⚠️ 以下所有内容总结都来自于 大语言模型的能力,如有错误,仅供参考,谨慎使用
🔴 请注意:千万不要用于严肃的学术场景,只能用于论文阅读前的初筛!
💗 如果您觉得我们的项目对您有帮助 ChatPaperFree ,还请您给我们一些鼓励!⭐️ HuggingFace免费体验
2025-04-23 更新
SuperCL: Superpixel Guided Contrastive Learning for Medical Image Segmentation Pre-training
Authors:Shuang Zeng, Lei Zhu, Xinliang Zhang, Hangzhou He, Yanye Lu
Medical image segmentation is a critical yet challenging task, primarily due to the difficulty of obtaining extensive datasets of high-quality, expert-annotated images. Contrastive learning presents a potential but still problematic solution to this issue. Because most existing methods focus on extracting instance-level or pixel-to-pixel representation, which ignores the characteristics between intra-image similar pixel groups. Moreover, when considering contrastive pairs generation, most SOTA methods mainly rely on manually setting thresholds, which requires a large number of gradient experiments and lacks efficiency and generalization. To address these issues, we propose a novel contrastive learning approach named SuperCL for medical image segmentation pre-training. Specifically, our SuperCL exploits the structural prior and pixel correlation of images by introducing two novel contrastive pairs generation strategies: Intra-image Local Contrastive Pairs (ILCP) Generation and Inter-image Global Contrastive Pairs (IGCP) Generation. Considering superpixel cluster aligns well with the concept of contrastive pairs generation, we utilize the superpixel map to generate pseudo masks for both ILCP and IGCP to guide supervised contrastive learning. Moreover, we also propose two modules named Average SuperPixel Feature Map Generation (ASP) and Connected Components Label Generation (CCL) to better exploit the prior structural