⚠️ 以下所有内容总结都来自于 大语言模型的能力,如有错误,仅供参考,谨慎使用
🔴 请注意:千万不要用于严肃的学术场景,只能用于论文阅读前的初筛!
💗 如果您觉得我们的项目对您有帮助 ChatPaperFree ,还请您给我们一些鼓励!⭐️ HuggingFace免费体验
2025-04-23 更新
GIFDL: Generated Image Fluctuation Distortion Learning for Enhancing Steganographic Security
Authors:Xiangkun Wang, Kejiang Chen, Yuang Qi, Ruiheng Liu, Weiming Zhang, Nenghai Yu
Minimum distortion steganography is currently the mainstream method for modification-based steganography. A key issue in this method is how to define steganographic distortion. With the rapid development of deep learning technology, the definition of distortion has evolved from manual design to deep learning design. Concurrently, rapid advancements in image generation have made generated images viable as cover media. However, existing distortion design methods based on machine learning do not fully leverage the advantages of generated cover media, resulting in suboptimal security performance. To address this issue, we propose GIFDL (Generated Image Fluctuation Distortion Learning), a steganographic distortion learning method based on the fluctuations in generated images. Inspired by the idea of natural steganography, we take a series of highly similar fluctuation images as the input to the steganographic distortion generator and introduce a new GAN training strategy to disguise stego images as fluctuation images. Experimental results demonstrate that GIFDL, compared with state-of-the-art GAN-based distortion learning methods, exhibits superior resistance to steganalysis, increasing the detection error rates by an average of 3.30% across three steganalyzers.
