⚠️ 以下所有内容总结都来自于 大语言模型的能力,如有错误,仅供参考,谨慎使用
🔴 请注意:千万不要用于严肃的学术场景,只能用于论文阅读前的初筛!
💗 如果您觉得我们的项目对您有帮助 ChatPaperFree ,还请您给我们一些鼓励!⭐️ HuggingFace免费体验
2025-04-23 更新
ECViT: Efficient Convolutional Vision Transformer with Local-Attention and Multi-scale Stages
Authors:Zhoujie Qian
Vision Transformers (ViTs) have revolutionized computer vision by leveraging self-attention to model long-range dependencies. However, ViTs face challenges such as high computational costs due to the quadratic scaling of self-attention and the requirement of a large amount of training data. To address these limitations, we propose the Efficient Convolutional Vision Transformer (ECViT), a hybrid architecture that effectively combines the strengths of CNNs and Transformers. ECViT introduces inductive biases such as locality and translation invariance, inherent to Convolutional Neural Networks (CNNs) into the Transformer framework by extracting patches from low-level features and enhancing the encoder with convolutional operations. Additionally, it incorporates local-attention and a pyramid structure to enable efficient multi-scale feature extraction and representation. Experimental results demonstrate that ECViT achieves an optimal balance between performance and efficiency, outperforming state-of-the-art models on various image classification tasks while maintaining low computational and storage requirements. ECViT offers an ideal solution for applications that prioritize high efficiency without compromising performance.
视觉Transformer(ViT)通过利用自注意力机制对远程依赖关系进行建模,已经彻底改变了计算机视觉领域。然而,ViT面临着高计算成本和需要大量训练数据的挑战。为了解决这些局限性,我们提出了高效的卷积视觉Transformer(ECViT),这是一种有效地结合了CNN和Transformer优势的混合架构。ECViT通过将卷积神经网络(CNN)所固有的局部性和平移不变性等归纳偏见引入Transformer框架,通过从低级特征中提取补丁并增强编码器中的卷积运算来实现这一点。此外,它结合了局部注意力和金字塔结构,以实现高效的多尺度特征提取和表示。实验结果表明,ECViT在性能和效率之间达到了最佳平衡,在各种图像分类任务上的性能优于最新模型,同时保持了较低的计算和存储需求。对于优先考虑高效率而不牺牲性能的应用,ECViT提供了理想的解决方案。
论文及项目相关链接
Summary
Vision Transformers的革命性在于通过自我关注机制建模长距离依赖关系。但面临计算成本高和对大量训练数据的需要等挑战。为此,我们提出了Efficient Convolutional Vision Transformer(ECViT),这是一种结合了CNN和Transformer优势的混合架构。ECViT通过将卷积神经网络中的局部性和平移不变性等归纳偏见引入Transformer框架,通过从低级特征中提取补丁并增强编码器中的卷积运算来实现。此外,它结合了局部注意力和金字塔结构,实现了高效的多尺度特征提取和表示。实验结果表明,ECViT在性能和效率之间达到了最佳平衡,在各种图像分类任务上优于最新模型,同时保持较低的计算和存储要求。ECViT是追求高效率而不妥协性能的应用的理想解决方案。
