⚠️ 以下所有内容总结都来自于 大语言模型的能力,如有错误,仅供参考,谨慎使用
🔴 请注意:千万不要用于严肃的学术场景,只能用于论文阅读前的初筛!
💗 如果您觉得我们的项目对您有帮助 ChatPaperFree ,还请您给我们一些鼓励!⭐️ HuggingFace免费体验
2025-04-22 更新
BEV-GS: Feed-forward Gaussian Splatting in Bird’s-Eye-View for Road Reconstruction
Authors:Wenhua Wu, Tong Zhao, Chensheng Peng, Lei Yang, Yintao Wei, Zhe Liu, Hesheng Wang
Road surface is the sole contact medium for wheels or robot feet. Reconstructing road surface is crucial for unmanned vehicles and mobile robots. Recent studies on Neural Radiance Fields (NeRF) and Gaussian Splatting (GS) have achieved remarkable results in scene reconstruction. However, they typically rely on multi-view image inputs and require prolonged optimization times. In this paper, we propose BEV-GS, a real-time single-frame road surface reconstruction method based on feed-forward Gaussian splatting. BEV-GS consists of a prediction module and a rendering module. The prediction module introduces separate geometry and texture networks following Bird’s-Eye-View paradigm. Geometric and texture parameters are directly estimated from a single frame, avoiding