⚠️ 以下所有内容总结都来自于 大语言模型的能力,如有错误,仅供参考,谨慎使用
🔴 请注意:千万不要用于严肃的学术场景,只能用于论文阅读前的初筛!
💗 如果您觉得我们的项目对您有帮助 ChatPaperFree ,还请您给我们一些鼓励!⭐️ HuggingFace免费体验
2025-04-19 更新
Real-time High-fidelity Gaussian Human Avatars with Position-based Interpolation of Spatially Distributed MLPs
Authors:Youyi Zhan, Tianjia Shao, Yin Yang, Kun Zhou
Many works have succeeded in reconstructing Gaussian human avatars from multi-view videos. However, they either struggle to capture pose-dependent appearance details with a single MLP, or rely on a computationally intensive neural network to reconstruct high-fidelity appearance but with rendering performance degraded to non-real-time. We propose a novel Gaussian human avatar representation that can reconstruct high-fidelity pose-dependence appearance with details and meanwhile can be rendered in real time. Our Gaussian avatar is empowered by spatially distributed MLPs which are explicitly located on different positions on human body. The parameters stored in each Gaussian are obtained by interpolating from the outputs of its nearby MLPs based on their distances. To avoid undesired smooth Gaussian property changing during interpolation, for each Gaussian we define a set of Gaussian offset basis, and a linear combination of basis represents the Gaussian property offsets relative to the neutral properties. Then we propose to let the