⚠️ 以下所有内容总结都来自于 大语言模型的能力,如有错误,仅供参考,谨慎使用
🔴 请注意:千万不要用于严肃的学术场景,只能用于论文阅读前的初筛!
💗 如果您觉得我们的项目对您有帮助 ChatPaperFree ,还请您给我们一些鼓励!⭐️ HuggingFace免费体验
2025-04-23 更新
DreamID: High-Fidelity and Fast diffusion-based Face Swapping via Triplet ID Group Learning
Authors:Fulong Ye, Miao Hua, Pengze Zhang, Xinghui Li, Qichao Sun, Songtao Zhao, Qian He, Xinglong Wu
In this paper, we introduce DreamID, a diffusion-based face swapping model that achieves high levels of ID similarity, attribute preservation, image fidelity, and fast inference speed. Unlike the typical face swapping training process, which often relies on implicit supervision and struggles to achieve satisfactory results. DreamID establishes explicit supervision for face swapping by constructing Triplet ID Group data, significantly enhancing identity similarity and attribute preservation. The iterative nature of diffusion models poses challenges for utilizing efficient image-space loss functions, as performing time-consuming multi-step sampling to obtain the generated image during training is impractical. To address this issue, we leverage the accelerated diffusion model SD Turbo, reducing the inference steps to a single iteration, enabling efficient pixel-level end-to-end training with explicit Triplet ID Group supervision. Additionally, we propose an improved diffusion-based model architecture comprising SwapNet, FaceNet, and ID Adapter. This robust architecture fully unlocks the power of the Triplet ID Group explicit supervision. Finally, to further extend our method, we explicitly modify the Triplet ID Group data during training to fine-tune and preserve specific attributes, such as glasses and face shape. Extensive experiments demonstrate that DreamID outperforms state-of-the-art methods