per-scene optimization. In the rendering module, we utilize grid Gaussian for road surface representation and novel view synthesis, which better aligns with road surface characteristics. Our method achieves state-of-the-art performance on the real-world dataset RSRD. The road elevation error reduces to 1.73 cm, and the PSNR of novel view synthesis reaches 28.36 dB. The prediction and rendering FPS is 26, and 2061, respectively, enabling high-accuracy and real-time applications. The code will be available at: \href{https://github.com/cat-wwh/BEV-GS}{\texttt{https://github.com/cat-wwh/BEV-GS}}
路面是车轮或机器人脚部的唯一接触媒介。对于无人驾驶车辆和移动机器人来说,重建路面至关重要。最近关于神经辐射场(NeRF)和高斯平铺(GS)的研究在场景重建方面取得了显著成果。然而,它们通常依赖于多视角图像输入,并需要长时间的优化。在本文中,我们提出了基于前馈高斯平铺的实时单帧路面重建方法BEV-GS。BEV-GS由预测模块和渲染模块组成。预测模块采用鸟瞰视图范式的独立几何和纹理网络。几何和纹理参数直接从单帧中估计,避免了针对每个场景的优化。在渲染模块中,我们利用网格高斯进行路面表示和新视图合成,这更好地符合路面特性。我们的方法在真实世界数据集RSRD上实现了最先进的性能。路面高程误差降低到1.73厘米,新视图合成的峰值信噪比达到28.36分贝。预测和渲染的帧数分别为每秒26帧和2061帧,实现了高精度和实时应用。代码将在以下网址提供:https://github.com/cat-wwh/BEV-GS。
论文及项目相关链接
Summary
