⚠️ 以下所有内容总结都来自于 大语言模型的能力,如有错误,仅供参考,谨慎使用
🔴 请注意:千万不要用于严肃的学术场景,只能用于论文阅读前的初筛!
💗 如果您觉得我们的项目对您有帮助 ChatPaperFree ,还请您给我们一些鼓励!⭐️ HuggingFace免费体验
2025-04-19 更新
High-Fidelity Image Inpainting with Multimodal Guided GAN Inversion
Authors:Libo Zhang, Yongsheng Yu, Jiali Yao, Heng Fan
Generative Adversarial Network (GAN) inversion have demonstrated excellent performance in image inpainting that aims to restore lost or damaged image texture using its unmasked content. Previous GAN inversion-based methods usually utilize well-trained GAN models as effective priors to generate the realistic regions for missing holes. Despite excellence, they ignore a hard constraint that the unmasked regions in the input and the output should be the same, resulting in a gap between GAN inversion and image inpainting and thus degrading the performance. Besides, existing GAN inversion approaches often consider a single modality of the input image, neglecting other auxiliary cues in images for improvements. Addressing these problems, we propose a novel GAN inversion approach, dubbed MMInvertFill, for image inpainting. MMInvertFill contains primarily a multimodal guided encoder with a pre-modulation and a GAN generator with F&W+ latent space. Specifically, the multimodal encoder aims to enhance the multi-scale structures with additional semantic segmentation edge texture modalities through a gated mask-aware attention module. Afterwards, a pre-modulation is presented to encode these structures into style vectors. To mitigate issues of conspicuous color discrepancy and semantic inconsistency, we introduce the F&W+ latent space to bridge the gap between GAN inversion and image inpainting. Furthermore, in order to reconstruct faithful and photorealistic images, we devise a simple yet effective Soft-update Mean Latent module to capture more diversified in-domain patterns