⚠️ 以下所有内容总结都来自于 大语言模型的能力,如有错误,仅供参考,谨慎使用
🔴 请注意:千万不要用于严肃的学术场景,只能用于论文阅读前的初筛!
💗 如果您觉得我们的项目对您有帮助 ChatPaperFree ,还请您给我们一些鼓励!⭐️ HuggingFace免费体验
2025-04-22 更新
ChatNekoHacker: Real-Time Fan Engagement with Conversational Agents
Authors:Takuya Sera, Yusuke Hamano
ChatNekoHacker is a real-time conversational agent system that strengthens fan engagement for musicians. It integrates Amazon Bedrock Agents for autonomous dialogue, Unity for immersive 3D livestream sets, and VOICEVOX for high quality Japanese text-to-speech, enabling two virtual personas to represent the music duo Neko Hacker. In a one-hour YouTube Live with 30 participants, we evaluated the impact of the system. Regression analysis showed that agent interaction significantly elevated fan interest, with perceived fun as the dominant predictor. The participants also expressed a stronger intention to listen to the duo’s music and attend future concerts. These findings highlight entertaining, interactive broadcasts as pivotal to cultivating fandom. Our work offers actionable insights for the deployment of conversational agents in entertainment while pointing to next steps: broader response diversity, lower latency, and tighter fact-checking to curb potential misinformation.
ChatNekoHacker是一个实时对话代理系统,可以增强音乐家的粉丝参与度。它集成了亚马逊的Bedrock代理进行自主对话、Unity进行沉浸式3D直播表演,以及VOICEVOX进行高质量日语文本到语音的转换,让两个虚拟人格代表音乐组合Neko Hacker。在一场持续一小时、有30名参与者的YouTube直播中,我们评估了该系统的影响。回归分析显示,代理互动极大地提高了粉丝的兴趣,其中感知乐趣是主要预测因素。参与者还表达了更强烈的意愿,要听这对组合的音乐和参加未来的音乐会。这些发现突显出有趣、互动性强的广播在培养粉丝方面的重要性。我们的工作为部署娱乐对话代理提供了可操作的见解,同时指出了下一步的方向:更广泛的响应多样性、更低的延迟和更严格的事实核查,以遏制潜在的信息错误。
论文及项目相关链接
PDF Accepted to GenAICHI 2025: Generative AI and HCI at CHI 2025
Summary
ChatNekoHacker系统是一个实时对话代理系统,旨在增强音乐家的粉丝参与度。该系统集成了亚马逊Bedrock代理实现自主对话、Unity用于沉浸式3D直播舞台、VOICEVOX实现高质量日语文本到语音的转换,使得两个虚拟人格能够代表音乐组合Neko Hacker。在一场持续一小时的YouTube直播中,我们发现代理互动显著提升了粉丝兴趣,其中感知的乐趣是主导因素。参与者还表达了更强烈的意愿来听取组合的音乐和参加未来的音乐会。这些发现强调了娱乐性和互动性直播在培养粉丝群体中的重要性。我们的研究为部署对话代理在娱乐领域提供了可操作的见解,并指出了下一步的方向:更广泛的响应多样性、更低的延迟以及更严格的事实核查,以遏制潜在的信息错误。
Key Takeaways
- ChatNekoHacker是一个用于增强音乐家粉丝参与度的实时对话代理系统。
