⚠️ 以下所有内容总结都来自于 大语言模型的能力,如有错误,仅供参考,谨慎使用
🔴 请注意:千万不要用于严肃的学术场景,只能用于论文阅读前的初筛!
💗 如果您觉得我们的项目对您有帮助 ChatPaperFree ,还请您给我们一些鼓励!⭐️ HuggingFace免费体验
2025-04-22 更新
Supervising 3D Talking Head Avatars with Analysis-by-Audio-Synthesis
Authors:Radek Daněček, Carolin Schmitt, Senya Polikovsky, Michael J. Black
In order to be widely applicable, speech-driven 3D head avatars must articulate their lips in accordance with speech, while also conveying the appropriate emotions with dynamically changing facial expressions. The key problem is that deterministic models produce high-quality lip-sync but without rich expressions, whereas stochastic models generate diverse expressions but with lower lip-sync quality. To get the best of both, we seek a stochastic model with accurate lip-sync. To that end, we develop a new approach based on the following observation: if a method generates realistic 3D lip motions, it should be possible to infer the spoken audio from the lip motion. The inferred speech should match the original input audio, and erroneous predictions create a novel supervision signal for training 3D talking head avatars with accurate lip-sync. To demonstrate this effect, we propose THUNDER (Talking Heads Under Neural Differentiable Elocution Reconstruction), a 3D talking head avatar framework that introduces a novel supervision mechanism via differentiable sound production. First, we train a novel mesh-to-speech model that regresses audio from facial animation. Then, we incorporate this model into a diffusion-based talking avatar framework. During training, the mesh-to-speech model takes the generated animation and produces a sound that is compared to the input speech, creating a differentiable analysis-by-audio-synthesis supervision loop. Our extensive qualitative and quantitative experiments demonstrate that THUNDER significantly improves the quality of the lip-sync of talking head avatars while still allowing for generation of diverse, high-quality, expressive facial animations.