for generating high-fidelity textures for massive corruptions. In our extensive experiments on six challenging datasets, we show that our MMInvertFill qualitatively and quantitatively outperforms other state-of-the-arts and it supports the completion of out-of-domain images effectively.
生成对抗网络(GAN)反转变现出在图像修复领域的优秀性能,旨在利用未遮挡的内容恢复丢失或损坏的图像纹理。之前的基于GAN反转的方法通常利用训练良好的GAN模型作为有效的先验来生成缺失区域的真实部分。尽管表现优秀,但它们忽略了一个硬性约束,即输入和输出的未遮挡区域应该相同,这导致GAN反转和图像修复之间存在差距,从而降低了性能。此外,现有的GAN反转方法往往只考虑输入图像的单模态,忽略了图像中其他辅助线索的改进。为了解决这些问题,我们提出了一种新型的GAN反转方法,名为MMInvertFill,用于图像修复。MMInvertFill主要包括一个带预调制的多模态引导编码器和带有F&W+潜在空间的GAN生成器。具体来说,多模态编码器旨在通过门控掩膜感知注意力模块增强多尺度结构,并附带额外的语义分割边缘纹理模态。之后,采用预调制将这些结构编码为风格向量。为了解决明显的颜色差异和语义不一致问题,我们引入了F&W+潜在空间来缩小GAN反转和图像修复之间的差距。此外,为了重建真实和逼真的图像,我们设计了一个简单有效的Soft-update Mean Latent模块,以捕获更多样化的域内模式,为大规模损坏生成高保真纹理。我们在六个具有挑战性的数据集上进行的广泛实验表明,我们的MMInvertFill在定性和定量上均优于其他最新技术,并且它能够有效支持跨域图像的完成。
论文及项目相关链接
PDF Accepted to IJCV. arXiv admin note: text overlap with arXiv:2208.11850
