⚠️ 以下所有内容总结都来自于 大语言模型的能力,如有错误,仅供参考,谨慎使用
🔴 请注意:千万不要用于严肃的学术场景,只能用于论文阅读前的初筛!
💗 如果您觉得我们的项目对您有帮助 ChatPaperFree ,还请您给我们一些鼓励!⭐️ HuggingFace免费体验
2025-04-24 更新
Text-based Animatable 3D Avatars with Morphable Model Alignment
Authors:Yiqian Wu, Malte Prinzler, Xiaogang Jin, Siyu Tang
The generation of high-quality, animatable 3D head avatars from text has enormous potential in content creation applications such as games, movies, and embodied virtual assistants. Current text-to-3D generation methods typically combine parametric head models with 2D diffusion models using score distillation sampling to produce 3D-consistent results. However, they struggle to synthesize realistic details and suffer from misalignments between the appearance and the driving parametric model, resulting in unnatural animation results. We discovered that these limitations stem from ambiguities in the 2D diffusion predictions during 3D avatar distillation, specifically: i) the avatar’s appearance and geometry is underconstrained by the text input, and ii) the semantic alignment between the predictions and the parametric head model is insufficient because the diffusion model alone cannot incorporate information from the parametric model. In this work, we propose a novel framework, AnimPortrait3D, for text-based realistic animatable 3DGS avatar generation with morphable model alignment, and introduce two key strategies to address these challenges. First, we tackle appearance and geometry ambiguities by utilizing prior information from a pretrained text-to-3D model to initialize a 3D avatar with robust appearance, geometry, and rigging