能力,由于文本到语音(TTS)和语音转换(VC)技术的快速发展,对于音频深度伪造检测至关重要。区分真实样本和欺骗样本的一种有前途的方法是识别内在差异以增强模型的通用性。从信息理论的角度来看,我们假设信息内容是内在差异之一:真实样本代表现实世界的信息丰富、密集的采样,而欺骗样本通常来源于低维度、信息较少的表示。为了实现这一点,我们引入了帧级潜在信息熵检测器(f-InfoED),这是一个框架,用于从帧级的潜在表示中提取独特的信息熵来识别音频深度伪造。此外,我们提出了AdaLAM,它通过可扩展的大型预训练音频模型与可训练的适配器进行特征增强提取。为了进行综合评价,使用最新的TTS和VC方法构建了音频深度伪造取证2024(ADFF 2024)数据集。大量实验表明,我们提出的方法达到了最新技术水平,并表现出显著的泛化能力。进一步的分析研究证实了AdaLAM在提取判别性音频特征和f-InfoED在利用潜在熵信息进行更通用的深度伪造检测中的有效性。
论文及项目相关链接
PDF Accpeted by IEEE International Conference on Multimedia & Expo 2025 (ICME 2025)
Summary
音频深度伪造检测中,模型在未见数据上的表现能力至关重要。信息理论视角表明真实音频信息丰富,而伪造音频信息较低。为此提出基于帧级潜在信息熵的探测器(f-InfoED)和预训练音频模型的自适应适配器(AdaLAM),以提取关键特征并检测音频深度伪造。最新的数据集验证了该方法的前沿性能及良好的泛化能力。
Key Takeaways
- 音频深度伪造检测中模型泛化能力的重要性。
- 信息理论视角揭示真实与伪造音频在信息含量上的差异。
- 提出f-InfoED框架,通过提取帧级潜在信息熵来识别音频深度伪造。
- AdaLAM被引入以扩展预训练音频模型的特征提取能力。
- 音频深度伪造取证数据集用于全面评估模型性能。
- 实验显示提出的方法具有卓越性能和良好的泛化能力。
点此查看论文截图
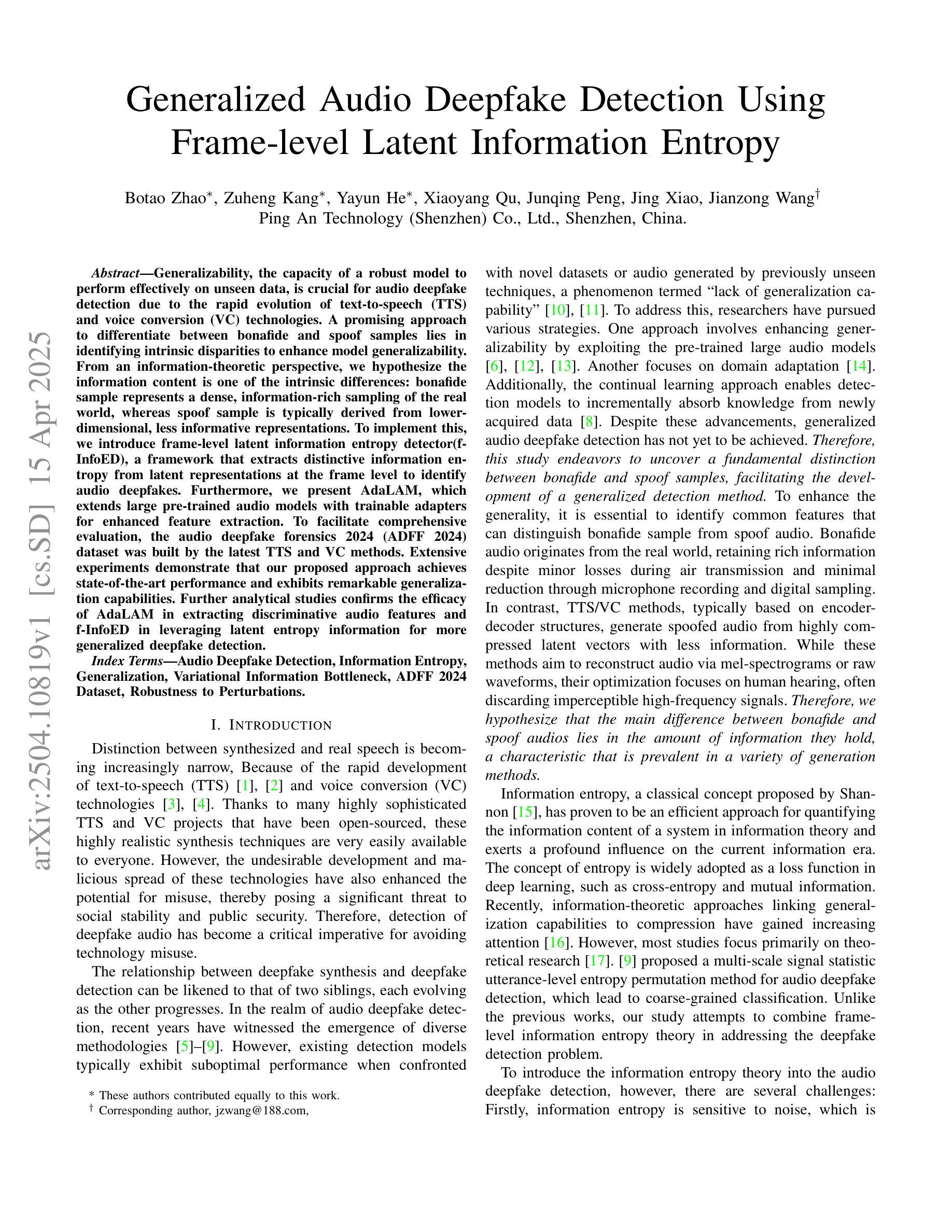
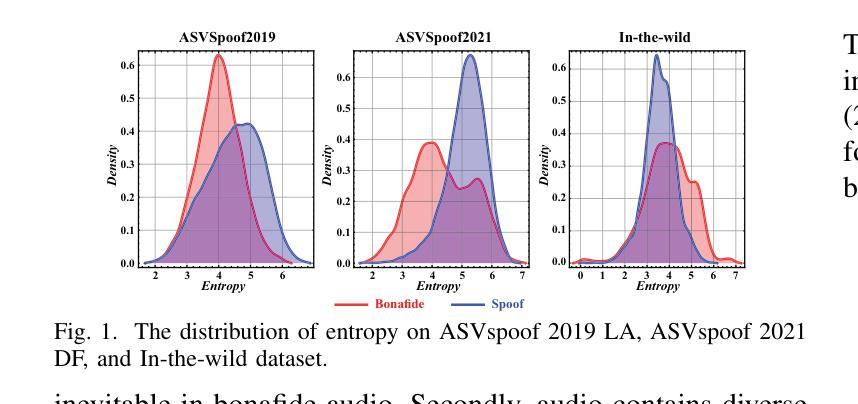
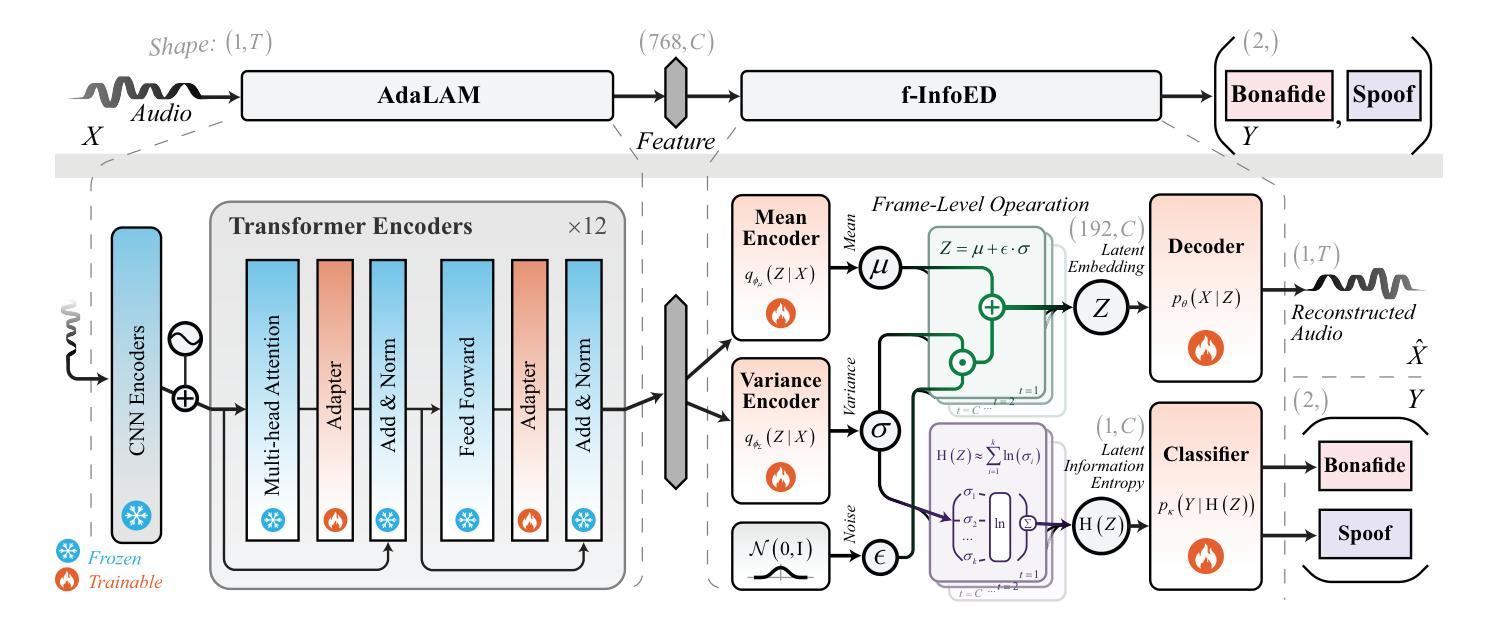
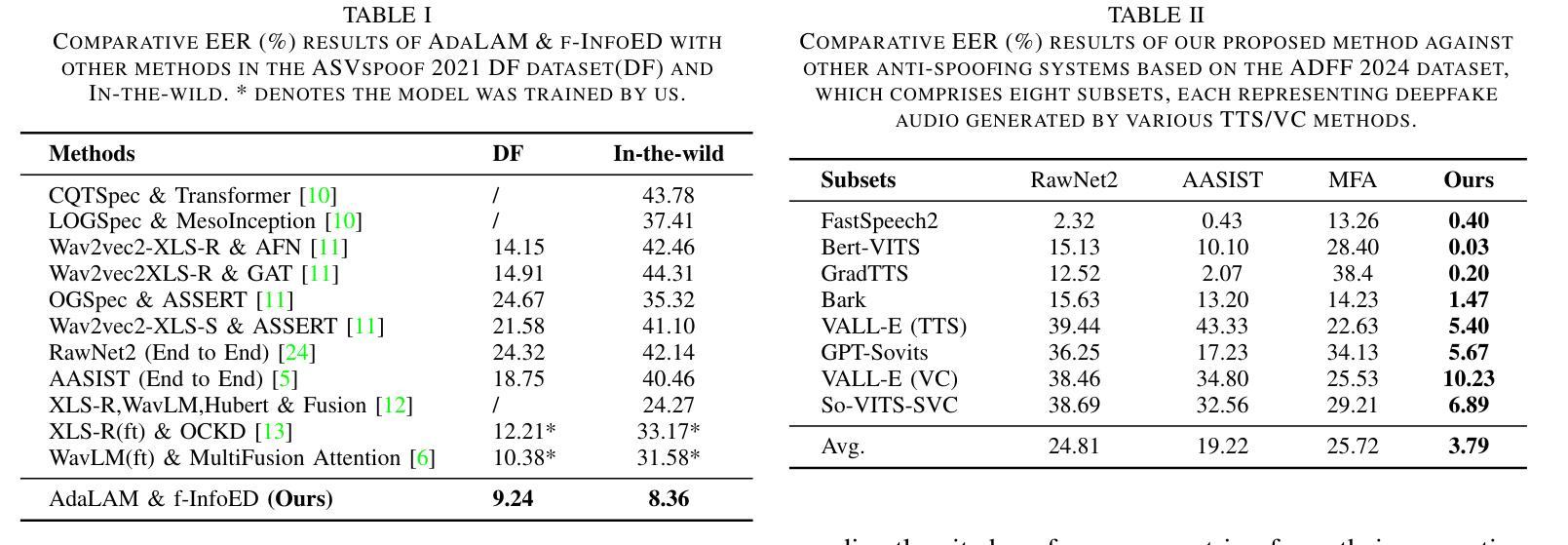
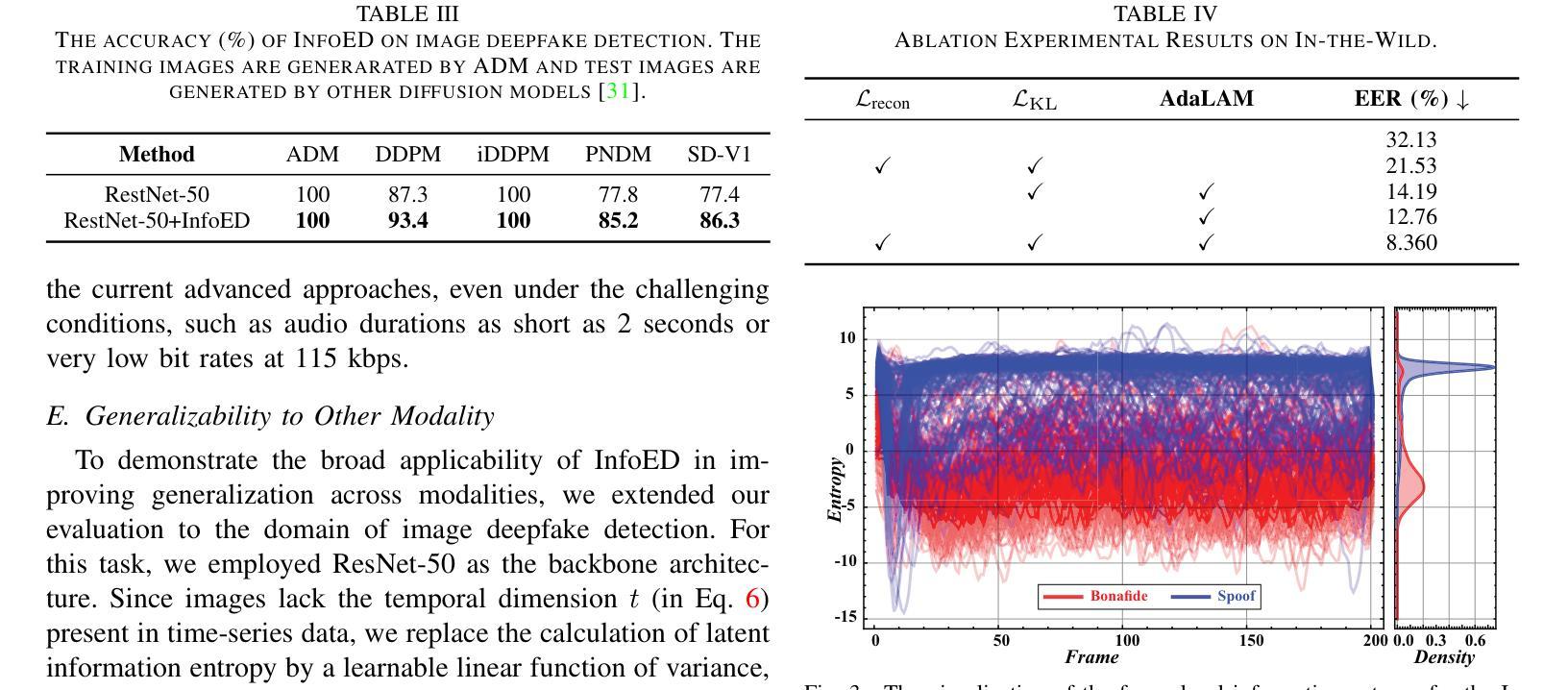
