⚠️ 以下所有内容总结都来自于 大语言模型的能力,如有错误,仅供参考,谨慎使用
🔴 请注意:千万不要用于严肃的学术场景,只能用于论文阅读前的初筛!
💗 如果您觉得我们的项目对您有帮助 ChatPaperFree ,还请您给我们一些鼓励!⭐️ HuggingFace免费体验
2025-04-22 更新
Collective Learning Mechanism based Optimal Transport Generative Adversarial Network for Non-parallel Voice Conversion
Authors:Sandipan Dhar, Md. Tousin Akhter, Nanda Dulal Jana, Swagatam Das
After demonstrating significant success in image synthesis, Generative Adversarial Network (GAN) models have likewise made significant progress in the field of speech synthesis, leveraging their capacity to adapt the precise distribution of target data through adversarial learning processes. Notably, in the realm of State-Of-The-Art (SOTA) GAN-based Voice Conversion (VC) models, there exists a substantial disparity in naturalness between real and GAN-generated speech samples. Furthermore, while many GAN models currently operate on a single generator discriminator learning approach, optimizing target data distribution is more effectively achievable through a single generator multi-discriminator learning scheme. Hence, this study introduces a novel GAN model named Collective Learning Mechanism-based Optimal Transport GAN (CLOT-GAN) model, incorporating multiple discriminators, including the Deep Convolutional Neural Network (DCNN) model, Vision Transformer (ViT), and conformer. The objective of integrating various discriminators lies in their ability to comprehend the formant distribution of mel-spectrograms, facilitated by a collective learning mechanism. Simultaneously, the inclusion of Optimal Transport (OT) loss aims to precisely bridge the gap between the source and target data distribution, employing the principles of OT theory. The experimental validation on VCC 2018, VCTK, and CMU-Arctic datasets confirms that the CLOT-GAN-VC model outperforms existing VC models in objective and subjective assessments.
在图像合成领域取得显著成功后,生成对抗网络(GAN)模型在语音合成领域也取得了重大进展,它们利用对抗学习过程来适应目标数据的精确分布。特别是在最先进的(SOTA)基于GAN的语音转换(VC)模型中,真实和GAN生成的语音样本之间的自然度存在很大差异。此外,虽然许多GAN模型目前采用单一生成器鉴别器学习方法,但通过单一生成器多鉴别器学习方案可以更有效地优化目标数据分布。