⚠️ 以下所有内容总结都来自于 大语言模型的能力,如有错误,仅供参考,谨慎使用
🔴 请注意:千万不要用于严肃的学术场景,只能用于论文阅读前的初筛!
💗 如果您觉得我们的项目对您有帮助 ChatPaperFree ,还请您给我们一些鼓励!⭐️ HuggingFace免费体验
2025-04-23 更新
SOLIDO: A Robust Watermarking Method for Speech Synthesis via Low-Rank Adaptation
Authors:Yue Li, Weizhi Liu, Dongdong Lin
The accelerated advancement of speech generative models has given rise to security issues, including model infringement and unauthorized abuse of content. Although existing generative watermarking techniques have proposed corresponding solutions, most methods require substantial computational overhead and training costs. In addition, some methods have limitations in robustness when handling variable-length inputs. To tackle these challenges, we propose \textsc{SOLIDO}, a novel generative watermarking method that integrates parameter-efficient fine-tuning with speech watermarking through low-rank adaptation (LoRA) for speech diffusion models. Concretely, the watermark encoder converts the watermark to align with the input of diffusion models. To achieve precise watermark extraction from variable-length inputs, the watermark decoder based on depthwise separable convolution is designed for watermark recovery. To further enhance speech generation performance and watermark extraction capability, we propose a speech-driven lightweight fine-tuning strategy, which reduces computational overhead through LoRA. Comprehensive