⚠️ 以下所有内容总结都来自于 大语言模型的能力,如有错误,仅供参考,谨慎使用
🔴 请注意:千万不要用于严肃的学术场景,只能用于论文阅读前的初筛!
💗 如果您觉得我们的项目对您有帮助 ChatPaperFree ,还请您给我们一些鼓励!⭐️ HuggingFace免费体验
2025-04-18 更新
Cross-Frequency Collaborative Training Network and Dataset for Semi-supervised First Molar Root Canal Segmentation
Authors:Zhenhuan Zhou, Yuchen Zhang, Along He, Peng Wang, Xueshuo Xie, Tao Li
Root canal (RC) treatment is a highly delicate and technically complex procedure in clinical practice, heavily influenced by the clinicians’ experience and subjective judgment. Deep learning has made significant advancements in the field of computer-aided diagnosis (CAD) because it can provide more objective and accurate diagnostic results. However, its application in RC treatment is still relatively rare, mainly due to the lack of public datasets in this field. To address this issue, in this paper, we established a First Molar Root Canal segmentation dataset called FMRC-2025. Additionally, to alleviate the workload of manual annotation for dentists and fully leverage the unlabeled data, we designed a Cross-Frequency Collaborative training semi-supervised learning (SSL) Network called CFC-Net. It consists of two components: (1) Cross-Frequency Collaborative Mean Teacher (CFC-MT), which introduces two specialized students (SS) and one comprehensive teacher (CT) for collaborative multi-frequency training. The CT and SS are trained on different frequency components while fully integrating multi-frequency knowledge through cross and full frequency consistency supervisions. (2) Uncertainty-guided Cross-Frequency Mix (UCF-Mix) mechanism enables the network to generate high-confidence pseudo-labels