⚠️ 以下所有内容总结都来自于 大语言模型的能力,如有错误,仅供参考,谨慎使用
🔴 请注意:千万不要用于严肃的学术场景,只能用于论文阅读前的初筛!
💗 如果您觉得我们的项目对您有帮助 ChatPaperFree ,还请您给我们一些鼓励!⭐️ HuggingFace免费体验
2025-04-23 更新
SOLIDO: A Robust Watermarking Method for Speech Synthesis via Low-Rank Adaptation
Authors:Yue Li, Weizhi Liu, Dongdong Lin
The accelerated advancement of speech generative models has given rise to security issues, including model infringement and unauthorized abuse of content. Although existing generative watermarking techniques have proposed corresponding solutions, most methods require substantial computational overhead and training costs. In addition, some methods have limitations in robustness when handling variable-length inputs. To tackle these challenges, we propose \textsc{SOLIDO}, a novel generative watermarking method that integrates parameter-efficient fine-tuning with speech watermarking through low-rank adaptation (LoRA) for speech diffusion models. Concretely, the watermark encoder converts the watermark to align with the input of diffusion models. To achieve precise watermark extraction from variable-length inputs, the watermark decoder based on depthwise separable convolution is designed for watermark recovery. To further enhance speech generation performance and watermark extraction capability, we propose a speech-driven lightweight fine-tuning strategy, which reduces computational overhead through LoRA. Comprehensive experiments demonstrate that the proposed method ensures high-fidelity watermarked speech even at a large capacity of 2000 bps. Furthermore, against common individual and compound speech attacks, our SOLIDO achieves a maximum average extraction accuracy of 99.20% and 98.43%, respectively. It surpasses other state-of-the-art methods by nearly 23% in resisting time-stretching attacks.