experiments demonstrate that the proposed method ensures high-fidelity watermarked speech even at a large capacity of 2000 bps. Furthermore, against common individual and compound speech attacks, our SOLIDO achieves a maximum average extraction accuracy of 99.20% and 98.43%, respectively. It surpasses other state-of-the-art methods by nearly 23% in resisting time-stretching attacks.
随着语音生成模型的快速发展,出现了一些安全问题,包括模型侵权和未经授权的滥用内容。尽管现有的生成水印技术已经提出了相应的解决方案,但大多数方法需要大量的计算开销和训练成本。此外,一些方法在处理可变长度输入时存在稳健性方面的局限性。为了应对这些挑战,我们提出了名为SOLIDO的新型生成水印方法,该方法将参数高效的微调与语音水印结合,通过低秩适应(LoRA)技术应用于语音扩散模型。具体来说,水印编码器将水印转换为与扩散模型的输入对齐的格式。为了实现从可变长度输入中进行精确的水印提取,我们设计了基于深度可分离卷积的水印解码器以恢复水印。为了进一步提高语音生成性能和水印提取能力,我们提出了一种语音驱动的轻量级微调策略,通过LoRA技术降低计算开销。综合实验表明,该方法能够确保即使在2000 bps的大容量下,也能生成高保真度的水印语音。此外,面对常见的个人和复合语音攻击,我们的SOLIDO方案达到了最高平均提取准确率99.20%和98.43%。在抵抗时间拉伸攻击方面,它超越了其他先进的方法,提高了近23%。
论文及项目相关链接
Summary
本文介绍了语音生成模型的快速发展带来的安全问题,包括模型侵权和未经授权的内容滥用。针对现有生成水印技术的计算开销大、训练成本高以及处理可变长度输入时鲁棒性有限等问题,提出了一种新的生成水印方法SOLIDO。该方法结合了参数高效的微调与语音水印技术,通过低阶适应(LoRA)为语音扩散模型提供支持。实验表明,该方法在保证高保真水印语音的同时,对多种常见语音攻击具有良好的抵御能力。
Key Takeaways
- 语音生成模型的快速发展引发了包括模型侵权和未经授权内容滥用在内的安全问题。
- 现有生成水印技术存在计算开销大、训练成本高以及处理可变长度输入时鲁棒性有限等问题。
- SOLIDO是一种新的生成水印方法,结合了参数高效的微调与语音水印技术。
- SOLIDO通过低阶适应(LoRA)支持语音扩散模型,提高了语音生成性能和水印提取能力。
- 水印编码器能够将水印转换为与扩散模型输入对齐的格式。
- 基于深度可分离卷积的水印解码器设计用于精确地从可变长度输入中提取水印。
点此查看论文截图
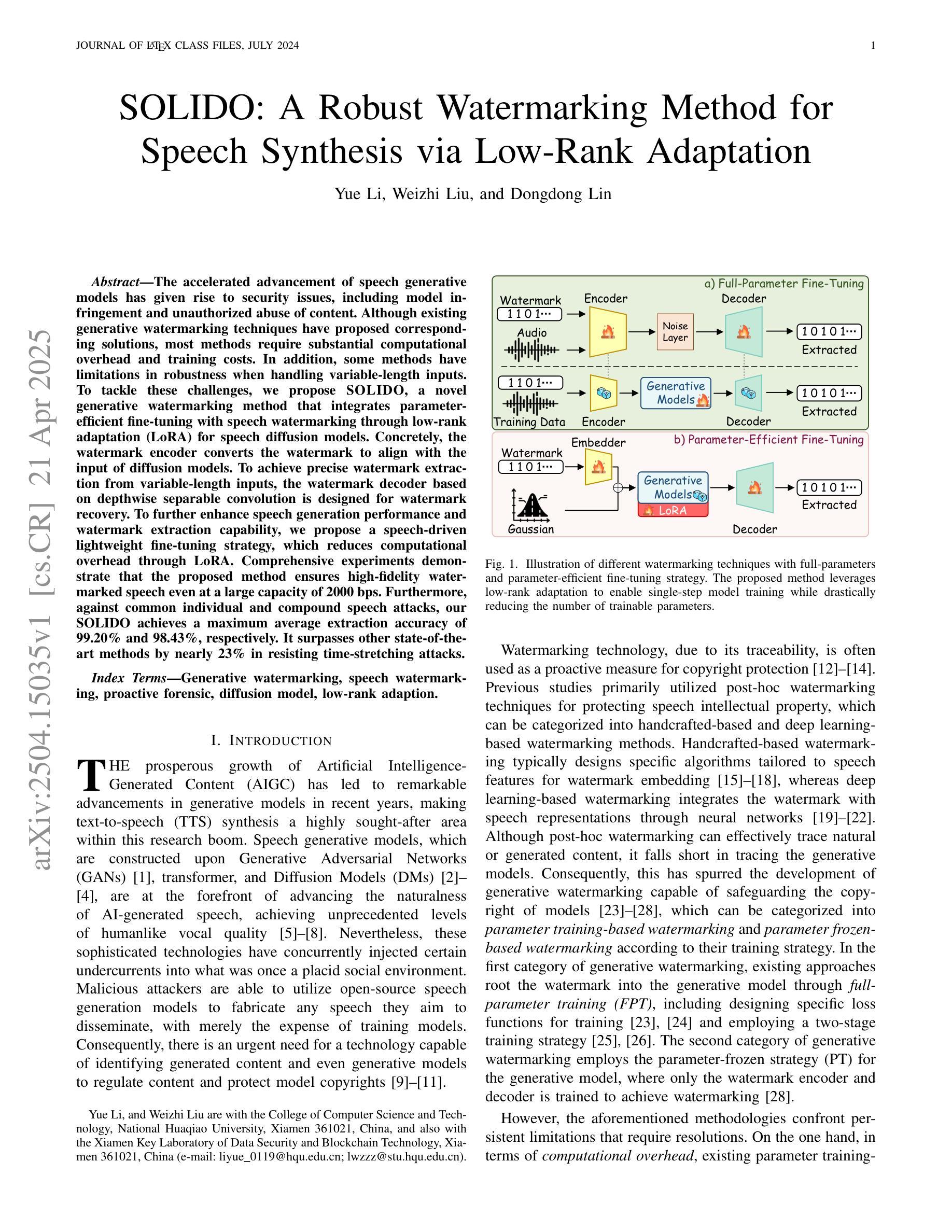
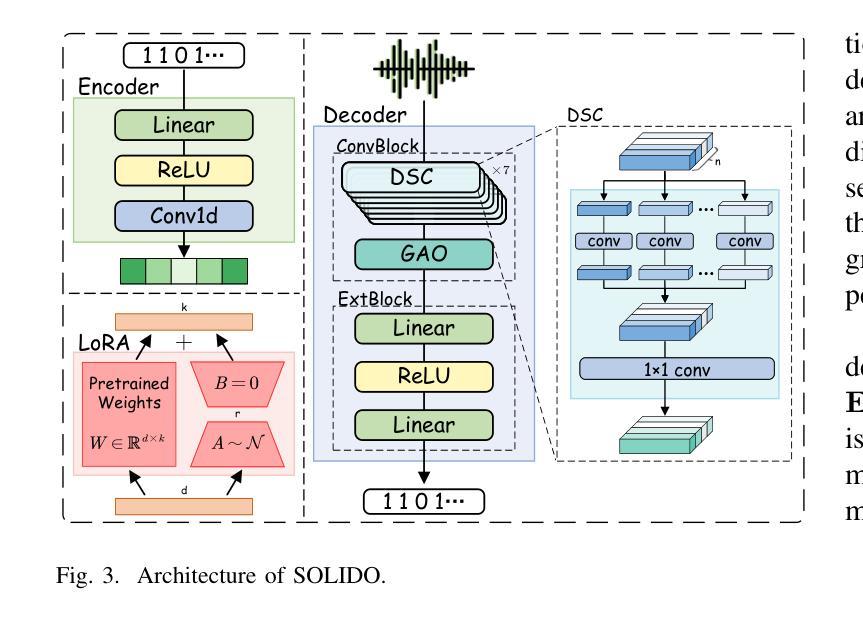