while learning to integrate multi-frequency information and maintaining the structural integrity of the targets. Extensive experiments on FMRC-2025 and three public dental datasets demonstrate that CFC-MT is effective for RC segmentation and can also exhibit strong generalizability on other dental segmentation tasks, outperforming state-of-the-art SSL medical image segmentation methods. Codes and dataset will be released.
根管治疗(RC)是一种在临床上非常精细且技术复杂的程序,深受临床医生经验和主观判断的影响。深度学习在计算机辅助诊断(CAD)领域取得了显著进展,因为它能提供更客观和准确的诊断结果。然而,其在根管治疗中的应用仍然相对罕见,主要是由于该领域缺乏公共数据集。为解决这一问题,本文建立了一个名为FMRC-2025的第一磨牙根管数据集。此外,为了减轻牙医手动标注的工作量并充分利用未标记的数据,我们设计了一种跨频率协同训练半监督学习(SSL)网络,称为CFC-Net。它包含两个组件:(1)跨频率协同均值教师(CFC-MT),它引入了两个专门的学生(SS)和一个全面的教师(CT)进行协同多频率训练。全面教师和学生在不同的频率成分上进行训练,同时通过跨频率和全程一致性监督来完全整合多频率知识。(2)不确定性引导的跨频率混合(UCF-Mix)机制使网络能够在学习整合多频率信息的同时,生成高置信度的伪标签,并保持目标的结构完整性。在FMRC-2025和三个公共牙科数据集上的大量实验表明,CFC-MT对于根管治疗分割是有效的,并且在其他牙科分割任务上也表现出强大的泛化能力,超越了最先进的SSL医学图像分割方法。代码和数据集将公开发布。