最小失真隐写术是当前基于修改的隐写术的主流方法。该方法的一个关键问题是如何定义隐写失真。随着深度学习技术的飞速发展,失真的定义已经从手动设计演变为深度学习设计。同时,图像生成的快速发展使得生成的图像可以作为载体媒体。然而,现有的基于机器学习的失真设计方法并没有充分利用生成的载体媒体优势,导致安全性能不佳。为了解决这一问题,我们提出了基于生成图像波动失真的隐写失真学习方法GIFDL(Generated Image Fluctuation Distortion Learning)。我们受到自然隐写的启发,将一系列高度相似的波动图像作为隐写失真生成器的输入,并引入了一种新的GAN训练策略,将隐写图像伪装成波动图像。实验结果表明,与最新的基于GAN的失真学习方法相比,GIFDL对隐写分析具有更强的抵抗力,在三个隐写分析器上的检测错误率平均提高了3.30%。
论文及项目相关链接
PDF Accepted by IEEE TIFS
Summary
基于深度学习和图像生成技术的迅速发展,当前主流的最小失真隐写术面临新的挑战。为解决现有隐写术在利用生成图像的优势上的不足,提出一种基于生成图像波动失真的隐写术(GIFDL)。GIFDL以一系列高度相似的波动图像为输入,通过新型GAN训练策略将隐藏信息的图像伪装成波动图像。实验表明,与当前先进的基于GAN的失真学习方法相比,GIFDL在抵抗隐写分析方面具有更强的优势,对三种隐写分析器的检测错误率平均提高了3.30%。
Key Takeaways
- 最小失真隐写术是当前的主流方法,但如何定义隐写失真是一个关键问题。
- 深度学习和图像生成技术的快速发展改变了隐写术中失真的定义方式。
- 现有基于机器学习的失真设计方法未能充分利用生成图像的优势,导致安全性能不足。
- 提出了一种基于生成图像波动失真的隐写术(GIFDL)。