随着语音生成模型的快速发展,也出现了一些安全问题,包括模型侵权和未经授权的滥用内容。尽管现有的生成水印技术已经提出了相应的解决方案,但大多数方法计算开销大、训练成本高。此外,一些方法在处理不同长度的输入时稳健性存在局限。为了应对这些挑战,我们提出了名为SOLIDO的新型生成水印方法,它将参数高效的微调与语音水印技术结合,通过低秩适应(LoRA)用于语音扩散模型。具体来说,水印编码器将水印转换为与扩散模型的输入对齐的格式。为了实现从可变长度输入中精确提取水印,我们设计了基于深度可分离卷积的水印解码器进行水印恢复。为了进一步改善语音生成性能和提取水印的能力,我们提出了一种语音驱动的轻量级微调策略,通过LoRA降低计算开销。综合实验表明,该方法可以确保即使在高达2000 bps容量下,仍能保持高质量的水印语音。此外,面对常见的个人和复合语音攻击,我们的SOLIDO方法最高平均提取准确率分别达到了99.20%和98.43%。在抵抗时间拉伸攻击方面,它比其他最先进的方法高出近23%。
论文及项目相关链接
Summary
语音生成模型的快速发展带来了包括模型侵权和未经授权的内容滥用在内的安全问题。现有生成水印技术虽然已有相应的解决方案,但大多数方法计算开销大且训练成本高。本文提出了一种新型生成水印方法——SOLIDO,通过低秩自适应(LoRA)实现了参数高效的微调与语音水印的结合。该方法能将水印编码与扩散模型输入对齐,并通过深度可分离卷积的水印解码器实现精确的水印提取。此外,本文还提出了一种基于语音驱动的微调策略,以提高语音生成性能和水印提取能力,减少计算开销。实验证明,该方法能在高保真度下嵌入水印,且在多种攻击下表现出强大的提取准确性。
Key Takeaways
- 语音生成模型的快速发展带来了安全问题,如模型侵权和未经授权的内容滥用。
- 现有生成水印技术存在计算开销大、训练成本高的问题。
- SOLIDO是一种新型的生成水印方法,结合了参数高效的微调与语音水印技术。
- SOLIDO实现了水印与扩散模型输入的对齐,并通过深度可分离卷积实现了精确的水印提取,适用于处理可变长度的输入。
- SOLIDO提出了一种基于语音驱动的微调策略,以提高语音生成性能和水印提取能力,同时降低计算开销。