因此,本研究引入了一种新型的GAN模型,名为基于集体学习机制的优化传输GAN(CLOT-GAN)模型,该模型结合了多个鉴别器,包括深度卷积神经网络(DCNN)模型、视觉变压器(ViT)和conformer。集成多种鉴别器的目的在于它们能够借助集体学习机制理解梅尔频谱的共振峰分布。同时,引入最优传输(OT)损失旨在精确弥合源数据分布与目标数据分布之间的差距,采用OT理论的原则。在VCC 2018、VCTK和CMU-Arctic数据集上的实验验证表明,CLOT-GAN-VC模型在客观和主观评估中优于现有VC模型。
论文及项目相关链接
PDF 7 pages, 2 figures, 3 tables
Summary
GAN模型在语音合成领域取得显著进展,通过对抗学习过程适应目标数据的精确分布。最新研究引入了一种名为CLOT-GAN的新型GAN模型,采用集体学习机制,结合多种鉴别器,包括深度卷积神经网络(DCNN)、视觉变压器(ViT)和conformer。CLOT-GAN旨在理解梅尔频谱图的音位分布,并借助最优传输(OT)损失精确弥合源数据与目标数据分布之间的差距。实验验证表明,CLOT-GAN-VC模型在客观和主观评估上均优于现有VC模型。
Key Takeaways
- GAN模型已成功应用于语音合成领域,通过对抗学习过程适应目标数据的分布。
- 现有GAN模型在真实和生成语音样本的自然度上存在显著差异。
- CLOT-GAN模型采用多种鉴别器,包括DCNN、ViT和conformer,以理解梅尔频谱图的音位分布。
- CLOT-GAN模型借助集体学习机制,提高了对目标数据分布的优化能力。
- CLOT-GAN模型引入最优传输(OT)损失,精确弥合源数据与目标数据分布之间的差距。
- 实验验证显示,CLOT-GAN-VC模型在客观和主观评估方面优于其他VC模型。
点此查看论文截图
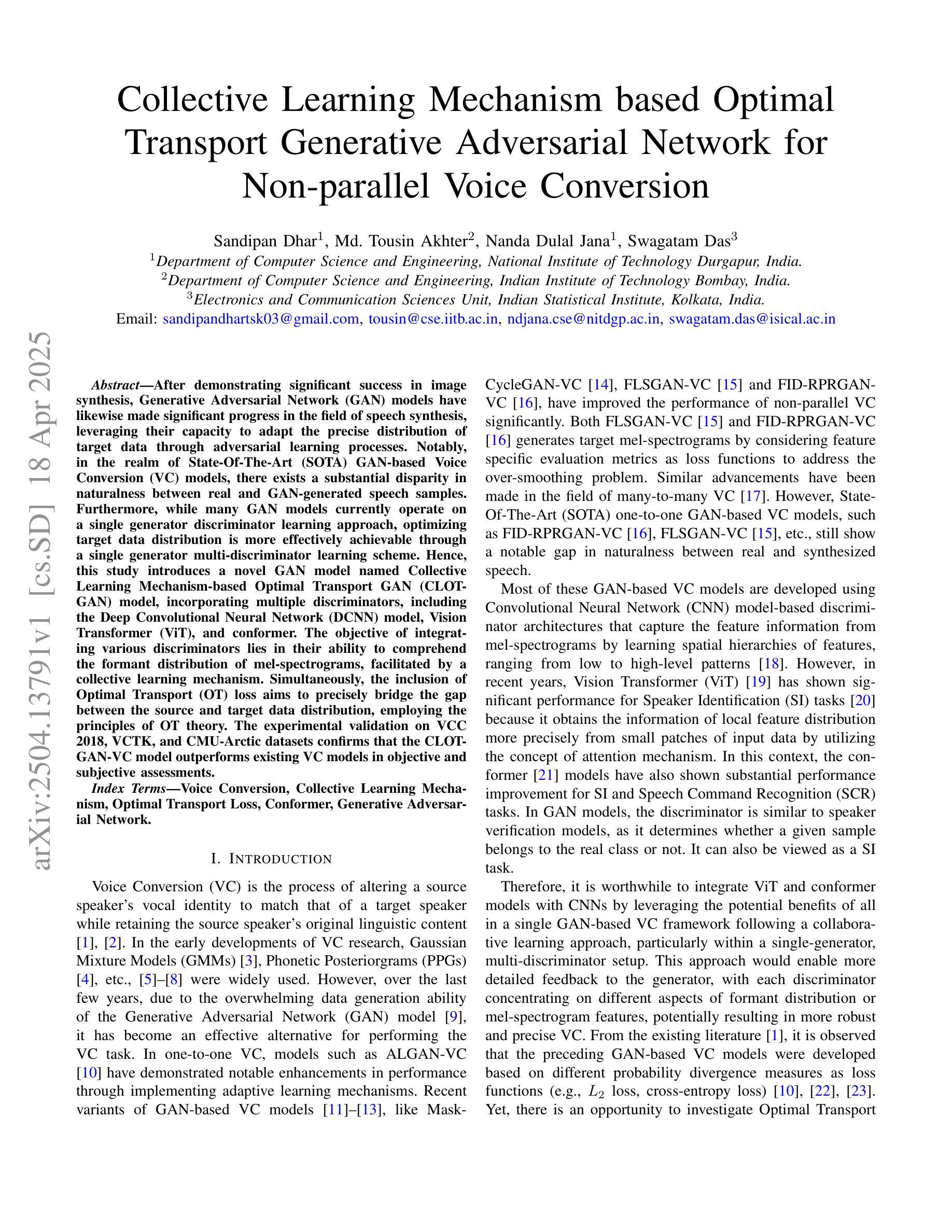
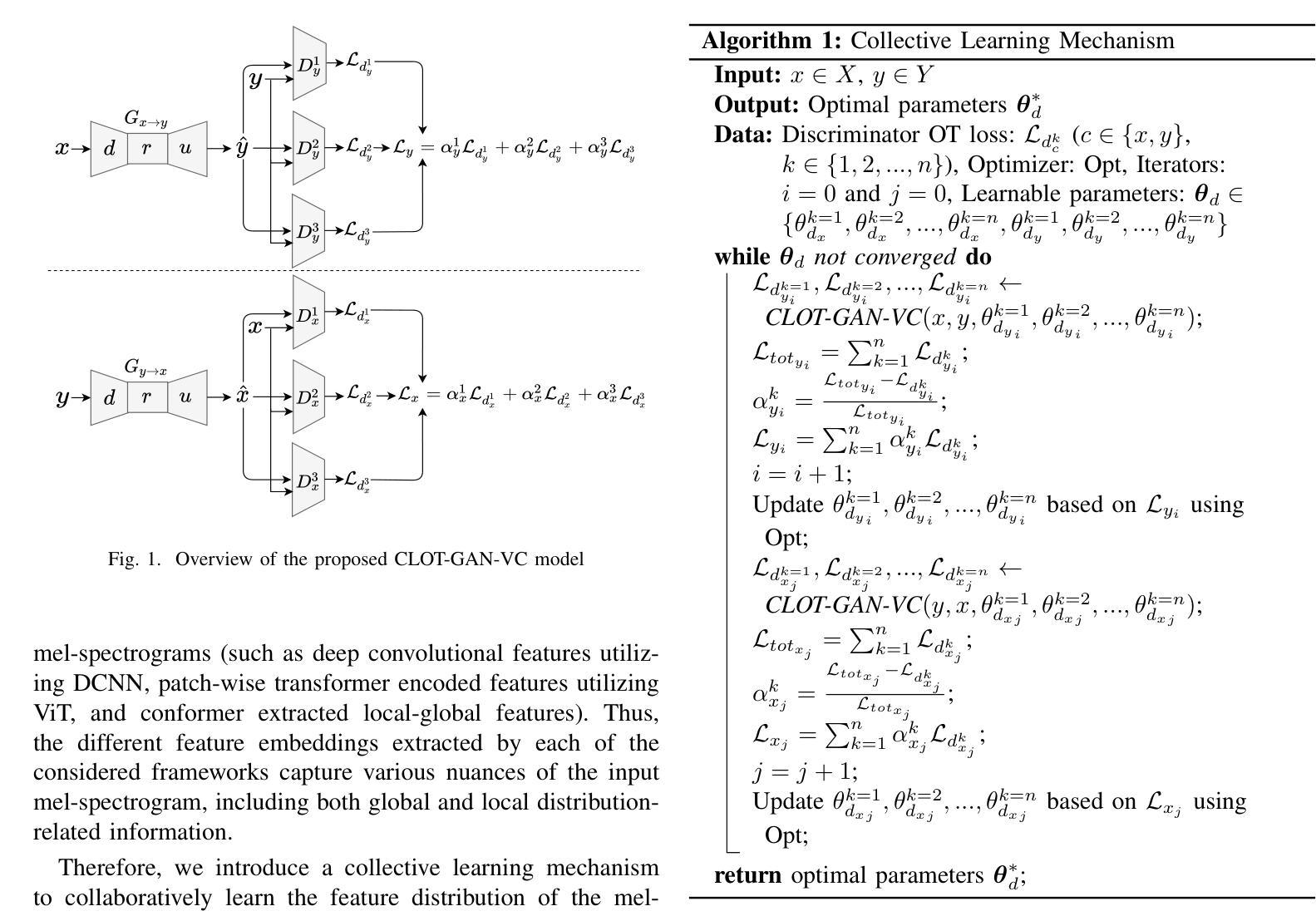
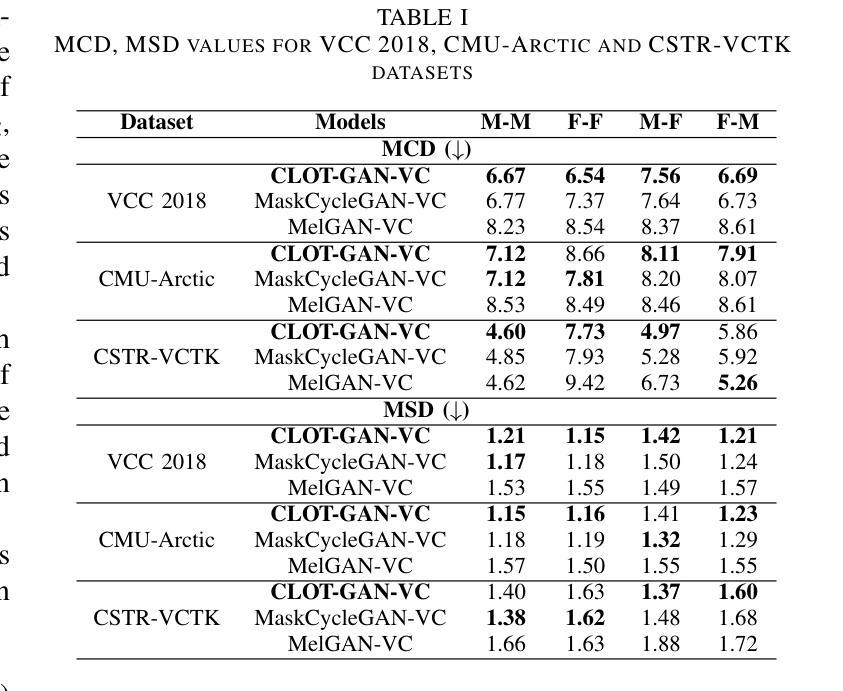
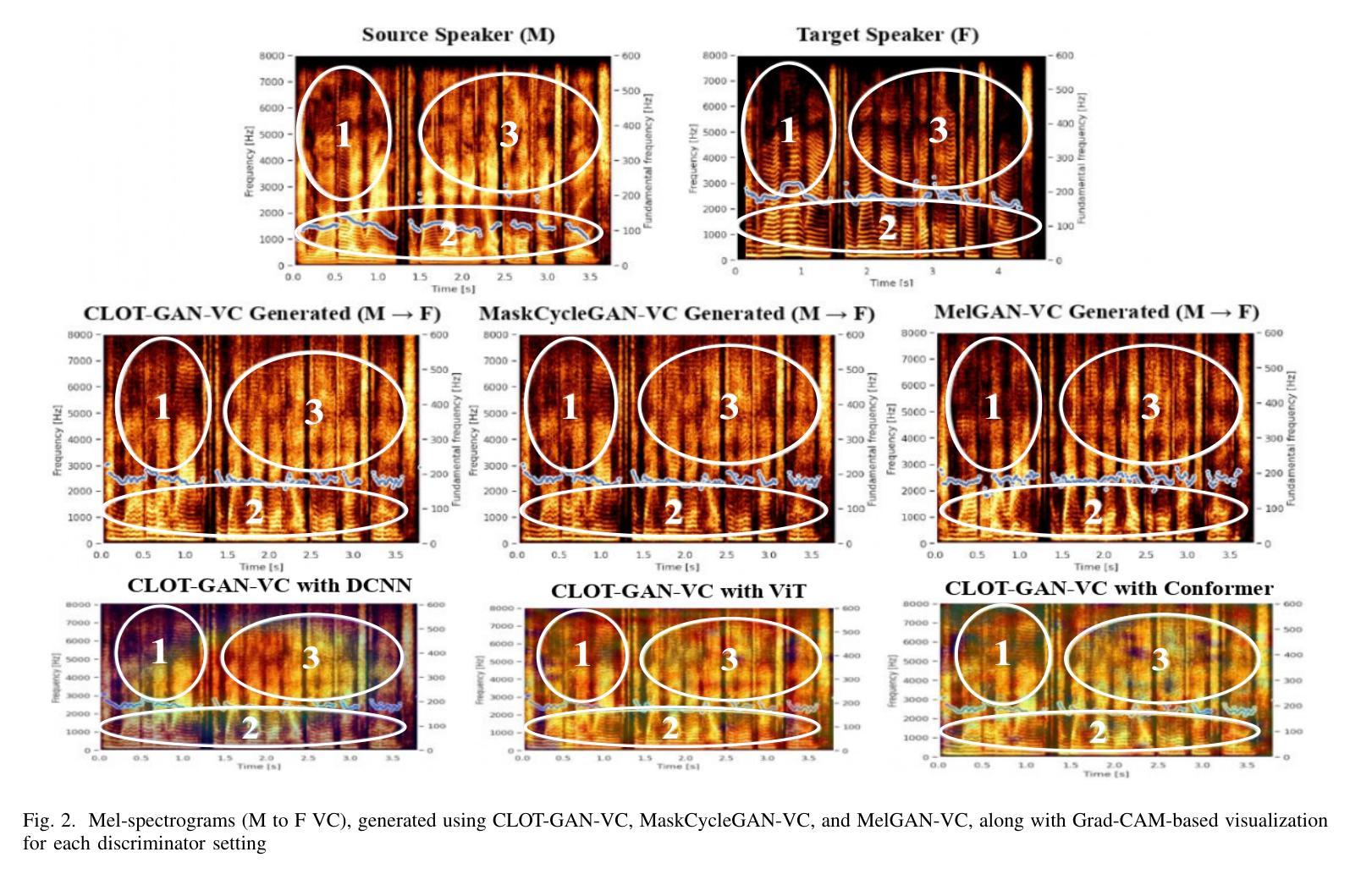
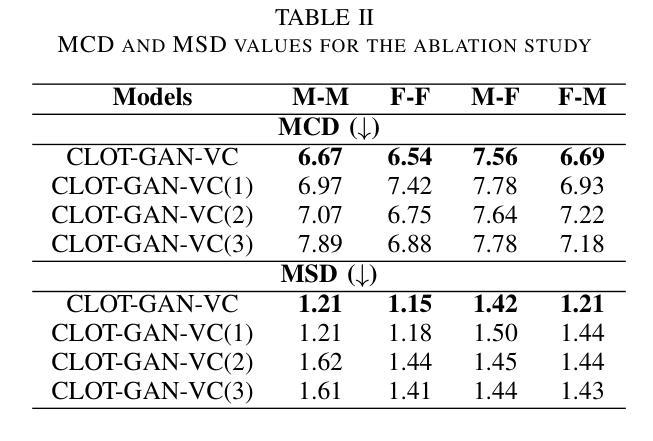