- GIFDL利用一系列高度相似的波动图像作为输入,通过新型GAN训练策略伪装隐藏信息的图像。
- 实验结果表明,GIFDL相较于其他先进方法具有更强的抵抗隐写分析能力。
点此查看论文截图
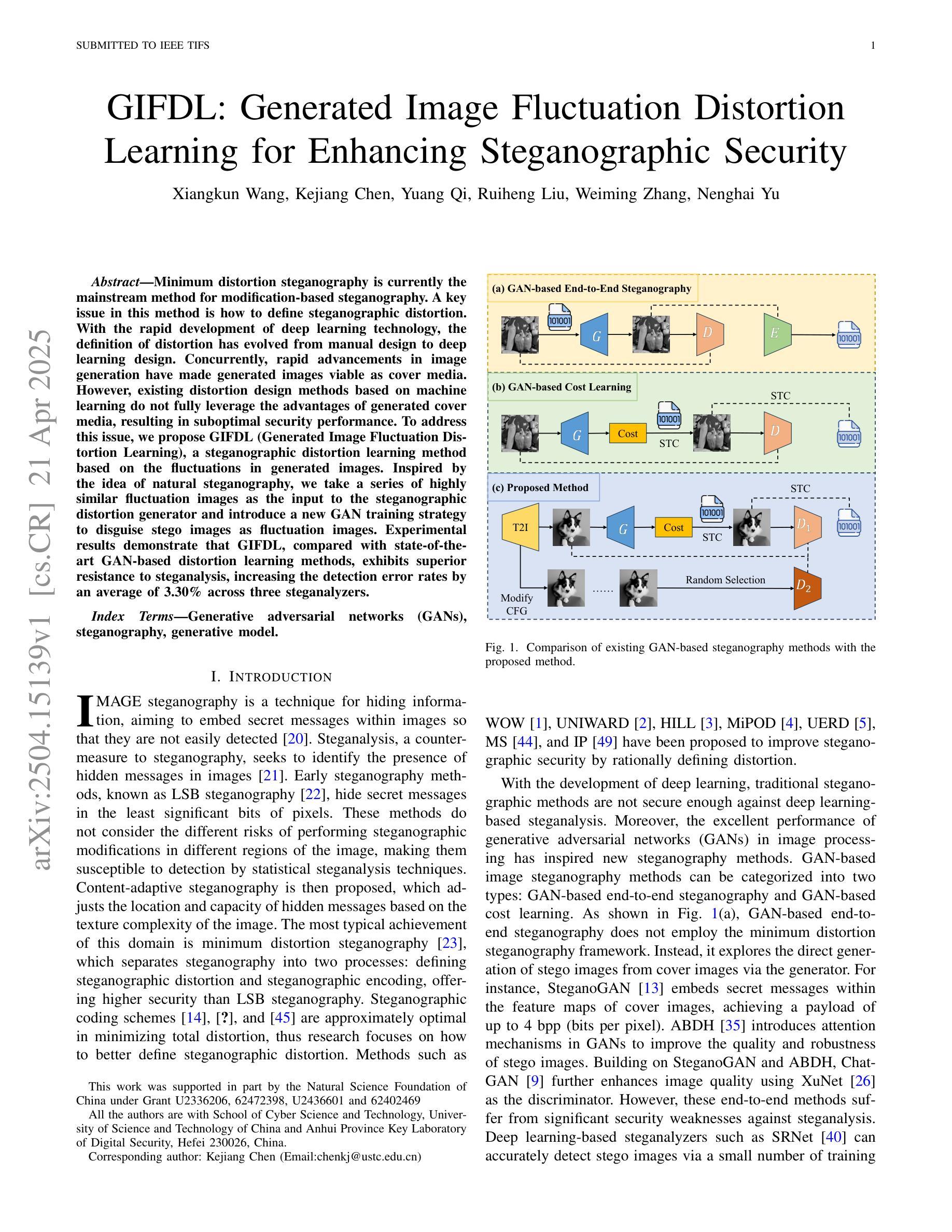
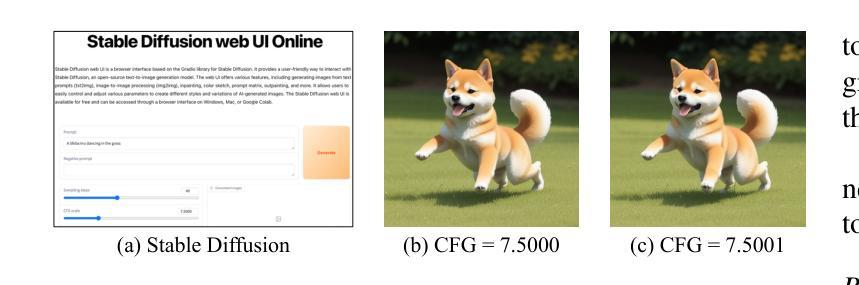
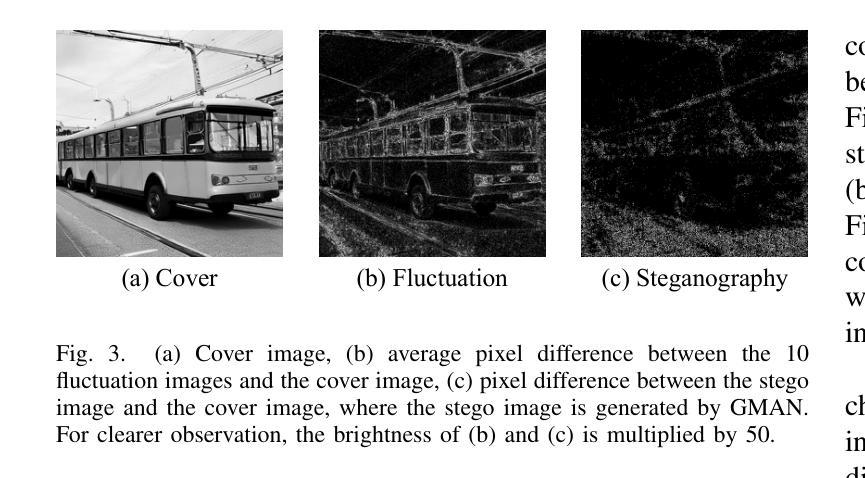
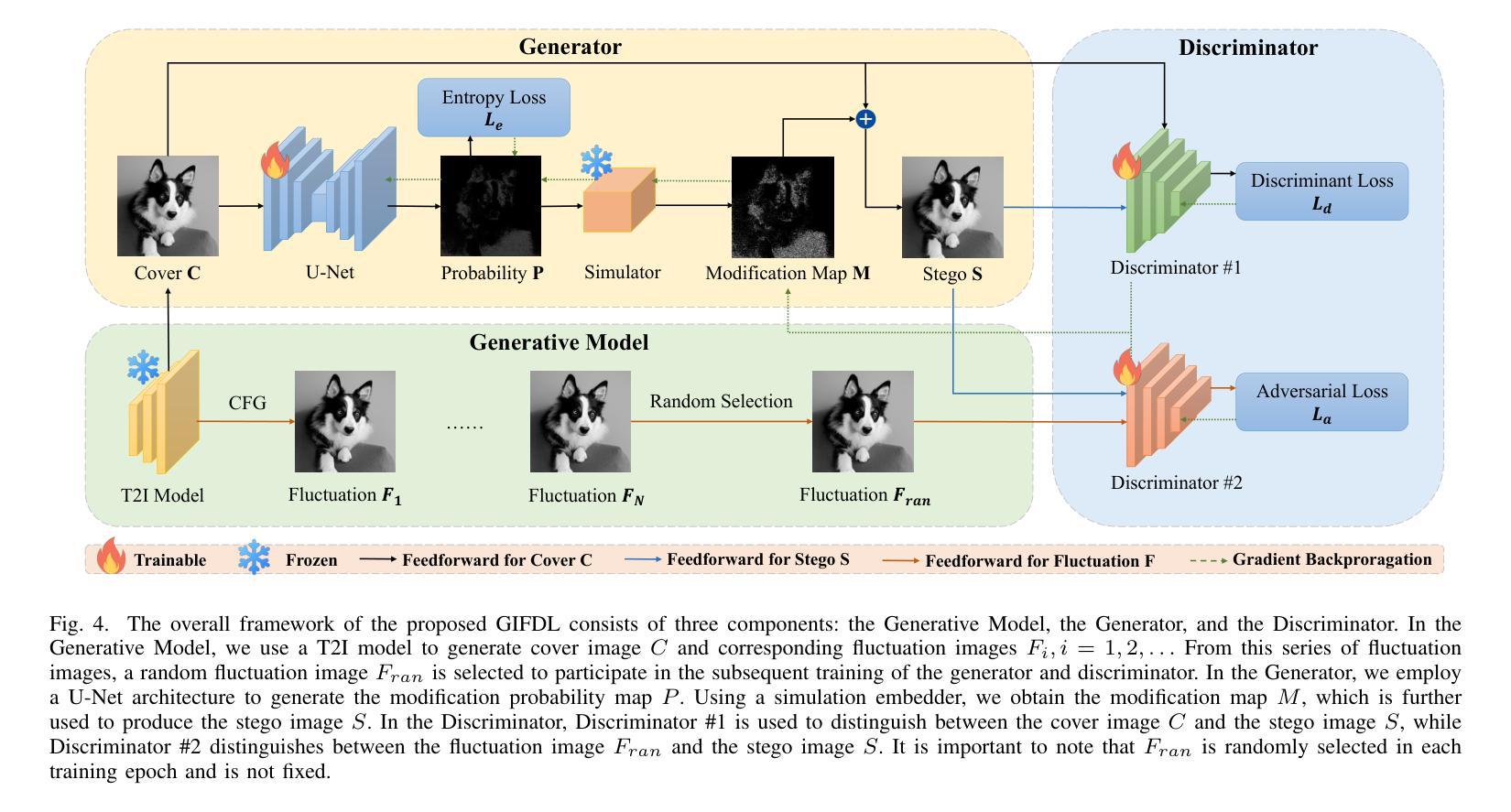
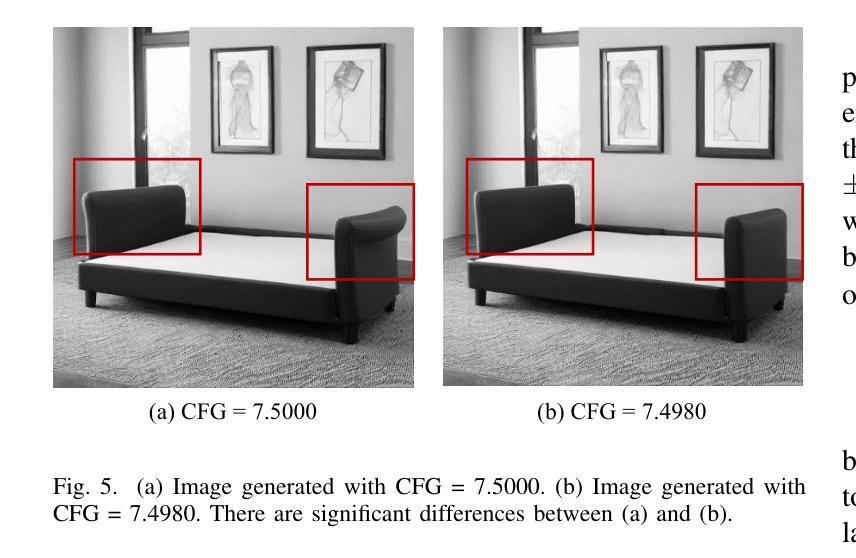
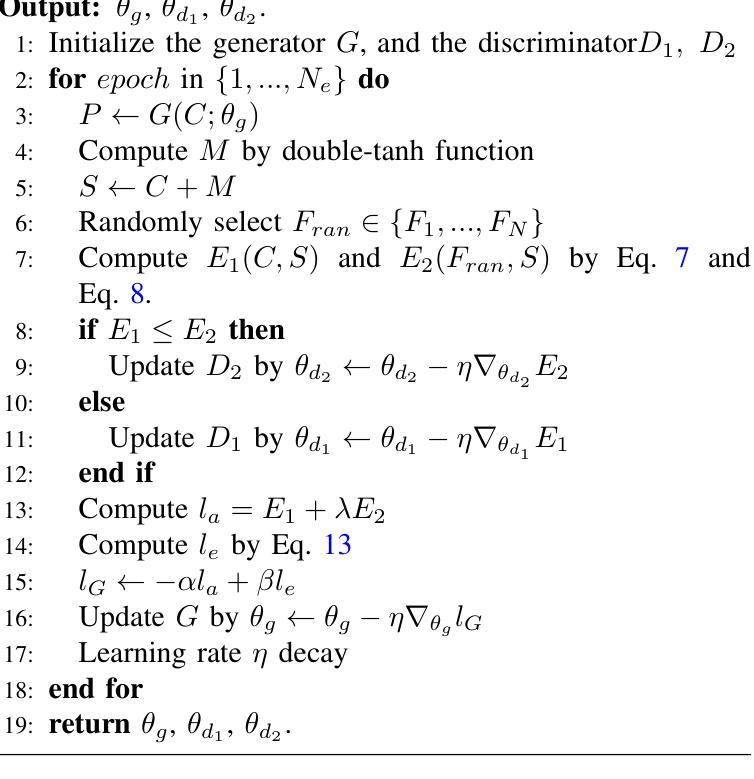
Comparative clinical evaluation of “memory-efficient” synthetic 3d generative adversarial networks (gan) head-to-head to state of art: results on computed tomography of the chest
Authors:Mahshid Shiri, Chandra Bortolotto, Alessandro Bruno, Alessio Consonni, Daniela Maria Grasso, Leonardo Brizzi, Daniele Loiacono, Lorenzo Preda
Generative Adversarial Networks (GANs) are increasingly used to generate synthetic medical images, addressing the critical