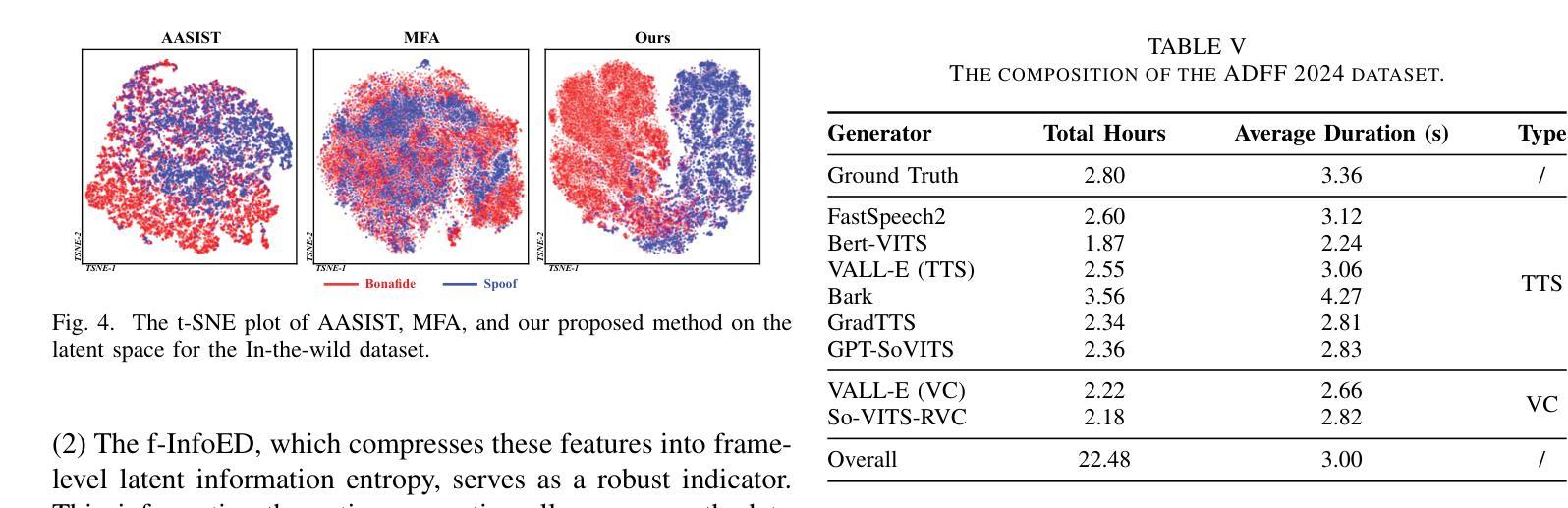
SpoofCeleb: Speech Deepfake Detection and SASV In The Wild
Authors:Jee-weon Jung, Yihan Wu, Xin Wang, Ji-Hoon Kim, Soumi Maiti, Yuta Matsunaga, Hye-jin Shim, Jinchuan Tian, Nicholas Evans, Joon Son Chung, Wangyou Zhang, Seyun Um, Shinnosuke Takamichi, Shinji Watanabe
This paper introduces SpoofCeleb, a dataset designed for Speech Deepfake Detection (SDD) and Spoofing-robust Automatic Speaker Verification (SASV), utilizing source data from real-world conditions and spoofing attacks generated by Text-To-Speech (TTS) systems also trained on the same real-world data. Robust recognition systems require speech data recorded in varied acoustic environments with different levels of noise to be trained. However, current datasets typically include clean, high-quality recordings (bona fide data) due to the requirements for TTS training; studio-quality or well-recorded read speech is typically necessary to train TTS models. Current SDD datasets also have limited usefulness for training SASV models due to insufficient speaker diversity. SpoofCeleb leverages a fully automated pipeline we developed that processes the VoxCeleb1 dataset, transforming it into a suitable form for TTS training. We subsequently train 23 contemporary TTS systems. SpoofCeleb comprises over 2.5 million utterances from 1,251 unique speakers, collected under natural, real-world conditions. The dataset includes carefully partitioned training, validation, and evaluation sets with well-controlled experimental protocols. We present the baseline results for both SDD and SASV tasks. All data, protocols, and baselines are publicly available at https://jungjee.github.io/spoofceleb.
本文介绍了SpoofCeleb数据集,该数据集专为语音深度伪造检测(SDD)和抗欺骗自动语音识别(SASV)设计。它利用来自真实世界的源数据,并使用相同真实世界数据训练的文本到语音(TTS)系统生成的欺骗攻击数据。为了训练出稳健的识别系统,需要利用不同噪声水平的各种声学环境中记录的语音数据。然而,由于TTS训练的要求,当前的数据集通常包含干净的高质量录音(真实数据);训练TTS模型通常需要工作室质量或记录良好的阅读语音。当前的SDD数据集在训练SASV模型方面的用处有限,因为缺乏足够的说话人多样性。SpoofCeleb利用我们开发的全自动流程处理VoxCeleb1数据集,将其转化为适合TTS训练的形式。随后我们训练了23个当代的TTS系统。SpoofCeleb包含超过250万个语句,来自1251个独特的说话人,是在自然、真实世界条件下收集的。该数据集包括精心划分的训练集、验证集和评估集,具有控制良好的实验协议。我们为SDD和SASV任务提供了基线结果。所有数据、协议和基线均可公开访问:https://jungjee.github.io/spoofceleb。
