⚠️ 以下所有内容总结都来自于 大语言模型的能力,如有错误,仅供参考,谨慎使用
🔴 请注意:千万不要用于严肃的学术场景,只能用于论文阅读前的初筛!
💗 如果您觉得我们的项目对您有帮助 ChatPaperFree ,还请您给我们一些鼓励!⭐️ HuggingFace免费体验
2025-04-24 更新
Text-based Animatable 3D Avatars with Morphable Model Alignment
Authors:Yiqian Wu, Malte Prinzler, Xiaogang Jin, Siyu Tang
The generation of high-quality, animatable 3D head avatars from text has enormous potential in content creation applications such as games, movies, and embodied virtual assistants. Current text-to-3D generation methods typically combine parametric head models with 2D diffusion models using score distillation sampling to produce 3D-consistent results. However, they struggle to synthesize realistic details and suffer from misalignments between the appearance and the driving parametric model, resulting in unnatural animation results. We discovered that these limitations stem from ambiguities in the 2D diffusion predictions during 3D avatar distillation, specifically: i) the avatar’s appearance and geometry is underconstrained by the text input, and ii) the semantic alignment between the predictions and the parametric head model is insufficient because the diffusion model alone cannot incorporate information from the parametric model. In this work, we propose a novel framework, AnimPortrait3D, for text-based realistic animatable 3DGS avatar generation with morphable model alignment, and introduce two key strategies to address these challenges. First, we tackle appearance and geometry ambiguities by utilizing prior information from a pretrained text-to-3D model to initialize a 3D avatar with robust appearance, geometry, and rigging relationships to the morphable model. Second, we refine the initial 3D avatar for dynamic expressions using a ControlNet that is conditioned on semantic and normal maps of the morphable model to ensure accurate alignment. As a result, our method outperforms existing approaches in terms of synthesis quality, alignment, and animation fidelity. Our experiments show that the proposed method advances the state of the art in text-based, animatable 3D head avatar generation.