shortage of annotated data for training Artificial Intelligence systems. This study introduces CRF-GAN, a novel memory-efficient GAN architecture that enhances structural consistency in 3D medical image synthesis. Integrating Conditional Random Fields within a two-step generation process allows CRF-GAN improving spatial coherence while maintaining high-resolution image quality. The model’s performance is evaluated against the state-of-the-art hierarchical (HA)-GAN model. Materials and Methods: We evaluate the performance of CRF-GAN against the HA-GAN model. The comparison between the two models was made through a quantitative evaluation, using FID and MMD metrics, and a qualitative evaluation, through a two-alternative forced choice (2AFC) test completed by a pool of 12 resident radiologists, to assess the realism of the generated images. Results: CRF-GAN outperformed HA-GAN with lower FID and MMD scores, indicating better image fidelity. The 2AFC test showed a significant preference for images generated by CRF-Gan over those generated by HA-GAN. Additionally, CRF-GAN demonstrated 9.34% lower memory usage and achieved up to 14.6% faster training speeds, offering substantial computational savings. Discussion: CRF-GAN model successfully generates high-resolution 3D medical images with non-inferior quality to conventional models, while being more memory-efficient and faster. The key objective was not only to lower the computational cost but also to reallocate the freed-up resources towards the creation of higher-resolution 3D imaging, which is still a critical factor limiting