- 系统集成了亚马逊Bedrock代理、Unity和VOICEVOX技术,创建了两个虚拟人格代表音乐组合Neko Hacker。
- 在YouTube直播中测试了该系统,发现代理互动显著提升了粉丝兴趣。
- 感知的乐趣是提升粉丝兴趣的主导因素,参与者更愿意听取组合的音乐和参加未来音乐会。
- 娱乐性和互动性直播在培养粉丝群体方面至关重要。
- 研究提供了部署对话代理在娱乐领域的可操作见解。
点此查看论文截图
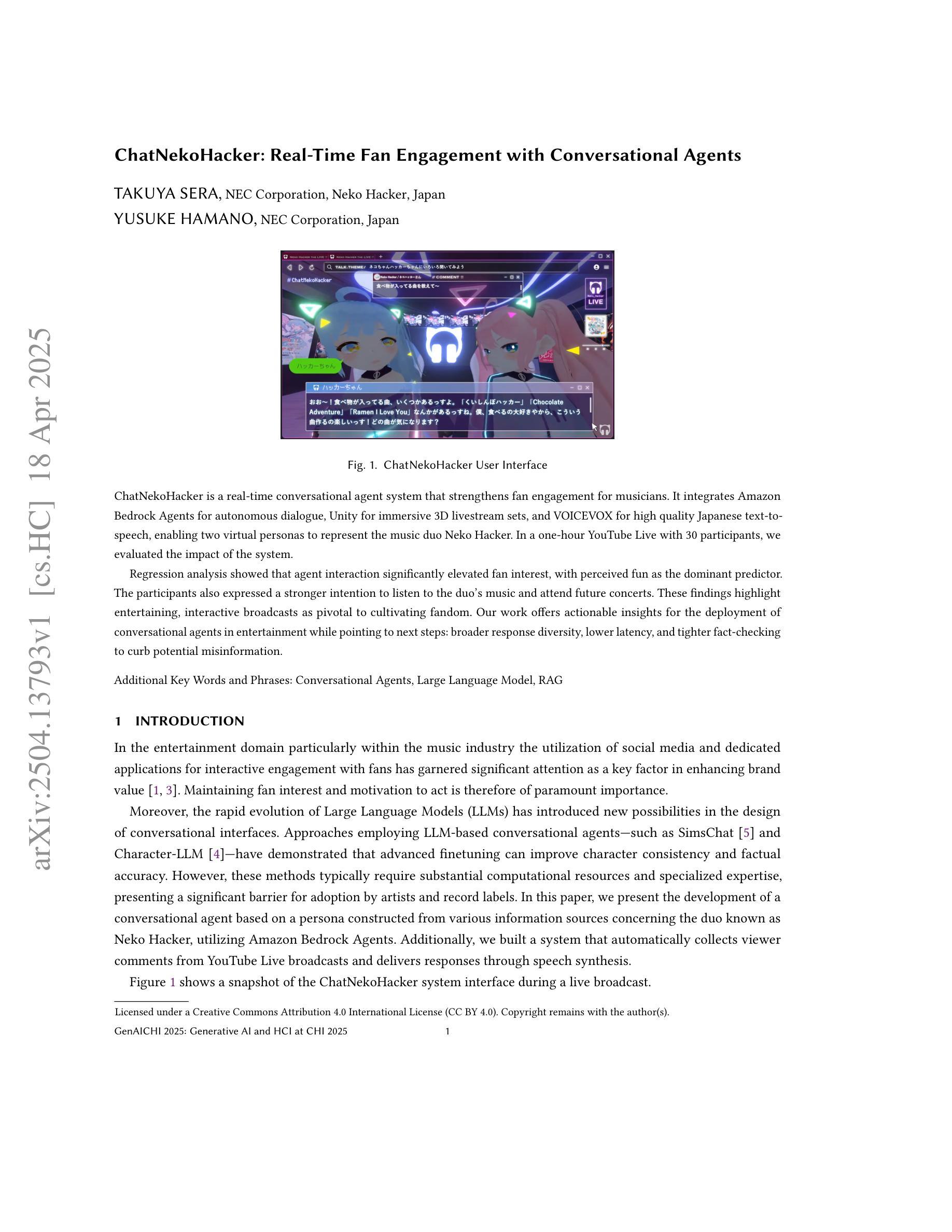
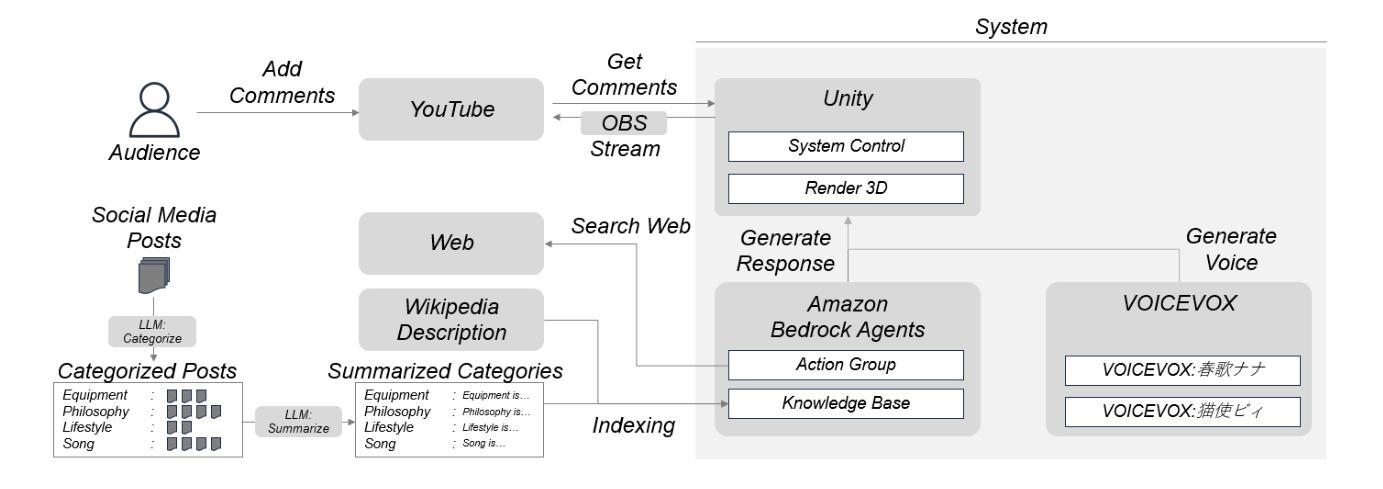
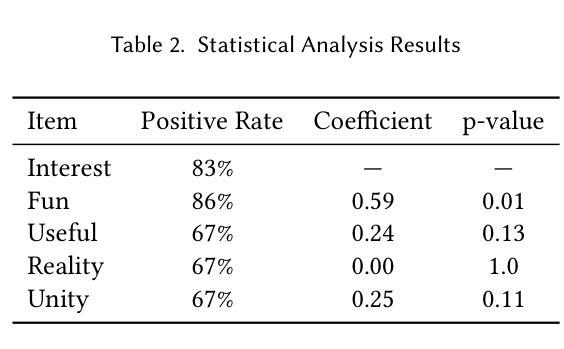
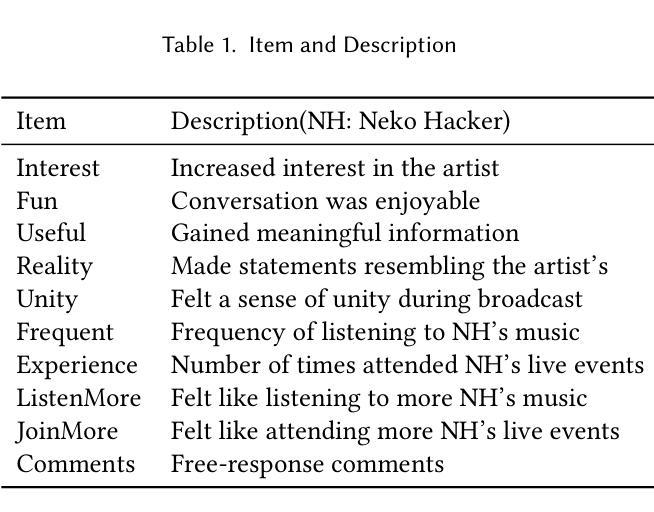
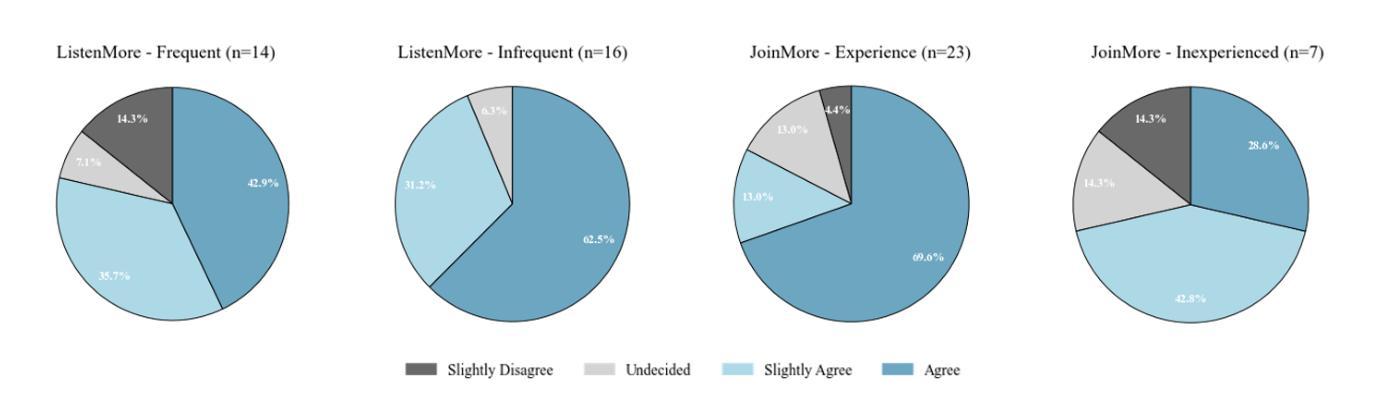
Collective Learning Mechanism based Optimal Transport Generative Adversarial Network for Non-parallel Voice Conversion
Authors:Sandipan Dhar, Md. Tousin Akhter, Nanda Dulal Jana, Swagatam Das
After demonstrating significant success in image synthesis, Generative Adversarial Network (GAN) models have likewise made significant progress in the field of speech synthesis, leveraging their capacity to adapt the precise distribution of target data through adversarial learning processes. Notably, in the realm of State-Of-The-Art (SOTA) GAN-based Voice Conversion (VC) models, there exists a substantial disparity in naturalness between real