- 实验证明,SOLIDO方法能在保证高保真度的情况下嵌入水印。
点此查看论文截图
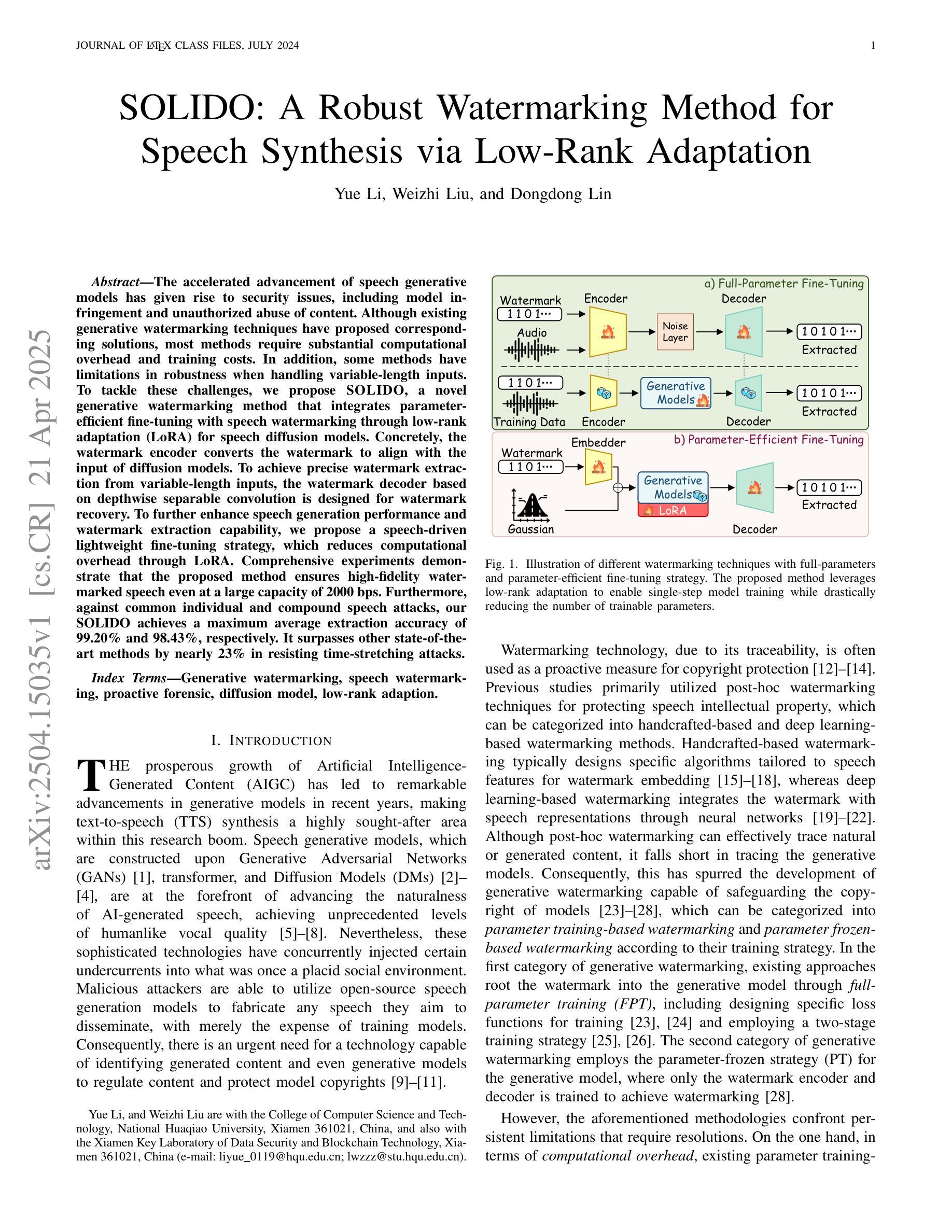
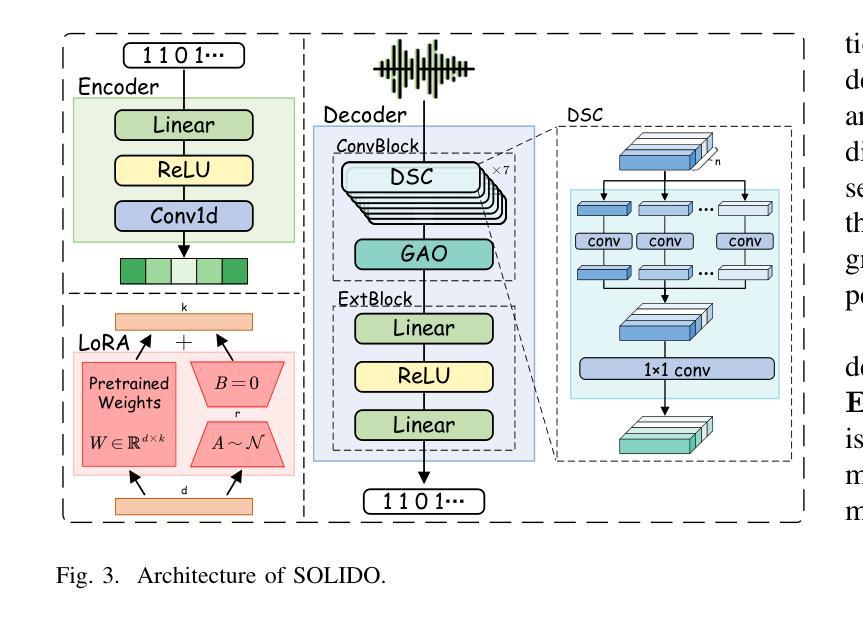
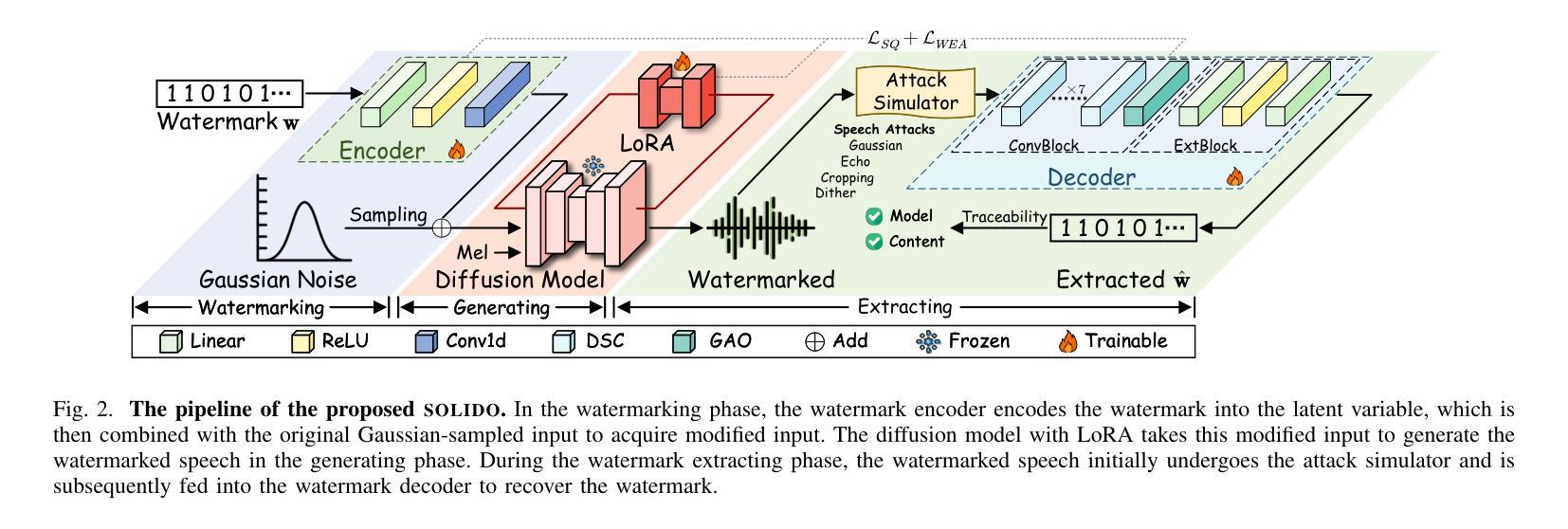
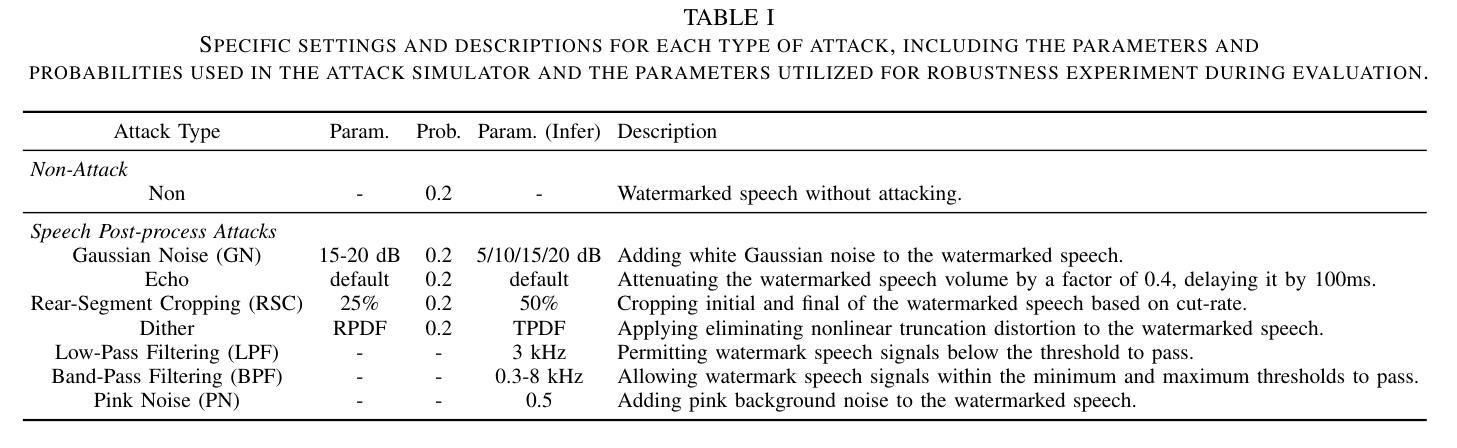
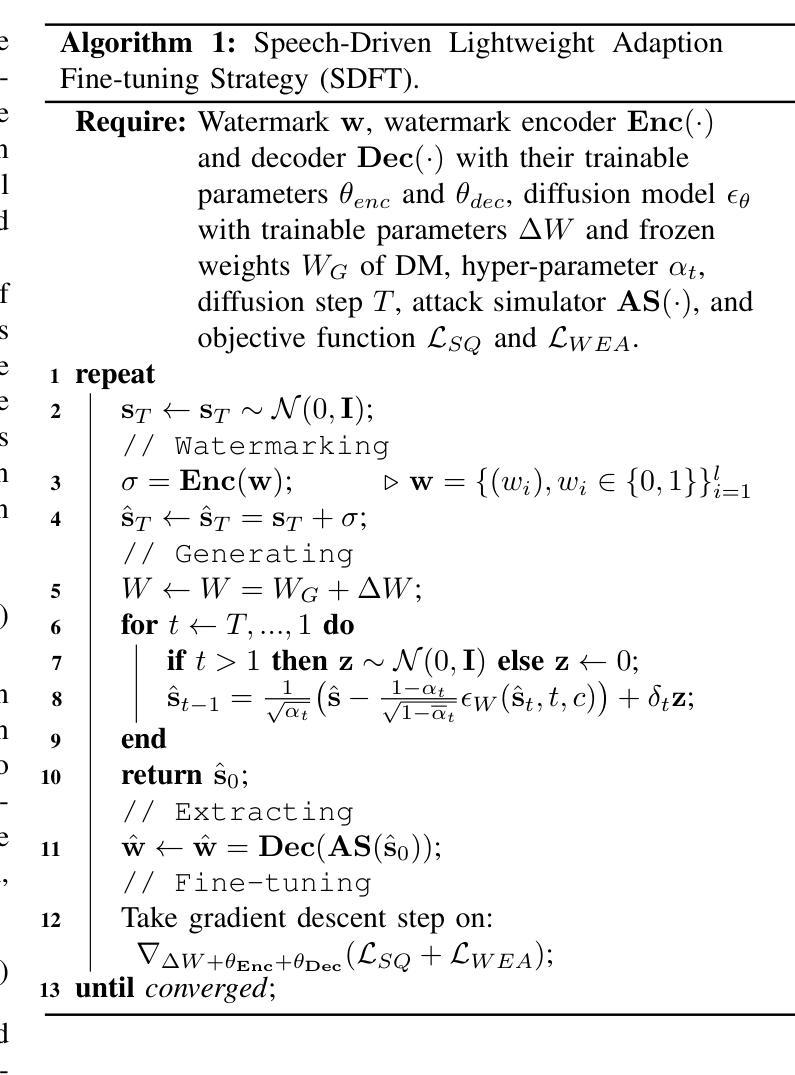
Talk is Not Always Cheap: Promoting Wireless Sensing Models with Text Prompts
Authors:Zhenkui Yang, Zeyi Huang, Ge Wang, Han Ding, Tony Xiao Han, Fei Wang
Wireless signal-based human sensing technologies, such as WiFi, millimeter-wave (mmWave) radar, and Radio Frequency Identification (RFID), enable the detection and interpretation of human presence, posture, and activities, thereby providing critical support for applications in public security, healthcare, and smart environments. These technologies exhibit notable advantages due to their non-contact operation and environmental adaptability; however, existing systems often fail to leverage the textual information inherent in datasets. To address this, we propose an innovative text-enhanced wireless sensing framework, WiTalk, that seamlessly integrates semantic knowledge through three hierarchical prompt strategies-label-only, brief description, and detailed action description-without requiring architectural modifications or incurring additional data costs. We rigorously validate this framework across three public benchmark datasets: XRF55 for human action recognition (HAR), and WiFiTAL and XRFV2 for WiFi temporal action localization (TAL). Experimental results demonstrate significant performance improvements: on XRF55, accuracy for WiFi, RFID, and mmWave increases by 3.9%, 2.59%, and 0.46%, respectively; on WiFiTAL, the average performance of WiFiTAD improves by 4.98%; and on XRFV2, the mean average precision gains across various methods range from 4.02% to 13.68%. Our codes have been included in https://github.com/yangzhenkui/WiTalk.