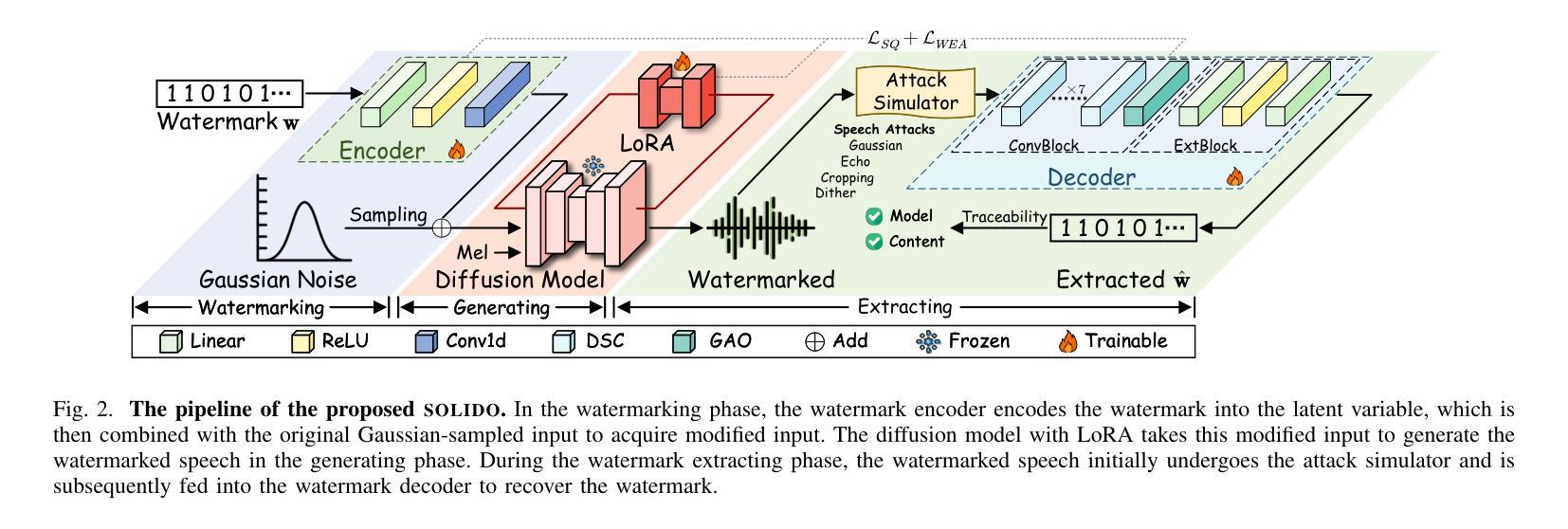
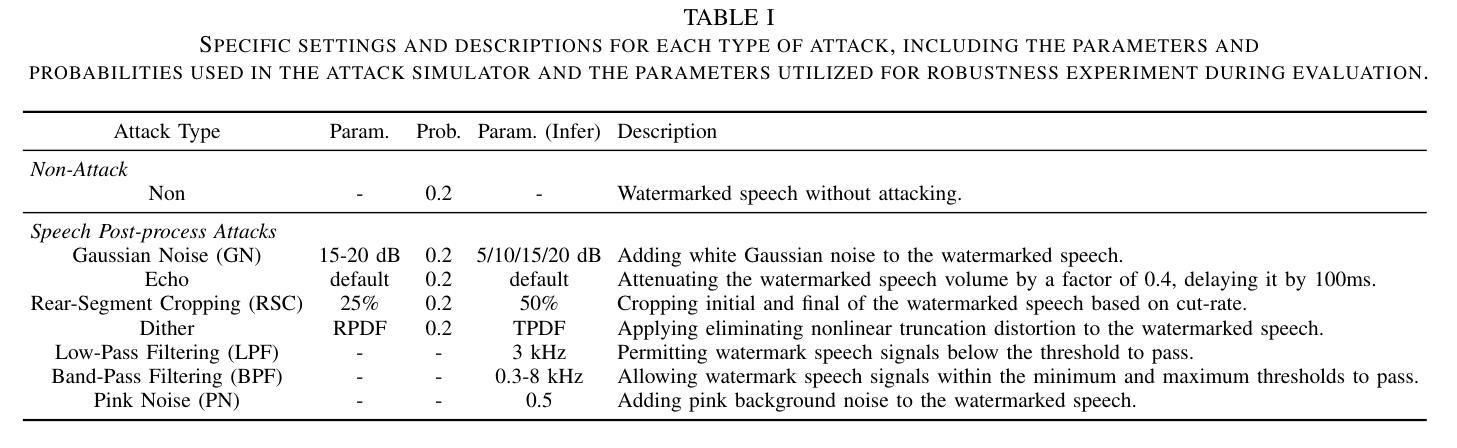
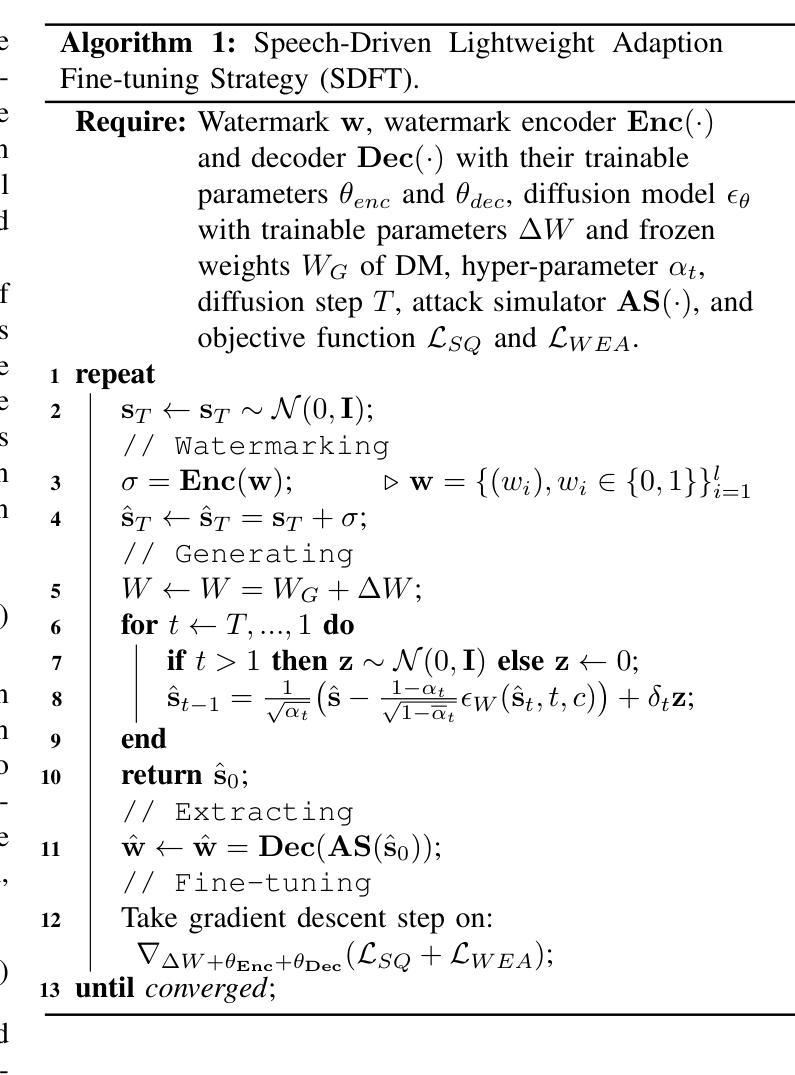
DialogueAgents: A Hybrid Agent-Based Speech Synthesis Framework for Multi-Party Dialogue
Authors:Xiang Li, Duyi Pan, Hongru Xiao, Jiale Han, Jing Tang, Jiabao Ma, Wei Wang, Bo Cheng
Speech synthesis is crucial for human-computer interaction, enabling natural and intuitive communication. However, existing datasets involve high construction costs due to manual annotation and suffer from limited character diversity, contextual scenarios, and emotional expressiveness. To address these issues, we propose DialogueAgents, a novel hybrid agent-based speech synthesis