and GAN-generated speech samples. Furthermore, while many GAN models currently operate on a single generator discriminator learning approach, optimizing target data distribution is more effectively achievable through a single generator multi-discriminator learning scheme. Hence, this study introduces a novel GAN model named Collective Learning Mechanism-based Optimal Transport GAN (CLOT-GAN) model, incorporating multiple discriminators, including the Deep Convolutional Neural Network (DCNN) model, Vision Transformer (ViT), and conformer. The objective of integrating various discriminators lies in their ability to comprehend the formant distribution of mel-spectrograms, facilitated by a collective learning mechanism. Simultaneously, the inclusion of Optimal Transport (OT) loss aims to precisely bridge the gap between the source and target data distribution, employing the principles of OT theory. The experimental validation on VCC 2018, VCTK, and CMU-Arctic datasets confirms that the CLOT-GAN-VC model outperforms existing VC models in objective and subjective assessments.
在图像合成领域取得巨大成功后,生成对抗网络(GAN)模型在语音合成领域也取得了显著进展,利用对抗学习过程来适应目标数据的精确分布。特别是,在最先进的基于GAN的语音转换(VC)模型中,真实和GAN生成的语音样本之间在自然度上存在很大差异。此外,虽然许多GAN模型目前采用单一生成器鉴别器学习方法,但通过单一生成器多鉴别器学习方案可以更有效地优化目标数据分布。因此,本研究引入了一种新型GAN模型,称为基于集体学习机制的优化传输GAN(CLOT-GAN)模型,该模型结合了多个鉴别器,包括深度卷积神经网络(DCNN)模型、视觉变压器(ViT)和符合作者。集成各种鉴别器的目的在于它们理解熔谱图的共振峰分布的能力,这是通过集体学习机制实现的。同时,引入最优传输(OT)损失旨在通过最优传输理论的原则,精确弥合源数据分布与目标数据分布之间的差距。在VCC 2018、VCTK和CMU-Arctic数据集上的实验验证表明,CLOT-GAN-VC模型在客观和主观评估中优于现有VC模型。
论文及项目相关链接
PDF 7 pages, 2 figures, 3 tables
Summary
基于生成对抗网络(GAN)的模型在语音合成领域取得了显著进展,特别是在最先进(SOTA)的基于GAN的语音转换(VC)模型中。本研究引入了一种名为CLOT-GAN的新型GAN模型,采用多种鉴别器,包括深度卷积神经网络(DCNN)、视觉变压器(ViT)和确认器。该模型通过集体学习机制理解梅尔频谱图的音域分布,并精确缩小源和目标数据分布之间的差距。实验验证表明,CLOT-GAN-VC模型在客观和主观评估中优于现有VC模型。
Key Takeaways
- GAN模型在语音合成领域取得显著进展,尤其是基于GAN的语音转换(VC)模型。
- 现有GAN模型大多采用单一生成器鉴别器学习方式,而优化目标数据分布可通过单一生成器多鉴别器学习方案更有效实现。
- CLOT-GAN模型引入多种鉴别器,包括DCNN、ViT和确认器,以理解梅尔频谱图的音域分布。
- CLOT-GAN采用集体学习机制,旨在提高模型性能。
- CLOT-GAN模型结合最优传输(OT)损失,旨在精确缩小源和目标数据分布之间的差距。
- 实验验证显示,CLOT-GAN-VC模型在客观和主观评估中均优于现有VC模型。