基于无线信号的人体感知技术,如WiFi、毫米波(mmWave)雷达和射频识别(RFID),能够检测和解读人的存在、姿势和活动,从而为公共安全、医疗护理和智能环境等领域的应用提供关键支持。这些技术具有非接触性操作和环境适应性强等优点;然而,现有系统往往未能充分利用数据集内在的文本信息。针对这一问题,我们提出了一种创新的文本增强无线感知框架WiTalk,它通过三种分层提示策略——仅标签、简短描述和详细动作描述——无缝集成语义知识,无需进行架构修改或产生额外数据成本。我们在三个人体行为识别(HAR)公共基准数据集XRF55,以及两个WiFi临时动作定位(TAL)数据集WiFiTAL和XRFV2上严格验证了此框架。实验结果表明,性能有显著改进:在XRF55上,WiFi、RFID和mmWave的准确率分别提高了3.9%、2.59%和0.46%;在WiFiTAL上,WiFiTAD的平均性能提高了4.98%;在XRFV2上,各种方法的平均精度增益在4.02%到13.68%之间。我们的代码已发布在https://github.com/yangzhenkui/WiTalk。
论文及项目相关链接
PDF 10 pages
摘要
无线信号基人体感知技术,如WiFi、毫米波雷达和射频识别,能检测并解读人的存在、姿势和活动,为公共安全、医疗和智能环境等领域提供重要支持。尽管这些技术具有非接触式操作和适应环境等优点,但现有系统往往未能充分利用数据集中的文本信息。为解决这一问题,本文提出一种创新的文本增强无线感知框架WiTalk,它通过三种层次化的提示策略——仅标签、简短描述和详细动作描述,无缝集成语义知识,无需修改架构或产生额外数据成本。在XRF55人体动作识别、WiFiTAL和XRFV2 WiFi时序动作定位三个公共基准数据集上的实验结果表明,该框架显著提高了性能:在XRF55上,WiFi、RFID和毫米波雷达的准确率分别提高了3.9%、2.59%和0.46%;在WiFiTAL上,WiFiTAD的平均性能提高了4.98%;在XRFV2上,各种方法的平均精度增益在4.02%至13.68%之间。