in terms of identity similarity, pose and expression preservation, and image fidelity. Overall, DreamID achieves high-quality face swapping results at 512*512 resolution in just 0.6 seconds and performs exceptionally well in challenging scenarios such as complex lighting, large angles, and occlusions.
本文介绍了DreamID,这是一种基于扩散的面貌替换模型,实现了高水平的身份相似性、属性保留、图像保真度和快速推理速度。不同于典型的面貌替换训练过程,通常依赖于隐式监督并难以取得令人满意的结果。DreamID通过构建Triplet ID Group数据建立显式监督,显著提高了身份相似性和属性保留。扩散模型的迭代性质给利用高效的图像空间损失函数带来了挑战,因为在训练期间进行耗时的多步采样来获得生成图像是不切实际的。为了解决这个问题,我们利用了加速扩散模型SD Turbo,将推理步骤减少到单次迭代,能够在显式Triplet ID Group监督下进行高效的像素级端到端训练。此外,我们提出了一种改进的基于扩散的模型架构,包括SwapNet、FaceNet和ID适配器。这种稳健的架构充分释放了Triplet ID Group显式监督的威力。最后,为了进一步完善我们的方法,我们在训练过程中显式地修改Triplet ID Group数据,以微调并保留特定属性,如眼镜和脸型。大量实验表明,DreamID在身份相似性、姿势和表情保留以及图像保真度方面优于最先进的方法。总的来说,DreamID在512*512分辨率下实现了高质量的面貌替换结果,仅需0.6秒,并在复杂光照、大角度和遮挡等挑战场景下表现尤为出色。
论文及项目相关链接
摘要