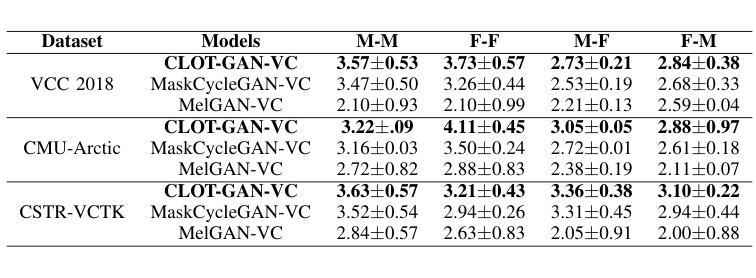
SupResDiffGAN a new approach for the Super-Resolution task
Authors:Dawid Kopeć, Wojciech Kozłowski, Maciej Wizerkaniuk, Dawid Krutul, Jan Kocoń, Maciej Zięba
In this work, we present SupResDiffGAN, a novel hybrid architecture that combines the strengths of Generative Adversarial Networks (GANs) and diffusion models for super-resolution tasks. By leveraging latent space representations and reducing the number of diffusion steps, SupResDiffGAN achieves significantly faster inference times than other diffusion-based super-resolution models while maintaining competitive perceptual quality. To prevent discriminator overfitting, we propose adaptive noise corruption, ensuring a stable balance between the generator and the discriminator during training. Extensive experiments on benchmark datasets show that our approach outperforms traditional diffusion models such as SR3 and I$^2$SB in efficiency and image quality. This work bridges the performance gap between diffusion- and GAN-based methods, laying the foundation for real-time applications of diffusion models in high-resolution image generation.