点此查看论文截图
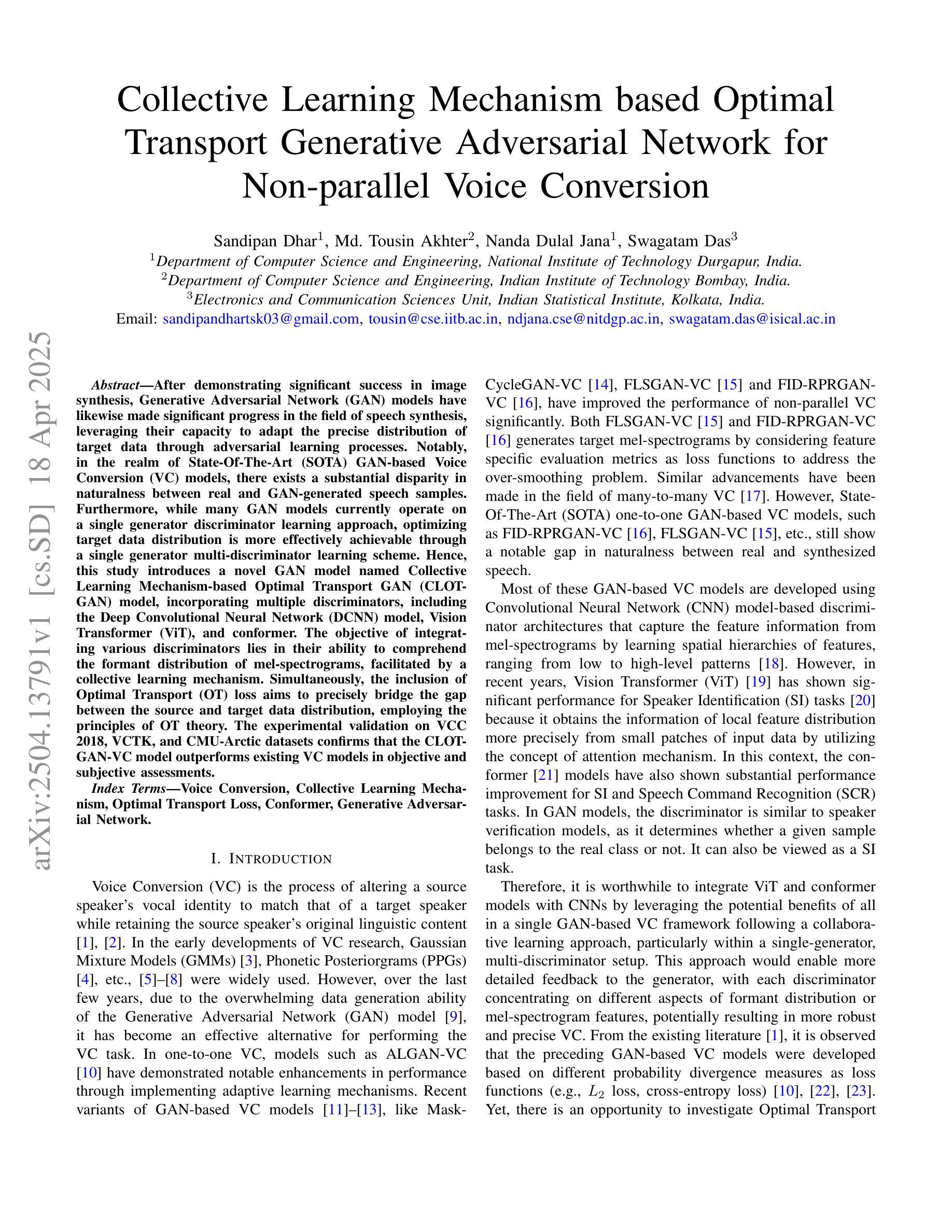
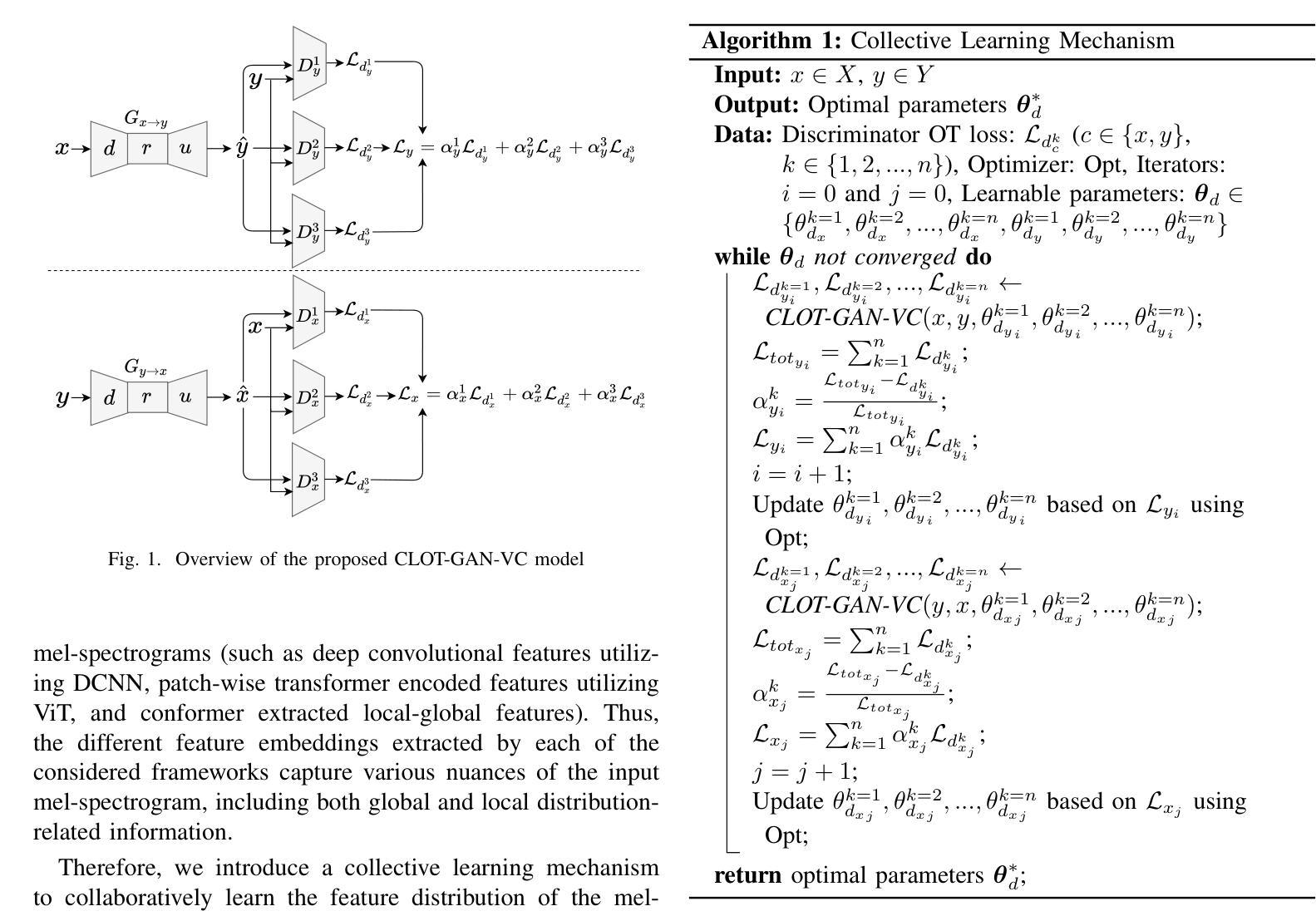
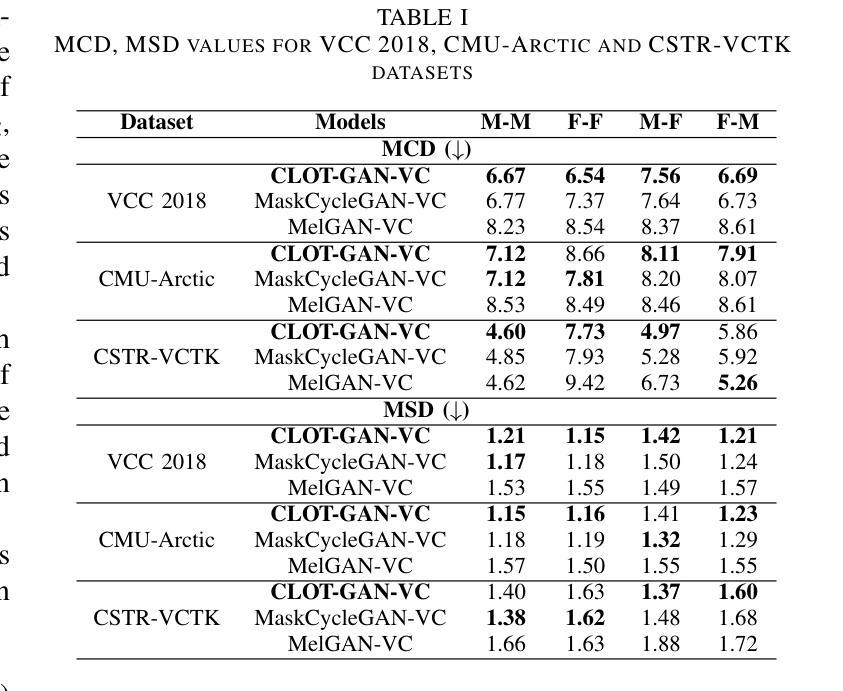
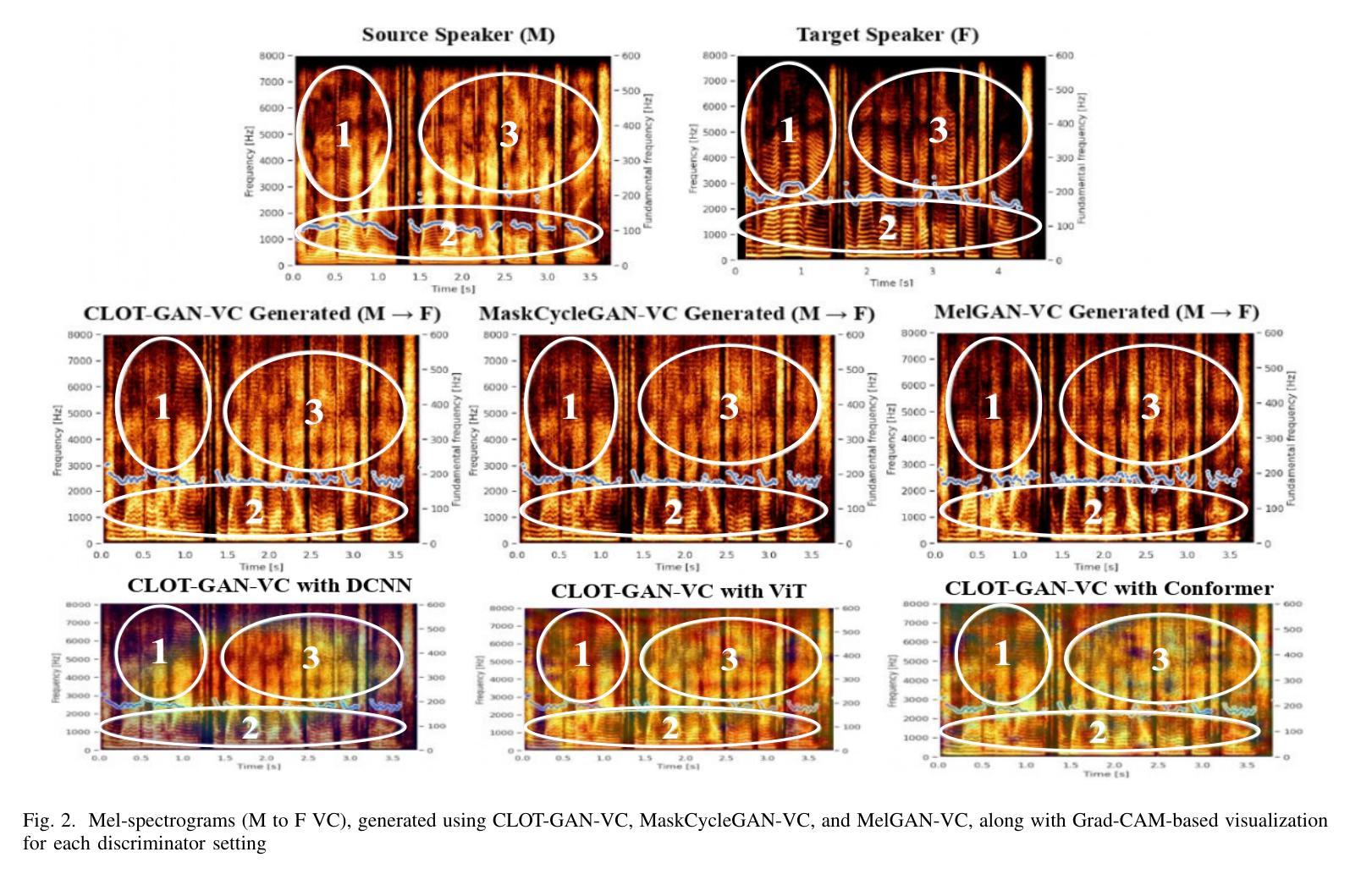
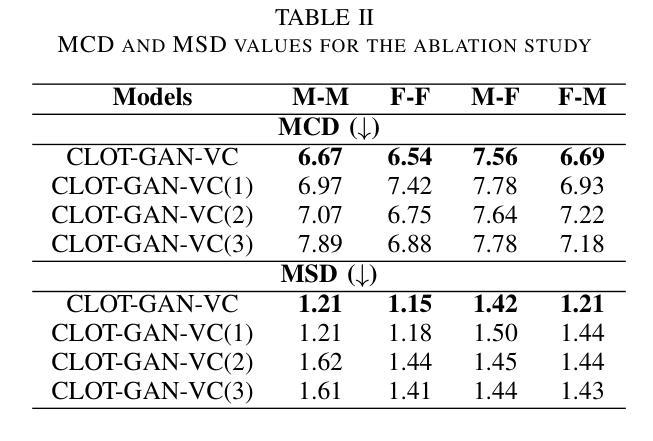
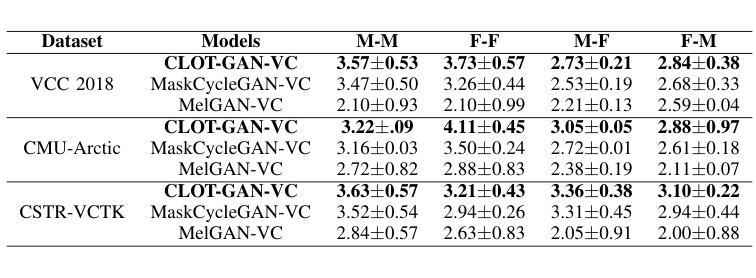
EmoVoice: LLM-based Emotional Text-To-Speech Model with Freestyle Text Prompting
Authors:Guanrou Yang, Chen Yang, Qian Chen, Ziyang Ma, Wenxi Chen, Wen Wang, Tianrui Wang, Yifan Yang, Zhikang Niu, Wenrui Liu, Fan Yu, Zhihao Du, Zhifu Gao, ShiLiang Zhang, Xie Chen
Human speech goes beyond the mere transfer of information; it is a profound exchange of emotions and a connection between individuals. While Text-to-Speech (TTS) models have made huge progress, they still face challenges in controlling the emotional expression in the generated speech. In this work, we propose EmoVoice, a novel emotion-controllable TTS model that exploits large language models (LLMs) to enable fine-grained freestyle natural language emotion control, and a phoneme boost variant design that