相关代码已发布在https://github.com/yangzhenkui/WiTalk。
关键见解
- 无线信号基人体感知技术可通过WiFi、毫米波雷达和射频识别等方式检测并解读人的存在、姿势和活动。
- 这些技术在公共安全、医疗和智能环境等领域有广泛应用。
- 现有系统常常忽略数据集中的文本信息,无法充分利用其优势。
- WiTalk框架通过三种层次化的提示策略无缝集成语义知识,提高无线感知技术的性能。
- WiTalk框架无需修改现有架构或产生额外数据成本。
- 在多个公共基准数据集上的实验结果表明WiTalk框架显著提高了无线信号基人体感知技术的性能。
点此查看论文截图
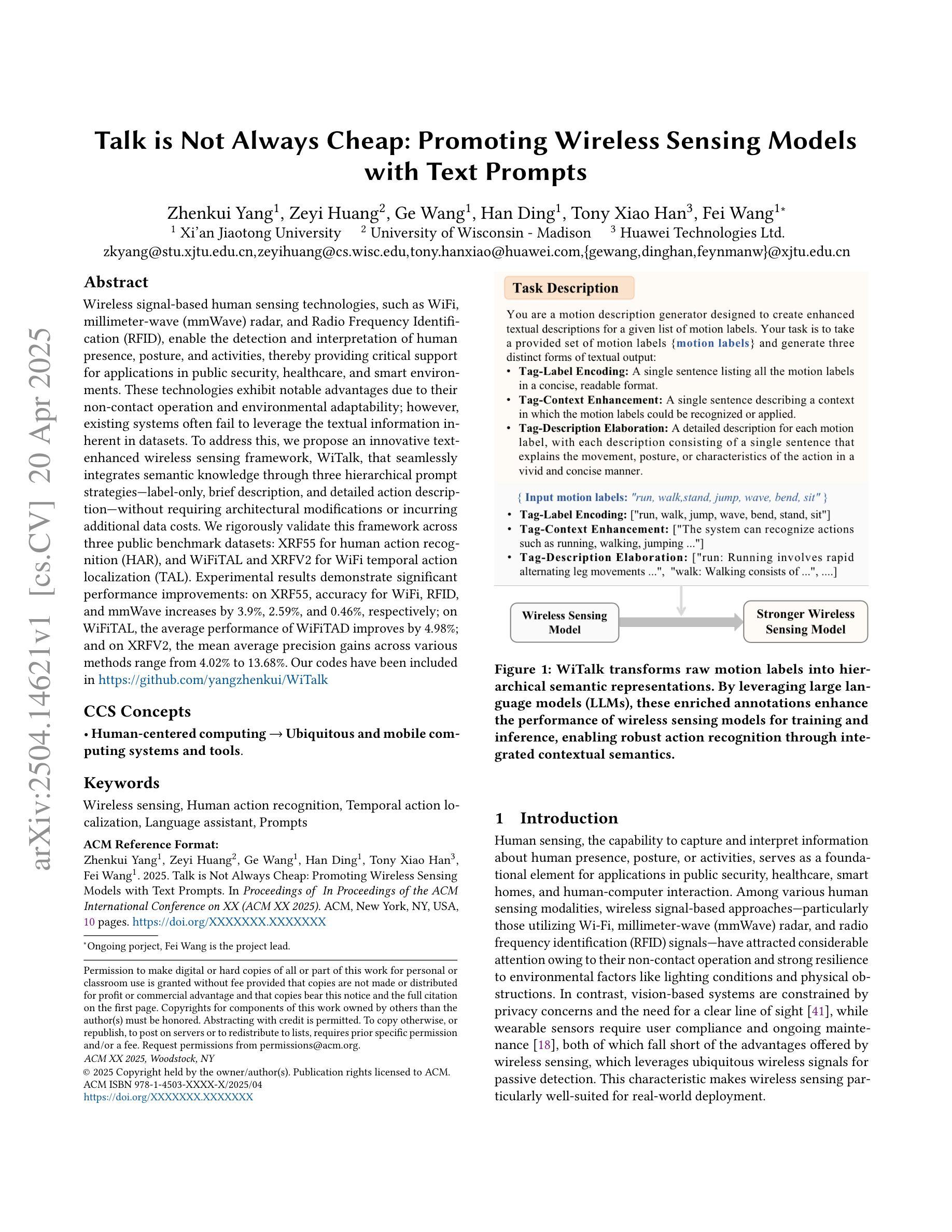
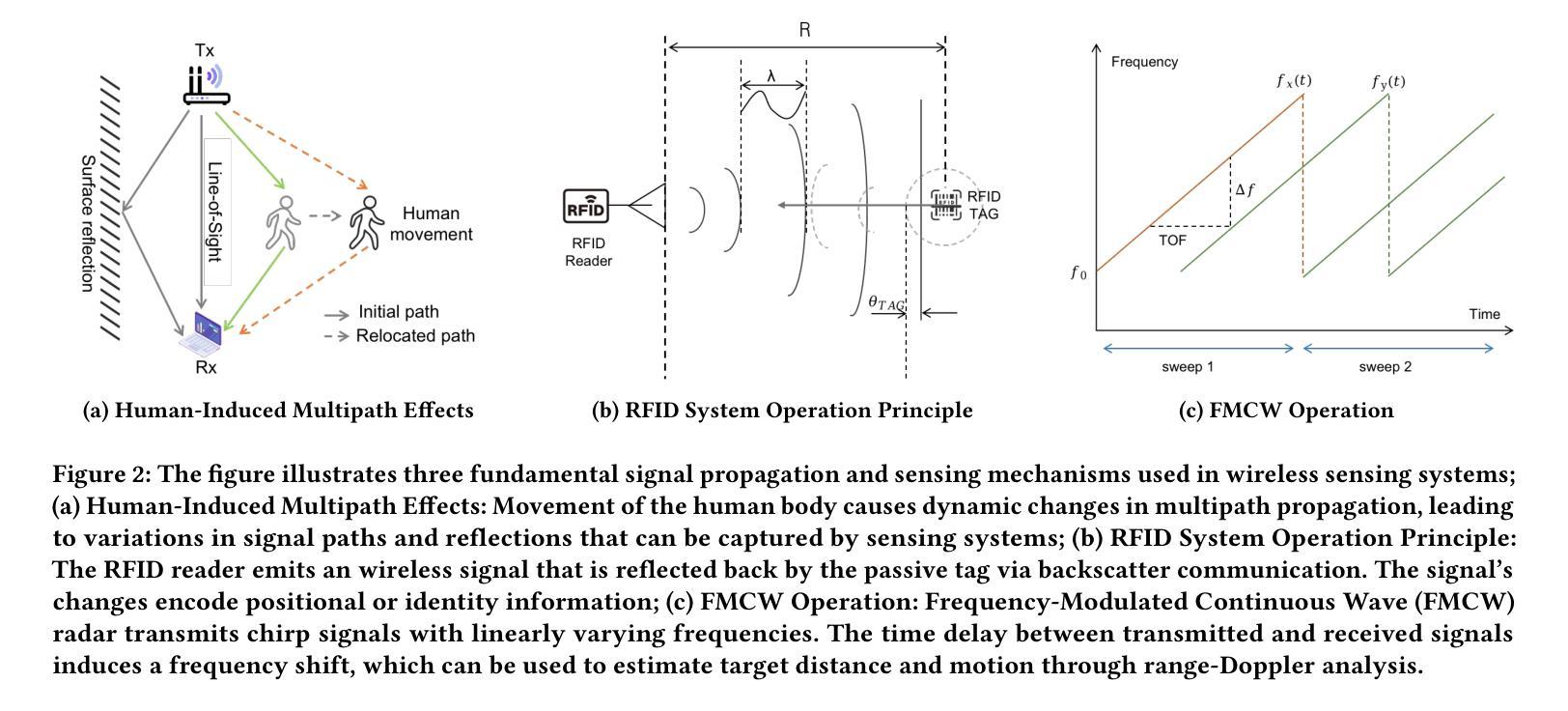
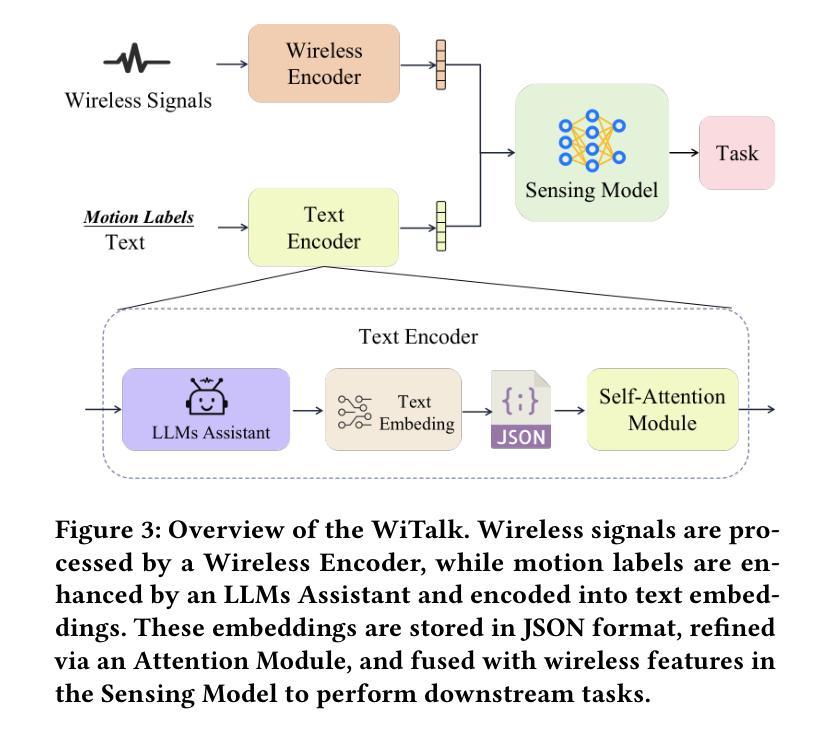