在这项工作中,我们提出了SupResDiffGAN,这是一种新型混合架构,结合了生成对抗网络(GANs)和扩散模型在超分辨率任务上的优势。通过利用潜在空间表示和减少扩散步骤的数量,SupResDiffGAN实现了比其他基于扩散的超分辨率模型更快的推理时间,同时保持了有竞争力的感知质量。为了防止判别器过拟合,我们提出了自适应噪声腐蚀,确保在训练过程中生成器和判别器之间的稳定平衡。在基准数据集上的大量实验表明,我们的方法在效率和图像质量方面优于传统的扩散模型,如SR3和I$^2$SB。这项工作缩小了扩散和基于GAN的方法之间的性能差距,为扩散模型在实时高分辨率图像生成中的应用奠定了基础。
论文及项目相关链接
PDF 25th International Conference on Computational Science
Summary
本文提出了SupResDiffGAN,这是一种结合生成对抗网络(GANs)和扩散模型优势的新型混合架构,用于超分辨率任务。通过利用潜在空间表示和减少扩散步骤数量,SupResDiffGAN在保持与其他基于扩散的超分辨率模型竞争性的感知质量的同时,实现了更快的推理时间。为防止判别器过拟合,我们提出了自适应噪声腐蚀方法,确保生成器和判别器在训练过程中的稳定平衡。在基准数据集上的广泛实验表明,我们的方法在效率和图像质量方面优于传统扩散模型,如SR3和I$^2$SB。这项工作缩小了扩散和GAN方法之间的性能差距,为扩散模型在高清图像生成中的实时应用奠定了基础。
Key Takeaways
- SupResDiffGAN是一个新型混合架构,结合了GANs和扩散模型的优点,用于超分辨率任务。
- 通过利用潜在空间表示和减少扩散步骤,实现了更快的推理时间。
- 为防止判别器过拟合,提出了自适应噪声腐蚀方法。
- 在基准数据集上,SupResDiffGAN在效率和图像质量方面超越了传统扩散模型。
- 该工作缩小了扩散模型和GAN之间的性能差距。
- SupResDiffGAN为扩散模型在高清图像生成的实时应用提供了可能。