Key Takeaways
- Vision Transformers (ViTs) 通过自我关注机制建模长距离依赖关系,在计算机视觉领域掀起革命。
- ViTs面临计算成本高和对大量训练数据的需求等挑战。
- ECViT是一种混合架构,结合了CNN和Transformer的优势。
- ECViT引入了卷积神经网络的归纳偏见,如局部性和平移不变性。
- ECViT通过结合局部注意力和金字塔结构,实现了高效的多尺度特征提取和表示。
- ECViT在图像分类任务上表现优异,实现了性能和效率之间的最佳平衡。
点此查看论文截图
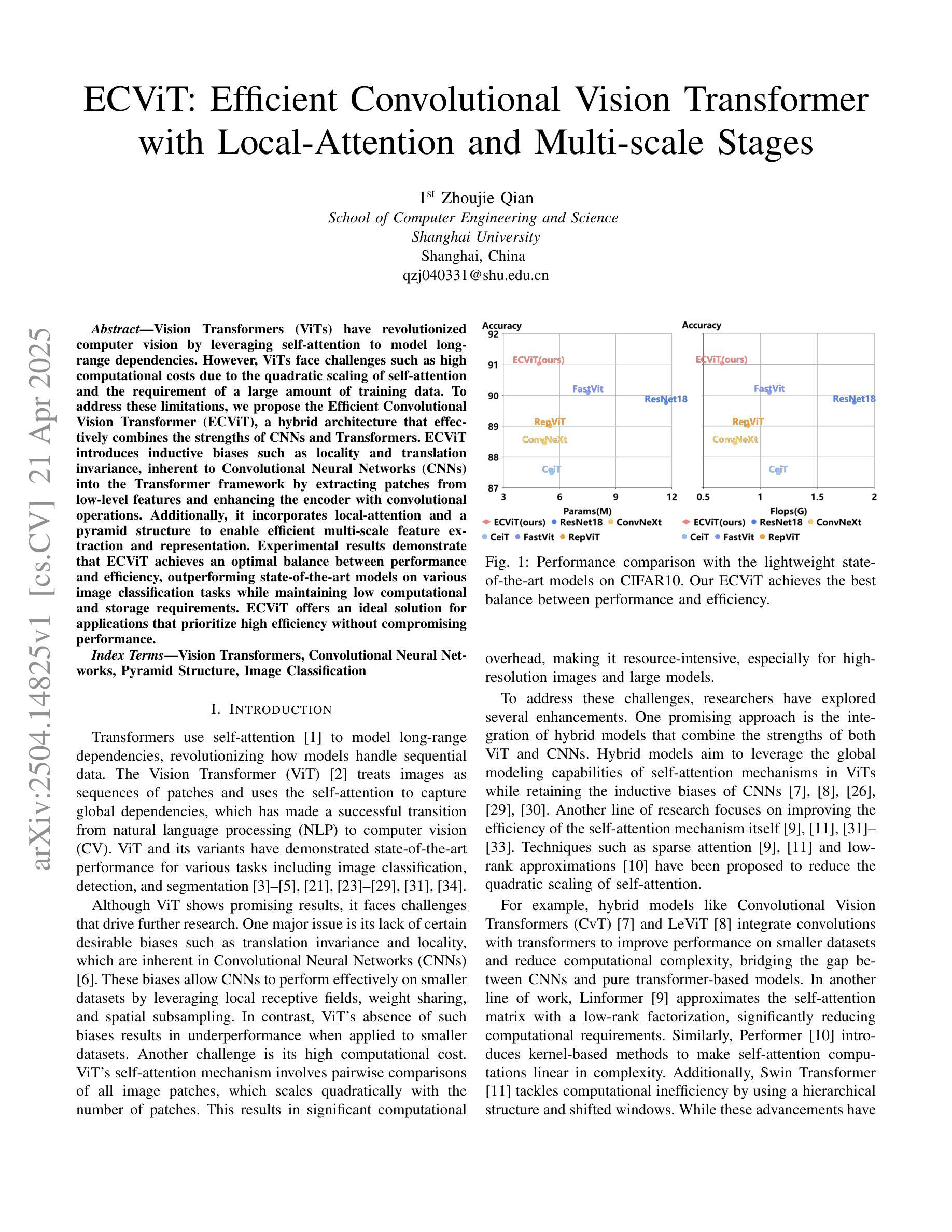
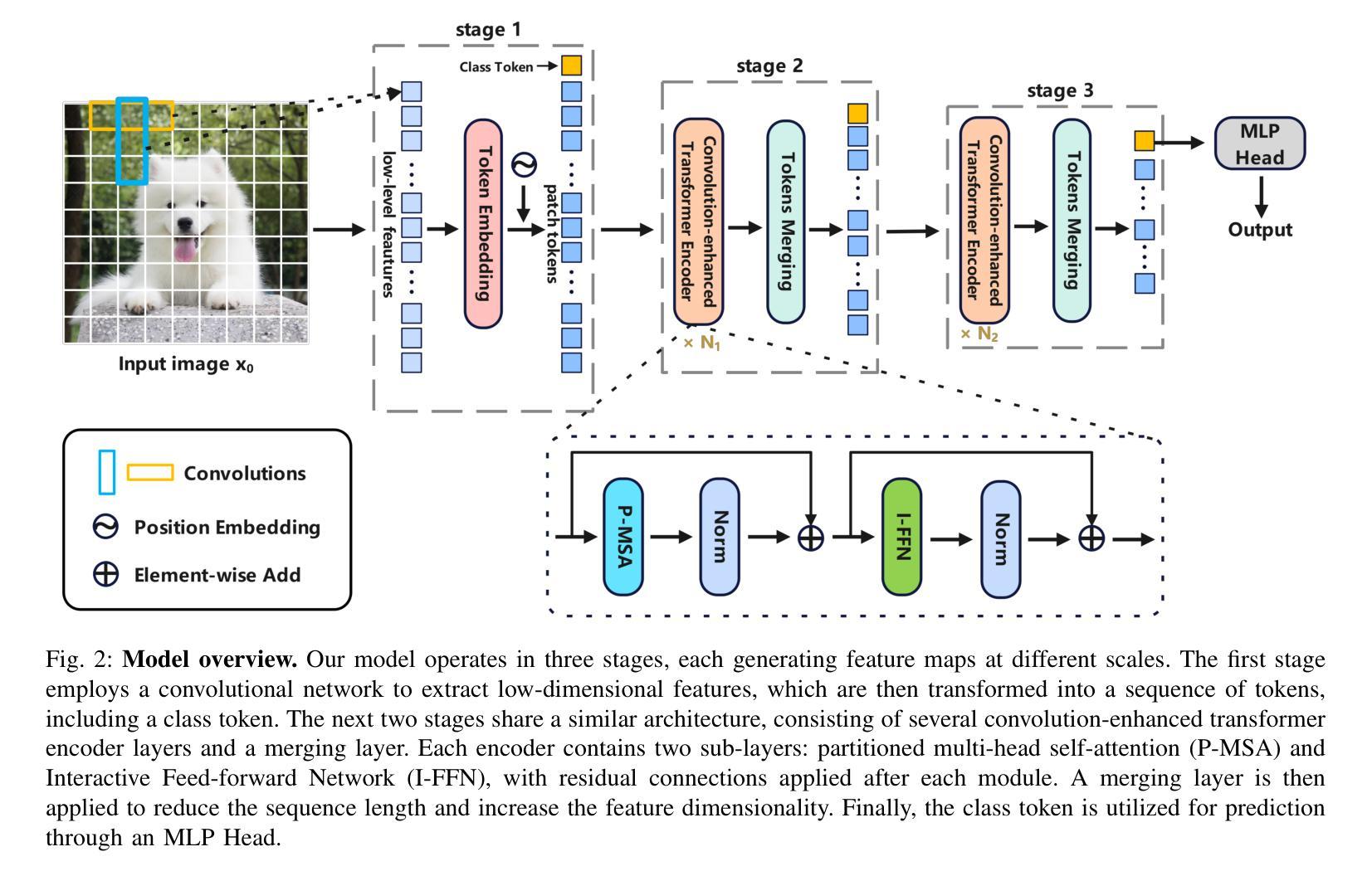
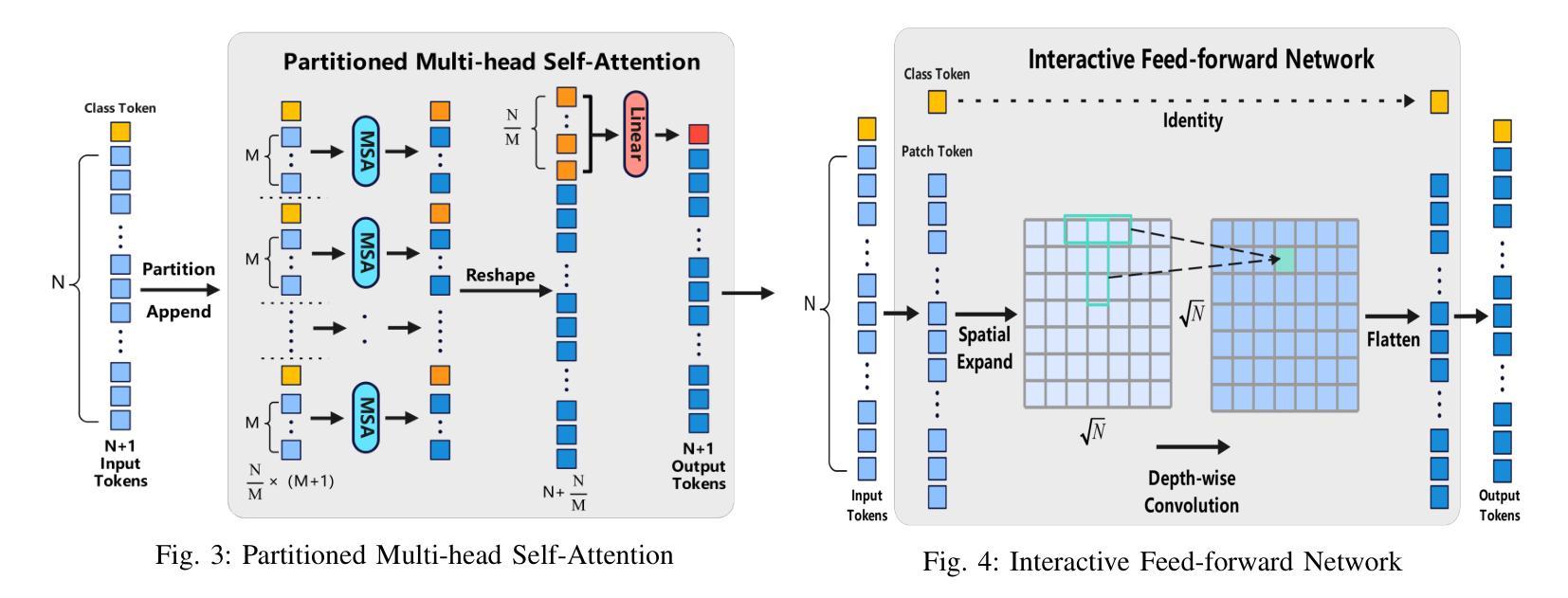
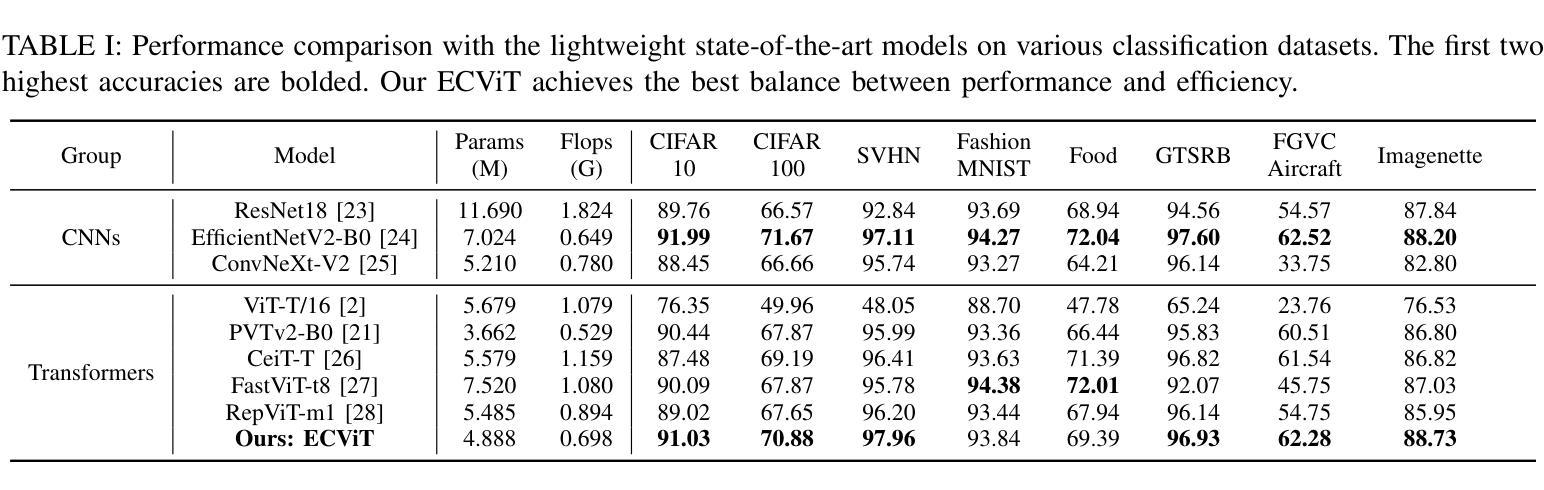
Using street view imagery and deep generative modeling for estimating the health of urban forests
Authors:Akshit Gupta, Remko Uijlenhoet
Healthy urban forests comprising of diverse trees and shrubs play a crucial role in mitigating climate change. They provide several key advantages such as providing shade for energy conservation, and intercepting rainfall to reduce flood runoff and soil erosion. Traditional approaches for monitoring the health of urban forests require instrumented inspection techniques, often involving a high amount of human labor and subjective evaluations. As a result, they are not scalable for cities which lack extensive resources. Recent approaches involving multi-spectral imaging data based on terrestrial sensing and satellites, are constrained respectively with challenges related to dedicated deployments and limited spatial resolutions. In this work, we propose an alternative approach for monitoring the urban forests using simplified inputs: street view imagery, tree inventory data and meteorological conditions. We propose to use image-to-image translation networks to estimate two urban forest health parameters, namely, NDVI and CTD. Finally, we aim to compare the generated results with ground truth data using an onsite campaign utilizing handheld multi-spectral and thermal imaging sensors. With the advent and expansion of street view imagery platforms such as Google Street View and Mapillary, this approach should enable effective management of urban forests for the authorities in cities at scale.