为了广泛应用于各个领域,语音驱动的三维头部化身必须根据语音进行唇部运动,同时借助动态变化的面部表情来传达适当的情绪。关键问题在于,确定性模型虽然能够产生高质量的唇部同步效果,但缺乏丰富的表情;而随机模型虽然能够生成多种表情,但唇部同步质量较低。为了结合两者的优点,我们寻求具有精确唇部同步的随机模型。为此,我们基于以下观察结果开发了一种新方法:如果一种方法能够生成逼真的3D唇部运动,那么就应该可以从唇部运动中推断出语音。推断出的语音应与原始输入音频匹配,错误的预测可以创建一种新的监督信号,用于训练具有精确唇部同步的3D对话头部化身。为了证明这一效果,我们提出了THUNDER(神经可微语音重建下的对话头部,Talking Heads Under Neural Differentiable Elocution Reconstruction),这是一个3D对话头部化身框架,通过可微声音生产引入了一种新型监督机制。首先,我们训练了一种新型网格到语音模型,该模型可以从面部动画中回归音频。然后,我们将该模型纳入基于扩散的谈话化身框架。在训练过程中,网格到语音模型接受生成的动画并产生声音,该声音与输入语音进行比较,从而创建一个可通过音频合成进行分析的可微监督循环。我们的广泛定性和定量实验表明,THUNDER显著提高了对话头部化身的唇部同步质量,同时仍能够生成多样、高质量、富有表现力的面部动画。
论文及项目相关链接
Summary
本文探讨了语音驱动的3D头像在生成过程中的核心问题,即需要在唇形同步与面部表情表达之间取得平衡。为此,提出了一种基于神经可微语音重建的新方法THUNDER,旨在通过创新的监督机制实现高质量且表情丰富的头像动画。其核心思想是训练一个从面部动画回归音频的模型,进而在训练过程中创建一个可微的音频合成监督循环,以改善头像的唇形同步质量并生成多样化的高质量表情动画。
Key Takeaways
- 语音驱动的3D头像需要实现唇形同步与情绪表达的平衡。
- 现有模型在确定性模型与随机模型之间存在权衡:确定性模型实现高质量的唇形同步但缺乏丰富表情,而随机模型生成多样表情但唇形同步质量较低。
- THUNDER方法结合神经可微语音重建技术,旨在解决上述问题。
- THUNDER引入了一种新型监督机制,通过训练一个从面部动画回归音频的模型,改善头像的唇形同步质量。
- 该模型被纳入基于扩散的头像动画框架中。
- 在训练过程中,创建的音频合成监督循环确保了生成的动画既具有高质量的唇形同步,又展现出多样化的表情。
点此查看论文截图
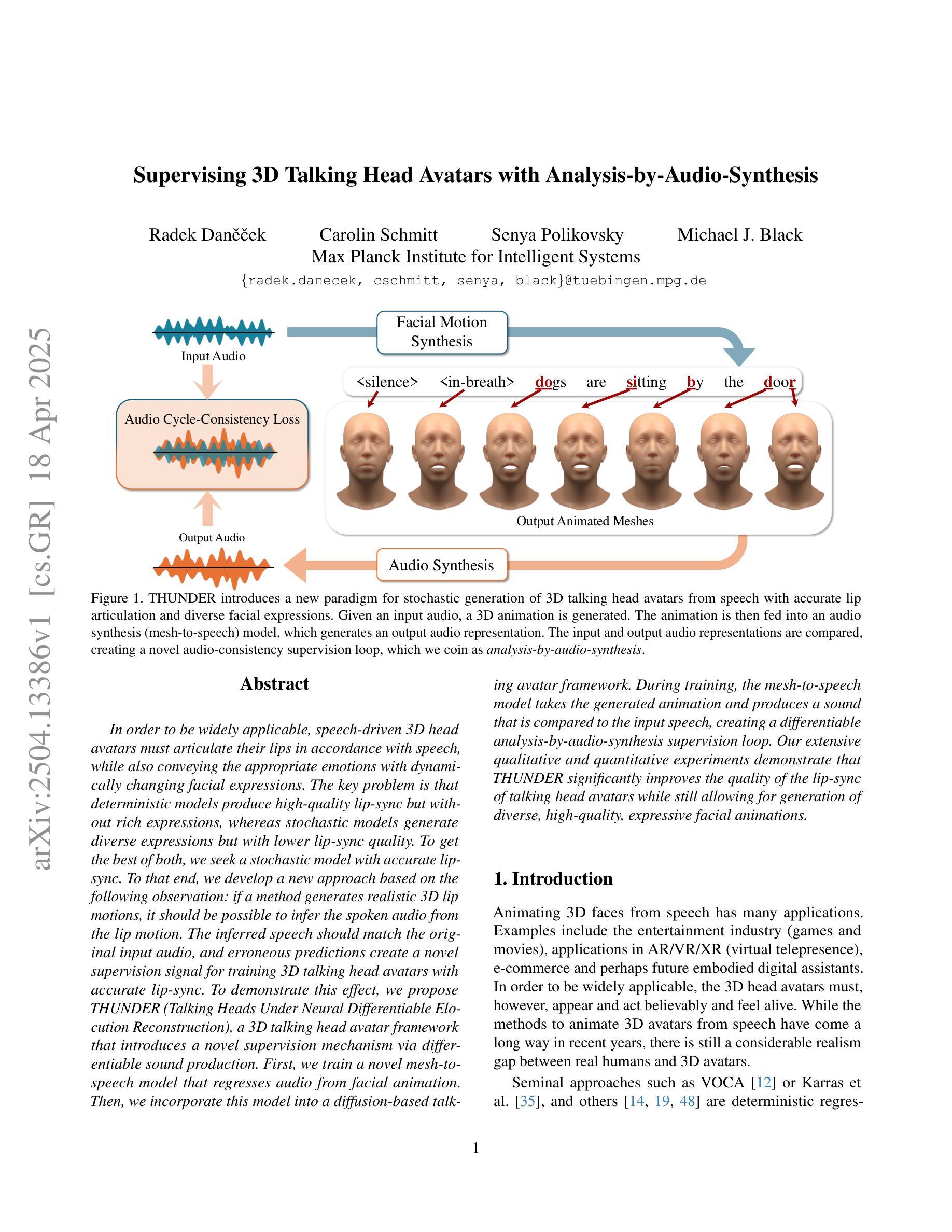
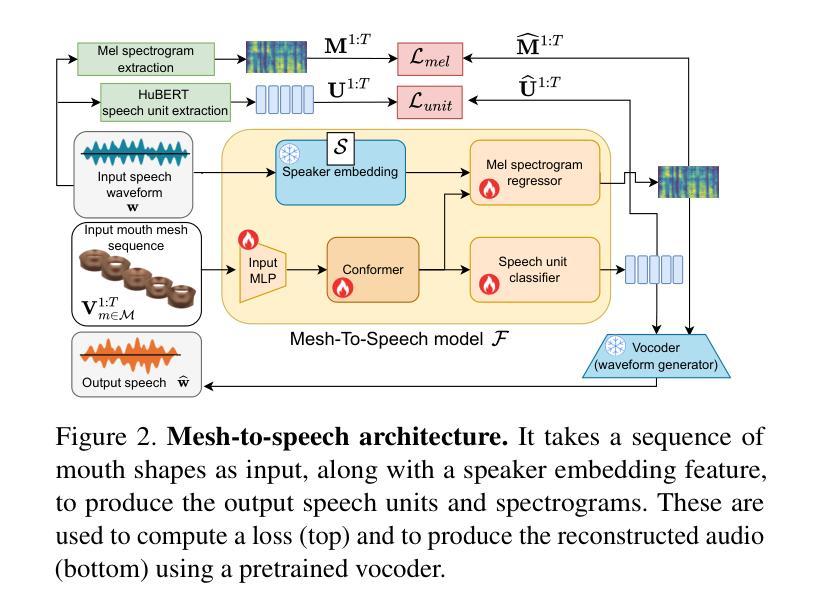
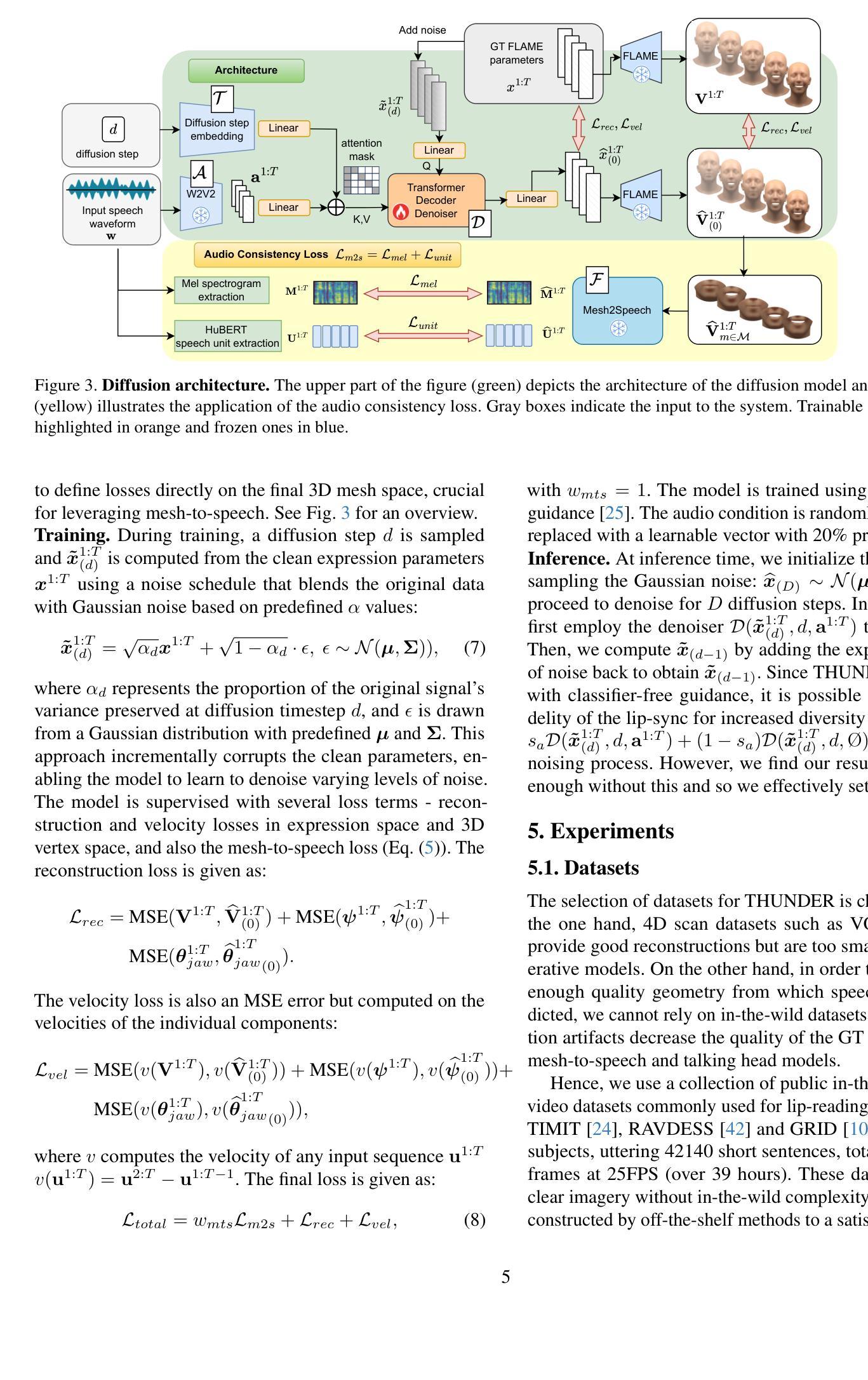
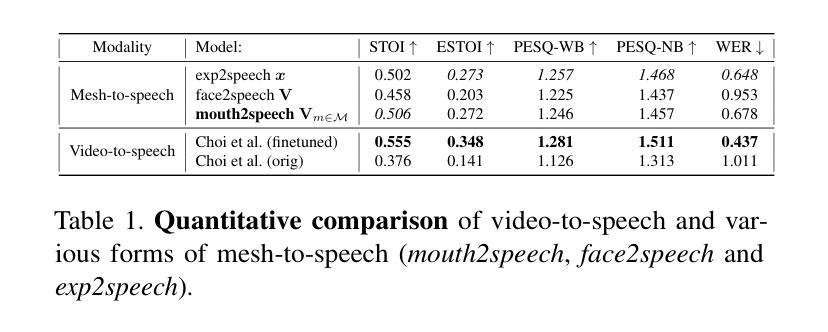