本文介绍了DreamID,一种基于扩散的面貌换脸模型,实现了高水平的身份相似性、属性保留、图像保真度和快速推理速度。通过构建Triplet ID Group数据实现显式监督,显著提高身份相似性和属性保留性。为解决扩散模型的迭代性质带来的挑战,采用加速扩散模型SD Turbo,将推理步骤减少到单次迭代,实现高效的像素级端到端训练。此外,提出改进的基于扩散的模型架构,包括SwapNet、FaceNet和ID Adapter,充分利用Triplet ID Group的显式监督。最后,通过训练时显式修改Triplet ID Group数据,对特定属性进行微调保留,如眼镜和脸型。实验表明,DreamID在身份相似性、姿势和表情保留、图像保真度等方面优于现有技术。总体而言,DreamID在512*512分辨率下实现高质量的面貌换脸结果,仅需0.6秒,并在复杂光照、大角度和遮挡等挑战场景中表现优异。
关键见解
- DreamID是一个基于扩散的面貌换脸模型,实现了高水平的身份相似性、属性保留和图像保真度。
- 通过构建Triplet ID Group数据实现显式监督,提高身份相似性和属性保留。
- 采用加速扩散模型SD Turbo,实现高效的像素级端到端训练。
- 改进的基于扩散的模型架构包括SwapNet、FaceNet和ID Adapter,充分利用显式监督。
- 通过训练时修改Triplet ID Group数据,可以微调并保留特定属性,如眼镜和脸型。
- DreamID在多项实验中表现出色,尤其在身份相似性、图像质量和推理速度方面。
点此查看论文截图
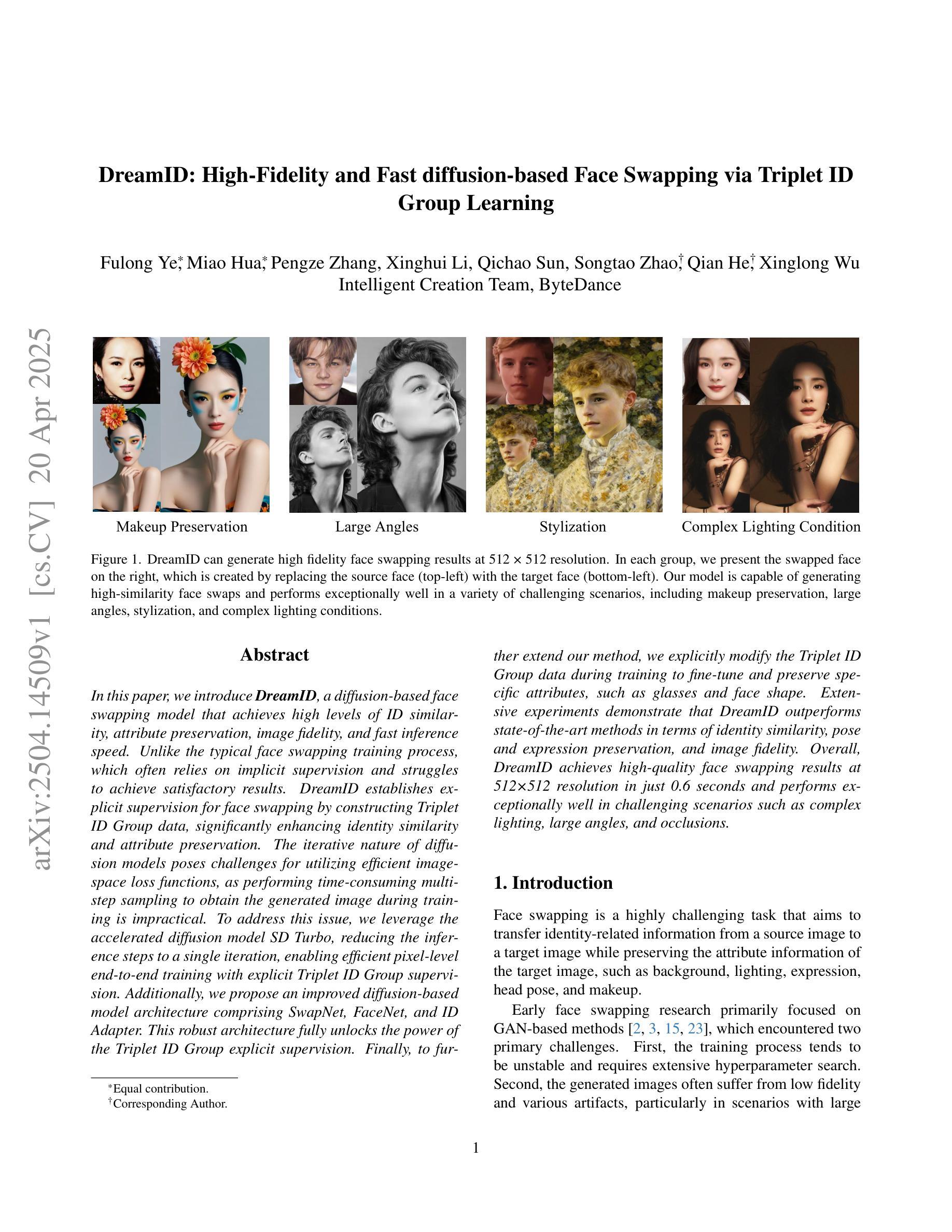
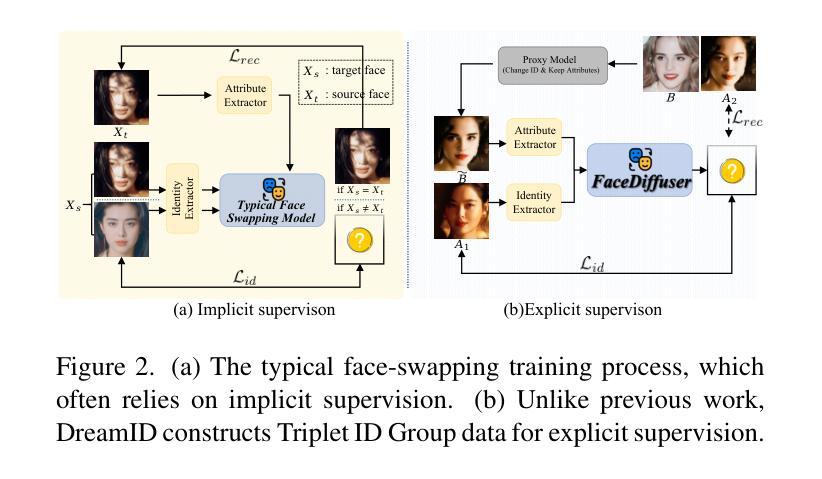
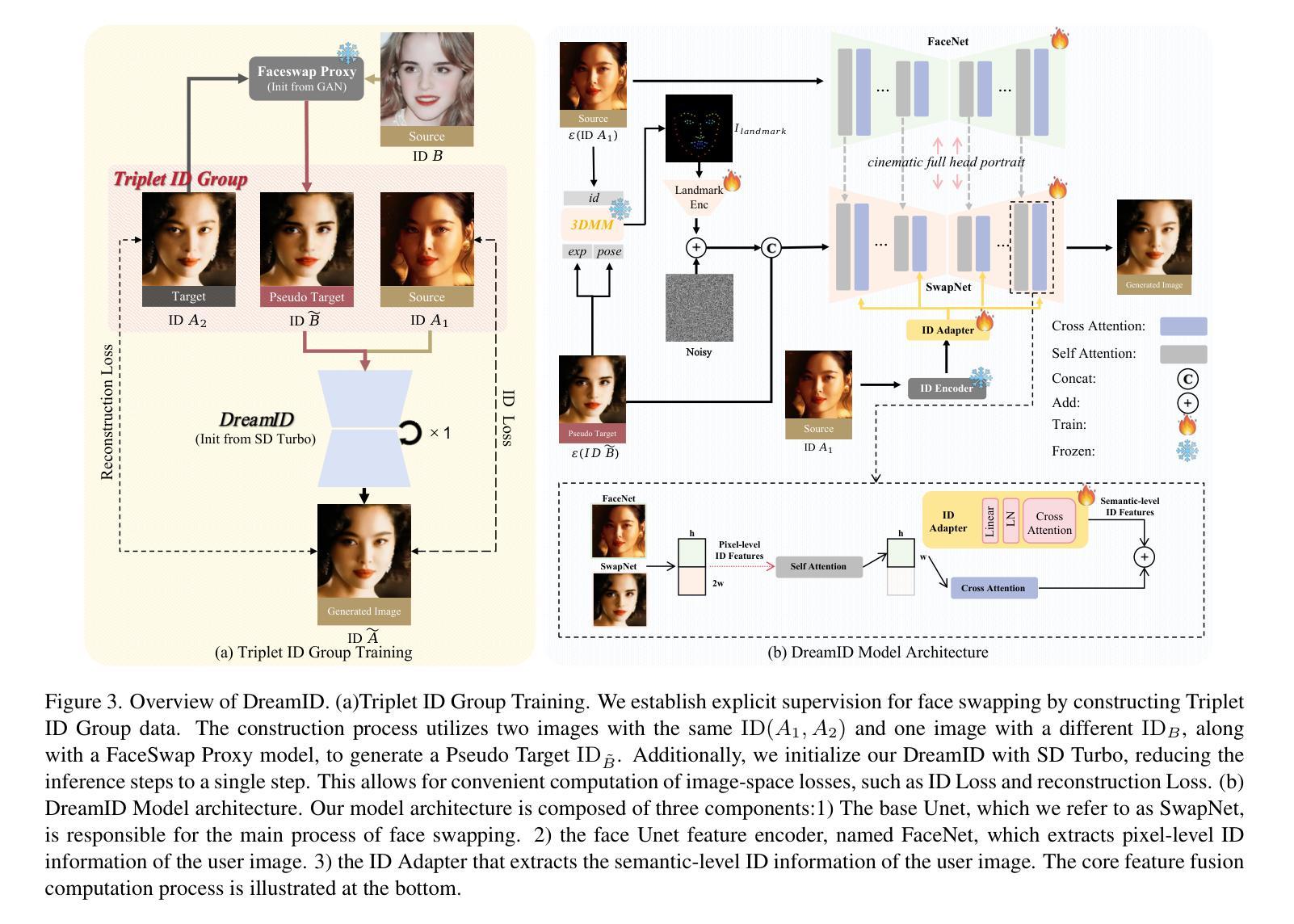
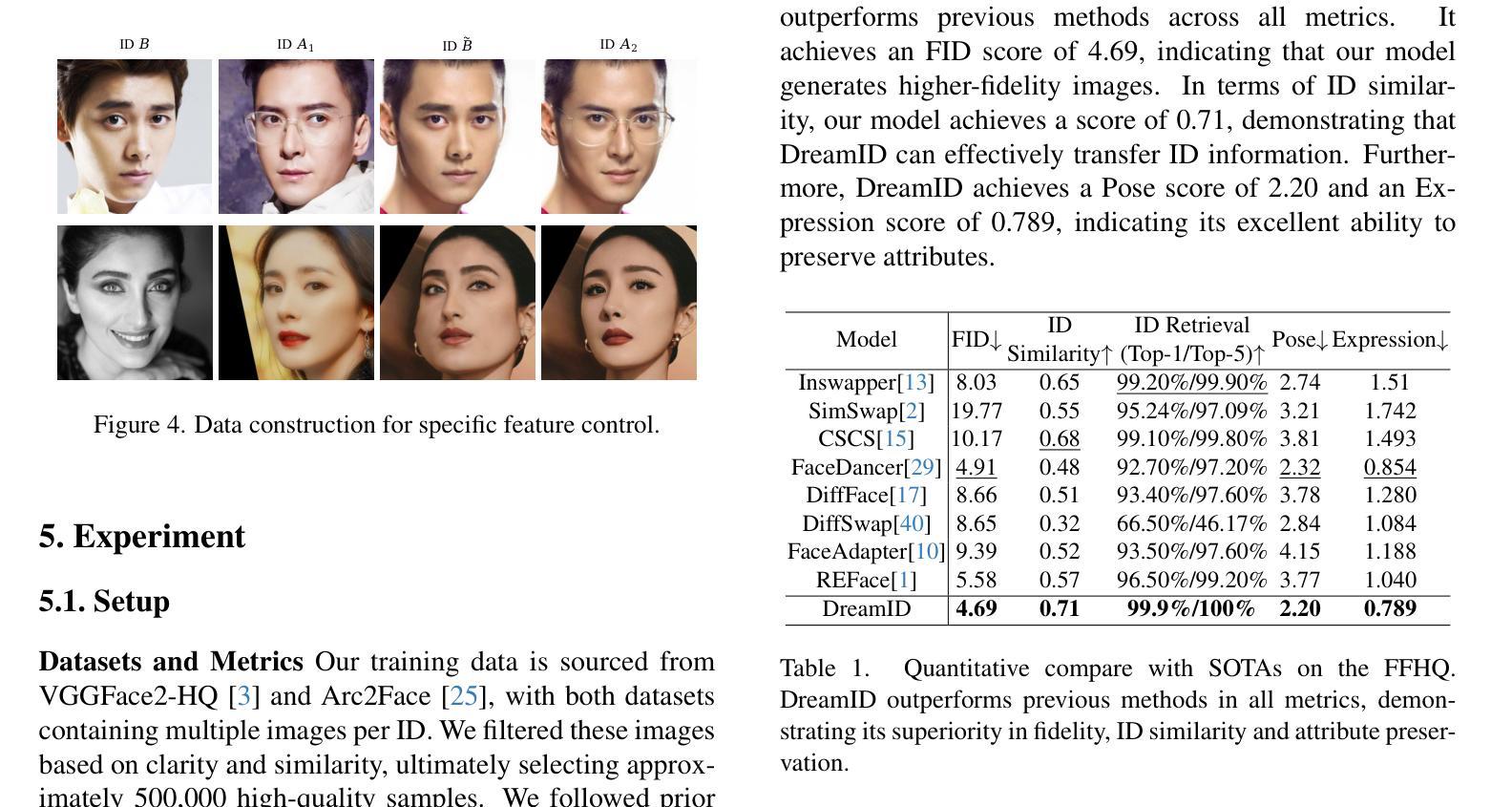
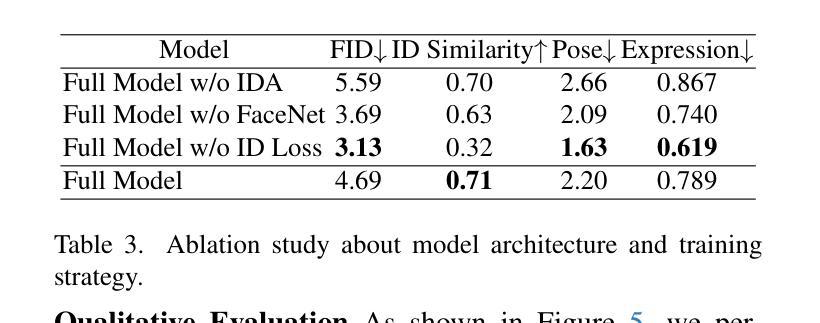