包含多种树木和灌木的城市森林在缓解气候变化方面发挥着至关重要的作用。它们提供了几个关键优势,例如提供遮荫以节能,以及拦截降雨以减少径流和土壤侵蚀。传统监测城市森林健康的方法需要仪器检测手段,通常涉及大量人力和主观评估。因此,对于缺乏大量资源的城市来说,它们并不具备可扩展性。涉及基于地面感知和卫星的多光谱成像数据的最新方法,分别面临有关专用部署和有限空间分辨率的挑战。在这项工作中,我们提出了一种使用简化输入来监测城市森林的替代方法:街道视图图像、树木库存数据和气象条件。我们建议使用图像到图像的翻译网络来估算两个城市森林健康参数,即归一化差异植被指数(NDVI)和树冠密度(CTD)。最后,我们将通过现场活动使用手持式多光谱和热成像传感器与地面真实数据进行比较来评估生成的结果。随着谷歌街景和Mapillary等街景图像平台的出现和扩展,这种方法应该能够使城市当局在大规模范围内有效地管理城市森林。
论文及项目相关链接
PDF Accepted at ICLR 2025 Workshop
Summary
城市森林的健康对于缓解气候变化至关重要,其包含多样化的树木和灌木,能够提供遮荫、节能、拦截降雨以减少洪水径流和土壤侵蚀等优势。传统监测城市森林健康的方法需要大量的人力进行仪器检测和主观评估,不适用于资源有限的城市。本研究提出了一种基于街道图像、树木清单数据和气象条件的简化输入来监测城市森林的新方法,利用图像到图像的翻译网络估算城市森林健康参数NDVI和CTD,并与手持多光谱和热成像传感器的现场活动所得的实际数据进行对比验证。此研究方法的成功实践有助于城市管理部门更有效地管理城市森林。
Key Takeaways
- 城市森林具有缓解气候变化的重要作用,包括多样化的树木和灌木,能提供多种生态服务功能。
- 传统城市森林健康监测方法成本高且不可扩展。
- 本研究提出了一种基于街道图像、树木清单数据和气象条件的简化输入来监测城市森林的新方法。
- 利用图像到图像的翻译网络估算城市森林健康参数NDVI和CTD。
- 该方法利用街道视图影像平台(如Google Street View和Mapillary)进行实践。
- 现场活动所得的实际数据将用于验证估算结果的准确性。
点此查看论文截图
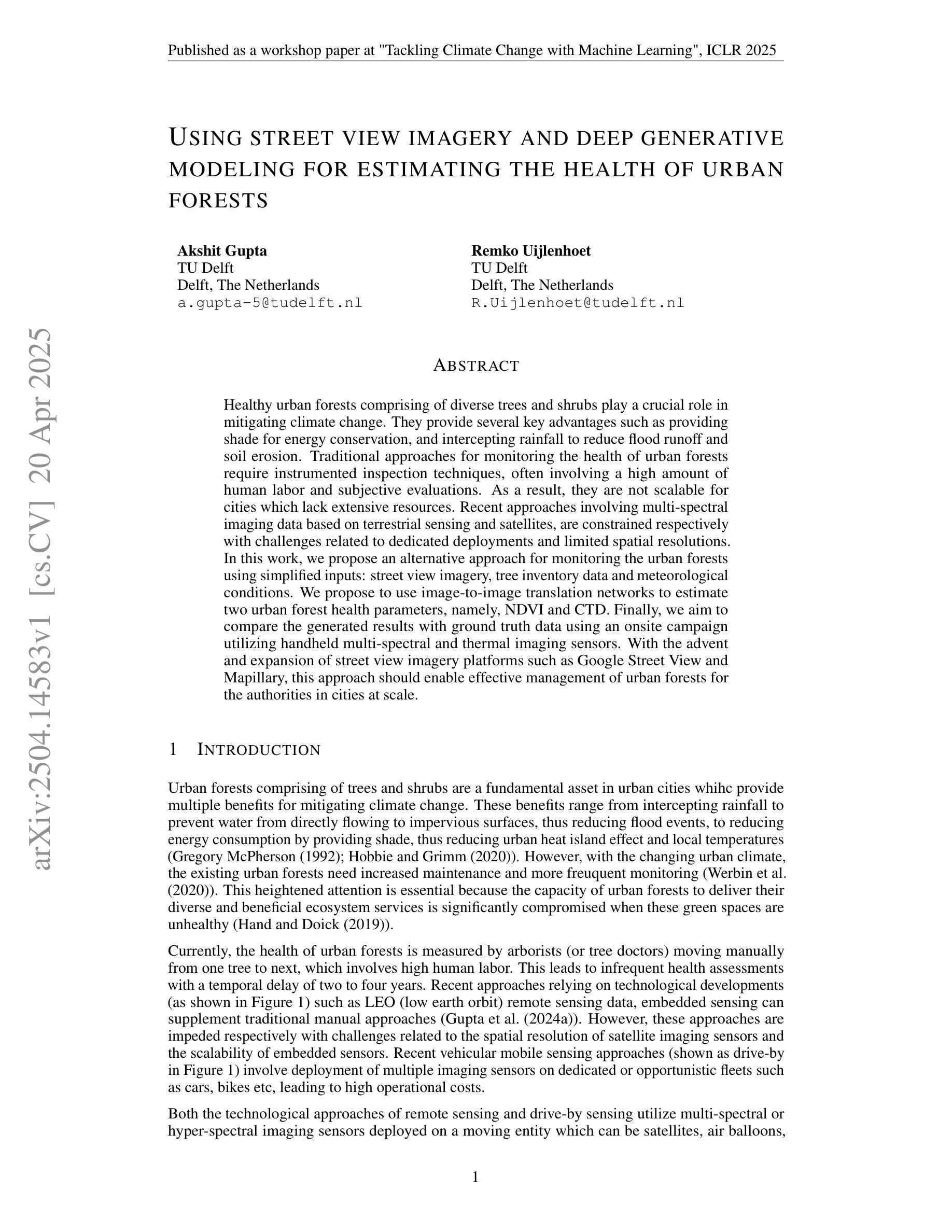
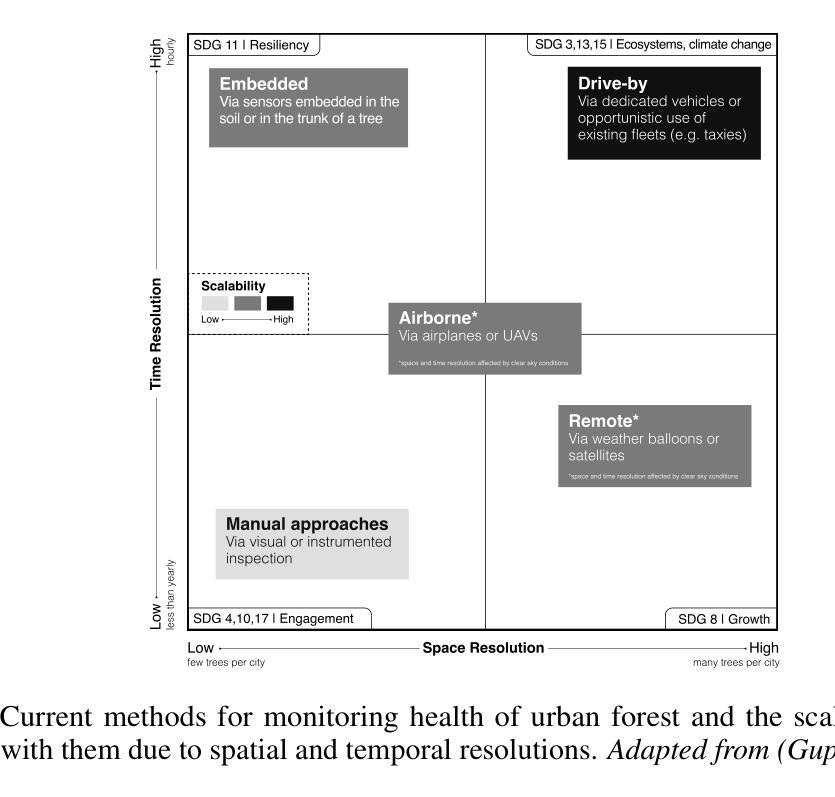
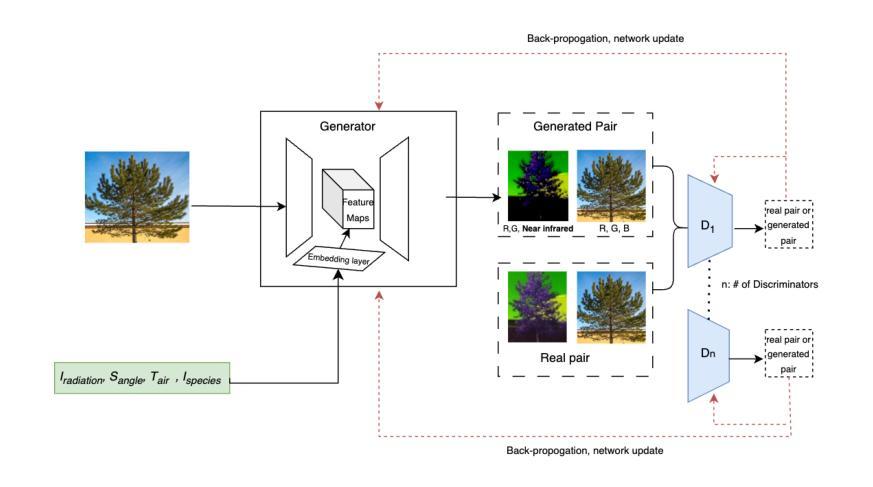
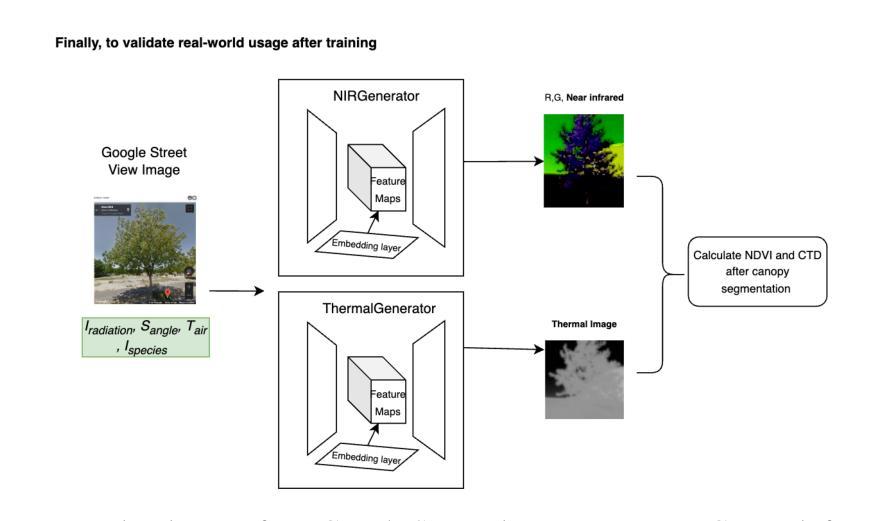