framework, which integrates three specialized agents – a script writer, a speech synthesizer, and a dialogue critic – to collaboratively generate dialogues. Grounded in a diverse character pool, the framework iteratively refines dialogue scripts and synthesizes speech based on speech review, boosting emotional expressiveness and paralinguistic features of the synthesized dialogues. Using DialogueAgent, we contribute MultiTalk, a bilingual, multi-party, multi-turn speech dialogue dataset covering diverse topics. Extensive experiments demonstrate the effectiveness of our framework and the high quality of the MultiTalk dataset. We release the dataset and code https://github.com/uirlx/DialogueAgents to facilitate future research on advanced speech synthesis models and customized data generation.
语音合成对于人机交互至关重要,能够实现自然直观的沟通。然而,现有数据集存在高构建成本问题,因为需要进行手动标注,并且在字符多样性、上下文场景和情感表达方面存在局限性。为了解决这些问题,我们提出了DialogueAgents,这是一种新型的基于代理的语音合成框架。它集成了脚本编写器、语音合成器和对话评论家三个专业代理,共同生成对话。该框架基于多样化的字符池,通过对话脚本迭代优化和基于语音评论的语音合成,提高合成对话的情感表达和非语言特征。使用DialogueAgent,我们创建了MultiTalk数据集,这是一个涵盖多种话题的双语、多方、多轮语音对话数据集。大量实验证明了我们框架的有效性和MultiTalk数据集的高质量。我们公开了数据集和代码https://github.com/uirlx/DialogueAgents,以促进未来对先进语音合成模型和定制数据生成的研究。
论文及项目相关链接
PDF Accepted by ICME 2025. Dataset and code are publicly available: https://github.com/uirlx/DialogueAgents
Summary