MLPs output a set of coefficients corresponding to the basis. In this way, although Gaussian coefficients are derived from interpolation and change smoothly, the Gaussian offset basis is learned freely without constraints. The smoothly varying coefficients combined with freely learned basis can still produce distinctly different Gaussian property offsets, allowing the ability to learn high-frequency spatial signals. We further use control points to constrain the Gaussians distributed on a surface layer rather than allowing them to be irregularly distributed inside the body, to help the human avatar generalize better when animated under novel poses. Compared to the state-of-the-art method, our method achieves better appearance quality with finer details while the rendering speed is significantly faster under novel views and novel poses.
许多工作已成功从多视角视频重建高斯人体化身。然而,它们要么使用单个多层感知器(MLP)难以捕捉姿势相关的外观细节,要么依赖计算密集型的神经网络来重建高保真外观,但渲染性能降低至非实时。我们提出了一种新型的高斯人体化身表示法,可以重建高保真姿势依赖的外观并带有细节,同时可以进行实时渲染。我们的高斯化身由空间分布的多层感知器赋予能力,这些感知器明确位于人体上的不同位置。每个高斯中的参数是通过根据其附近感知器的输出和距离进行插值获得的。为了避免插值过程中产生不希望有的平滑高斯属性变化,对于每个高斯,我们定义了一组高斯偏移基,基的线性组合表示相对于中性属性的高斯属性偏移。然后,我们提出让感知器输出对应于基的一组系数。通过这种方式,虽然高斯系数是通过插值得出的并平滑变化,但高斯偏移基可以自由地学习而没有约束。平滑变化的系数与自由学习的基相结合,仍然可以产生明显不同的高斯属性偏移,从而能够学习高频空间信号。我们进一步使用控制点来约束分布在表面层上的高斯,而不是允许它们在体内不规则分布,以帮助人体化身在新型姿势下的动画表现时更好地泛化。与最先进的方法相比,我们的方法在细节上达到了更好的外观质量,并且在新型视角和姿势下的渲染速度明显更快。