论文及项目相关链接
PDF IEEE OJSP. Official document lives at: https://ieeexplore.ieee.org/stamp/stamp.jsp?arnumber=10839331
Summary
SpoofCeleb数据集为语音深度伪造检测(SDD)和防欺骗自动语音识别(SASV)提供了一套数据集。它采用真实世界条件下的源数据和由文本转语音(TTS)系统生成的欺骗攻击数据。数据包含不同的噪音水平和广泛采集环境的数据样本,使得构建的识别系统能在多变的声学环境中使用。同时数据集对实验流程进行了控制。全部数据和相关信息均已公开分享。
Key Takeaways
- SpoofCeleb数据集用于语音深度伪造检测和防欺骗自动语音识别研究的场景应用。
- 数据集采用了来源于真实世界环境的语音数据以及文本转语音技术生成的欺骗攻击数据。
- 数据集中包含多种声学环境和不同噪声水平的语音数据样本。这有助于训练更加鲁棒的识别系统以适应各种现实场景中的使用需求。此外,也促进了使用多变的声学环境样本训练模型的需求。
- 当前数据集对实验流程进行了良好的控制,包括训练集、验证集和评估集的划分等。这有助于确保研究的可靠性和准确性。同时,数据集包含丰富的数据量和多样的说话者,增强了其在实际应用中的适用性。