their direct clinical applicability. Moreover, unlike many previous studies, we combined qualitative and quantitative assessments to obtain a more holistic feedback on the model’s performance.
生成对抗网络(GANs)越来越多地被用于生成合成医学图像,以解决训练人工智能系统所需的标注数据严重短缺的问题。本研究介绍了CRF-GAN,这是一种新型的内存高效的GAN架构,它提高了3D医学图像合成中的结构一致性。在两步生成过程中融入条件随机场,使得CRF-GAN在提高空间连贯性的同时,还能保持高分辨率的图像质量。我们通过与最先进的层次化(HA)-GAN模型对比,评估了CRF-GAN的性能。材料与方法:我们评估了CRF-GAN与HA-GAN模型的性能。通过定量评估(使用FID和MMD指标)和定性评估(通过由12名住院放射科医生完成的两选一强制选择测试),对两种模型进行了比较,以评估生成图像的真实性。结果:CRF-GAN在FID和MMD得分上优于HA-GAN,表明图像保真度更高。两选一测试显示,CRF-GAN生成的图像明显优于HA-GAN生成的图像。此外,CRF-GAN的内存使用率低9.34%,训练速度提高了高达14.6%,大大节省了计算成本。讨论:CRF-GAN模型成功生成了高分辨率的3D医学图像,其质量与传统模型相当或更好,同时更节省内存、速度更快。主要目标不仅是为了降低计算成本,而且是为了将释放的资源重新分配给创建更高分辨率的3D成像,这仍然是限制其直接临床应用的关键因素。此外,与许多以前的研究不同,我们结合了定性和定量评估,以更全面地了解模型的性能。
论文及项目相关链接