Assessing and Learning Alignment of Unimodal Vision and Language Models
Authors:Le Zhang, Qian Yang, Aishwarya Agrawal
How well are unimodal vision and language models aligned? Although prior work have approached answering this question, their assessment methods do not directly translate to how these models are used in practical vision-language tasks. In this paper, we propose a direct assessment method, inspired by linear probing, to assess vision-language alignment. We identify that the degree of alignment of the SSL vision models depends on their SSL training objective, and we find that the clustering quality of SSL representations has a stronger impact on alignment performance than their linear separability. Next, we introduce Swift Alignment of Image and Language (SAIL), a efficient transfer learning framework that aligns pretrained unimodal vision and language models for downstream vision-language tasks. Since SAIL leverages the strengths of pretrained unimodal models, it requires significantly fewer (6%) paired image-text data for the multimodal alignment compared to models like CLIP which are trained from scratch. SAIL training only requires a single A100 GPU, 5 hours of training and can accommodate a batch size up to 32,768. SAIL achieves 73.4% zero-shot accuracy on ImageNet (vs. CLIP’s 72.7%) and excels in zero-shot retrieval, complex reasoning, and semantic segmentation. Additionally, SAIL improves the language-compatibility of vision encoders that in turn enhance the performance of multimodal large language models. The entire codebase and model weights are open-source: https://lezhang7.github.io/sail.github.io/
单模态视觉和语言模型的对齐程度如何?尽管先前的工作已经尝试回答这个问题,但他们的评估方法并不能直接转化为这些模型在实际视觉语言任务中的使用方式。在本文中,我们提出了一种受线性探测启发的直接评估方法,以评估视觉语言对齐程度。我们发现SSL视觉模型的对齐程度取决于其SSL训练目标,并且我们发现SSL表示的聚类质量对对齐性能的影响强于其线性可分性。接下来,我们介绍了图像和语言快速对齐(SAIL)技术,这是一种高效的迁移学习框架,用于对齐预训练的单模态视觉和语言模型,以便进行下游视觉语言任务。由于SAIL利用了预训练单模态模型的优势,因此与从头开始训练的CLIP等模型相比,它在进行多模态对齐时所需的配对图像文本数据大大减少(仅需6%)。SAIL训练仅需一个A100 GPU,训练时间为5小时,并且可以容纳高达32,768的批次大小。SAIL在ImageNet上的零样本准确率达到了73.4%(相对于CLIP的72.7%),并且在零样本检索、复杂推理和语义分割方面表现出色。此外,SAIL提高了视觉编码器的语言兼容性,进而提高了多模态大型语言模型的性能。所有代码和模型权重均为开源:https://lezhang7.github.io/sail.github.io/。
论文及项目相关链接
PDF CVPR 2025 Highlight