点此查看论文截图
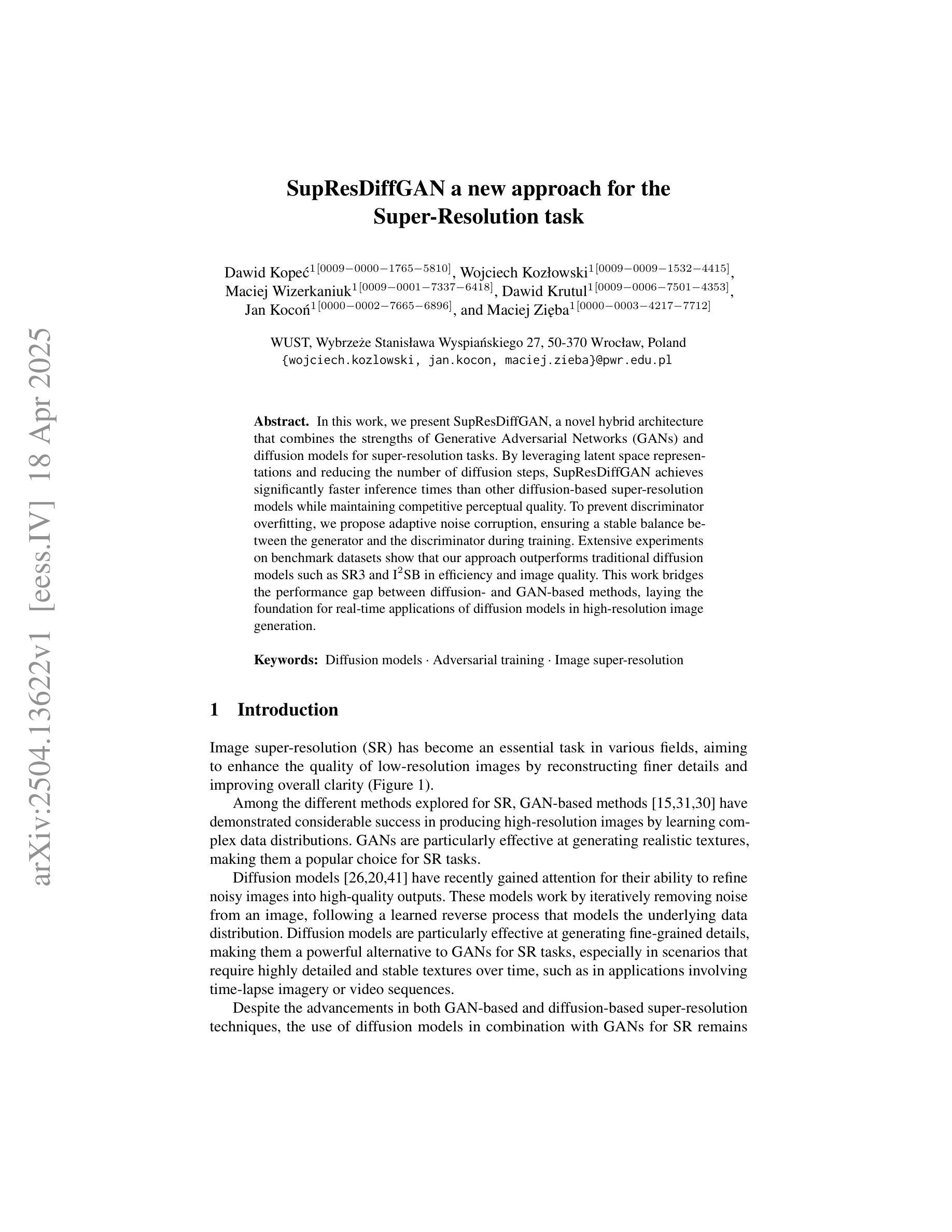
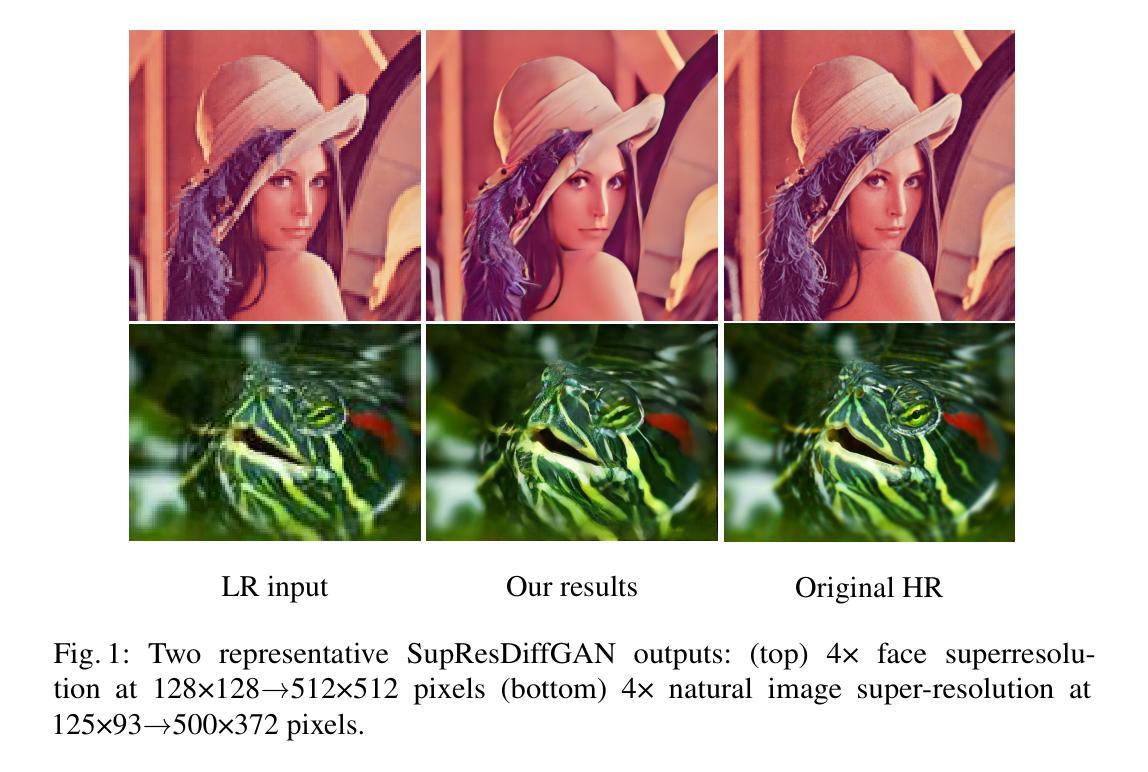
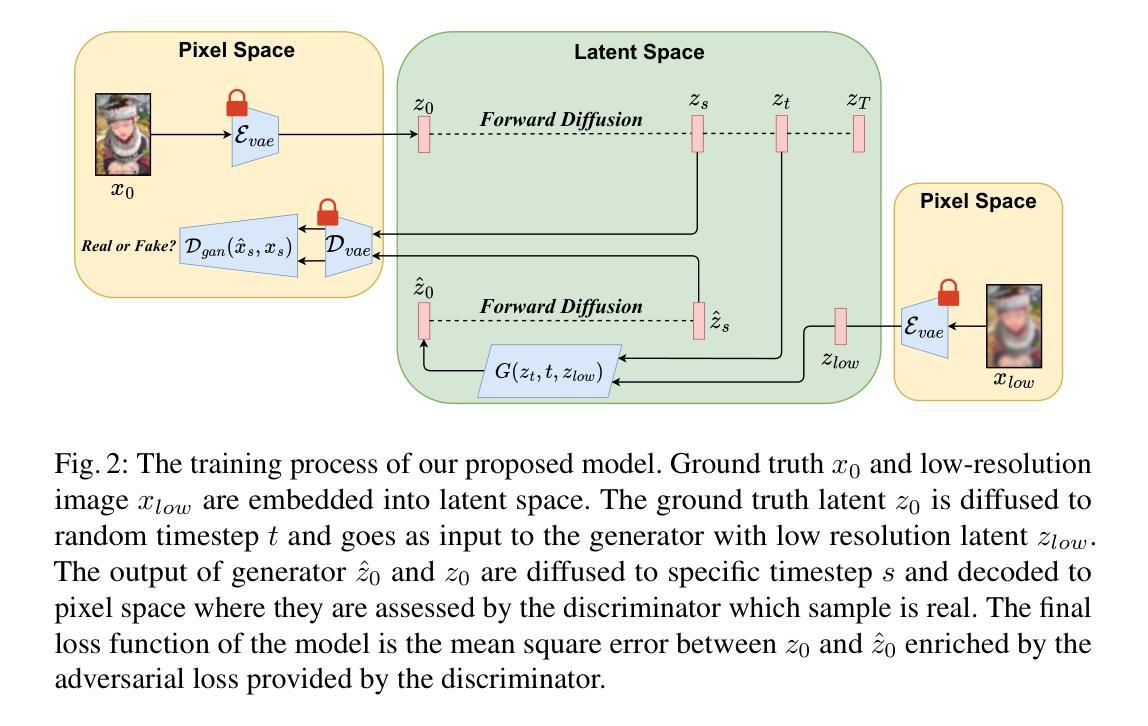
SMPL-GPTexture: Dual-View 3D Human Texture Estimation using Text-to-Image Generation Models
Authors:Mingxiao Tu, Shuchang Ye, Hoijoon Jung, Jinman Kim
Generating high-quality, photorealistic textures for 3D human avatars remains a fundamental yet challenging task in computer vision and multimedia field. However, real paired front and back images of human subjects are rarely available with privacy, ethical and cost of acquisition, which restricts scalability of the data. Additionally, learning priors from image inputs using deep generative models, such as GANs or diffusion models, to infer unseen regions such as the human back often leads to artifacts, structural inconsistencies, or loss of fine-grained detail. To address these issues, we present SMPL-GPTexture (skinned multi-person linear model - general purpose Texture), a novel pipeline that takes natural language prompts as