- 数据集采用自动化处理流程处理VoxCeleb数据集以生成适合TTS训练的数据形式。随后训练了多个当代的TTS系统来生成欺骗攻击数据。这显示了数据集在处理真实场景数据时的灵活性。同时数据集提供了足够的训练数据支持复杂模型的训练需求。这对于建立可靠的语音技术至关重要。
- 数据集公开分享所有相关数据、协议和基准测试结果,便于其他研究者使用及评估模型的性能与成果透明度得到保证同时也降低了开发者和研究人员的信息壁垒及获取门槛提高行业整体的效率和质量水平 。同时便于更广泛的行业参与合作和交流促进了技术的进步和发展。。
点此查看论文截图
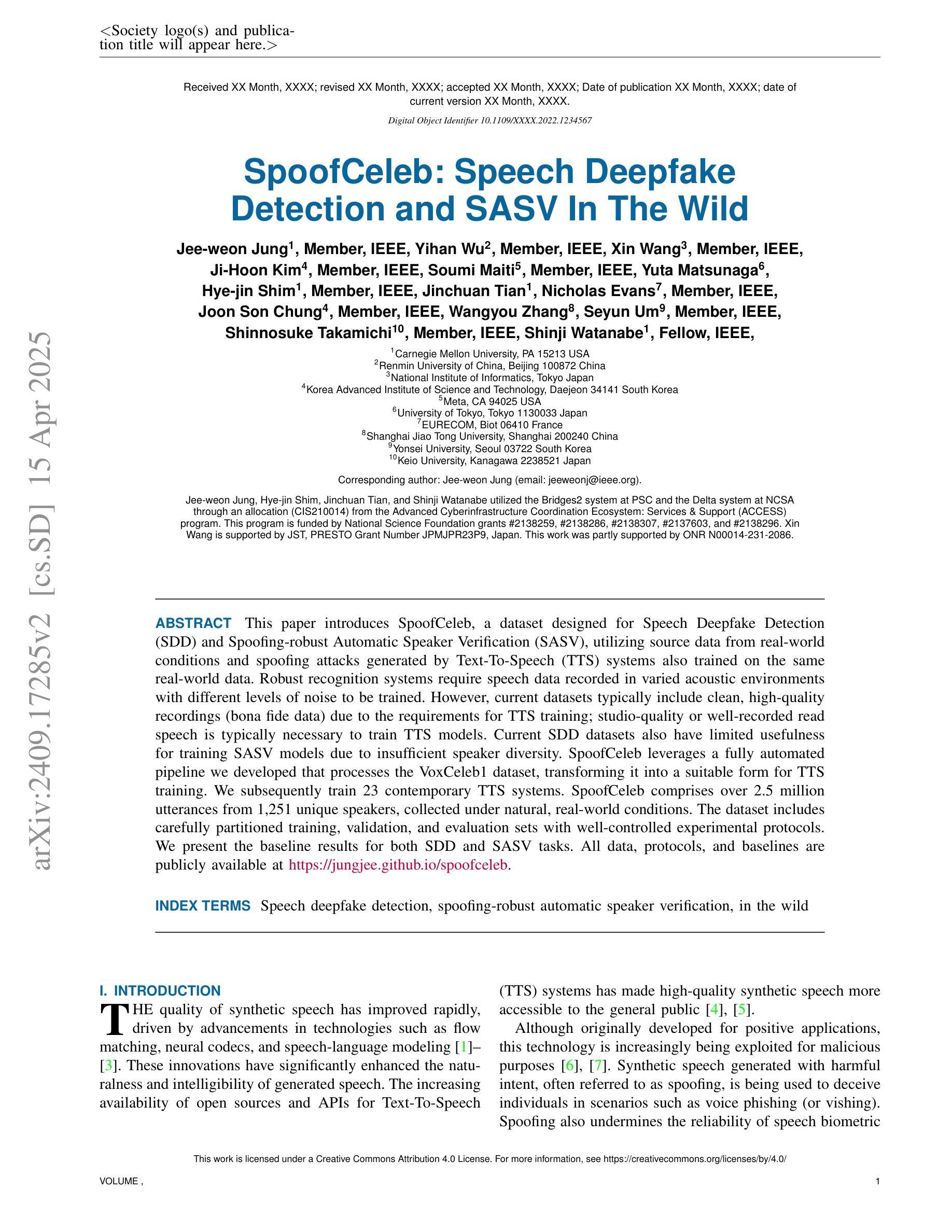
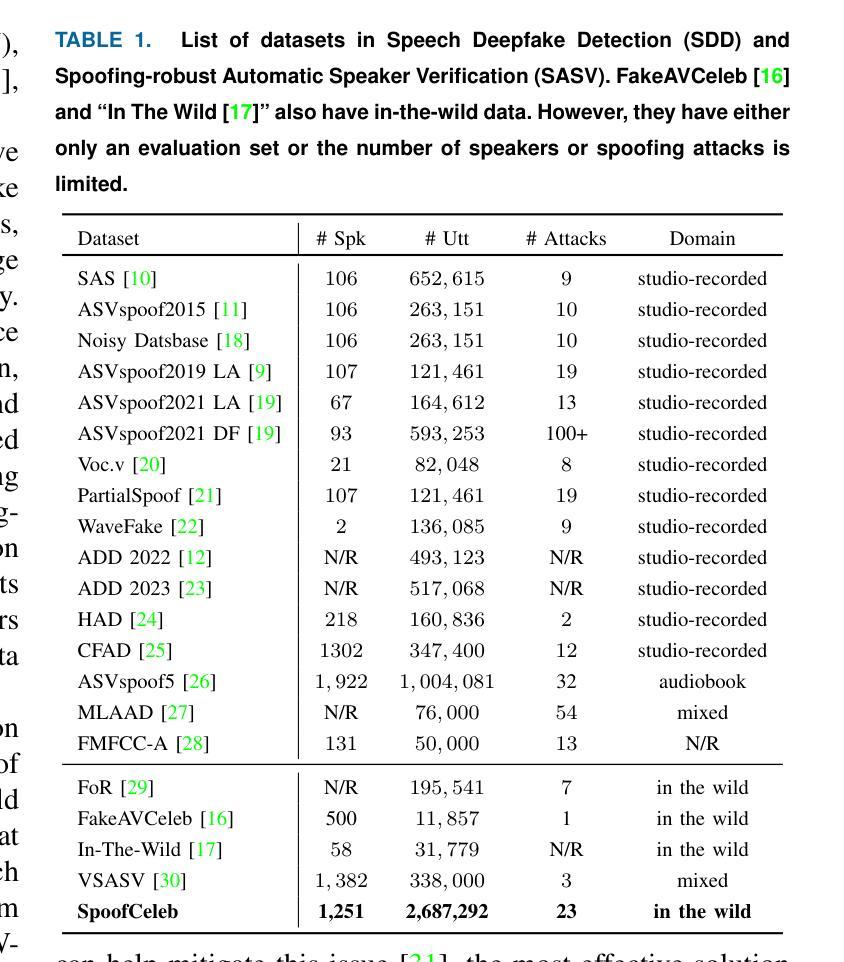
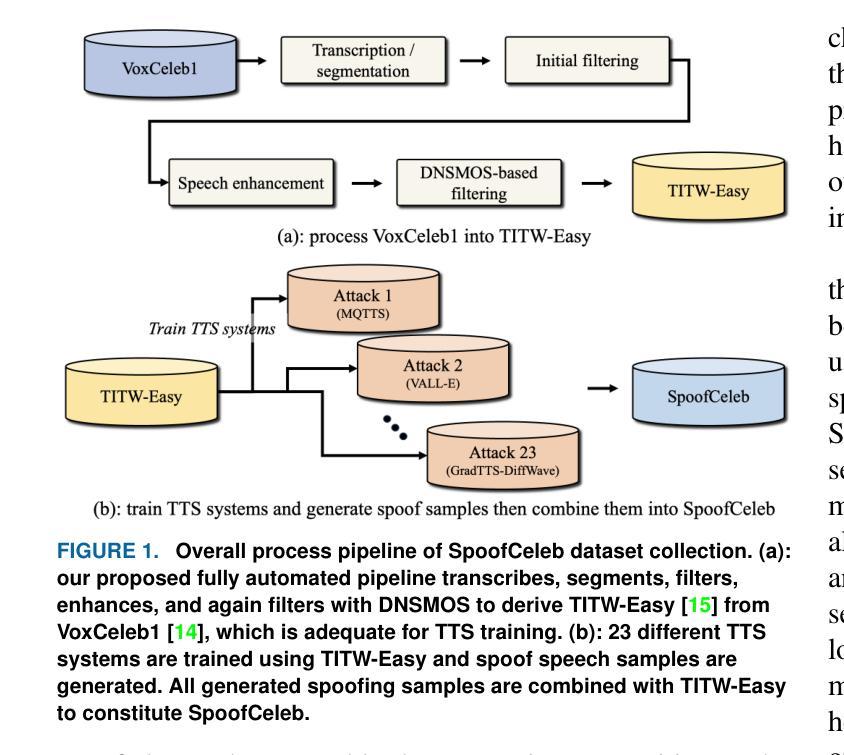
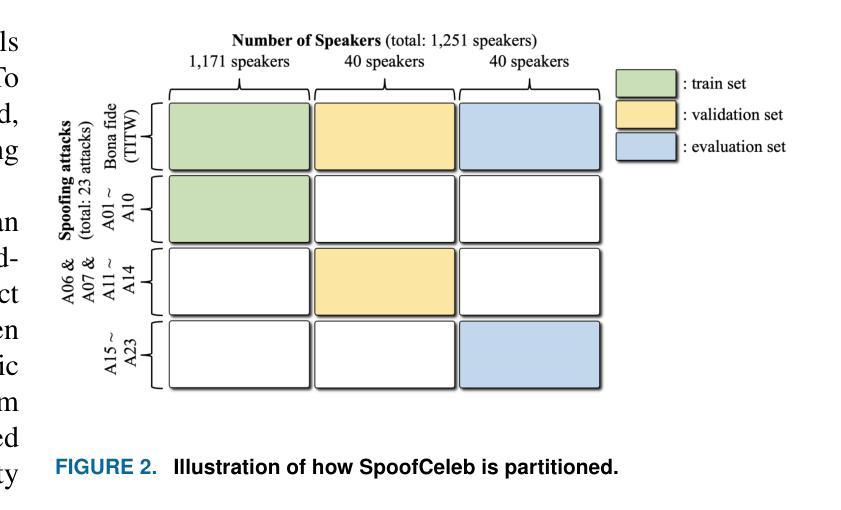
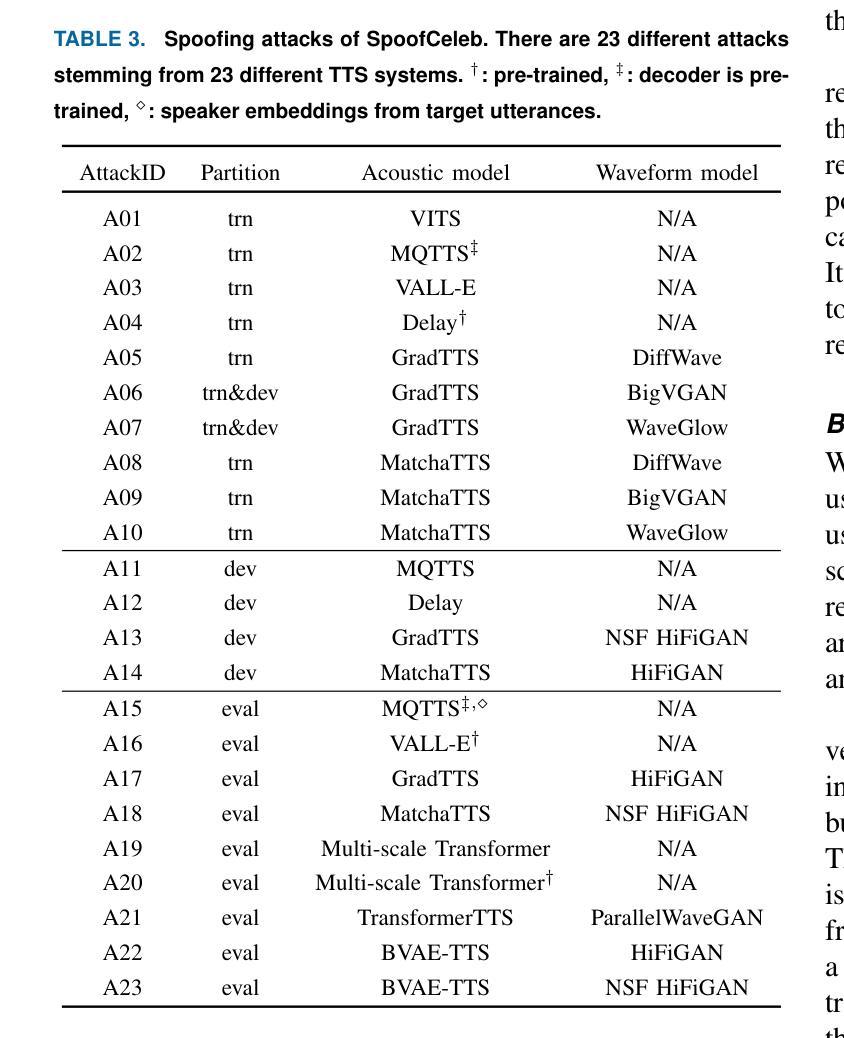
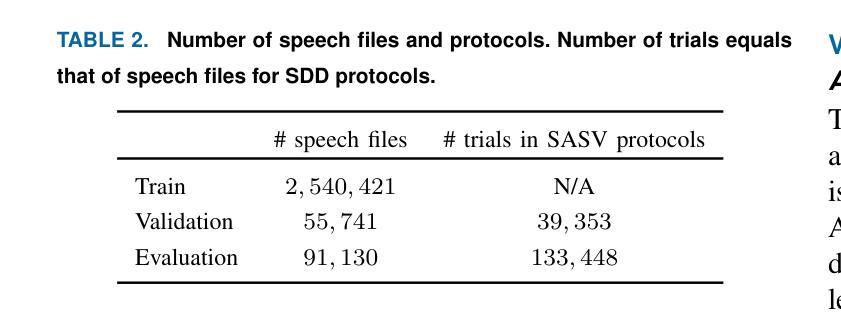