本文提出了一种基于前馈高斯拼贴技术的实时单帧路面重建方法BEV-GS。该方法包括预测模块和渲染模块,预测模块采用鸟瞰视角范式,从单帧图像直接估计几何和纹理参数,避免了逐场景的优化。渲染模块使用网格高斯进行路面表示和新颖视图合成,更好地符合路面特性。该方法在真实世界数据集RSRD上实现了卓越性能,路面高程误差降低到1.73厘米,新颖视图合成的峰值信噪比达到28.36分贝,预测和渲染帧率分别为每秒26帧和每秒2061帧,可实现高准确性和实时应用。
Key Takeaways
- 提出了基于前馈高斯拼贴技术(BEV-GS)的实时单帧路面重建方法。
- 方法包括预测模块和渲染模块,预测模块从单帧图像直接估计几何和纹理参数。
- 鸟瞰视角范式被用于预测模块中,避免了逐场景的优化。
- 渲染模块使用网格高斯进行路面表示和新颖视图合成。
- 该方法在真实世界数据集RSRD上实现了卓越性能,路面高程误差降低到较低水平。
- 预测和渲染帧率较高,可实现实时应用。
点此查看论文截图
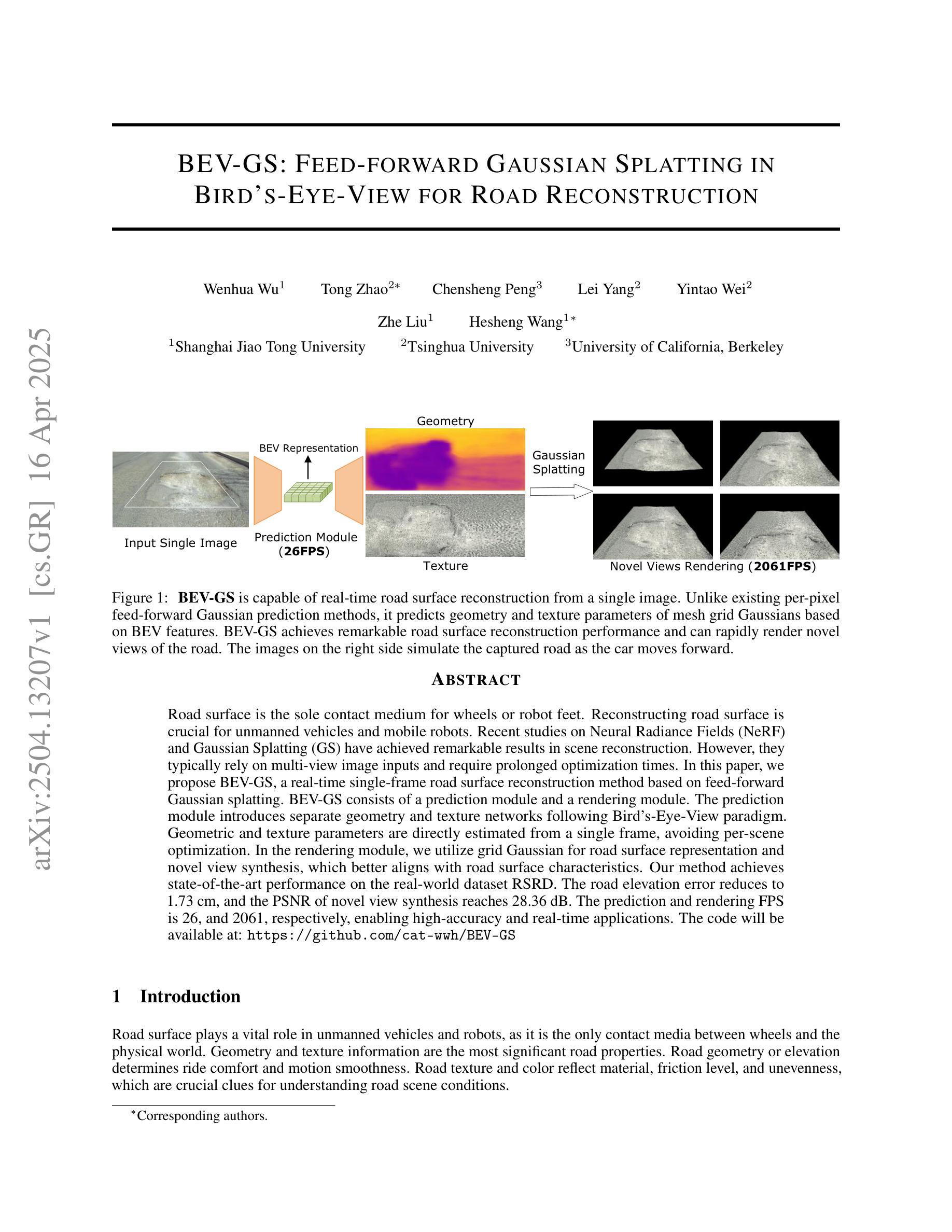
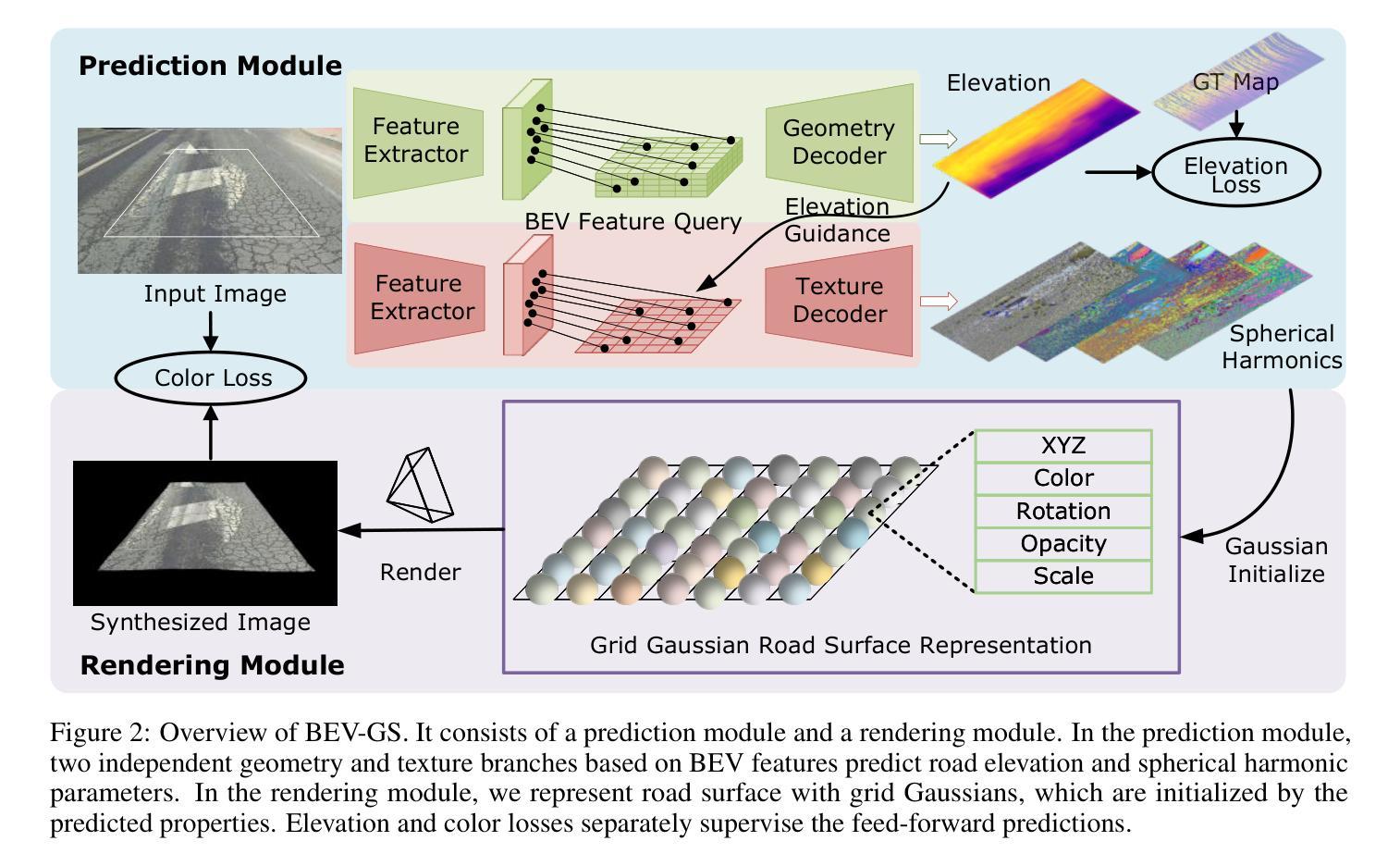
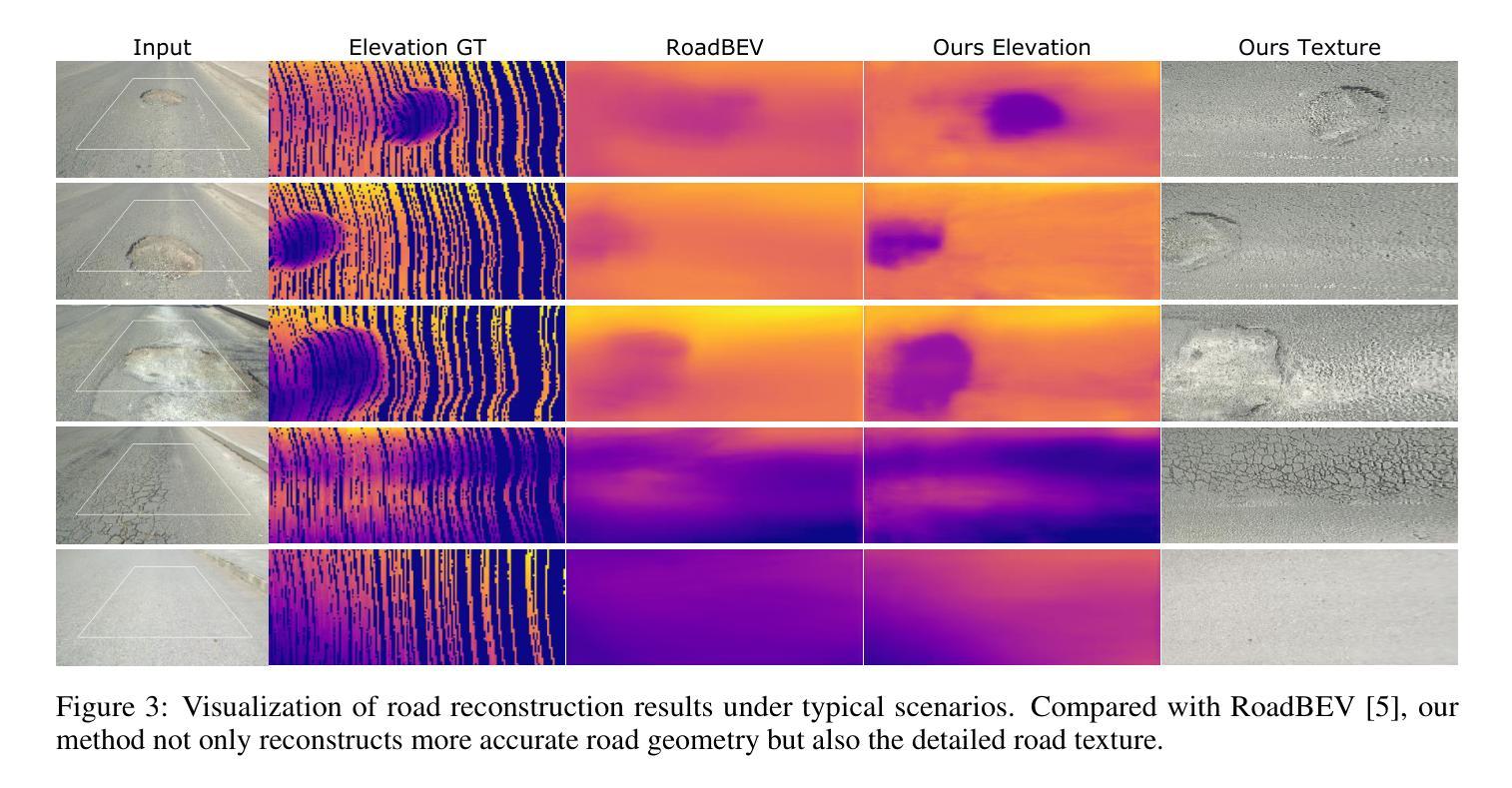
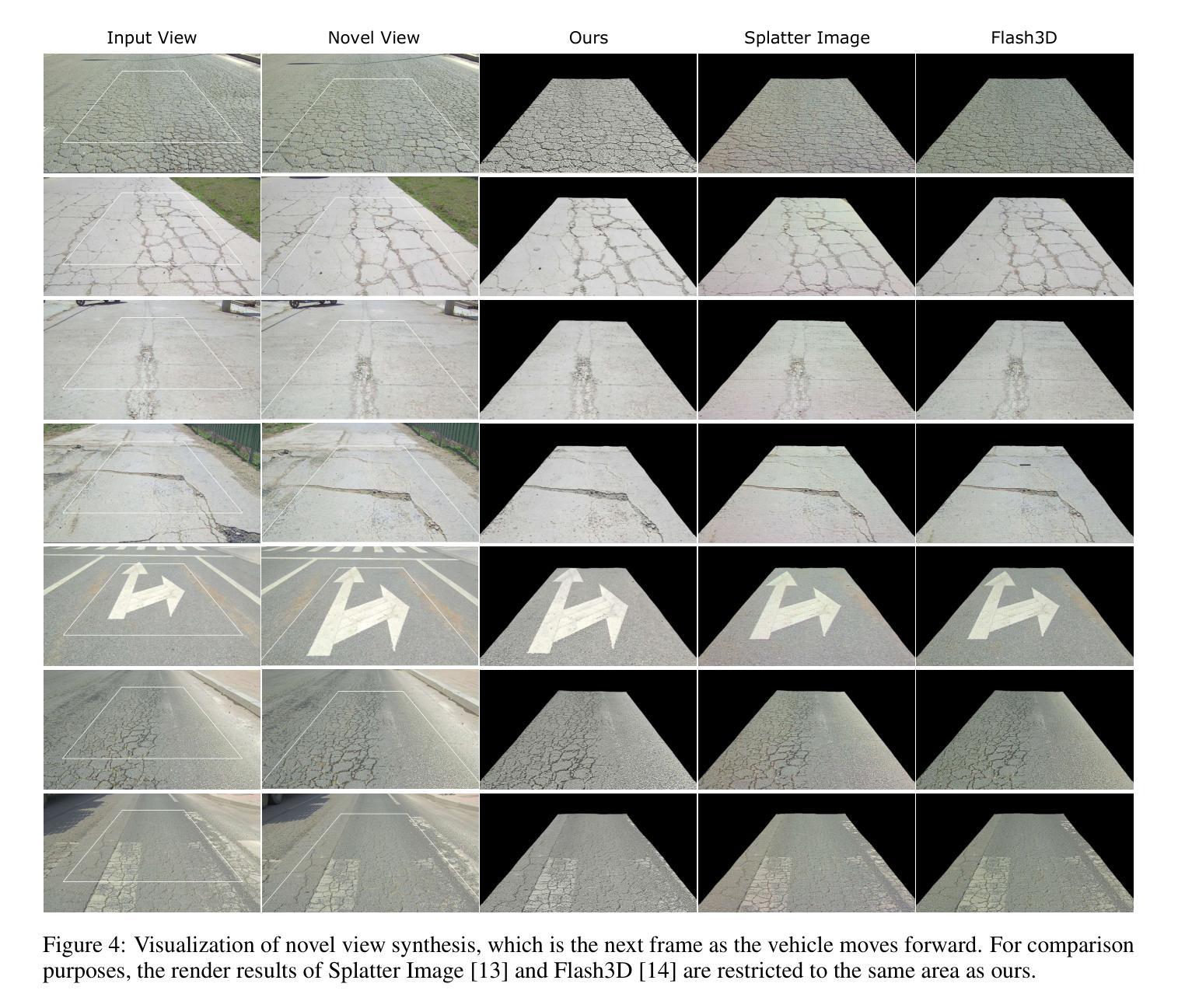