input and leverages a state-of-the-art text-to-image generation model to produce paired high-resolution front and back images of a human subject as the starting point for texture estimation. Using the generated paired dual-view images, we first employ a human mesh recovery model to obtain a robust 2D-to-3D SMPL alignment between image pixels and the 3D model’s UV coordinates for each views. Second, we use an inverted rasterization technique that explicitly projects the observed colour from the input images into the UV space, thereby producing accurate, complete texture maps. Finally, we apply a diffusion-based inpainting module to fill in the missing regions, and the fusion mechanism then combines these results into a unified full texture map. Extensive experiments shows that our SMPL-GPTexture can generate high resolution texture aligned with user’s prompts.
在计算机视觉和多媒体领域,为3D人类角色生成高质量、逼真的纹理仍然是一项基本且具有挑战性的任务。然而,由于隐私、道德和采集成本的问题,人类主题的真实配对正面和背面图像很少可用,这限制了数据的可扩展性。此外,使用深度生成模型(如GAN或扩散模型)从图像输入中学习先验知识,以推断未见区域(如人体背面),通常会导致伪影、结构不一致或丢失细节。为了解决这些问题,我们提出了SMPL-GPTexture(皮肤多人线性模型-通用纹理),这是一种新型流程,以自然语言提示为输入,并利用最先进的文本到图像生成模型来生成人类主题的配对高分辨率正面和背面图像,作为纹理估计的起点。使用生成的配对双视图像,我们首先采用人体网格恢复模型来获得图像像素和每个视图的3D模型的UV坐标之间的稳健的2D到3D SMPL对齐。其次,我们使用反向光栅化技术显式地将观察到的颜色从输入图像投影到UV空间中,从而生成准确、完整的纹理映射。最后,我们应用基于扩散的填充模块来填充缺失区域,融合机制然后将这些结果组合成统一的完整纹理映射图。大量实验表明,我们的SMPL-GPTexture可以生成与用户提示对齐的高分辨率纹理。
论文及项目相关链接
Summary
本文介绍了一种名为SMPL-GPTexture的新方法,用于生成高质量、逼真的三维人类角色纹理。该方法采用自然语言提示作为输入,并利用先进的文本到图像生成模型产生配对的高分辨率前视和背视图像作为纹理估计的起点。通过使用生成的双视点图像,结合人类网格恢复模型和逆向栅格化技术,将观察到的颜色从图像投影到UV空间,生成准确完整的纹理映射。最后,使用基于扩散的填充模块填充缺失区域,并通过融合机制将这些结果合并成统一的完整纹理图。此方法可生成与用户提示对齐的高分辨率纹理。
Key Takeaways
- SMPL-GPTexture方法解决了生成高质量三维人类角色纹理的挑战性问题。
- 利用自然语言提示作为输入,使用先进的文本到图像生成模型产生配对的高分辨率前视和背视图像。
- 通过人类网格恢复模型实现2D到3D SMPL的对齐。
- 使用逆向栅格化技术将观察到的颜色从图像投影到UV空间,生成纹理映射。
- 扩散填充模块用于填充缺失区域。
- 融合机制将不同结果合并成统一的完整纹理图。
点此查看论文截图
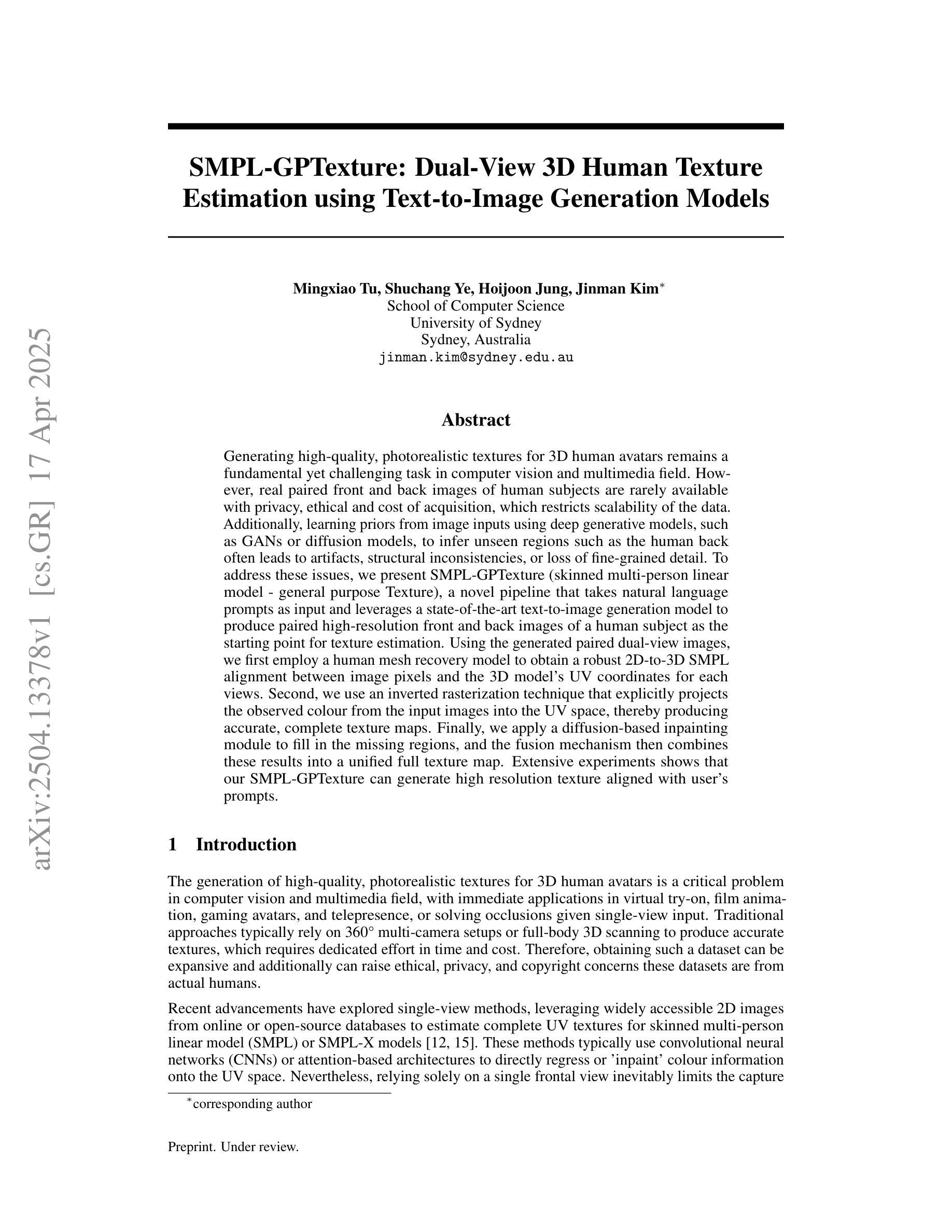
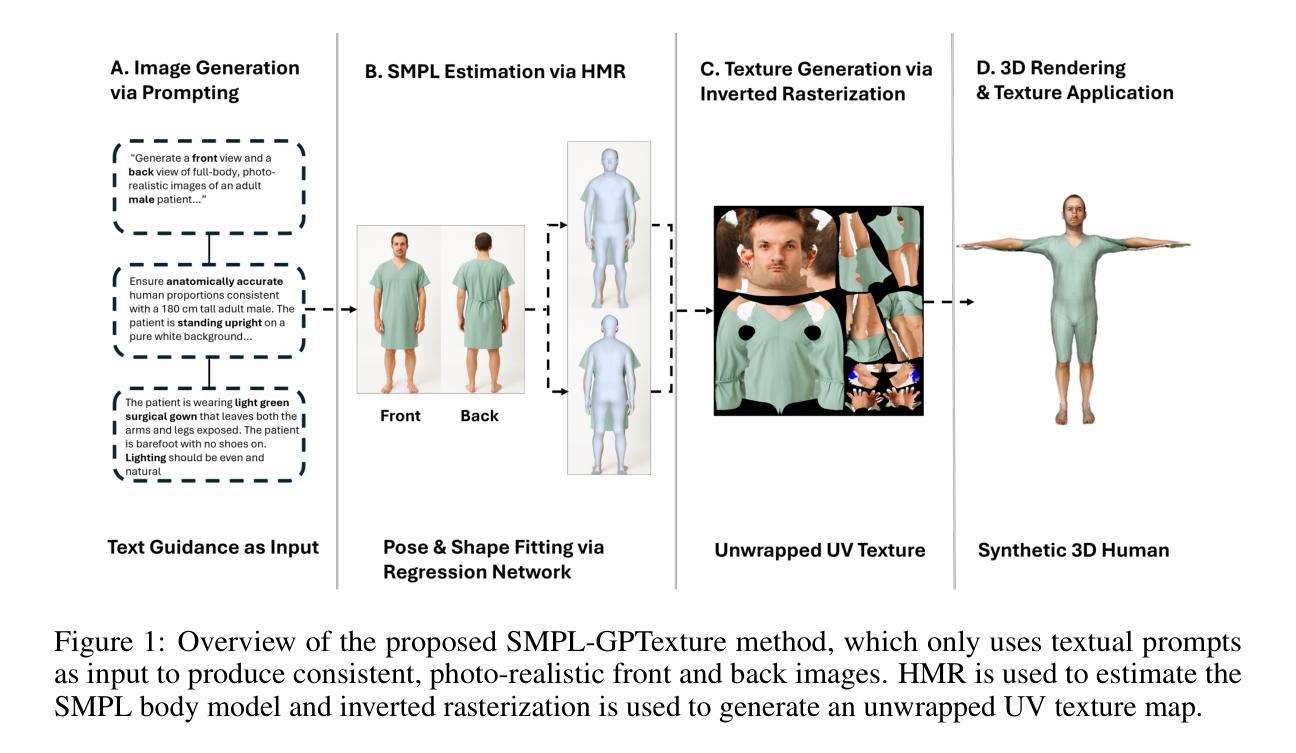
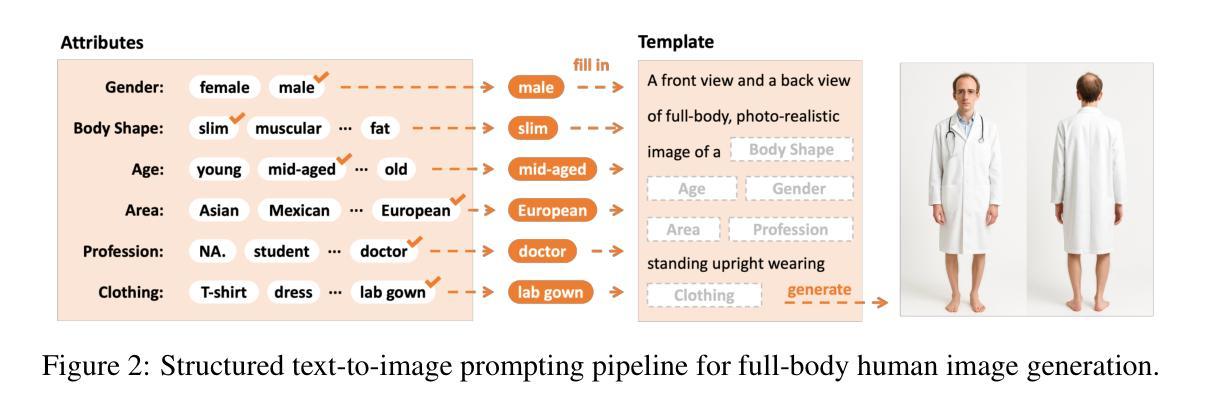
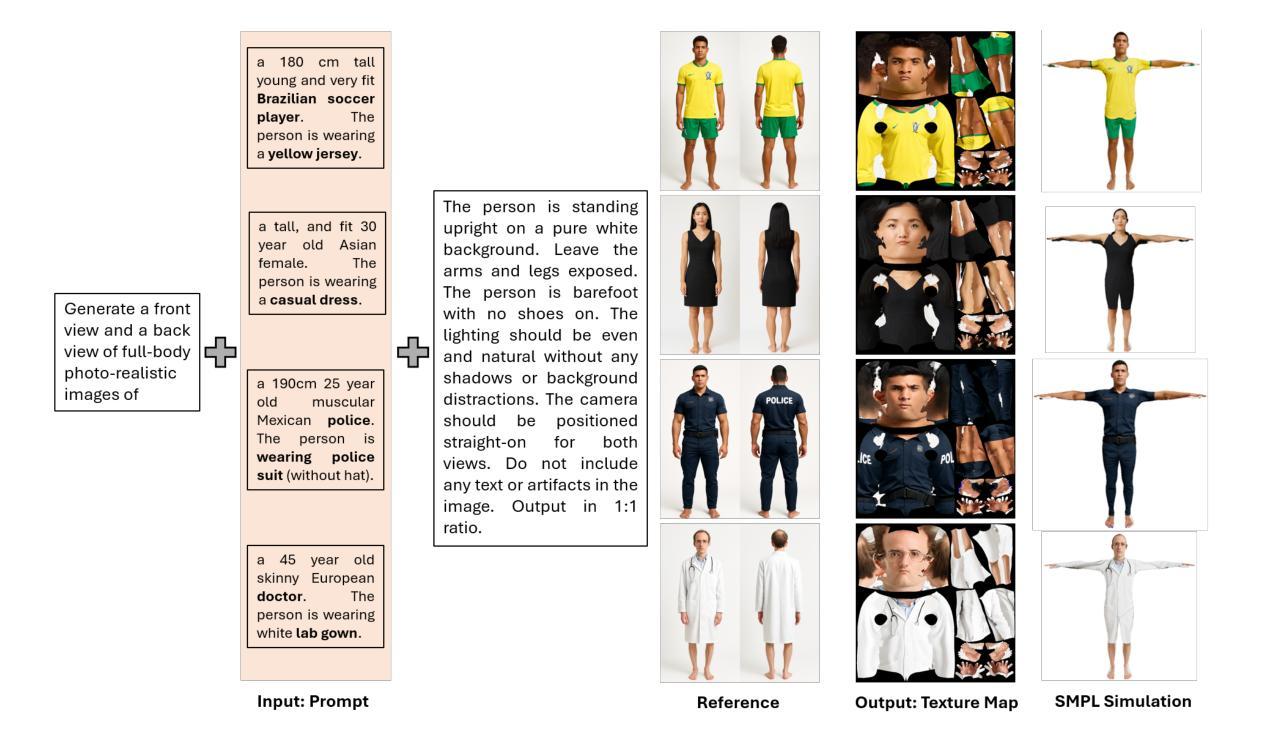
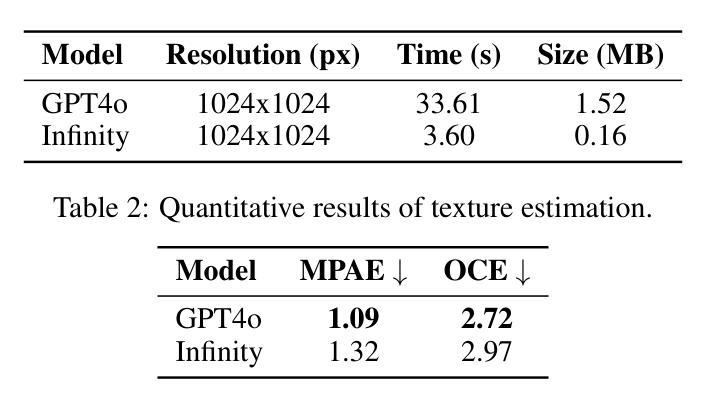