本文提出一种基于代理的语音合成框架DialogueAgents,集成了脚本编写器、语音合成器和对话评论家三个专业代理,以协作方式生成对话。该框架通过多样化的角色池为基础,根据语音评论迭代优化对话脚本并合成语音,提高了合成对话的情感表达和非语言特征。同时,本文贡献了一个双语、多方、多轮对话数据集MultiTalk,涵盖各种主题。实验证明该框架的有效性以及MultiTalk数据集的高质量。
Key Takeaways
- DialogueAgents是一个基于代理的语音合成框架,集成了脚本编写、语音合成和对话评论功能。
- 该框架利用多样化的角色池,提高了对话的自然度和丰富度。
- DialogueAgents通过语音评论迭代优化对话脚本,增强了情感表达和非语言特征。
- MultiTalk数据集是一个双语、多方、多轮对话数据集,涵盖各种主题,为高级语音合成模型的研究提供了资源。
- 本文通过实验证明了DialogueAgents框架和MultiTalk数据集的有效性。
- DialogueAgents和MultiTalk数据集已公开发布,便于未来相关研究。
点此查看论文截图
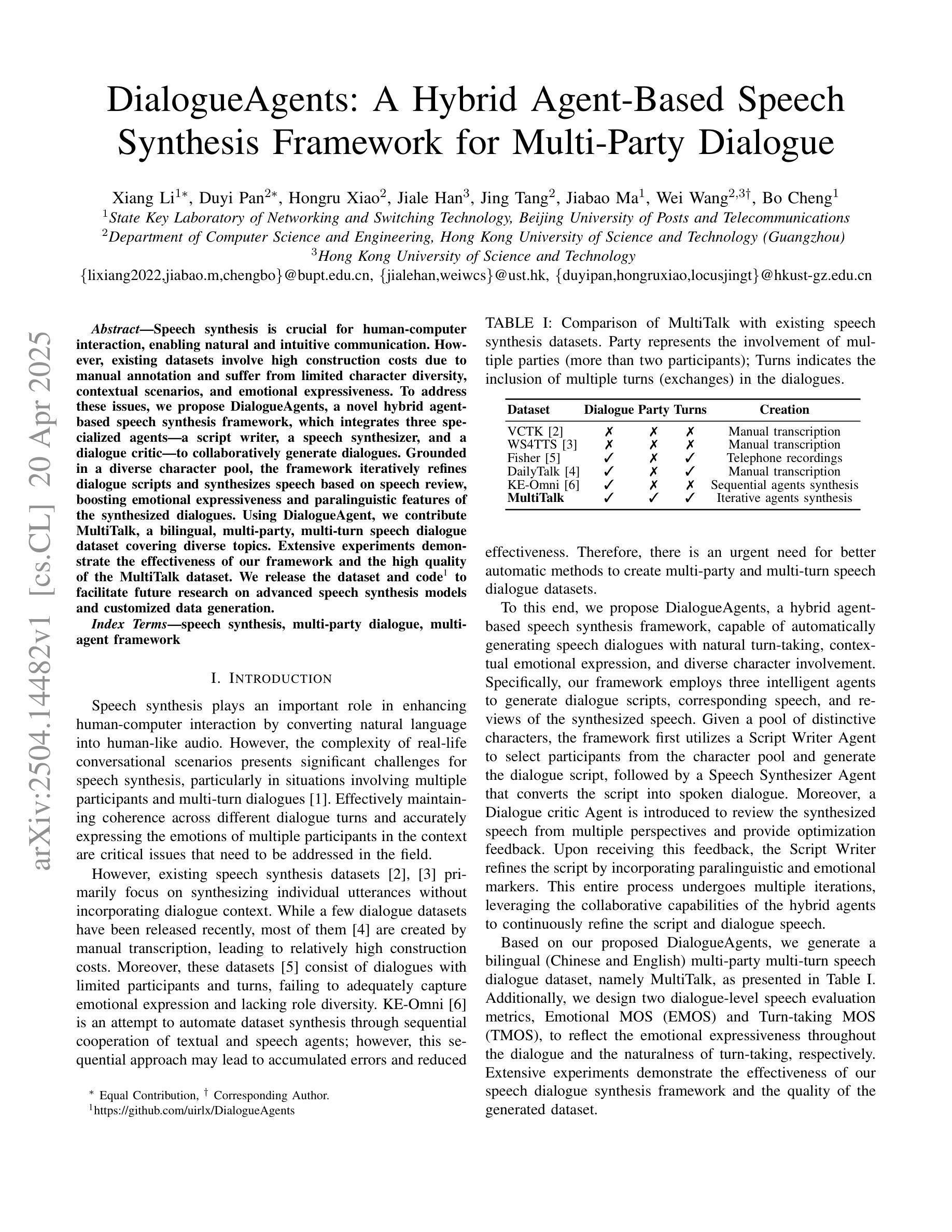
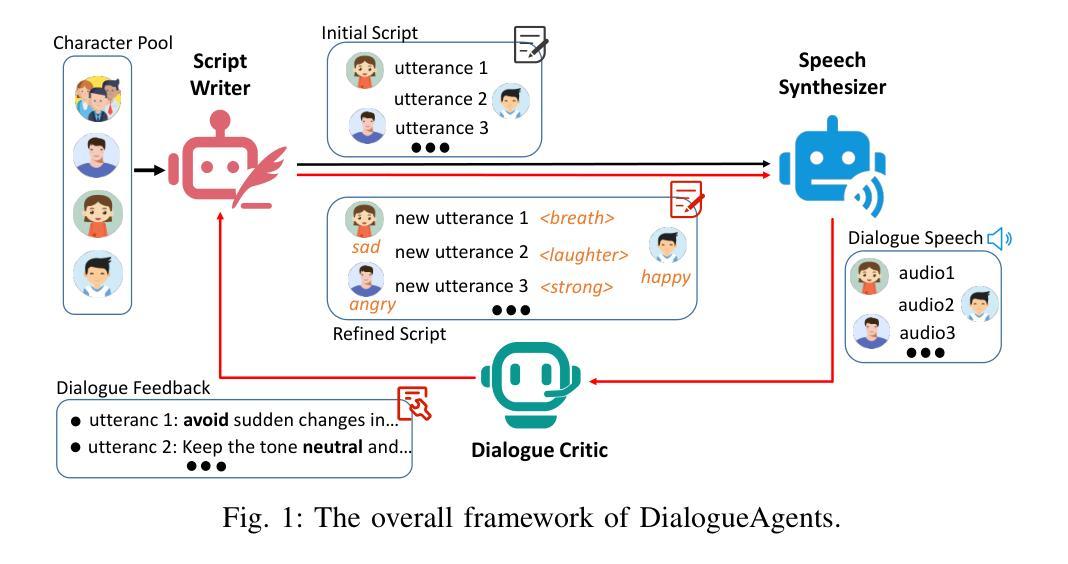
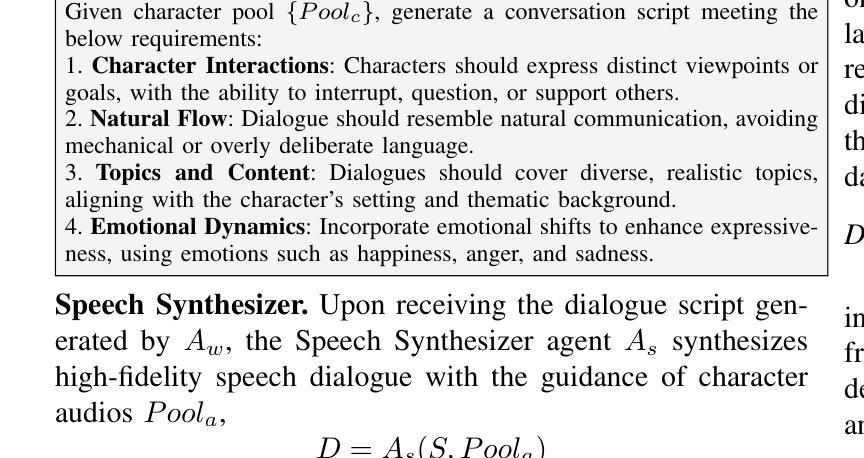
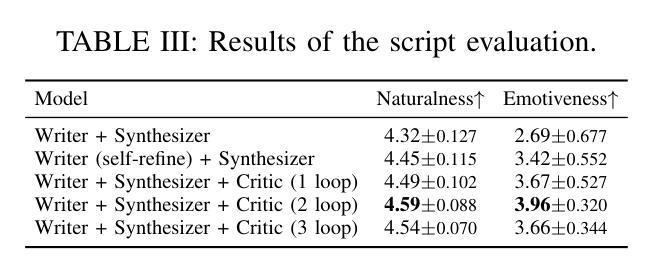
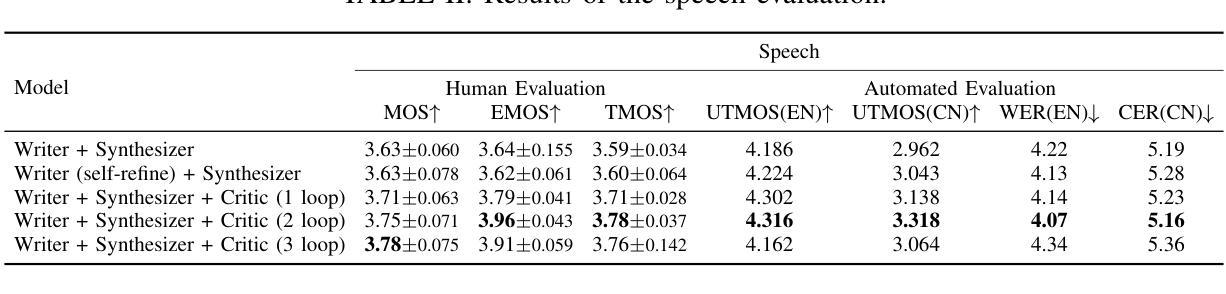
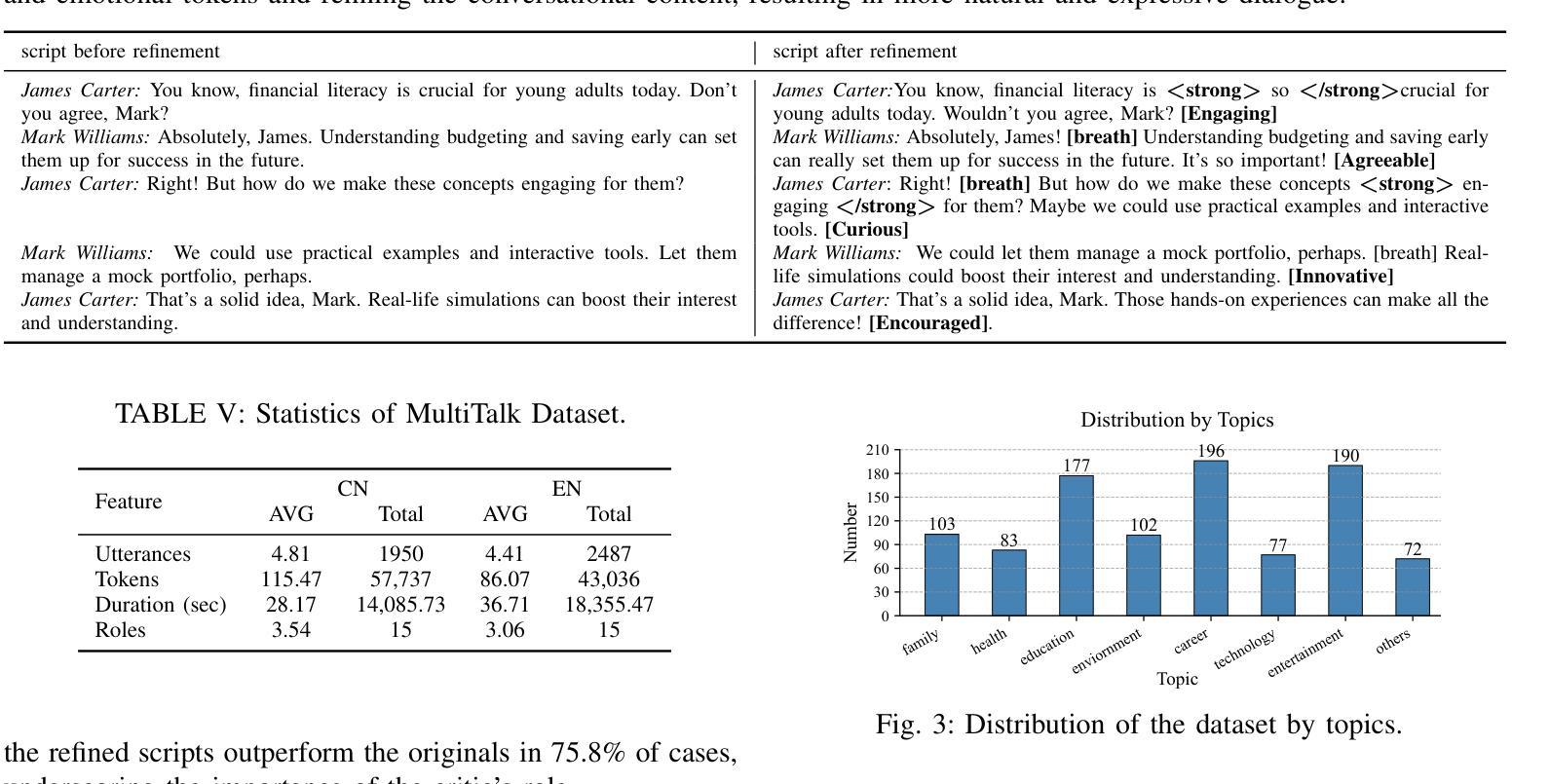
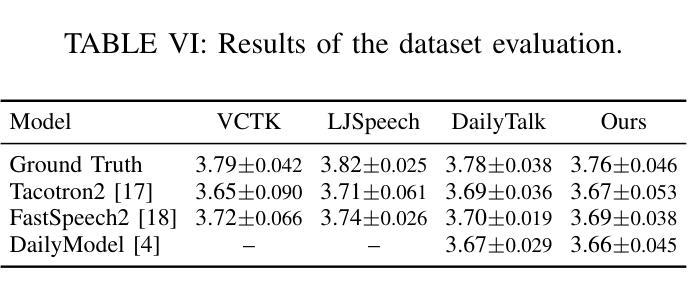