information for IGCP. Finally, experiments on 8 medical image datasets indicate our SuperCL outperforms existing 12 methods. i.e. Our SuperCL achieves a superior performance with more precise predictions from visualization figures and 3.15%, 5.44%, 7.89% DSC higher than the previous best results on MMWHS, CHAOS, Spleen with 10% annotations. Our code will be released after acceptance.
医疗图像分割是一项至关重要的任务,但由于难以获取大量高质量、专家标注的图像数据集,它同时也是一个巨大的挑战。对比学习为解决这一问题提供了潜在但仍有问题的解决方案。因为大多数现有方法主要关注实例级或像素到像素的表示提取,这忽略了图像内相似像素组之间的特征。此外,在生成对比对时,大多数最先进的方法主要依赖于人工设置阈值,这需要大量的梯度实验,缺乏效率和泛化能力。为了解决这些问题,我们提出了一种名为SuperCL的新型对比学习方法,用于医学图像分割的预训练。具体来说,我们的SuperCL通过引入两种新型的对比对生成策略来利用图像的结构先验知识和像素相关性:图像内局部对比对(ILCP)生成和图像间全局对比对(IGCP)生成。考虑到超像素集群与对比对生成的概念相符,我们利用超像素图生成ILCP和IGCP的伪掩码,以指导有监督的对比学习。此外,我们还提出了名为平均超像素特征图生成(ASP)和连通组件标签生成(CCL)的两个模块,以更好地利用IGCP的先验结构信息。最后,在8个医学图像数据集上的实验表明,我们的SuperCL优于现有的12种方法。例如,我们的SuperCL在可视化图上实现了更精确的预测,并且在MMWHS、CHAOS和Spleen数据集上的DSC得分比之前的最佳结果分别高出3.15%、5.44%、7.89%。代码将在接受后发布。