Summary
本论文关注于评估视觉和语言模型的跨模态对齐程度。通过借鉴线性探测技术,提出了一种新的评估方法。研究发现,自监督学习(SSL)模型的视觉对齐程度取决于其训练目标,且聚类质量相较于线性可分性对模型对齐性能影响更大。此外,论文还介绍了Swift Alignment of Image and Language(SAIL)框架,该框架利用预训练的视觉和语言模型的优点,对于下游的视觉语言任务具有高效迁移学习能力。相较于从头开始训练的模型如CLIP,SAIL在配对图像文本数据需求方面显著减少,仅需要单张A100 GPU进行训练,时间也缩短至五小时,并能支持更大的批处理量。在图像分类、零样本检索、复杂推理和语义分割等任务上取得了优异性能,同时提升了视觉编码器的语言兼容性,增强了多模态大语言模型的性能。代码和模型权重均已开源。
Key Takeaways
- 论文提出了一种新的评估视觉和语言模型跨模态对齐程度的方法,该方法基于线性探测技术。
- SSL模型的视觉对齐程度受训练目标影响,其中聚类质量对模型对齐性能的影响大于线性可分性。
- 介绍了Swift Alignment of Image and Language(SAIL)框架,该框架能够高效迁移学习预训练的视觉和语言模型至下游的视觉语言任务。
- SAIL相较于其他从头开始训练的模型显著减少了配对图像文本数据需求,并且训练时间大幅缩短。
- SAIL支持更大的批处理量并在图像分类、零样本检索、复杂推理和语义分割等任务上表现出卓越性能。
- SAIL提升了视觉编码器的语言兼容性。
点此查看论文截图
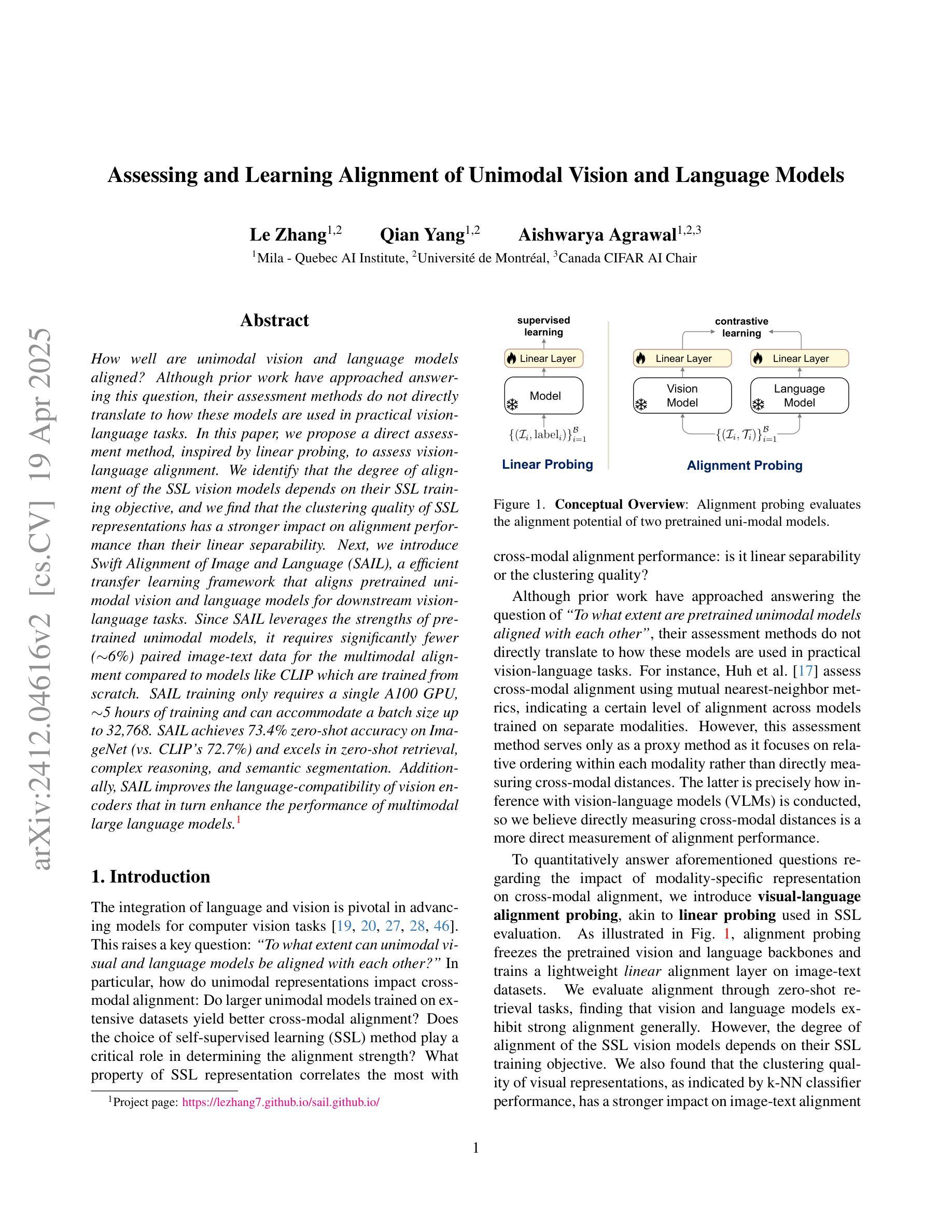
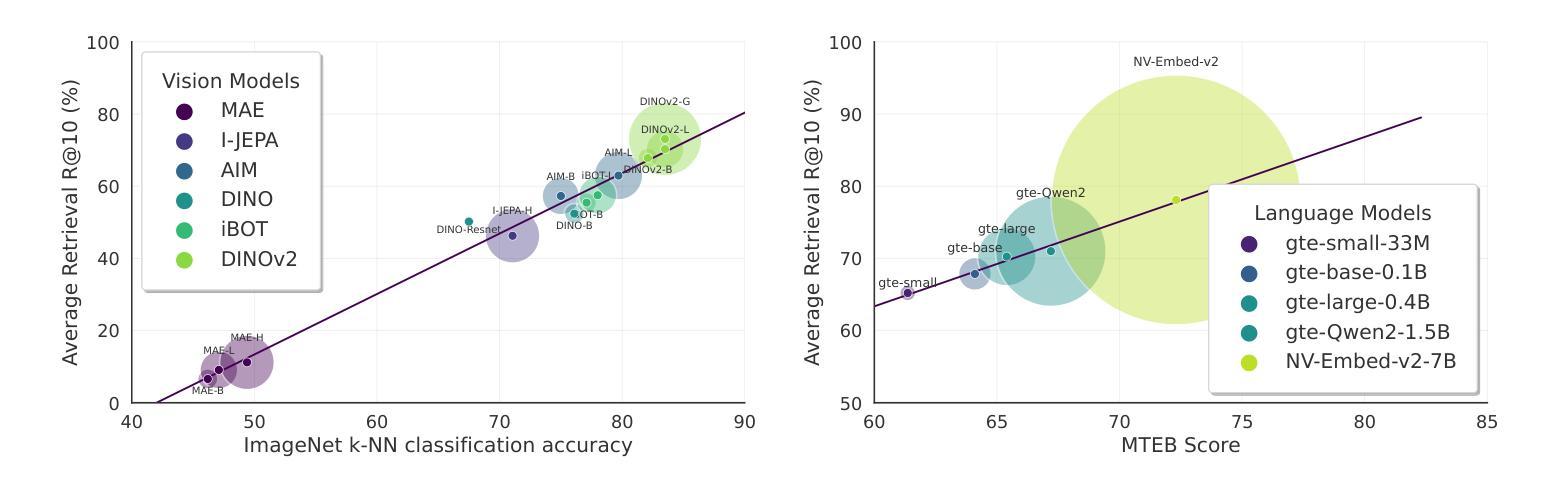
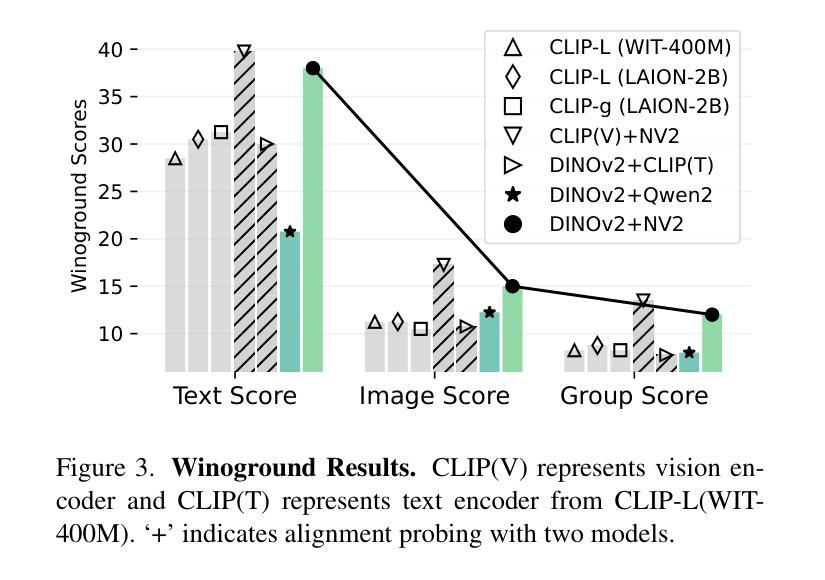
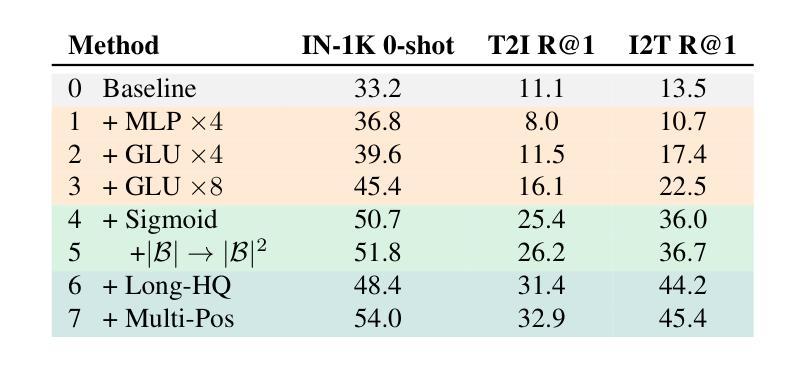
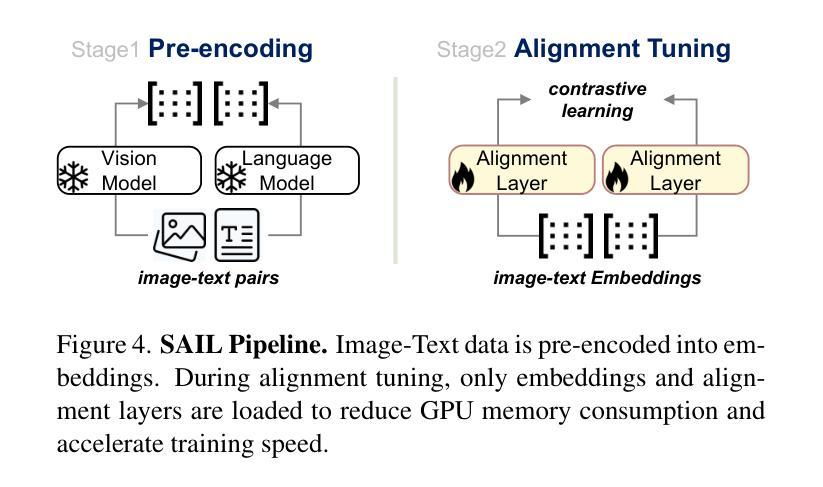
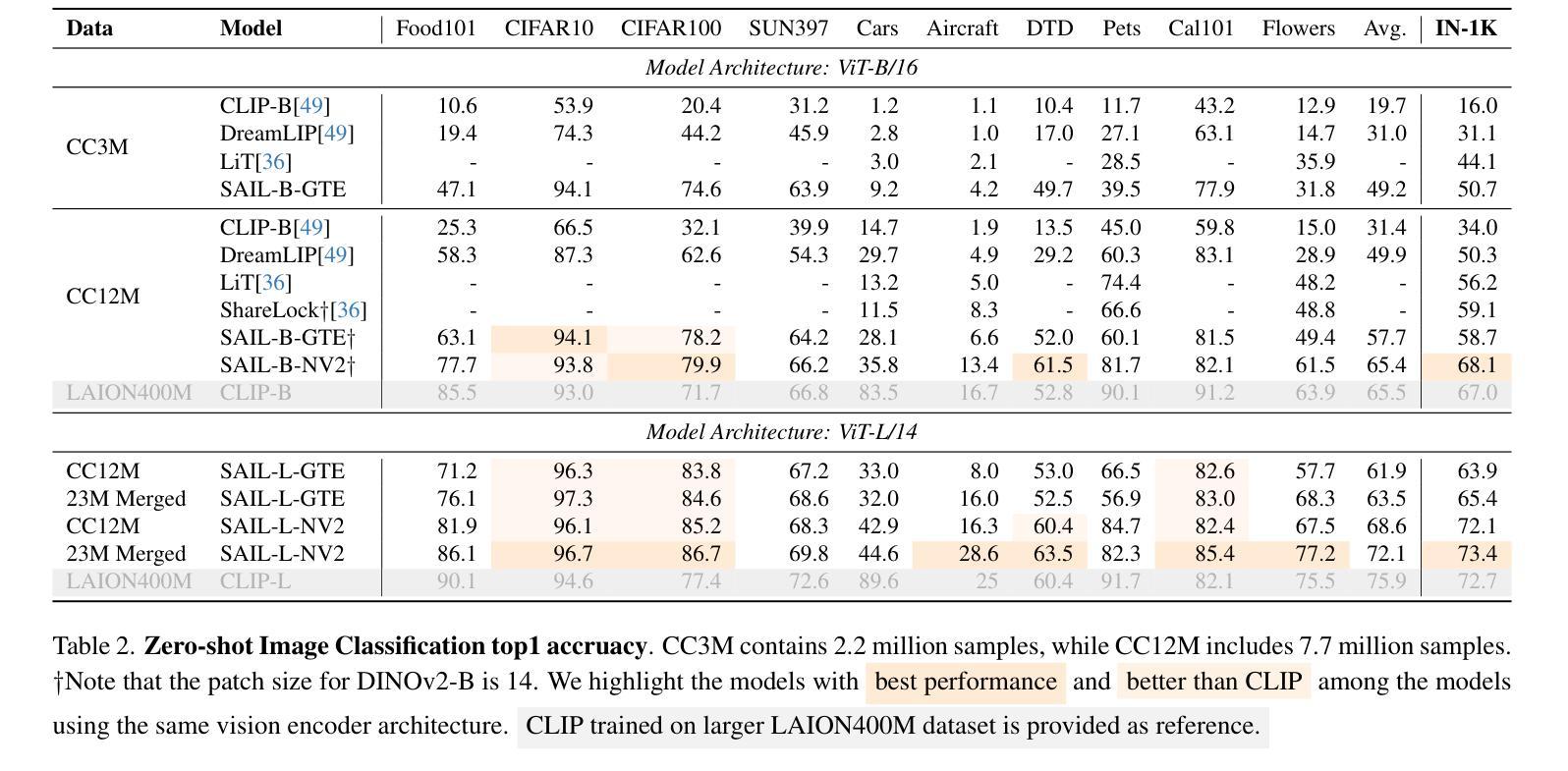
Controlling Space and Time with Diffusion Models
Authors:Daniel Watson, Saurabh Saxena, Lala Li, Andrea Tagliasacchi, David J. Fleet