makes the model output phoneme tokens and audio tokens in parallel to enhance content consistency, inspired by chain-of-thought (CoT) and chain-of-modality (CoM) techniques. Besides, we introduce EmoVoice-DB, a high-quality 40-hour English emotion dataset featuring expressive speech and fine-grained emotion labels with natural language descriptions. EmoVoice achieves state-of-the-art performance on the English EmoVoice-DB test set using only synthetic training data, and on the Chinese Secap test set using our in-house data. We further investigate the reliability of existing emotion evaluation metrics and their alignment with human perceptual preferences, and explore using SOTA multimodal LLMs GPT-4o-audio and Gemini to assess emotional speech. Demo samples are available at https://anonymous.4open.science/r/EmoVoice-DF55. Dataset, code, and checkpoints will be released.
人类语音不仅仅是为了传递信息,更是情感交流和个人之间联系的深刻体现。尽管文本转语音(TTS)模型已经取得了巨大的进步,但在控制生成语音的情感表达方面仍面临挑战。在这项工作中,我们提出了EmoVoice,一种新型的情感可控TTS模型,它利用大型语言模型(LLM)实现细粒度的自由式自然语言情感控制,并设计了一种音素增强变体,使模型能够并行输出音素标记和音频标记,以增强内容的一致性,这一设计灵感来源于思维链(CoT)和模态链(CoM)技术。此外,我们还介绍了EmoVoice-DB,这是一个高质量、包含40小时英语情感的数据集,其中包含表达性语音和带有自然语言描述的精细情感标签。EmoVoice仅使用合成训练数据即可在英文EmoVoice-DB测试集上实现最新性能,并在使用我们内部数据的中文Secap测试集上表现优异。我们进一步研究了现有情感评估指标的可靠性及其与人类感知偏好的一致性,并探讨了使用最先进的多模态LLM GPT-4o-audio和Gemini来评估情感语音。演示样本可在[https://anonymous.4open.science/r/EmoVoice-DF55找到。数据集、代码和检查点将一并发布。
论文及项目相关链接
Summary
本文提出了一项名为EmoVoice的创新性的情感可控的文本转语音模型。它借助大型语言模型实现精细自由度的自然语言情感控制,通过采用音素增强变体设计输出音素令牌和音频令牌,以提高内容一致性。此外,还介绍了高质量的情感数据集EmoVoice-DB,其特色是包含表达性语音和精细的情感标签。该模型在英文和中文数据集上实现了卓越性能,并探讨了现有情感评估指标的可靠性及其与人类感知偏好的一致性。
Key Takeaways
- EmoVoice是一个情感可控的文本转语音模型,利用大型语言模型实现精细的自由度自然语言情感控制。
- EmoVoice采用音素增强变体设计,同时输出音素令牌和音频令牌,以增强内容一致性。
- 引入了高质量的情感数据集EmoVoice-DB,包含表达性语音和精细的情感标签。
- EmoVoice在英文和中文数据集上实现了卓越性能。
- 现有情感评估指标的可靠性得到探讨,同时评估了其与人类感知偏好的一致性。
- 提供了模型演示样本,并计划公开数据集、代码和检查点。
点此查看论文截图
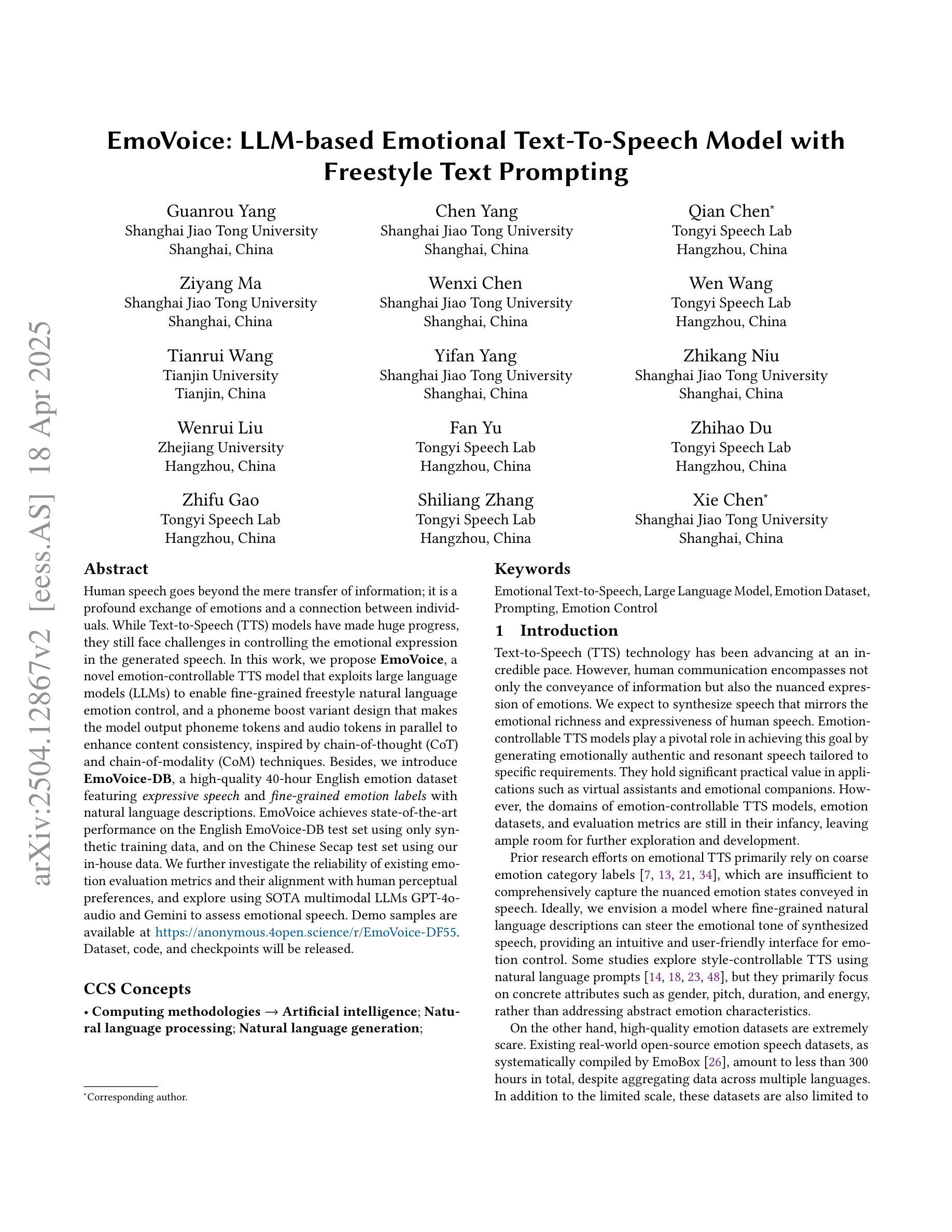
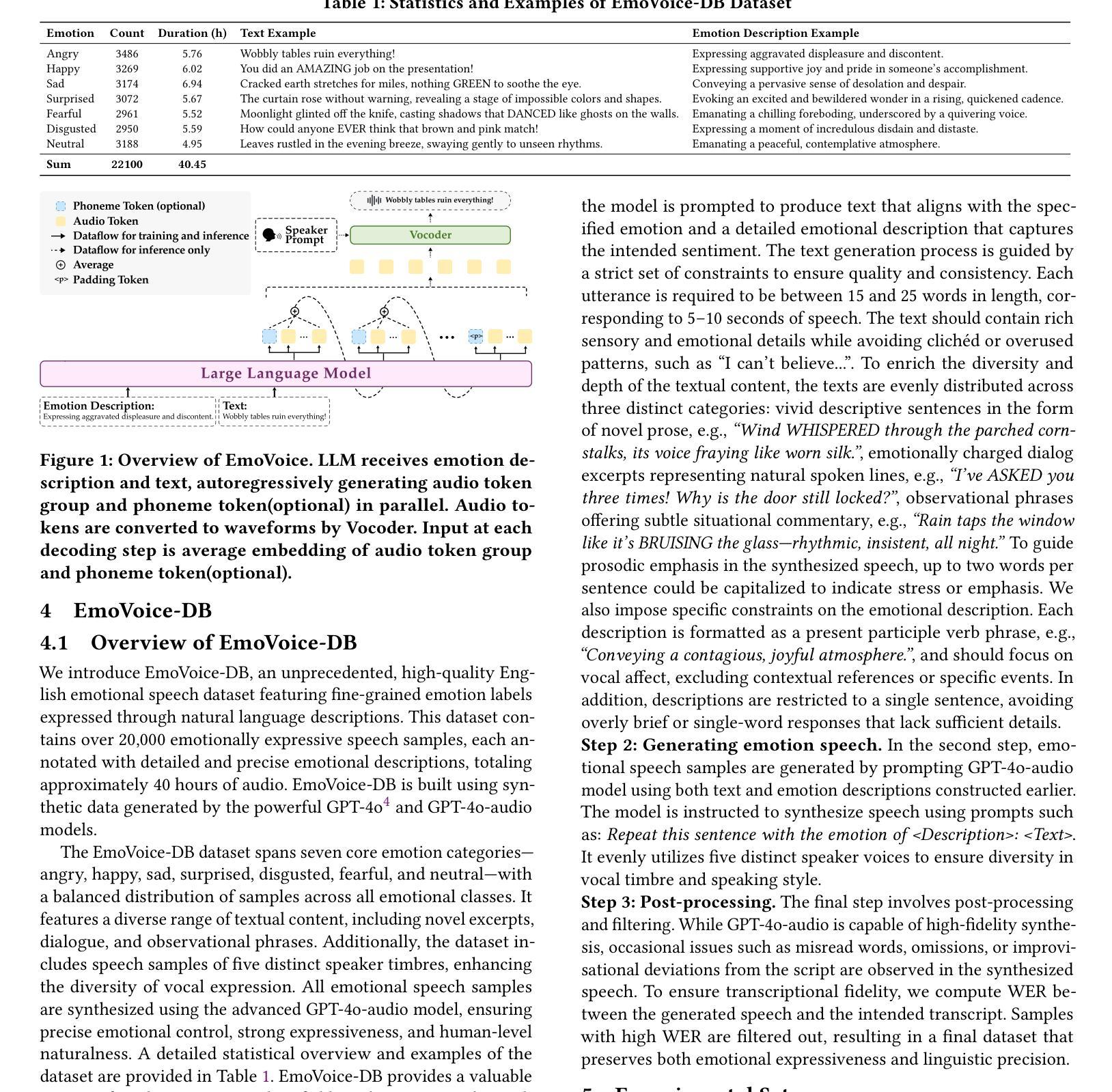
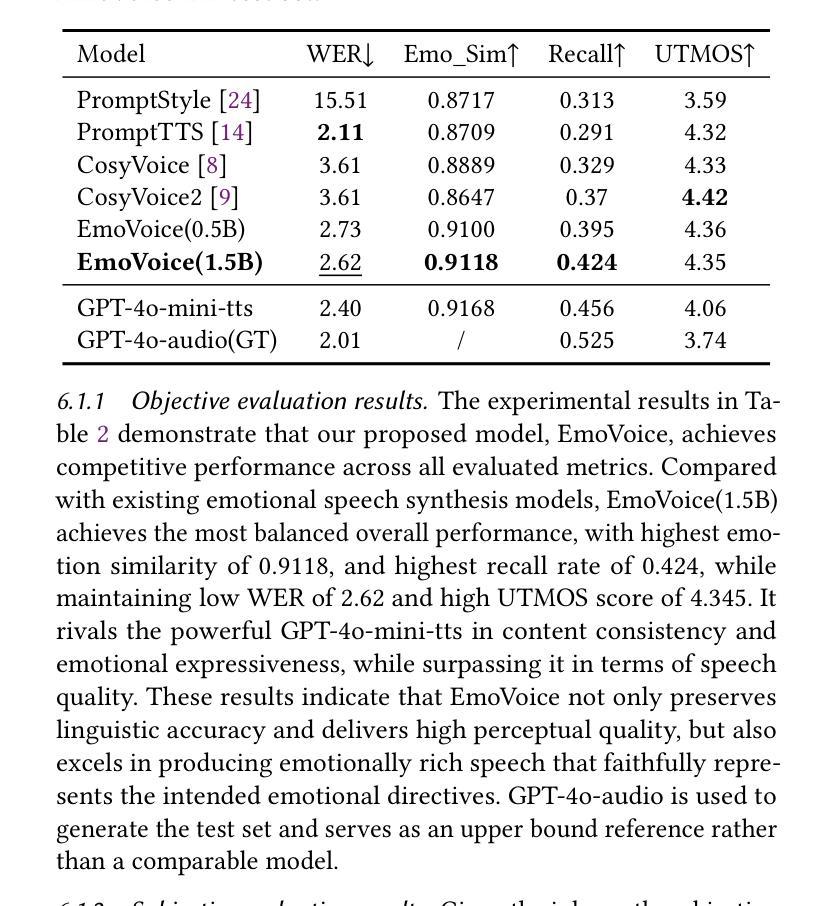
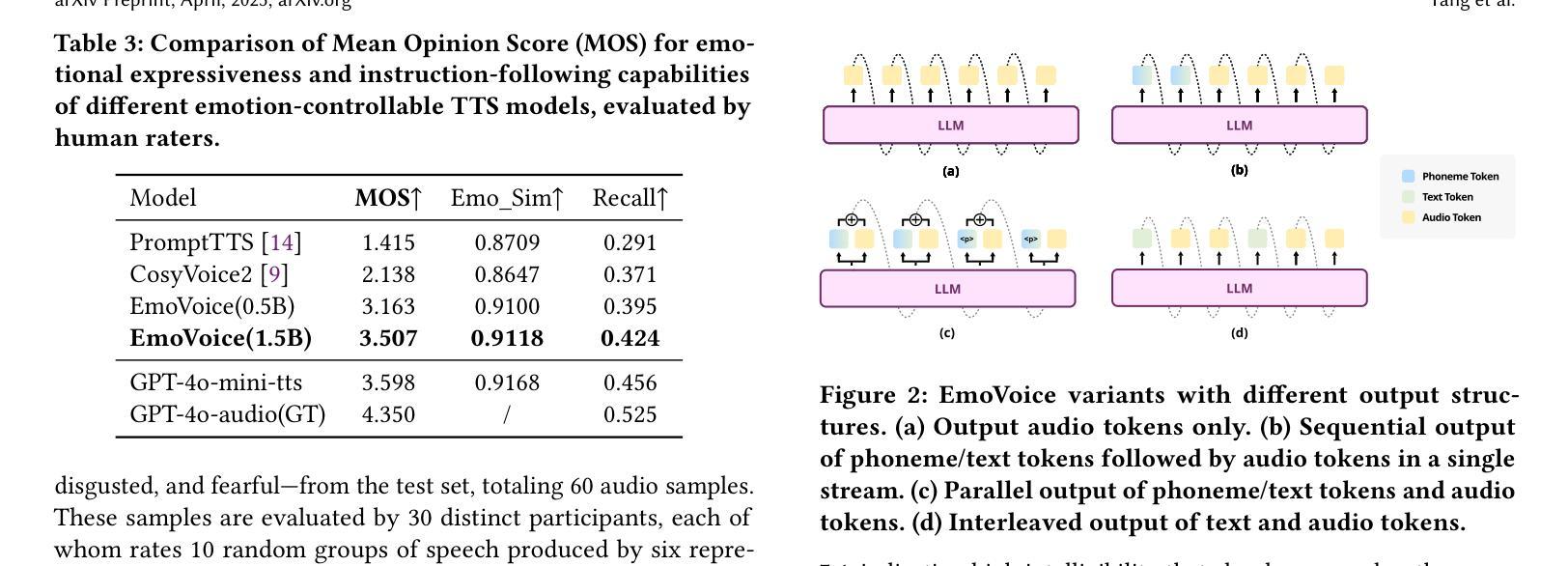
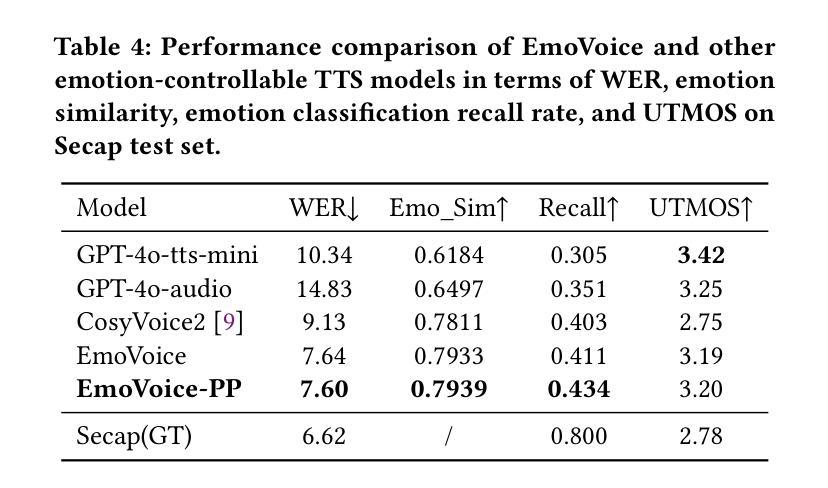