论文及项目相关链接
Summary
针对医学图像分割任务中数据集获取困难的问题,提出了一种名为SuperCL的新型对比学习方法。该方法通过引入两种新型对比对生成策略:ILCP(Intra-image Local Contrastive Pairs Generation)和IGCP(Inter-image Global Contrastive Pairs Generation),利用结构先验和像素相关性。通过超像素图生成伪掩膜来指导对比学习,并提出ASP和CCL两个模块以更好地利用先验结构信息。在8个医学图像数据集上的实验表明,SuperCL相较于现有方法表现出优越性能,预测更精确,并在MMWHS、CHAOS、Spleen等数据集上实现了较高的DSC得分。
Key Takeaways
- 医学图像分割任务面临数据集获取难题,特别是高质量、专家标注的图像。
- 对比学习为此提供了潜在解决方案,但现有方法忽略图像内相似像素群的特征。
- 提出的SuperCL方法利用结构先验和像素相关性,引入ILCP和IGCP两种新型对比对生成策略。
- 通过超像素图生成伪掩膜来指导对比学习,强化对比对生成的效果。
- ASP和CCL两个模块用于更好地利用先验结构信息,提高模型性能。
- 在多个医学图像数据集上的实验表明,SuperCL相较于现有方法具有优越性能。
点此查看论文截图
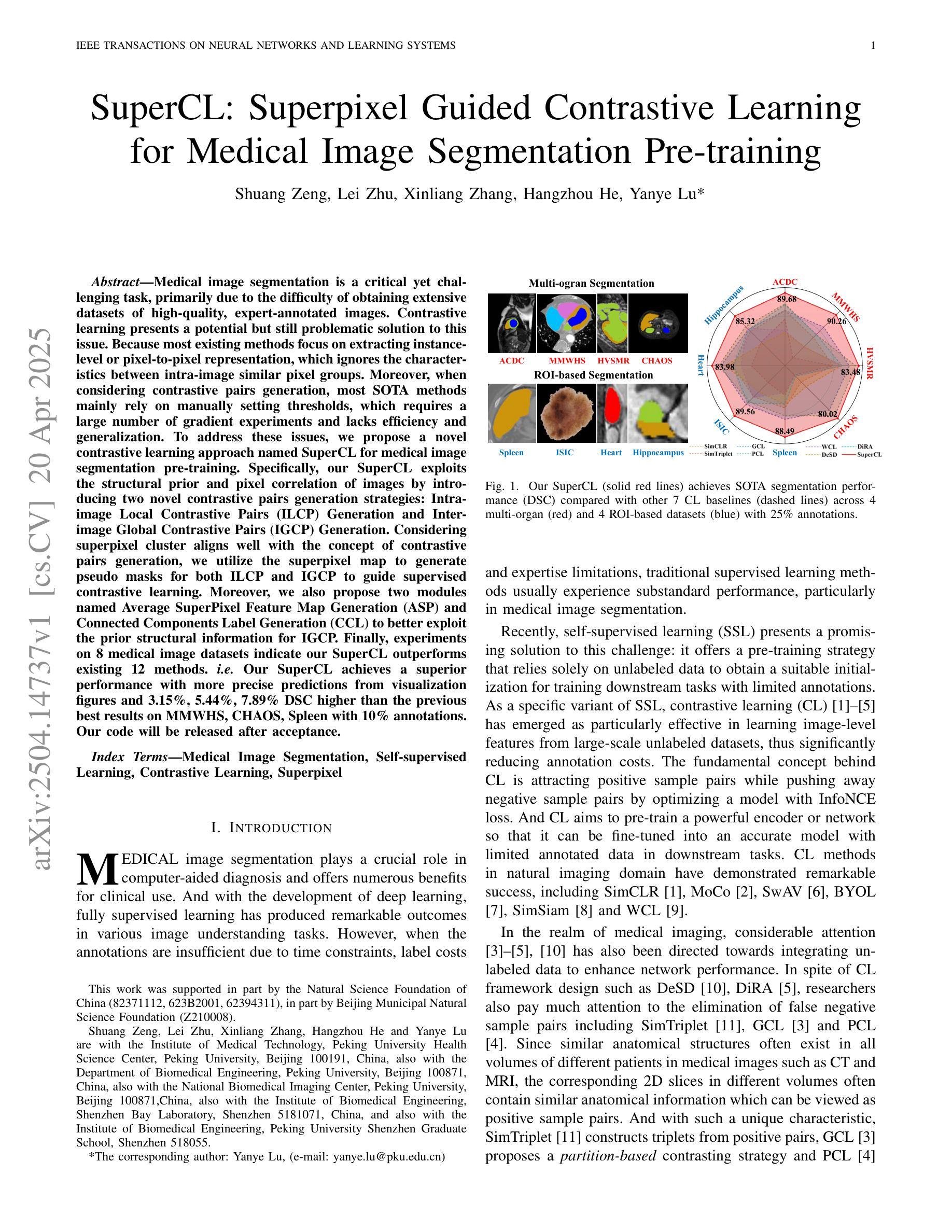
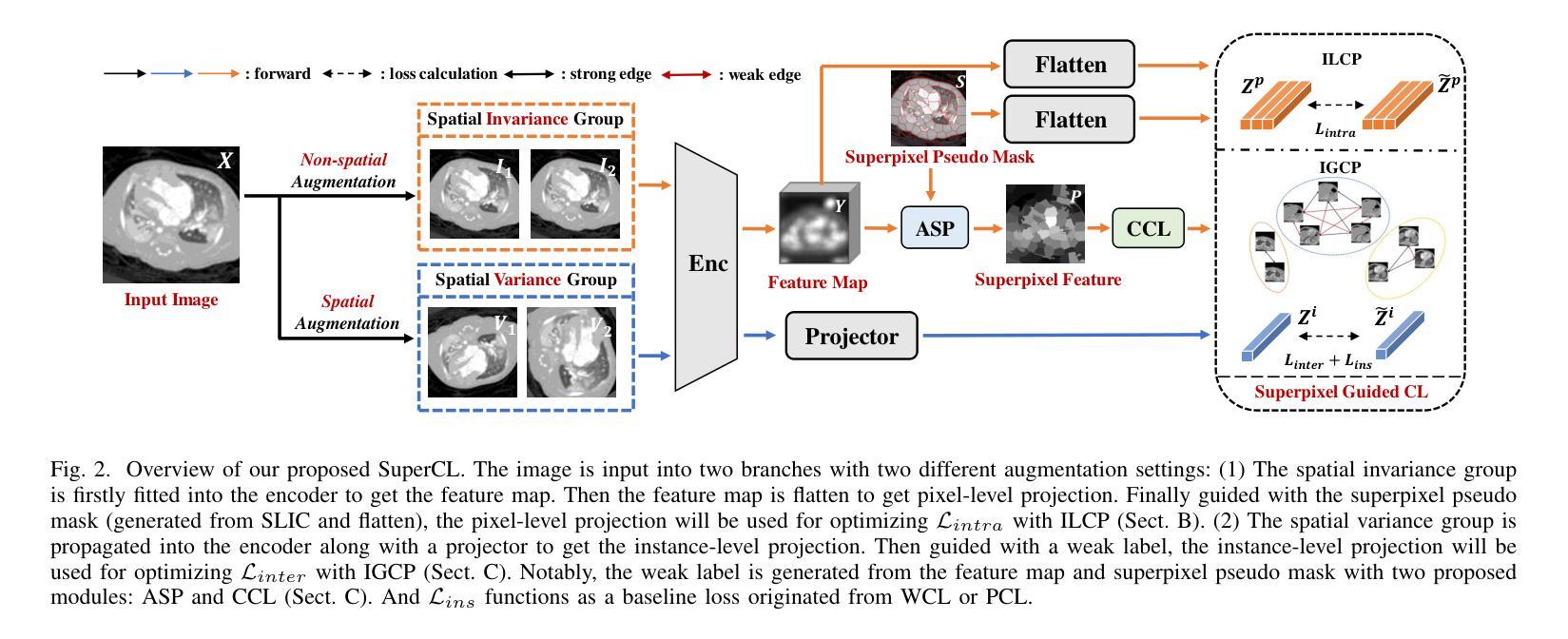
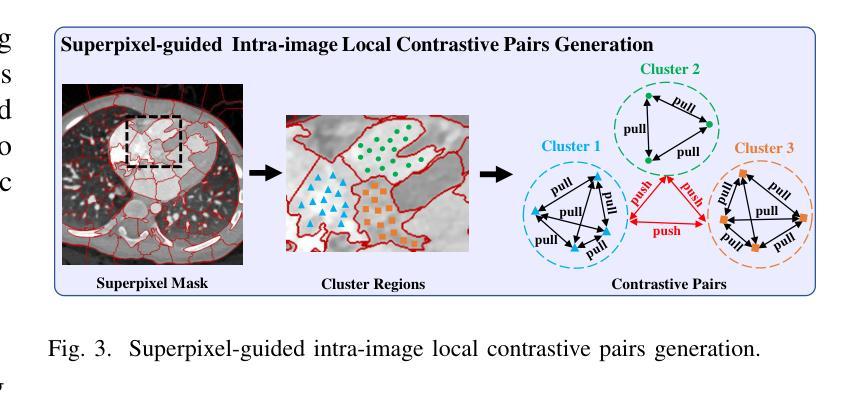
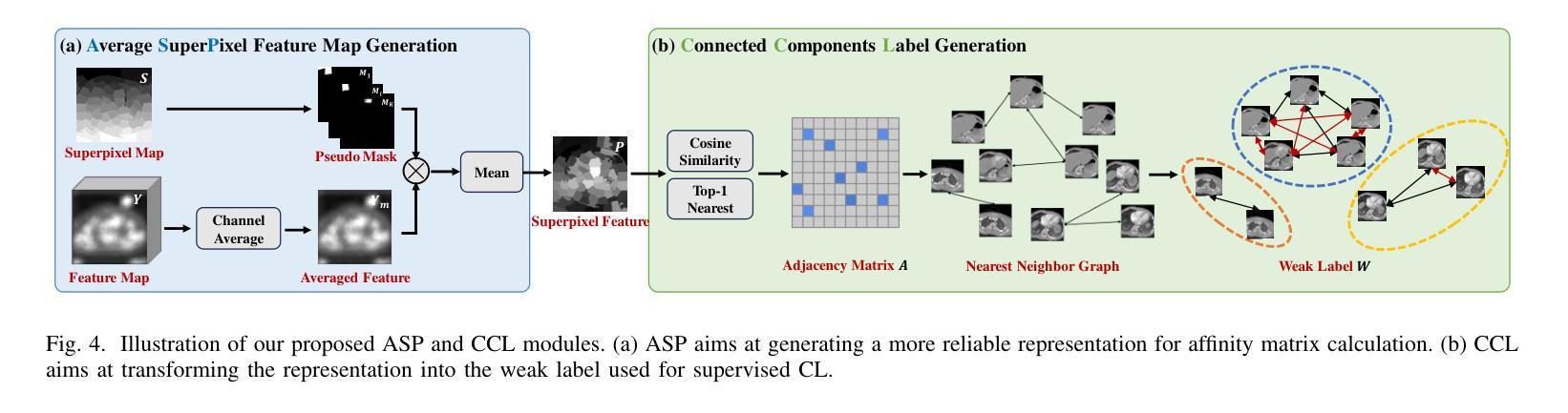
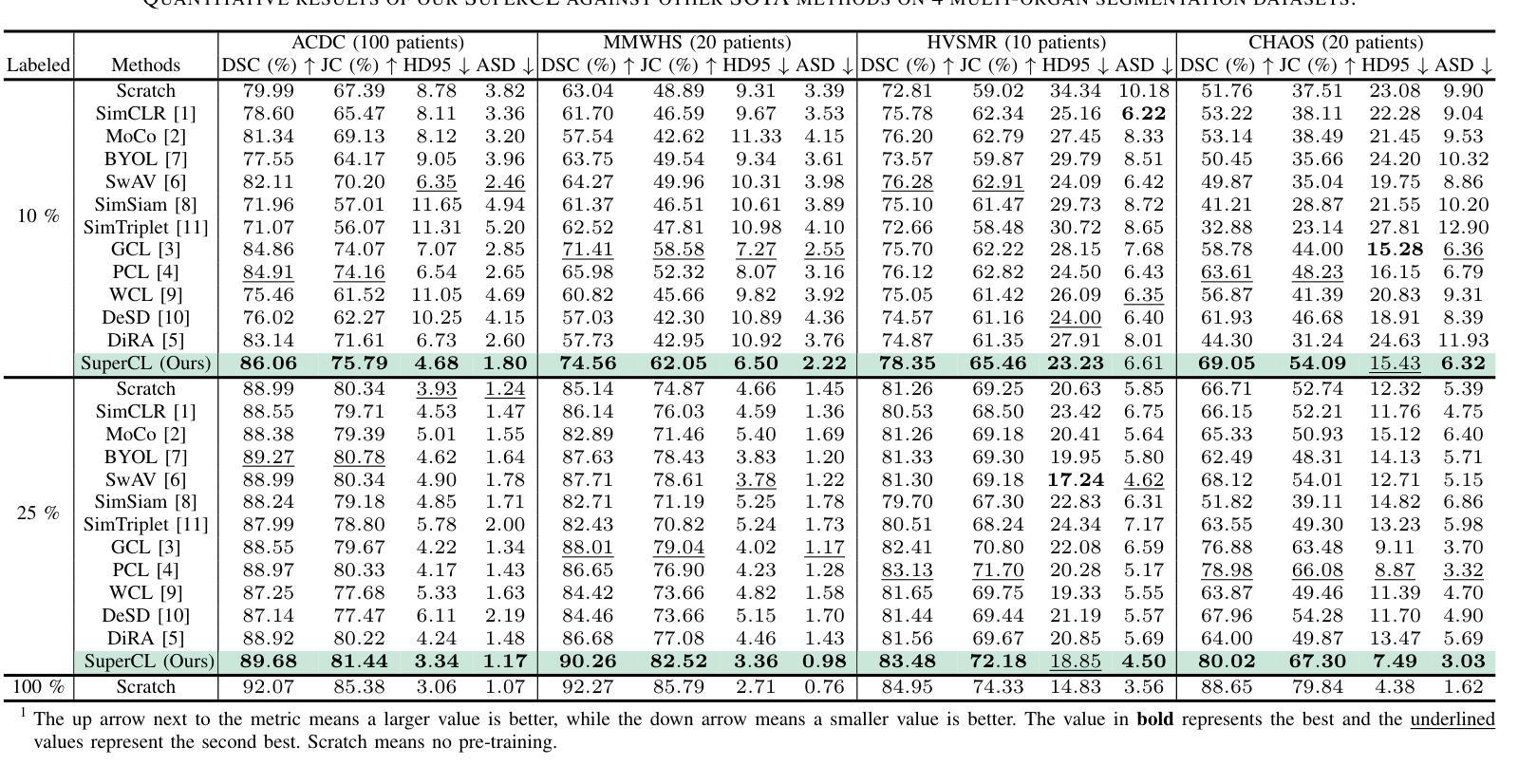