We present 4DiM, a cascaded diffusion model for 4D novel view synthesis (NVS), supporting generation with arbitrary camera trajectories and timestamps, in natural scenes, conditioned on one or more images. With a novel architecture and sampling procedure, we enable training on a mixture of 3D (with camera pose), 4D (pose+time) and video (time but no pose) data, which greatly improves generalization to unseen images and camera pose trajectories over prior works that focus on limited domains (e.g., object centric). 4DiM is the first-ever NVS method with intuitive metric-scale camera pose control enabled by our novel calibration pipeline for structure-from-motion-posed data. Experiments demonstrate that 4DiM outperforms prior 3D NVS models both in terms of image fidelity and pose alignment, while also enabling the generation of scene dynamics. 4DiM provides a general framework for a variety of tasks including single-image-to-3D, two-image-to-video (interpolation and extrapolation), and pose-conditioned video-to-video translation, which we illustrate qualitatively on a variety of scenes. For an overview see https://4d-diffusion.github.io
我们提出了4DiM,这是一种用于4D新型视图合成(NVS)的级联扩散模型,支持在自然环境场景中,根据一张或多张图像,生成具有任意相机轨迹和时间戳的内容。通过采用新颖的结构和采样流程,我们能够混合使用3D(带有相机姿态)、4D(姿态+时间)和视频(有时间但没有姿态)数据进行训练,这极大地提高了在未见过的图像和相机姿态轨迹上的泛化能力,超过了那些专注于有限领域(例如,以对象为中心)的先前工作。4DiM是首个能够通过我们的新型基于结构从运动定位数据的校准管道实现直观度量相机姿态控制的新型视图合成方法。实验表明,在图像保真度和姿态对齐方面,4DiM优于先前的3D NVS模型,同时还能够生成场景动态。4DiM为各种任务提供了一个通用框架,包括单图像到3D、两图像到视频(插值和外推)以及姿态条件视频到视频的翻译,我们在各种场景中对这些任务进行了定性的说明。有关概述,请参见:https://4d-diffusion.github.io。
论文及项目相关链接
PDF ICLR 2025, First three authors contributed equally
Summary
基于提供的文本,我们提出了一种名为4DiM的级联扩散模型,用于支持以任意相机轨迹和时间戳生成自然场景的四维新视角合成(NVS)。通过新颖架构和采样流程,我们的模型能在多种数据集上进行训练,包括混合的三维数据(带相机姿态)、四维数据(姿态+时间)和视频数据(时间但没有姿态)。这使得我们的模型在未见过的图像和相机姿态轨迹上具有更好的泛化能力。实验证明,在图像保真度和姿态对齐方面,我们的模型优于之前的三维NVS模型。此外,我们还展示了该模型在多种任务上的通用性,包括单图像到三维、双图像到视频插帧与外推,以及姿态调节的视频转换。对于总体介绍,请参阅链接。
Key Takeaways
- 提出了名为4DiM的级联扩散模型用于四维新视角合成(NVS)。
- 支持以任意相机轨迹和时间戳进行生成。
- 采用新颖架构和采样流程实现混合三维、四维和视频数据的训练。
- 显著提升在未见过的图像和相机姿态轨迹上的泛化性能。
- 在图像保真度和姿态对齐方面优于先前的三维NVS模型。
点此查看论文截图
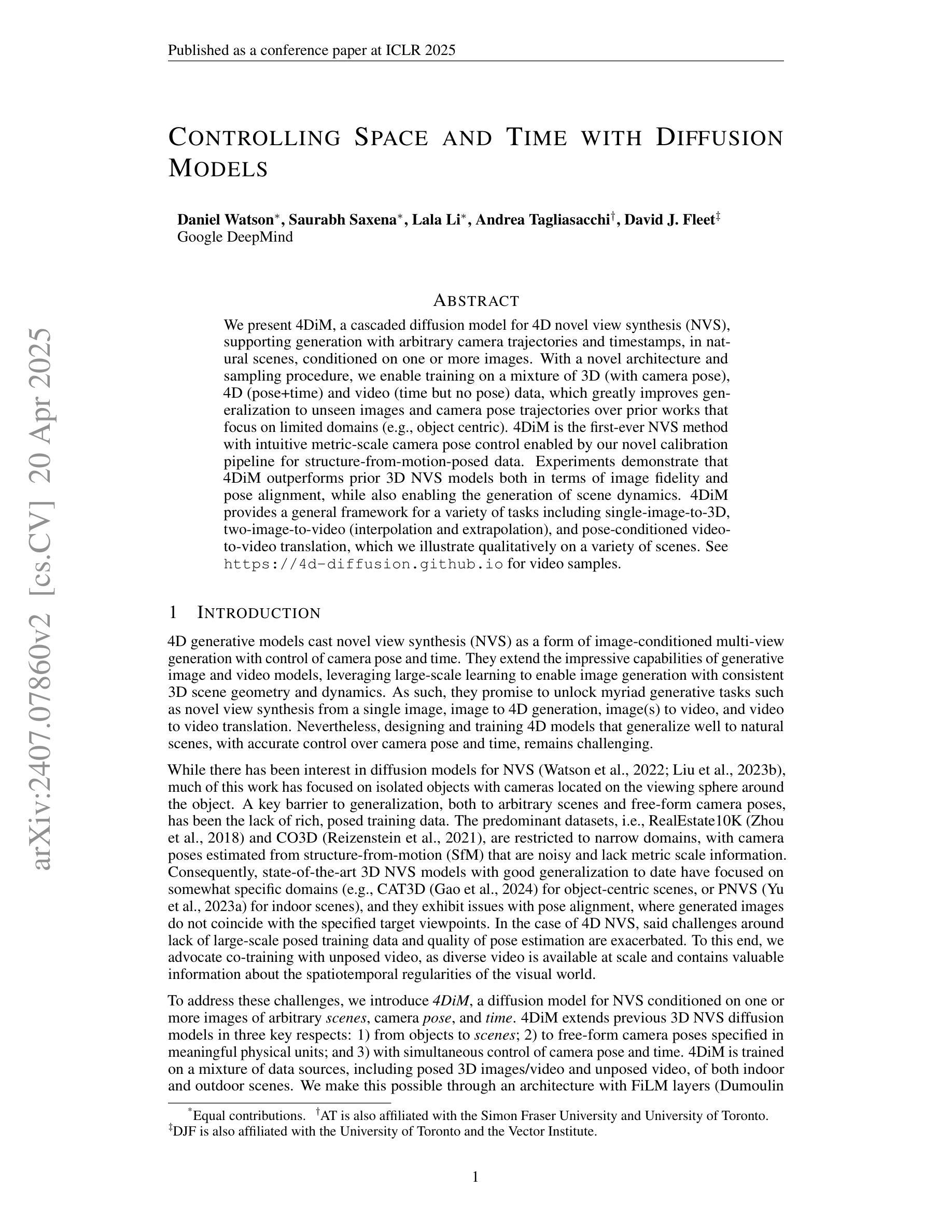
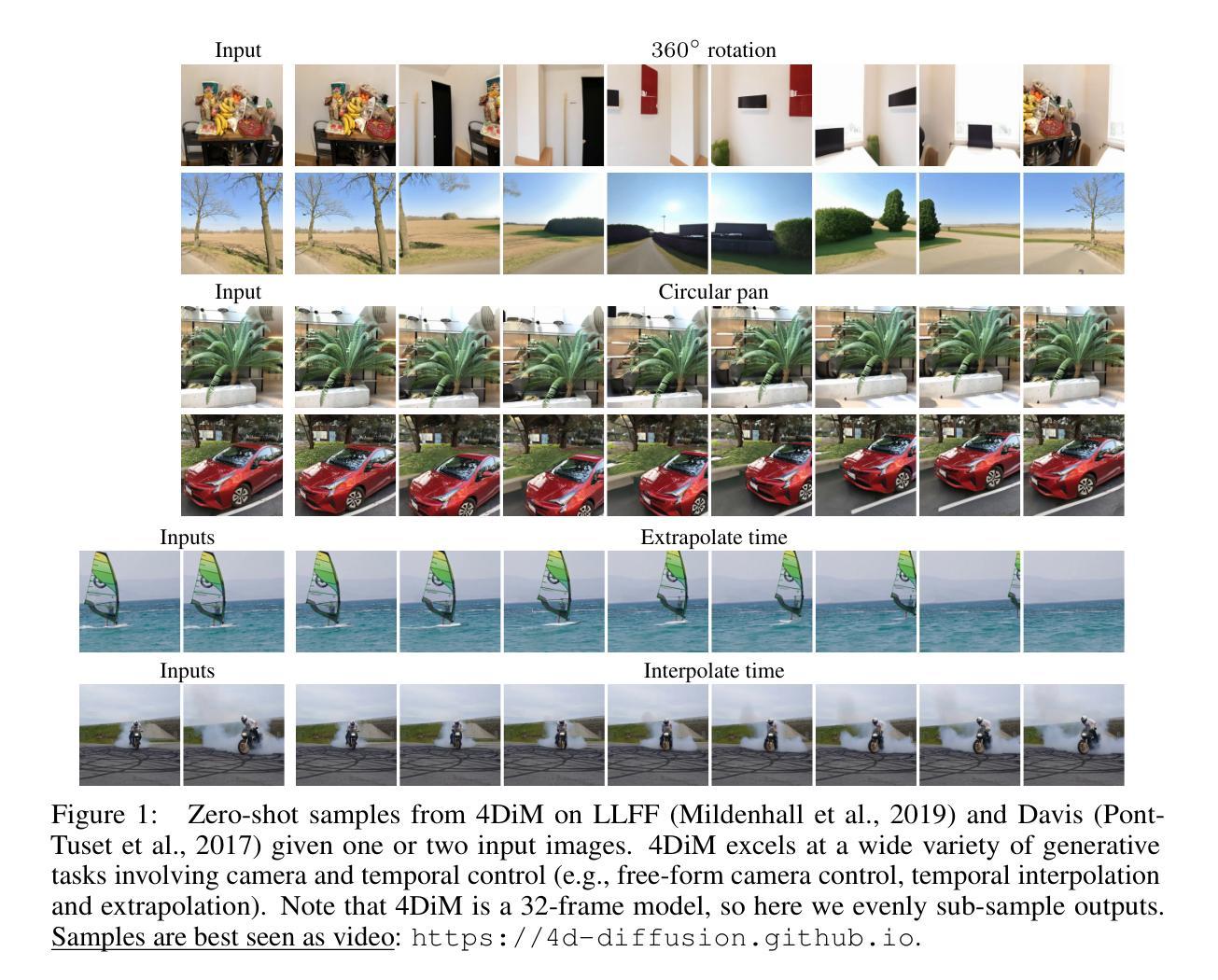
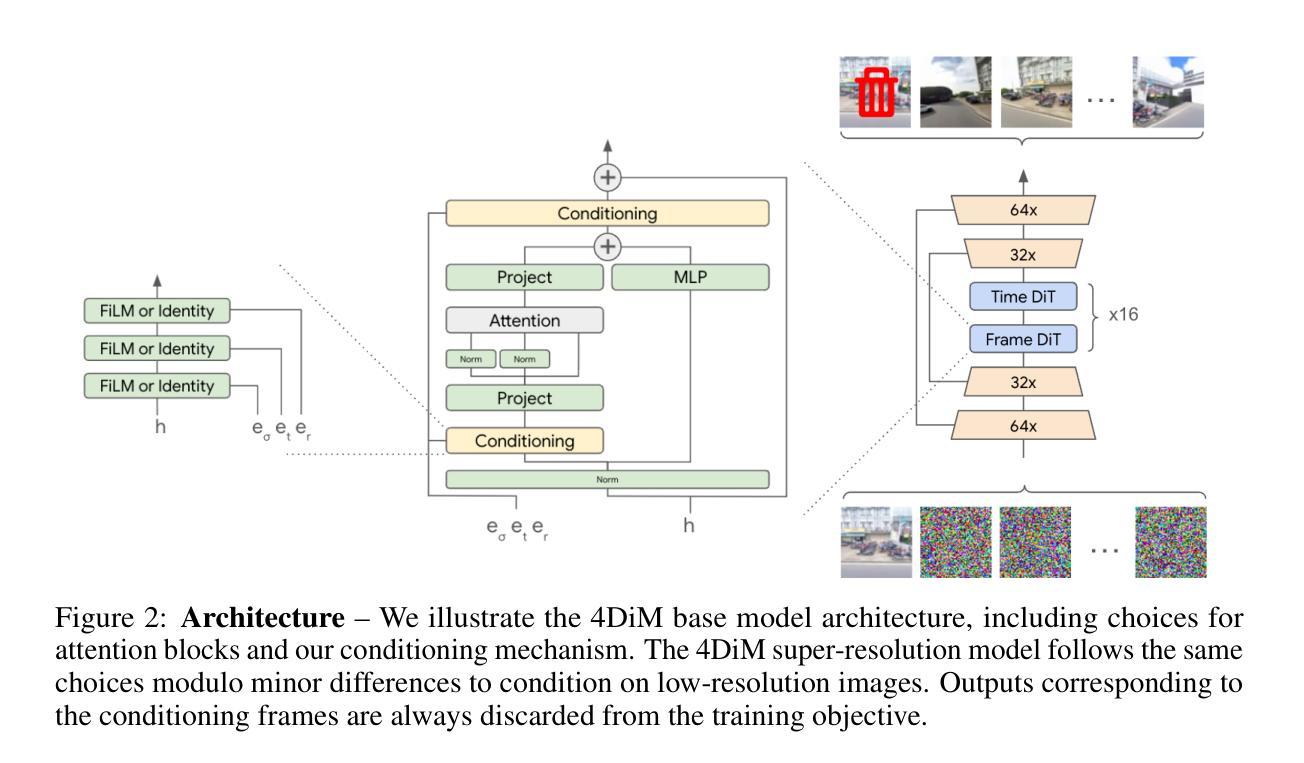
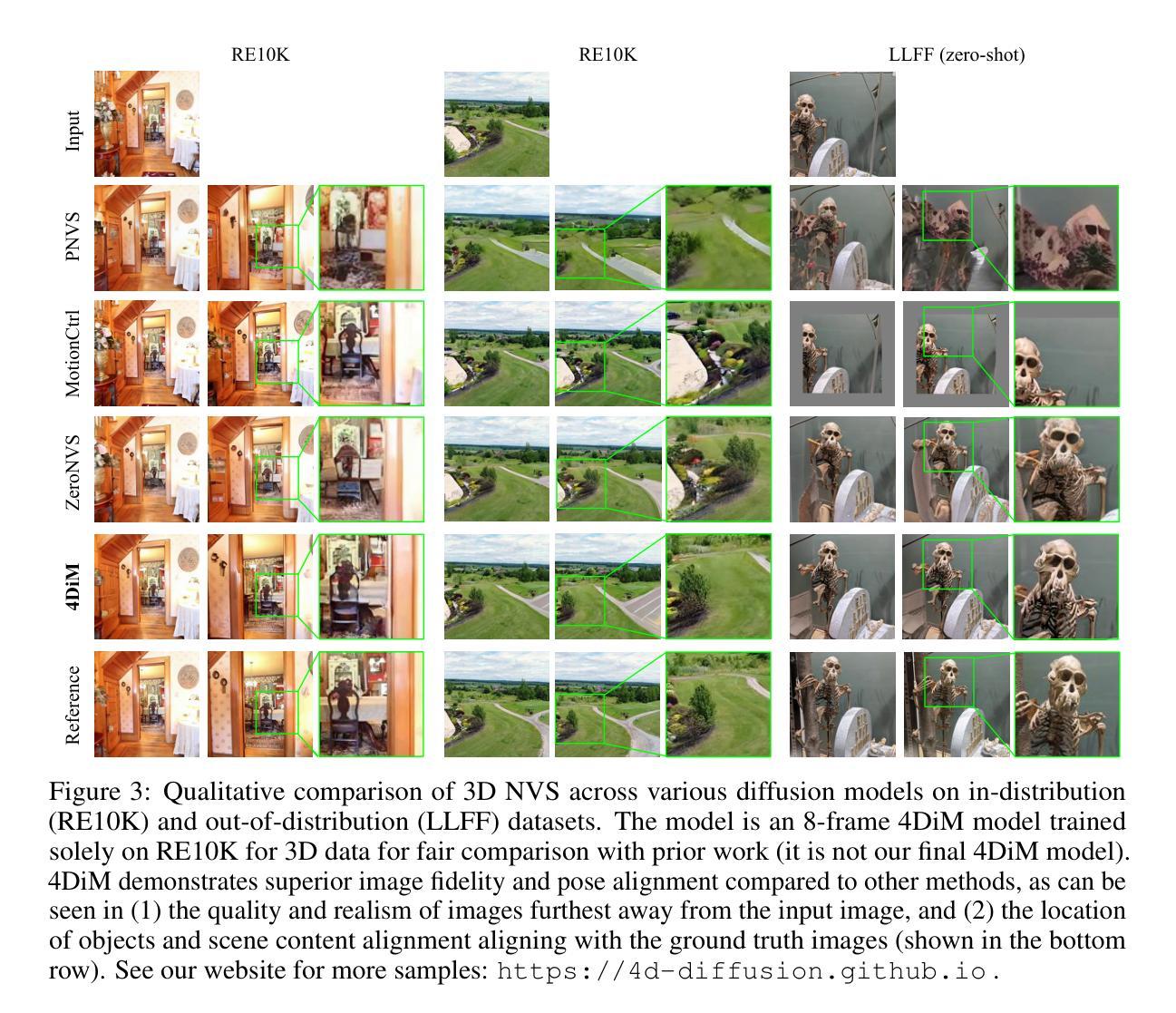