论文及项目相关链接
PDF CVPR 2025
Summary
本文提出一种新型的高斯人类角色表示方法,能够在重建高质量、姿态依赖的外观细节的同时实现实时渲染。该方法通过分布在身体上的多个MLP模型来增强高斯角色,并使用插值算法获取高斯参数。为避免出现插值过程中的平滑高斯属性变化,引入高斯偏移基,通过线性组合表示相对于中性属性的高斯属性偏移。此方法能够在重建过程中学习高频空间信号,并通过控制点约束高斯在表面层上的分布,提高角色在新型姿态下的泛化性能。相较于现有技术,该方法在外观质量和细节表现上更优,同时在新型视角和姿态下的渲染速度更快。
Key Takeaways
- 提出一种新型的高斯人类角色表示方法,能够重建高质量、姿态依赖的外观细节。
- 通过多个分布在身体上的MLP模型增强高斯角色,实现实时渲染。
- 使用插值算法获取高斯参数,引入高斯偏移基以避免插值过程中的平滑属性变化。
- 通过线性组合表示高斯属性偏移,能够在重建过程中学习高频空间信号。
- 通过控制点约束高斯在表面层上的分布,提高角色在新型姿态下的泛化性能。
- 与现有技术相比,该方法在外观质量和细节表现上更优。
点此查看论文截图
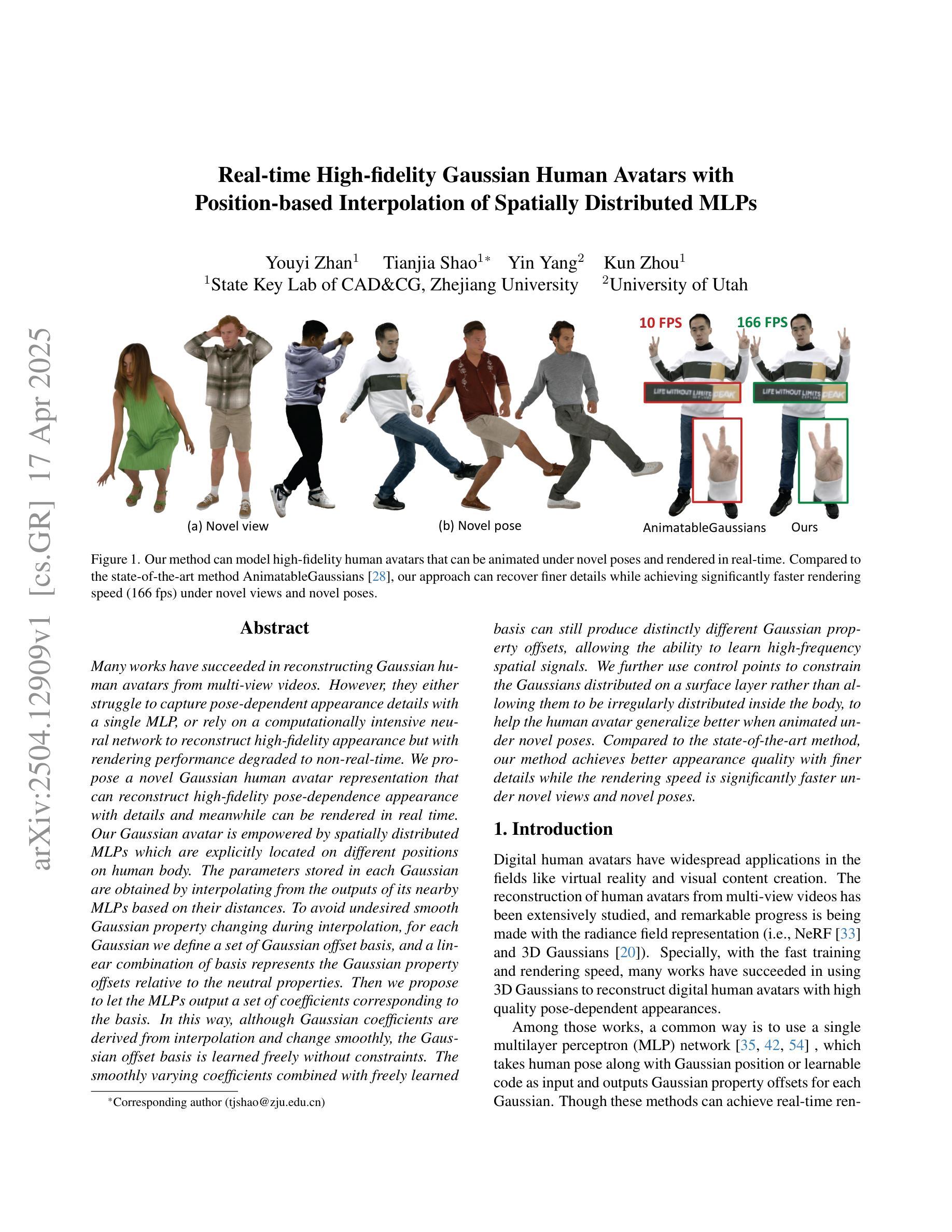
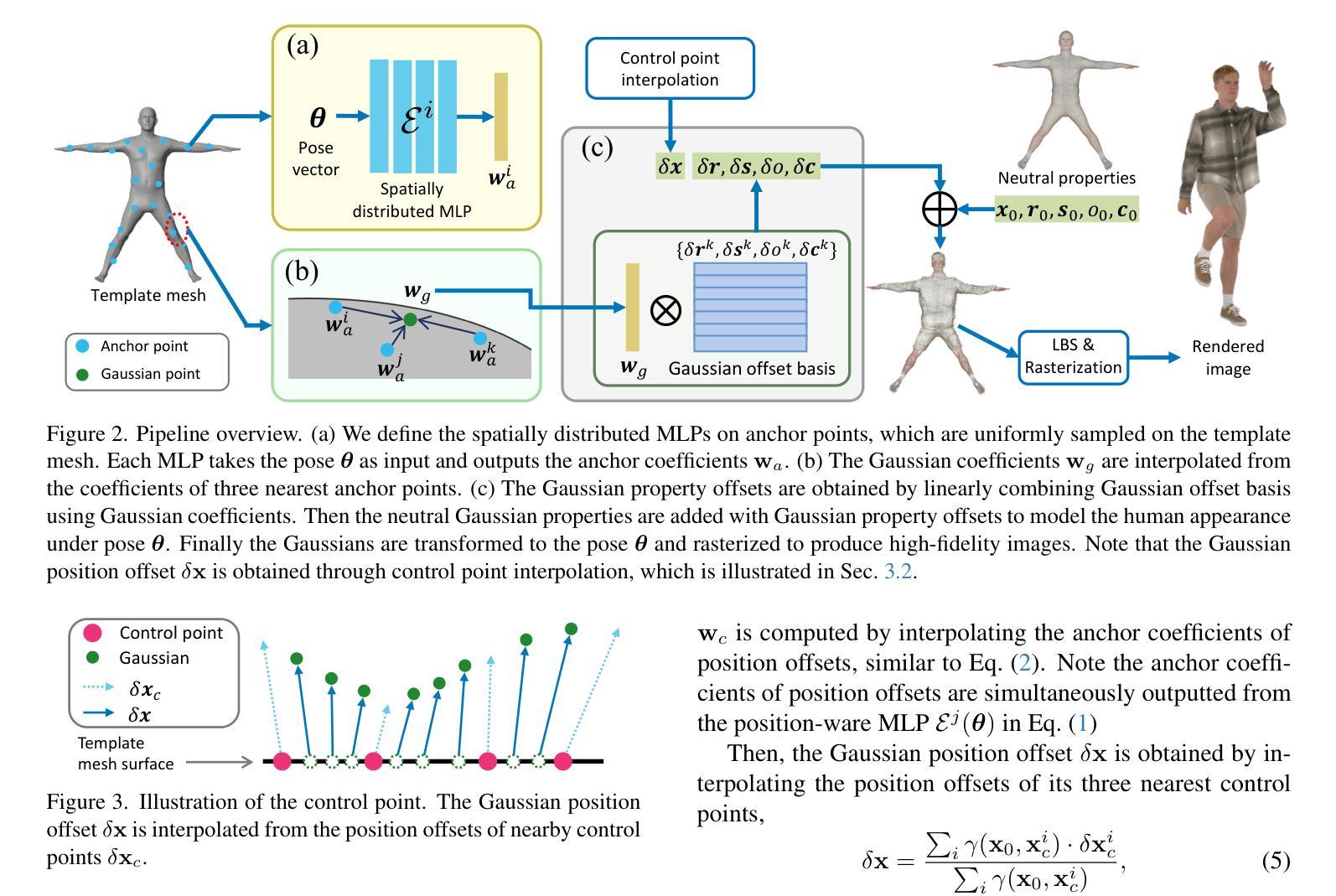
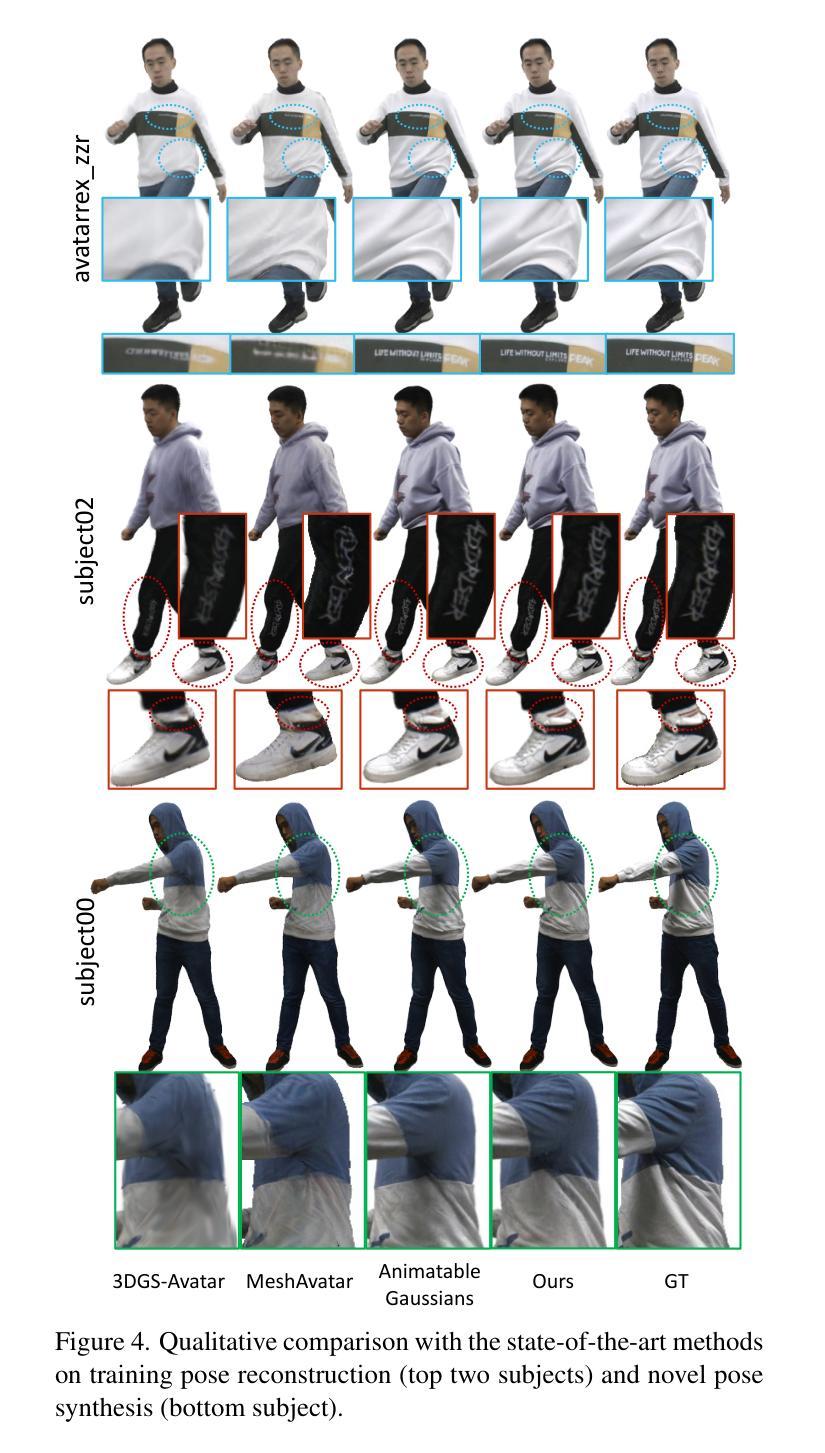
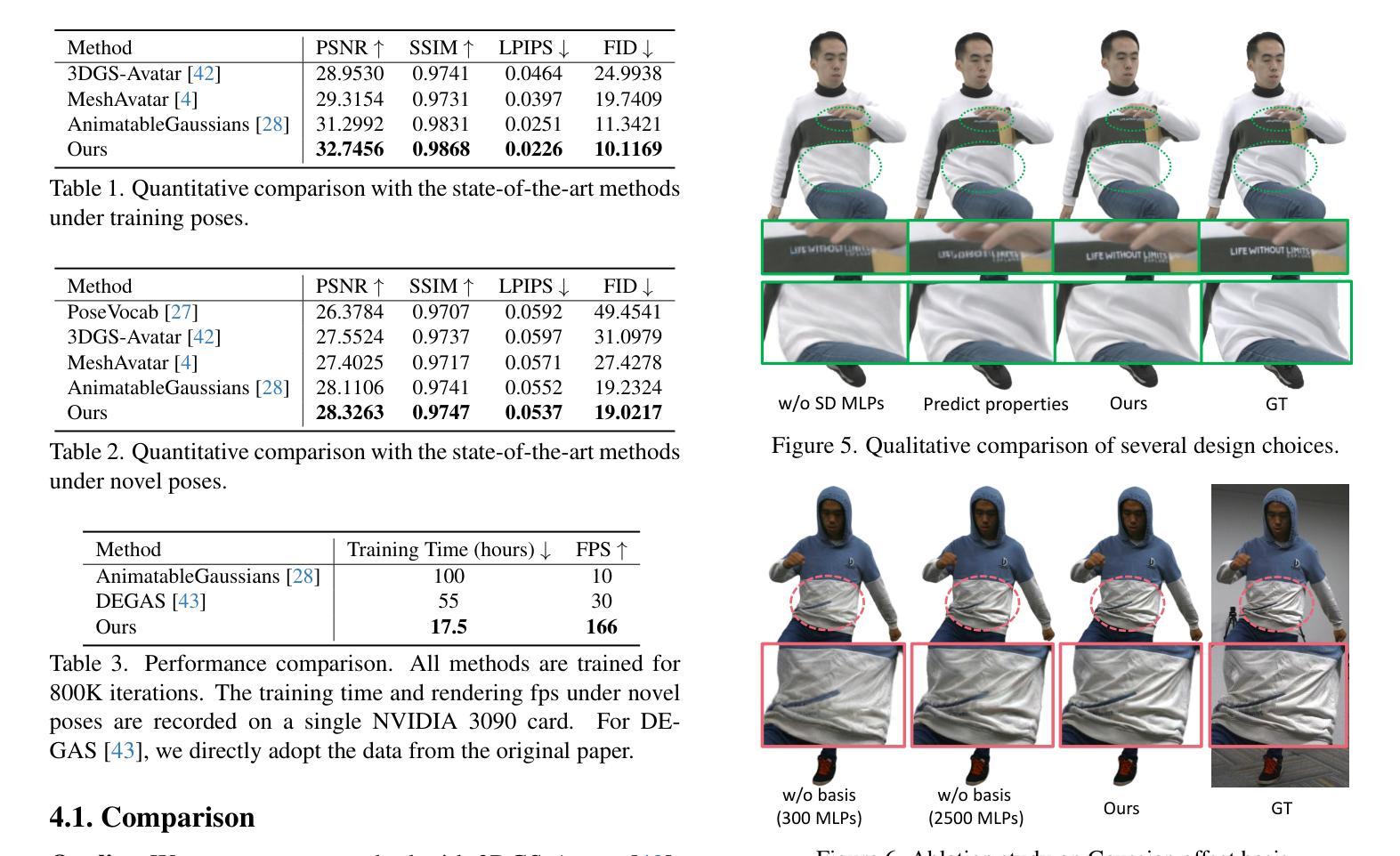