relationships to the morphable model. Second, we refine the initial 3D avatar for dynamic expressions using a ControlNet that is conditioned on semantic and normal maps of the morphable model to ensure accurate alignment. As a result, our method outperforms existing approaches in terms of synthesis quality, alignment, and animation fidelity. Our experiments show that the proposed method advances the state of the art in text-based, animatable 3D head avatar generation.
从文本生成高质量、可动画的3D头像在内容创建应用(如游戏、电影和实体虚拟助手)中具有巨大潜力。当前的文本到3D生成方法通常将参数化头部模型与二维扩散模型相结合,使用分数蒸馏采样技术产生一致的3D结果。然而,它们在合成真实细节方面遇到困难,并且在外观和驱动参数模型之间存在不匹配,导致动画结果不自然。我们发现这些限制源于在3D头像蒸馏期间二维扩散预测的模糊性,具体表现为:i)头像的外观和几何形状受到文本输入的约束较少;ii)由于扩散模型本身无法融入参数模型的信息,导致预测结果与参数模型之间的语义对齐不足。在这项工作中,我们提出了一种基于文本生成真实可动画的3DGS头像的新型框架AnimPortrait3D,并引入两种关键策略来解决这些挑战。首先,我们通过利用预训练的文本到3D模型的先验信息来解决外观和几何形状的模糊性,以初始化一个具有稳健外观、几何形状以及与可变形模型关联关系的3D头像。其次,我们使用以可变形模型的语义图和法线图为基础的ControlNet对初始的3D头像进行微调,以确保精确对齐。因此,我们的方法在合成质量、对齐和动画保真度方面优于现有方法。实验表明,该方法在基于文本的动画3D头像生成方面达到了最新水平。
论文及项目相关链接
Summary
本文介绍了基于文本生成高质量、可动画的3D头像的巨大潜力及其在内容创建应用(如游戏、电影和虚拟助手)中的应用。当前方法结合参数化头部模型和二维扩散模型,但存在合成细节不真实和对齐问题。本文提出一种新型框架AnimPortrait3D,采用可变模型对齐解决这些问题,利用先验信息和ControlNet技术,提高了合成质量、对齐性和动画保真度。
Key Takeaways
- 基于文本的3D头像生成在内容创建领域具有巨大潜力。
- 当前方法结合参数化头部模型和二维扩散模型,但存在合成细节不真实的问题。
- 新型框架AnimPortrait3D可以解决当前方法的局限性。
- AnimPortrait3D利用先验信息初始化3D头像,具有稳健的外观、几何和骨骼绑定关系。
- ControlNet技术用于精细调整3D头像的动态表情,确保与可变模型的语义和正常地图对齐。
- 该方法在提高合成质量、对齐性和动画保真度方面优于现有方法。
点此查看论文截图
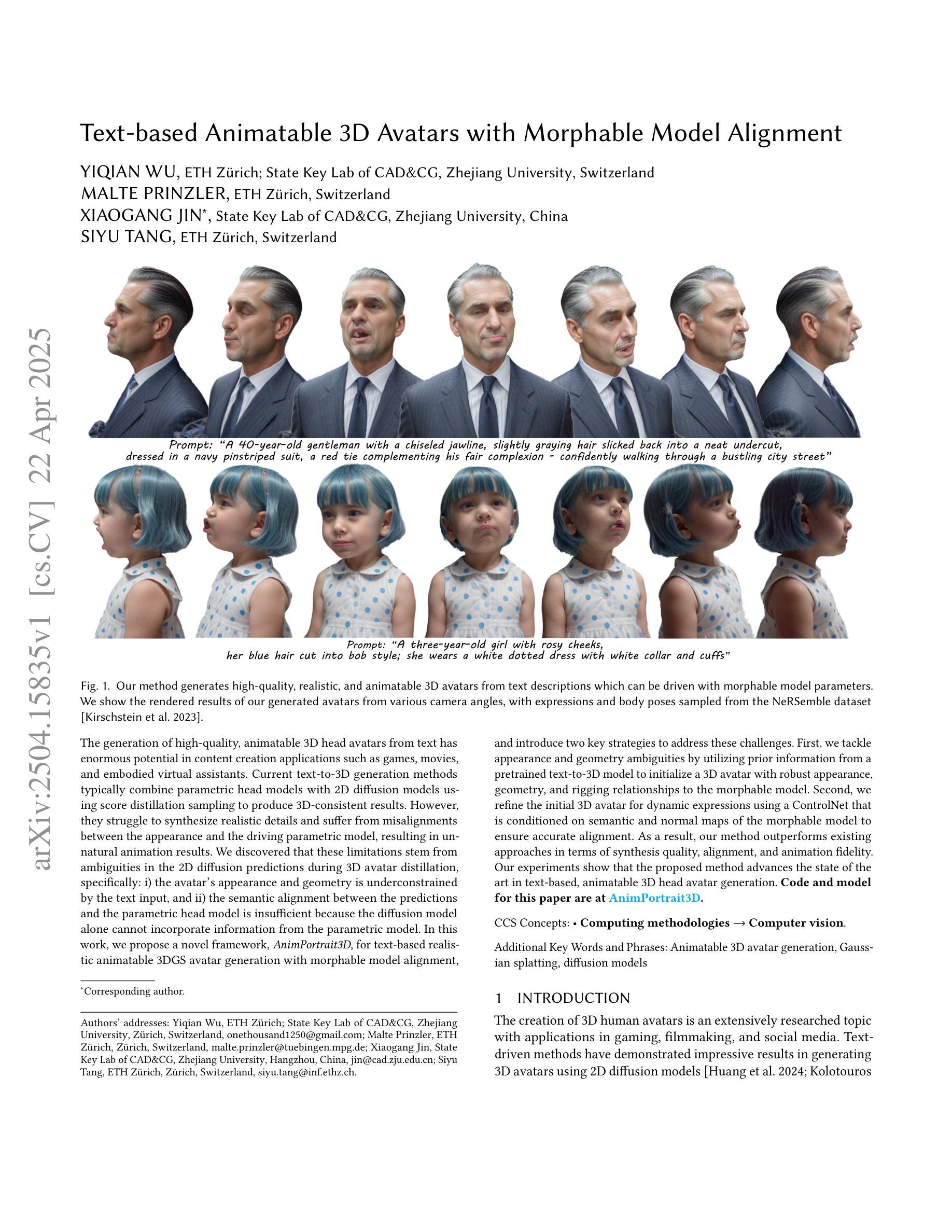
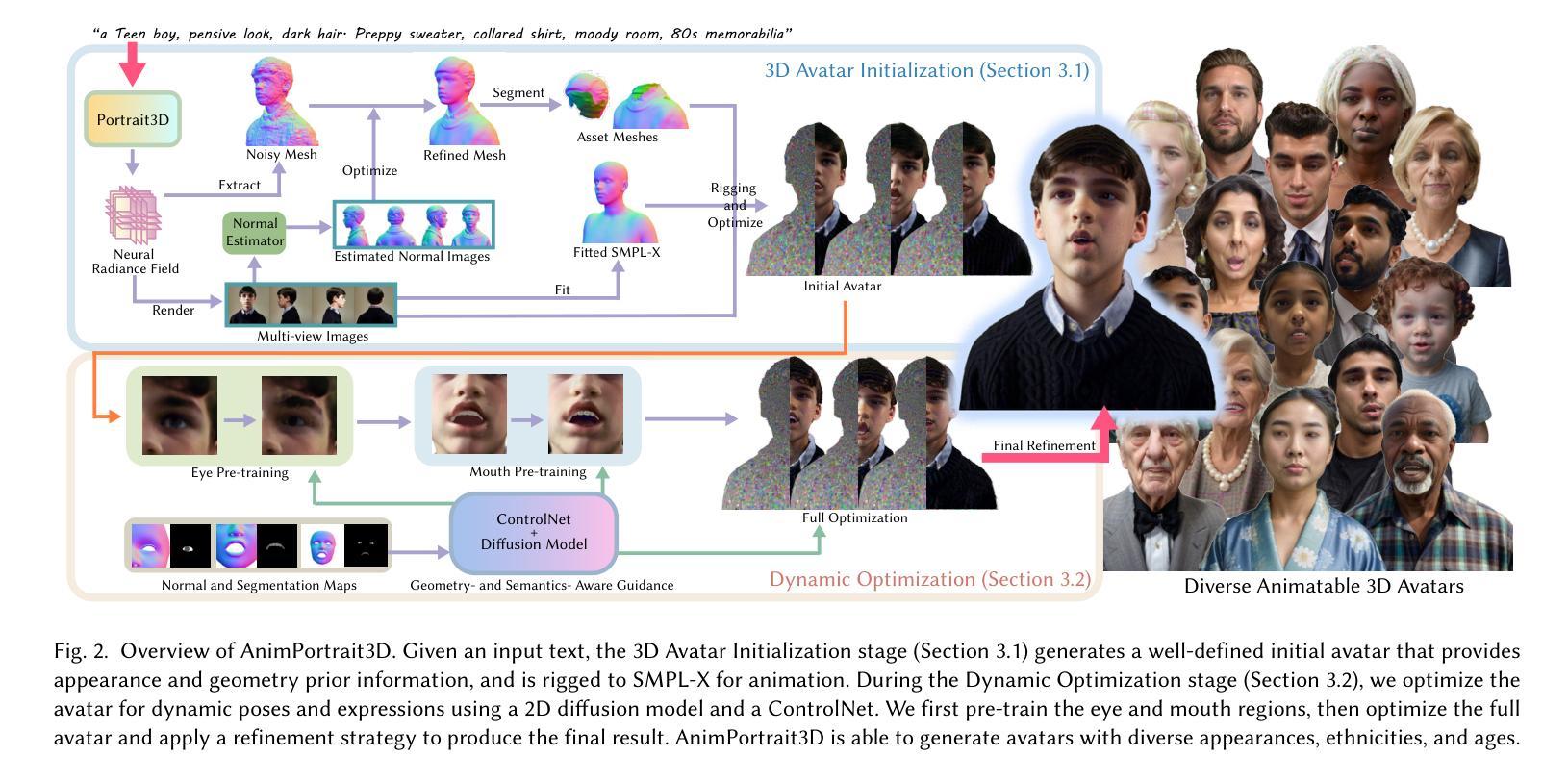
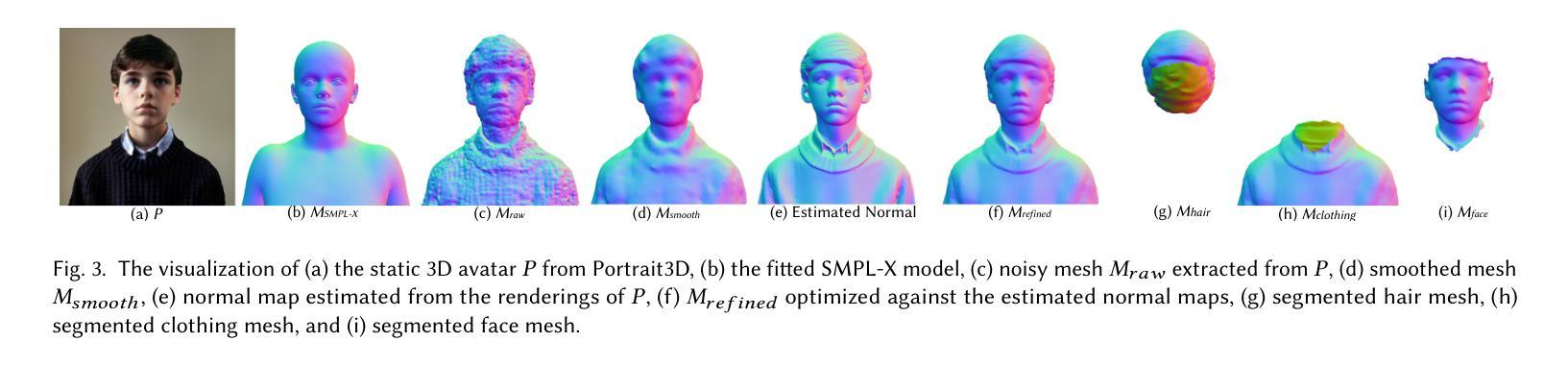
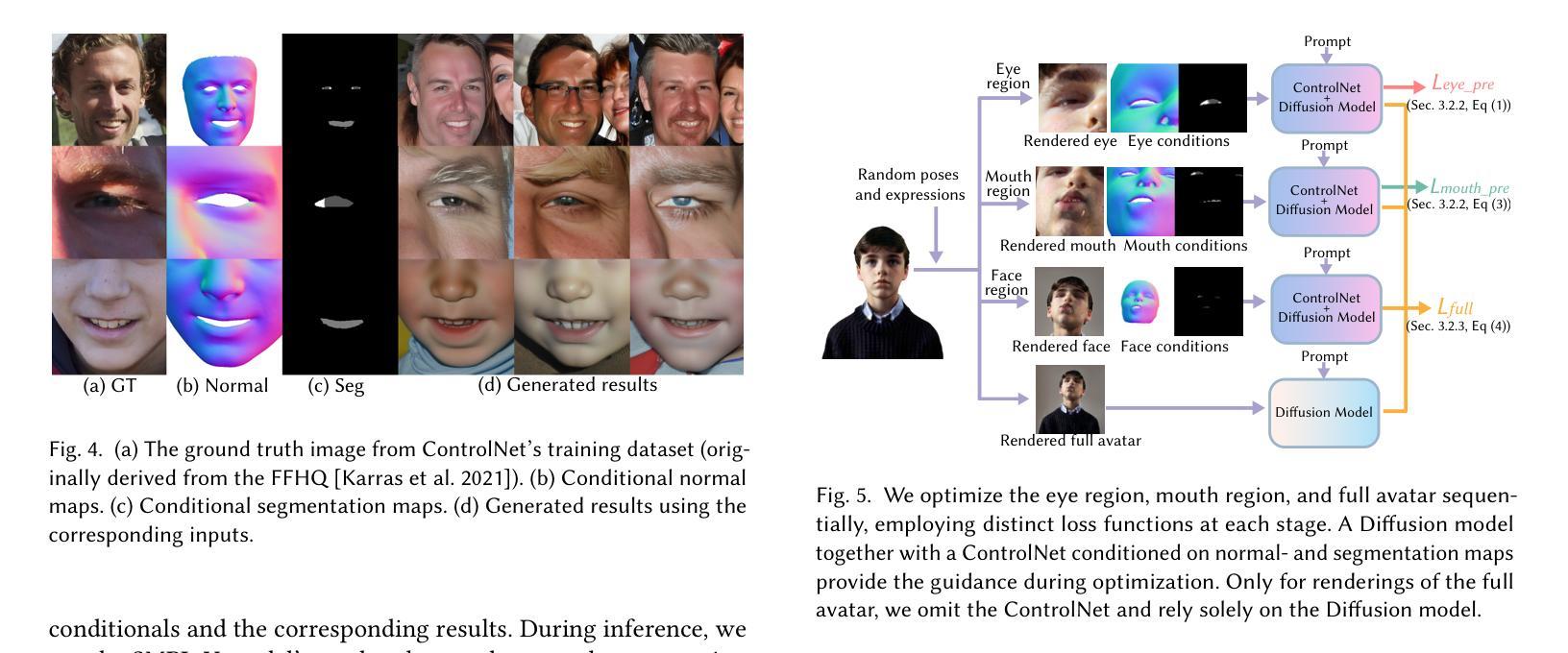
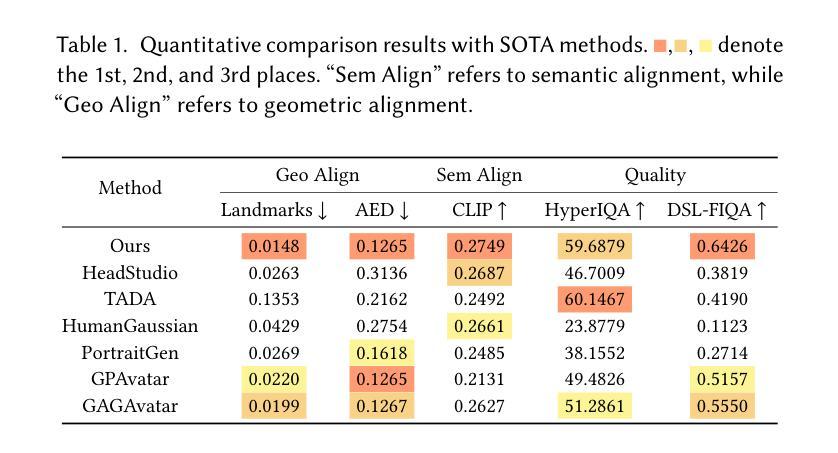