论文及项目相关链接
PDF 12 pages, Initial submission time 25 December 2024, Now Under Review
摘要
本文介绍了根管治疗(RC)的临床实践中的复杂性和重要性,强调临床医生经验和主观判断对治疗的影响。文章提出了建立第一个名为FMRC-2025的磨牙根管分割数据集的问题,并设计了名为CFC-Net的跨频协同训练半监督学习网络来辅助牙医进行手动标注并充分利用未标注数据。该网络包括两个组件:跨频协同均值教师(CFC-MT)和不确定性引导跨频混合(UCF-Mix)机制。实验证明,CFC-MT在根管分割上有效,并表现出在其他牙科分割任务上的强大泛化能力,优于最新的SSL医学图像分割方法。
关键见解
- 根管治疗是一项复杂且技术性强的临床程序,受到医生经验和主观判断的重大影响。
- 深度学习在计算机辅助诊断(CAD)领域取得了重大进展,但在根管治疗中的应用仍然相对较少。
- 缺乏公共数据集是限制深度学习在根管治疗应用的主要原因之一。
- 建立了名为FMRC-2025的磨牙根管分割数据集。
- 提出了名为CFC-Net的跨频协同训练半监督学习网络,包括两个组件:跨频协同均值教师和不确定性引导跨频混合机制。
- 实验证明,该网络在根管分割任务上表现优异,并具备强大的泛化能力。
点此查看论文截图
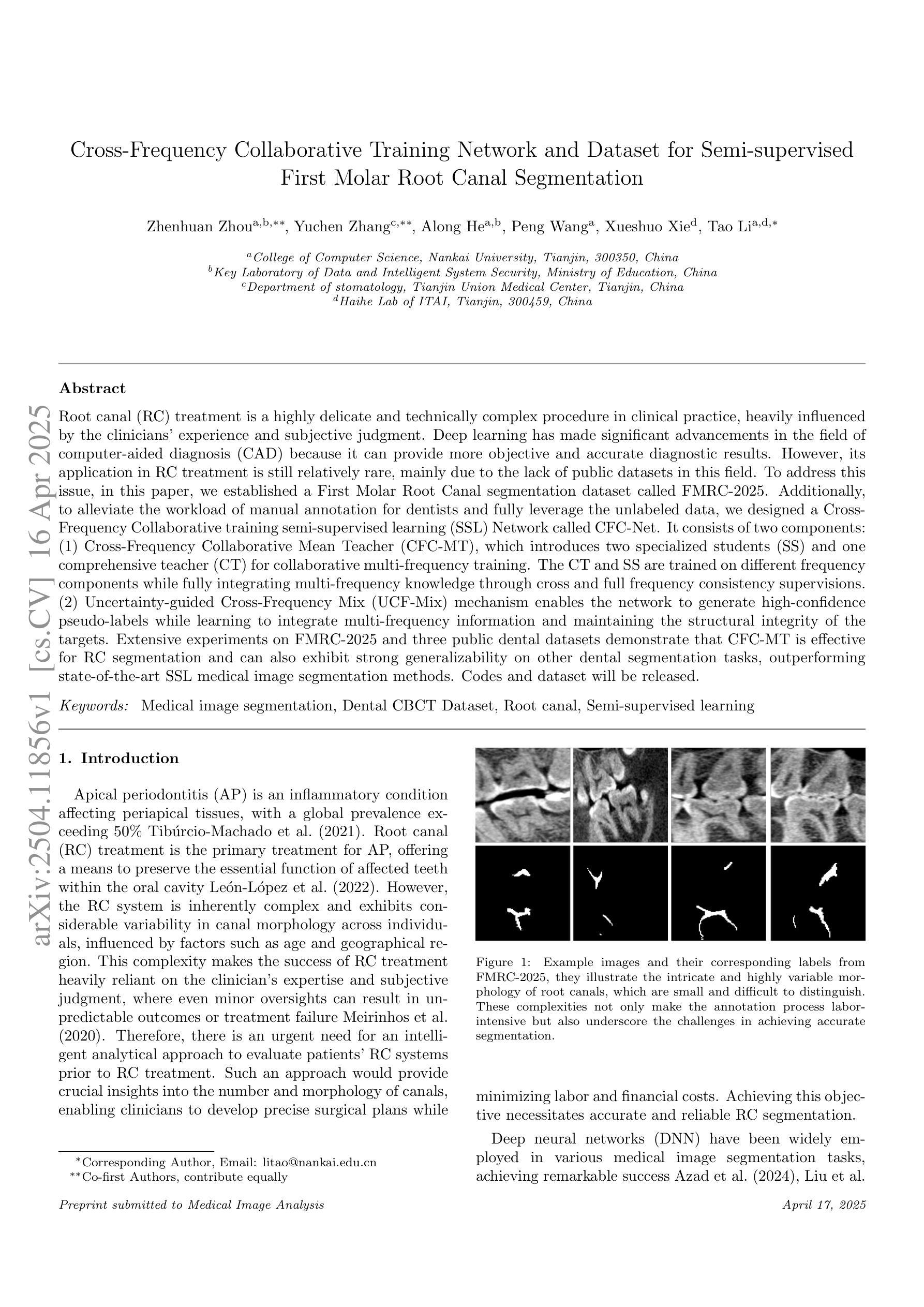
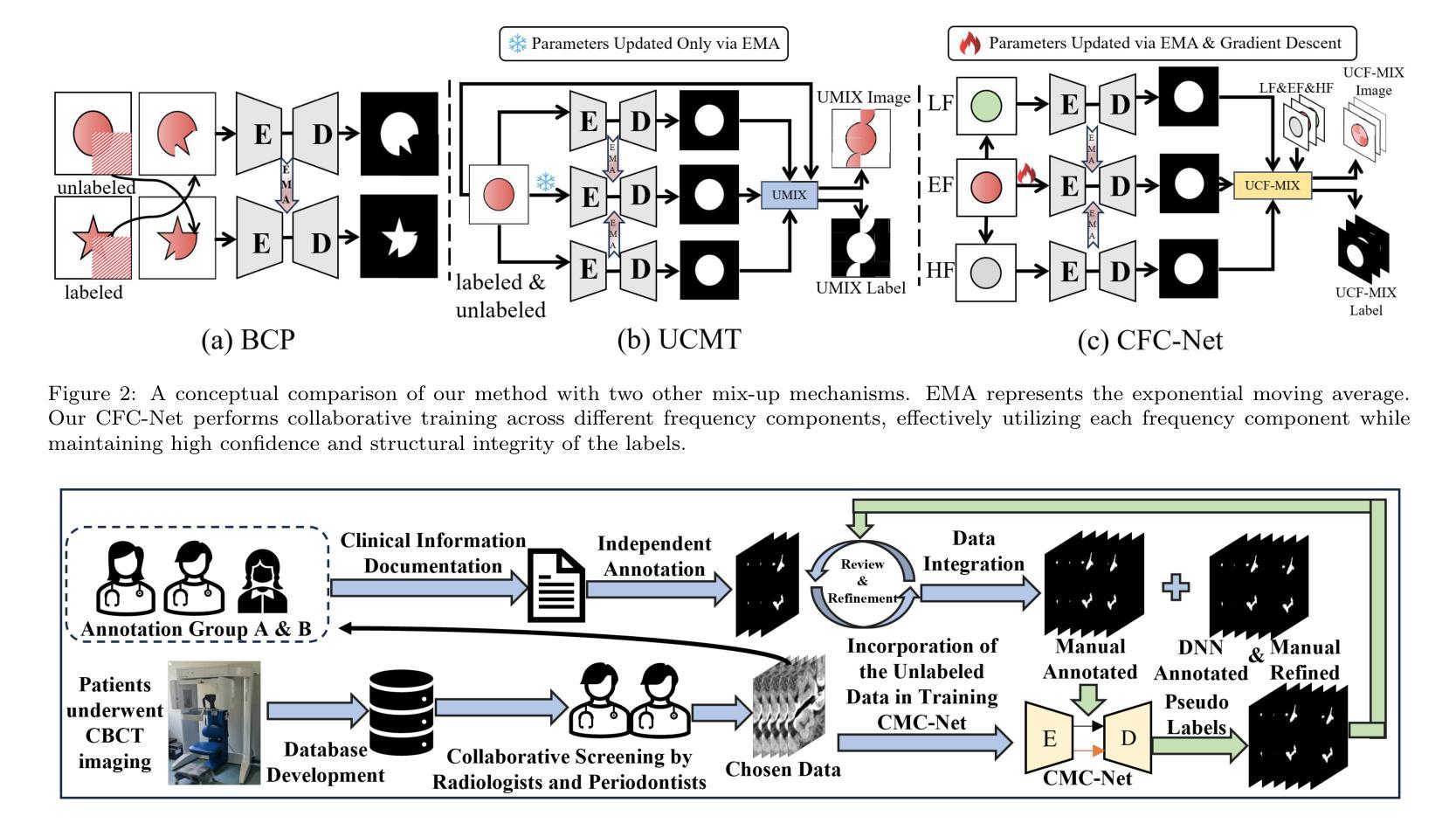
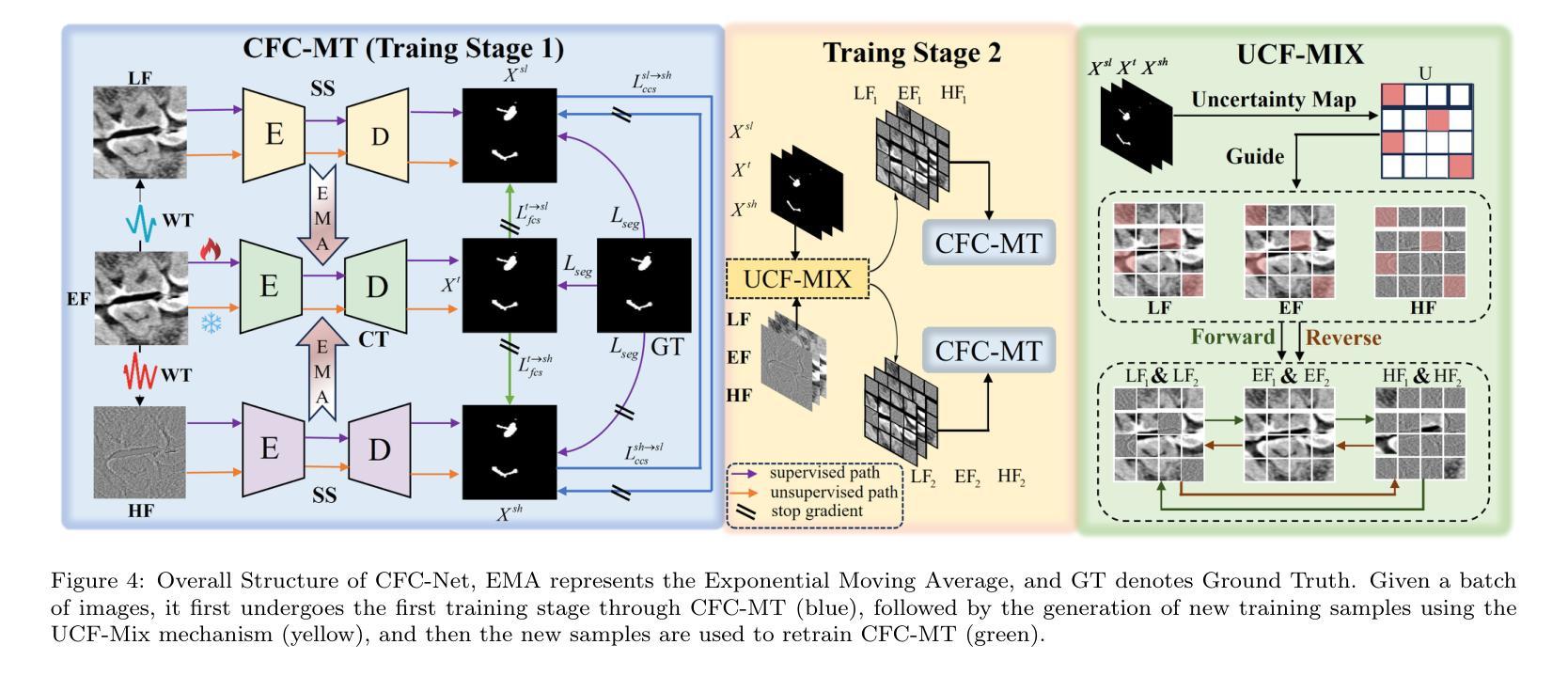