Summary
GAN反轉网络(GAN inversion)在图像修复(image inpainting)中表现出卓越性能,旨在利用未遮挡的内容恢复丢失或损坏的图像纹理。但现有方法忽略了一个硬约束,即输入和输出的未遮挡区域应该相同。本文提出了一种新型的GAN反轉方法MMInvertFill,用于图像修复。MMInvertFill主要包括多模态引导编码器、预调制、GAN生成器和F&W+潜在空间。其中,多模态编码器旨在通过门控掩膜注意模块增强多尺度结构与额外的语义分割边缘纹理模态;预调制用于将这些结构编码为风格向量;F&W+潜在空间旨在缩小GAN反轉和图像修复之间的差距,并缓解颜色和语义不一致的问题;为了重建忠实和逼真的图像,我们开发了一种简单有效的Soft-update Mean Latent模块,用于捕捉更多元化的域内模式,为大规模损坏生成高保真纹理。在六个具有挑战性的数据集上的实验表明,MMInvertFill在定性和定量上均优于其他最新技术,并有效地支持跨域图像的完成。
Key Takeaways
- GAN反轉网络在图像修复中有良好的性能表现。
- 现有GAN反轉方法忽略了一个硬约束:输入和输出的未遮挡区域应相同。
- MMInvertFill包含多模态引导编码器、预调制、GAN生成器和F&W+潜在空间等核心组件。
- 多模态编码器利用门控掩膜注意模块增强图像的多尺度结构和纹理信息。
- 预调制将结构编码为风格向量。
- F&W+潜在空间解决了颜色和语义不一致的问题,缩小了GAN反轉和图像修复之间的差距。
- Soft-update Mean Latent模块用于捕捉更多元化的域内模式,生成高保真纹理,适用于大规模图像修复。
点此查看论文截图
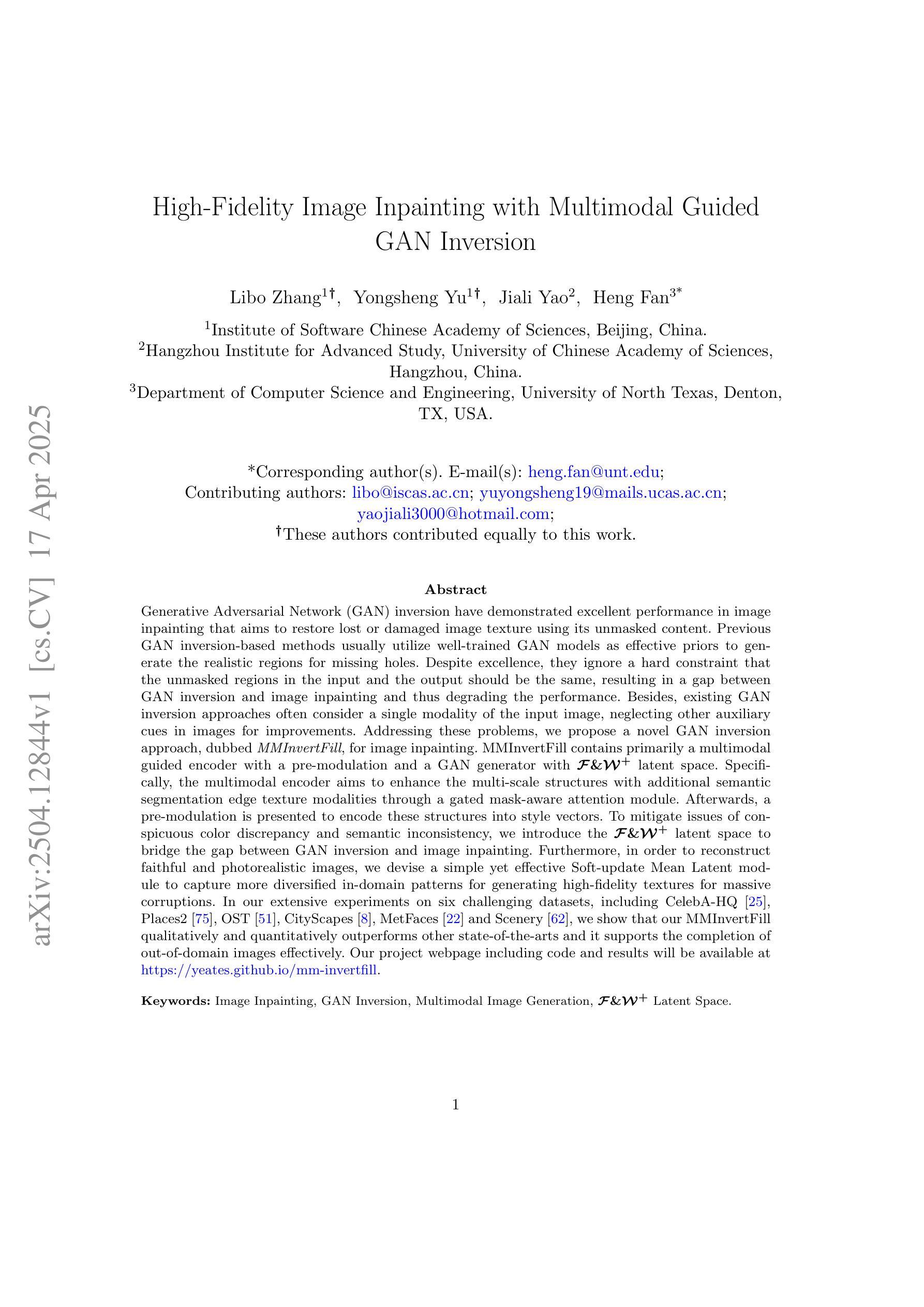
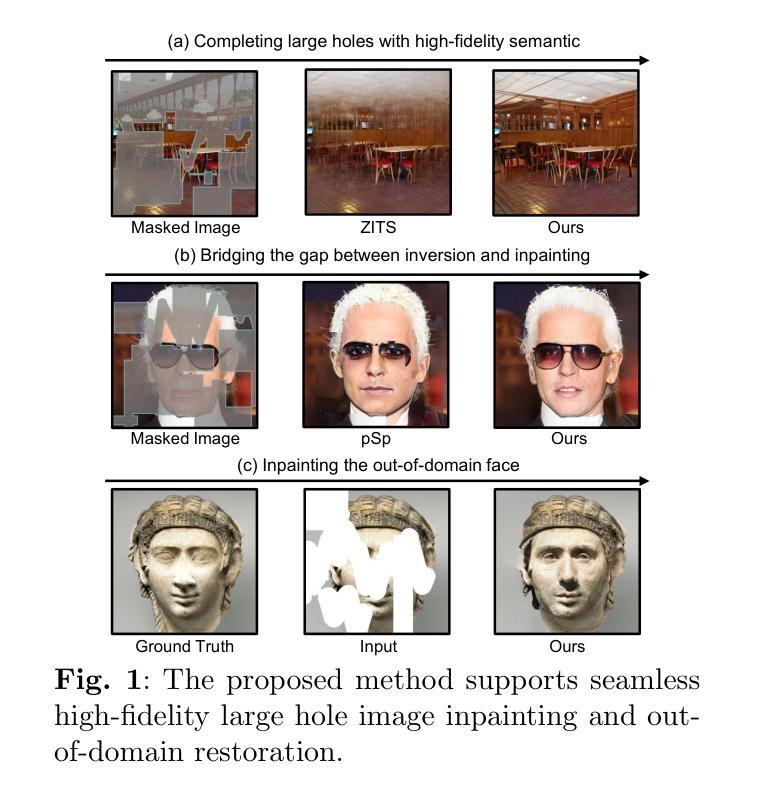
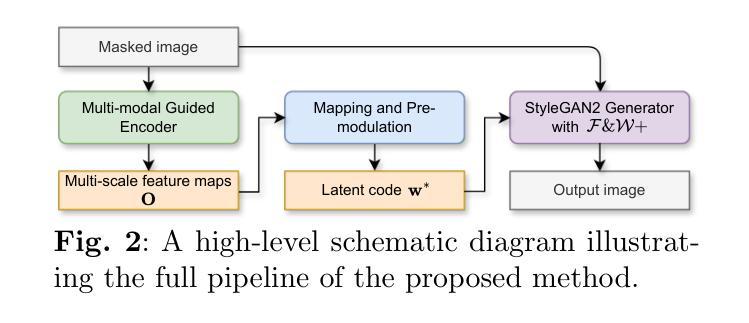
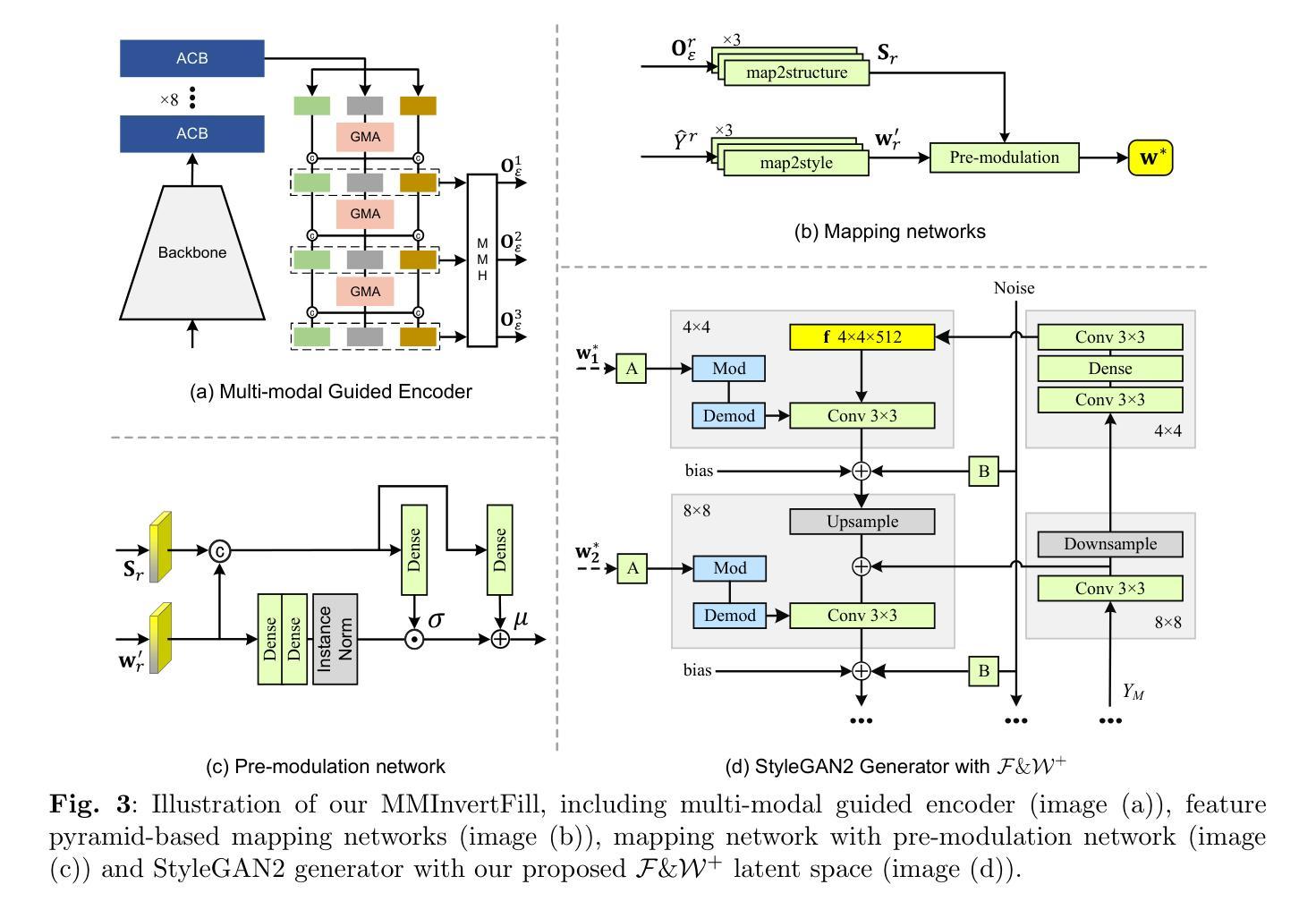