PDF Accpeted to Journal of Imaging Informatics in Medicine
Summary
GAN网络被广泛应用于生成合成医学图像,以解决人工智能系统训练中的标注数据短缺问题。本研究提出了一种新型的内存高效GAN架构CRF-GAN,它提高了三维医学图像合成中的结构一致性。通过将条件随机场集成到两步生成过程中,CRF-GAN在提高空间一致性的同时保持了高分辨率图像质量。模型性能通过与最先进的层次化(HA)-GAN模型进行评估。CRF-GAN在图像保真度方面表现出优于HA-GAN的性能,并且在内存使用方面降低了9.34%,训练速度提高了高达14.6%。这意味着CRF-GAN在生成高质量的三维医学图像方面更加内存高效和快速。
Key Takeaways
- GANs在医学图像生成中的应用:用于解决标注数据短缺问题。
- CRF-GAN是一种新型的、内存高效的GAN架构:提高了三维医学图像合成中的结构一致性。
- CRF-GAN通过集成条件随机场提高了空间一致性和图像质量。
- CRF-GAN比HA-GAN模型性能更佳:在图像保真度方面表现更优秀。
- CRF-GAN具有更低的内存使用和更快的训练速度:降低了9.34%的内存使用,并提高了高达14.6%的训练速度。
- CRF-GAN生成的三维医学图像具有非劣质量:生成的图像质量不亚于传统模型。
- 结合了定性和定量评估:对模型性能进行了更全面的反馈评估。
点此查看论文截图
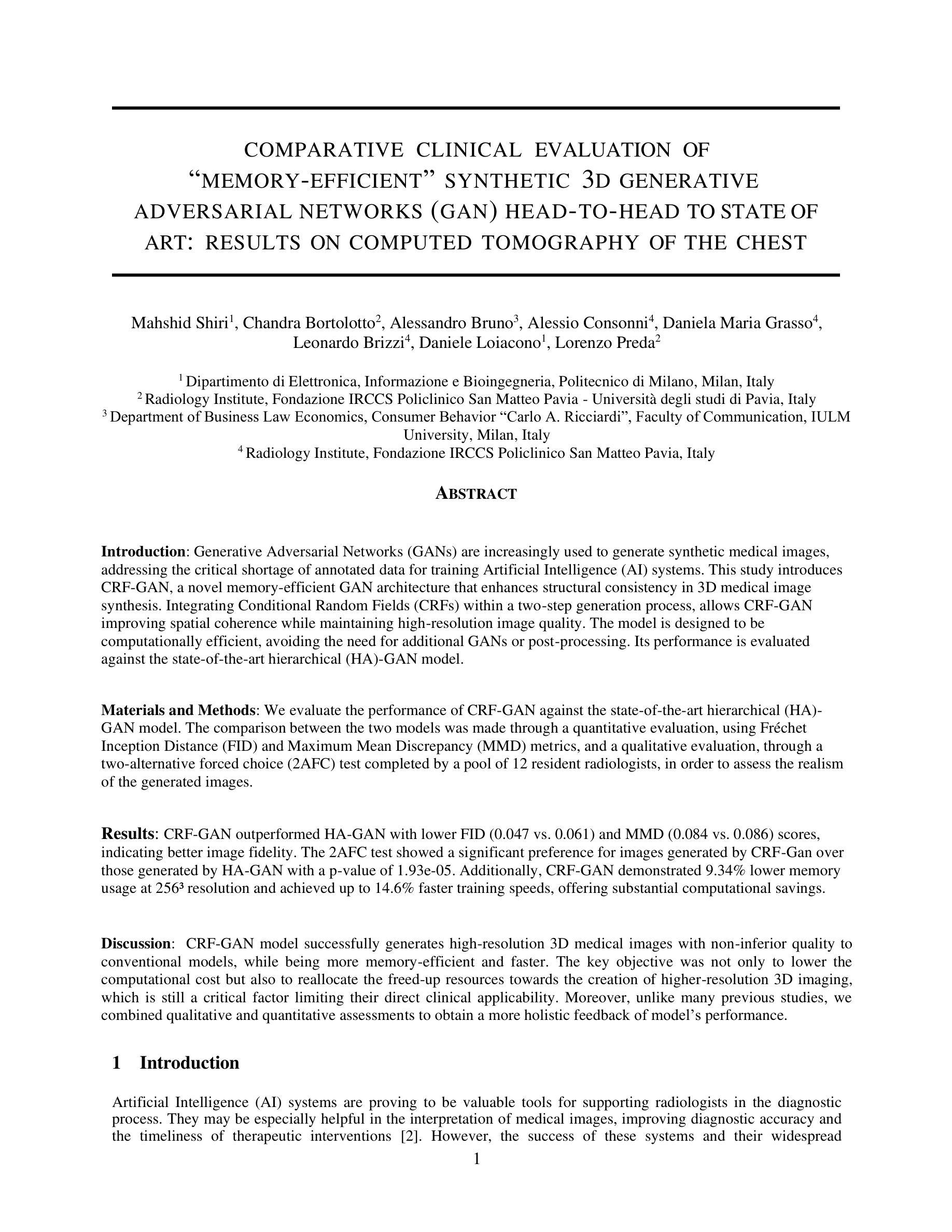
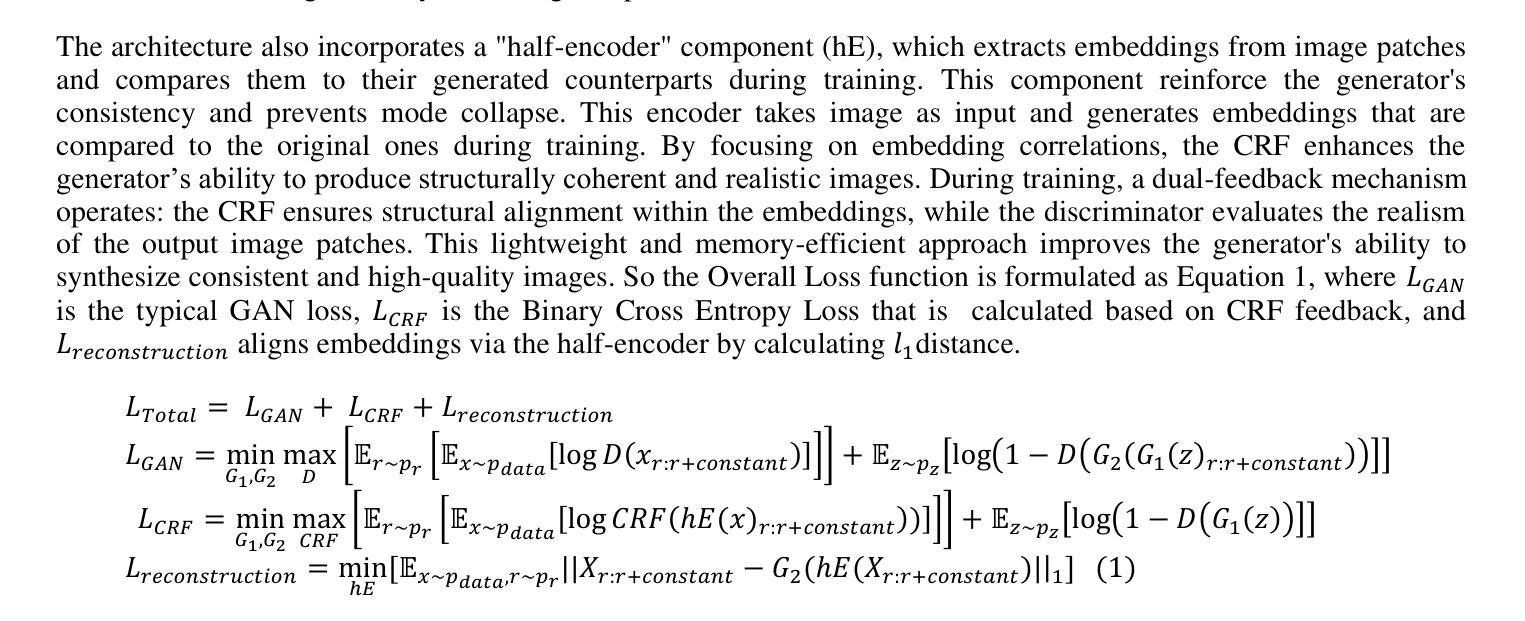
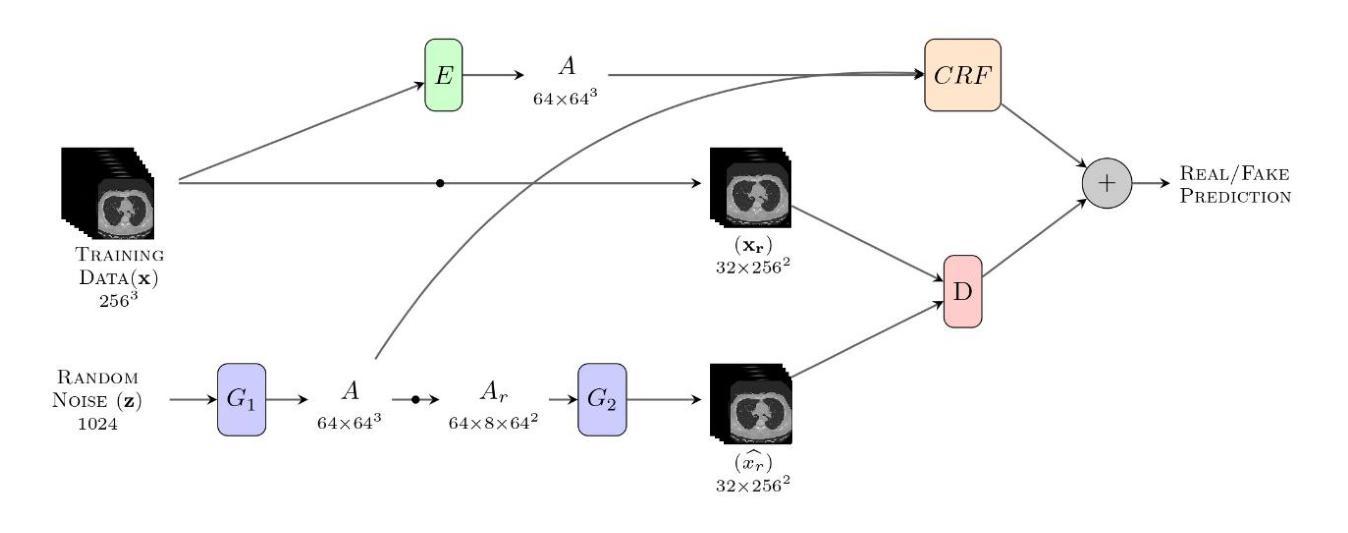
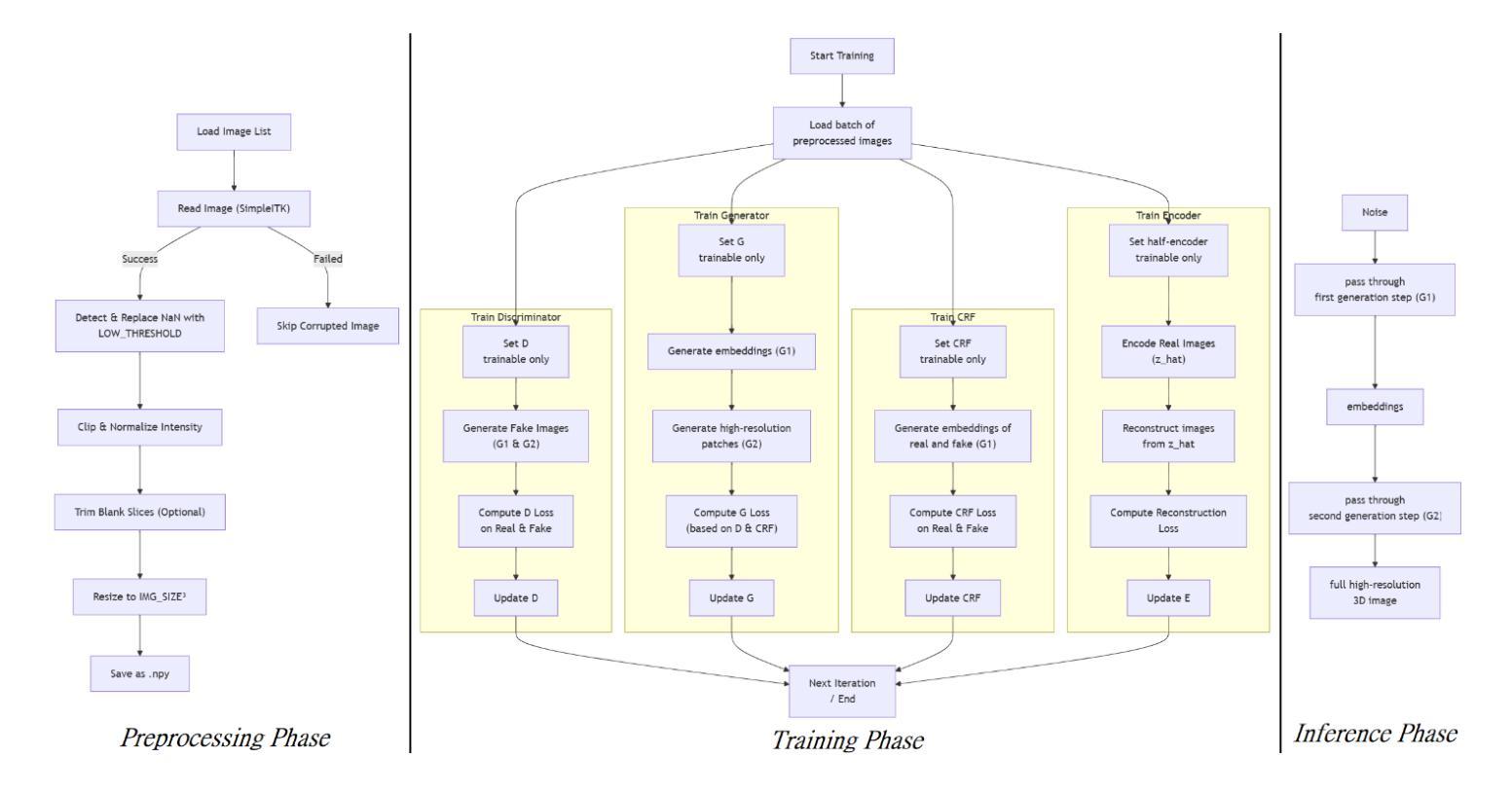