从文本生成高质量、可动画的3D头像在内容创建应用(如游戏、电影和虚拟助手)中具有巨大潜力。当前的文本到3D生成方法通常结合参数化头部模型与2D扩散模型,使用分数蒸馏采样以产生一致的3D结果。然而,它们在合成现实细节方面遇到困难,并且在外观和驱动参数模型之间存在不匹配,导致动画结果不自然。我们发现这些局限性源于3D头像蒸馏期间2D扩散预测的模糊性,具体表现为:i) 头像的外观和几何结构受到文本输入的约束较少;ii) 预测与参数化头部模型之间的语义对齐不足,因为单独的扩散模型无法融入参数模型的信息。在这项工作中,我们提出了一种用于基于文本的现实主义可动画3DGS头像生成的新型框架AnimPortrait3D,并引入了两个关键策略来解决这些挑战。首先,我们通过利用预训练的文本到3D模型的先验信息来解决外观和几何模糊性问题,以初始化具有稳健外观、几何结构和与可变形模型的骨骼关系的3D头像。其次,我们使用条件控制网(ControlNet)对初始的3D头像进行微调,以生成动态表情,该网络以可变形模型的语义和法线图作为条件,以确保精确对齐。因此,我们的方法在合成质量、对齐和动画逼真度方面优于现有方法。实验表明,所提出的方法在基于文本的动画3D头像生成方面达到了最新水平。
论文及项目相关链接
摘要
本文探讨文本驱动的高质量动画三维头像生成技术,在内容创作领域如游戏、电影和虚拟助手中有巨大潜力。针对现有方法合成细节不真实、参数模型驱动与外观不匹配的问题,提出一种基于文本的真实动画三维头像生成框架AnimPortrait3D,并引入两种关键策略解决挑战:利用预训练文本至三维模型的先验信息解决外观与几何体模糊问题,使用ControlNet细化三维头像动态表情,确保与可变形模型的语义和法线地图对齐。此方法在合成质量、对齐和动画保真度上优于现有方法。
关键见解
- 文本驱动的高质量动画三维头像生成在内容创作领域有巨大潜力,如游戏、电影和虚拟助手。
- 当前方法结合参数化头部模型和二维扩散模型,但合成细节不真实,存在模型驱动与外观不匹配的问题。
- 问题的根源在于二维扩散预测中的模糊性,特别是文本输入的约束不足以及预测与参数化头部模型之间的语义对齐不足。
- 引入AnimPortrait3D框架,利用预训练文本至三维模型的先验信息解决模糊性,初始化三维头像,建立稳健的外观、几何和可变形模型的关系。
- 使用ControlNet细化三维头像,基于可变形模型的语义和法线地图保证准确对齐,提高动画效果。
- 所提方法在提高合成质量、对齐和动画保真度上超越现有技术。
点此查看论文截图
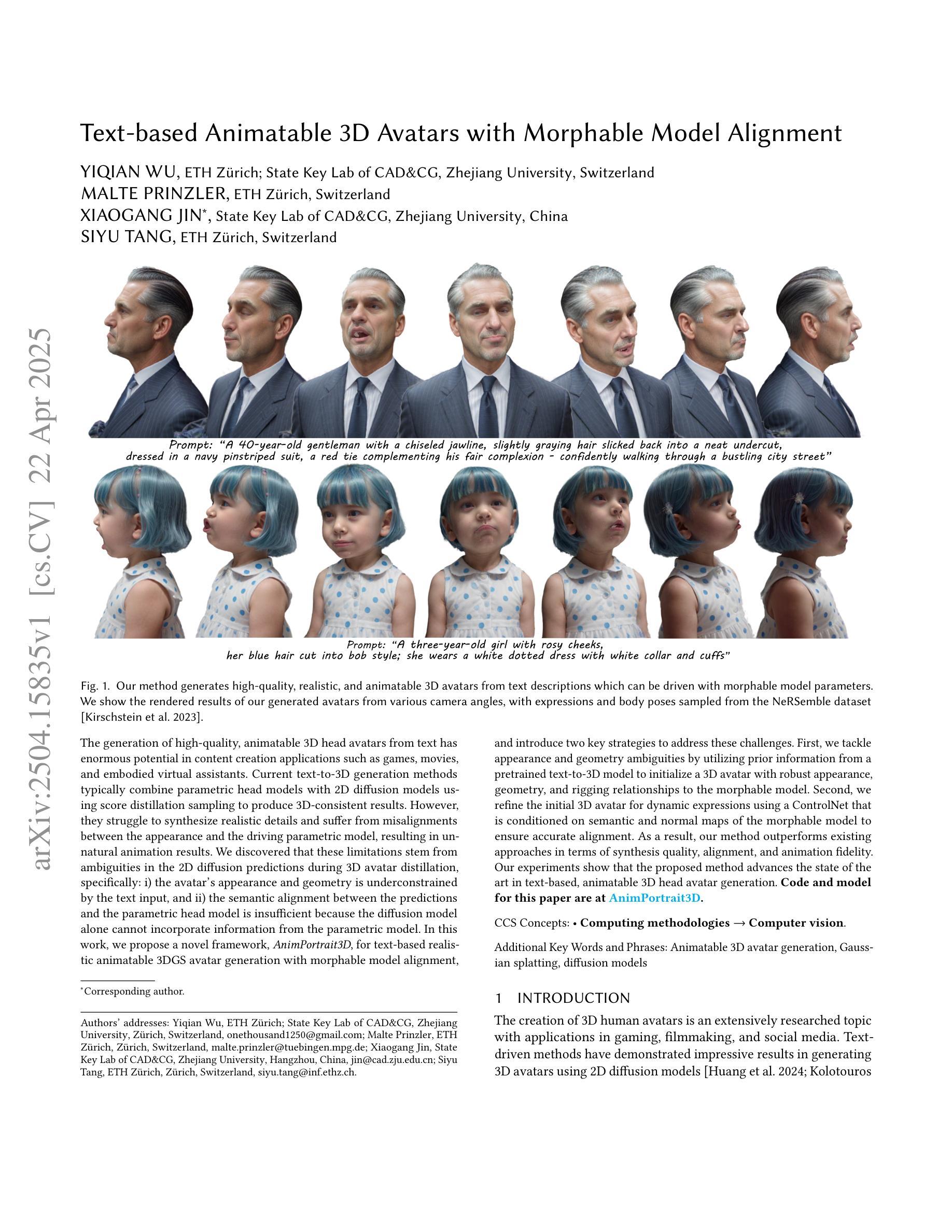
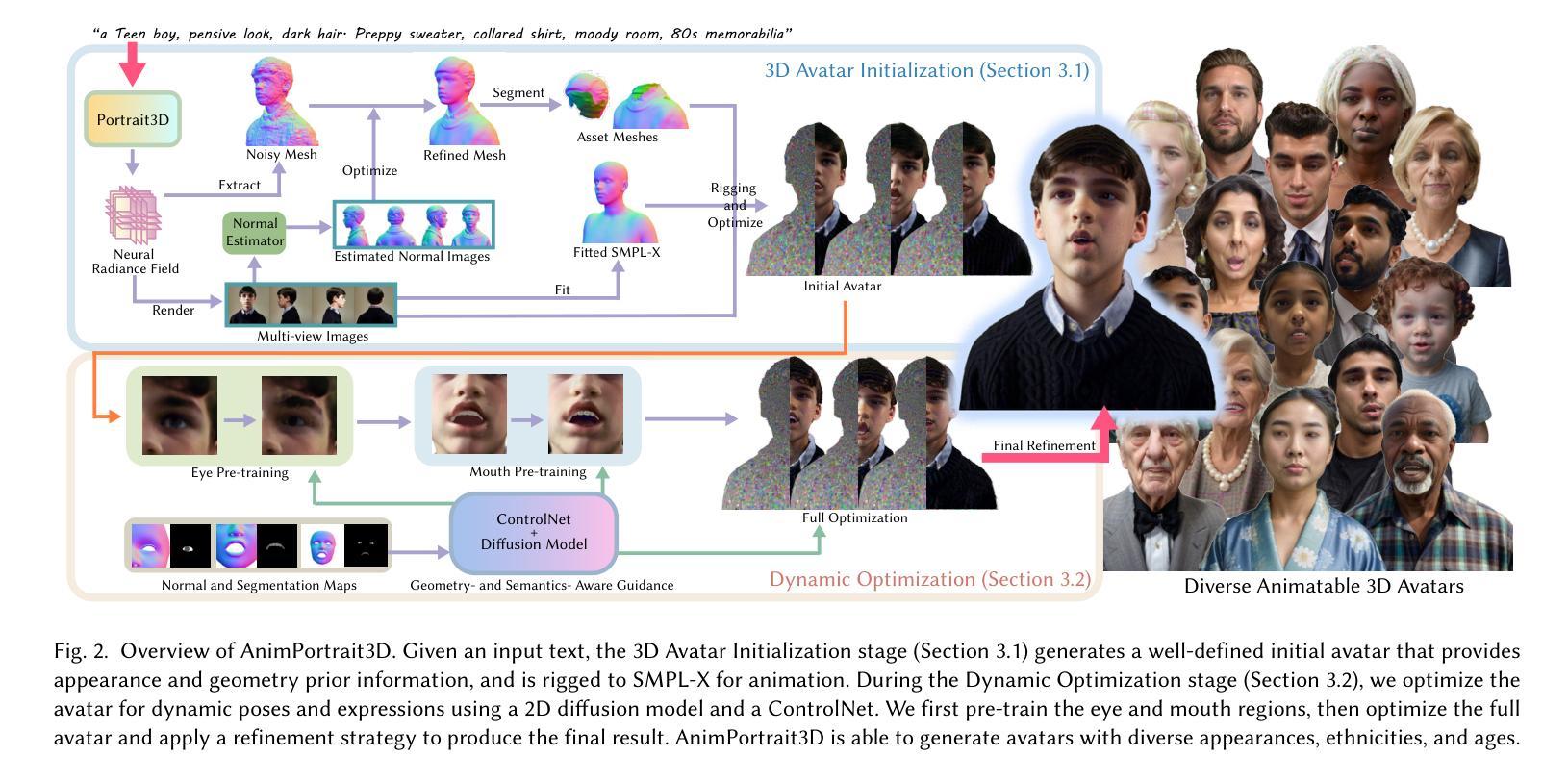
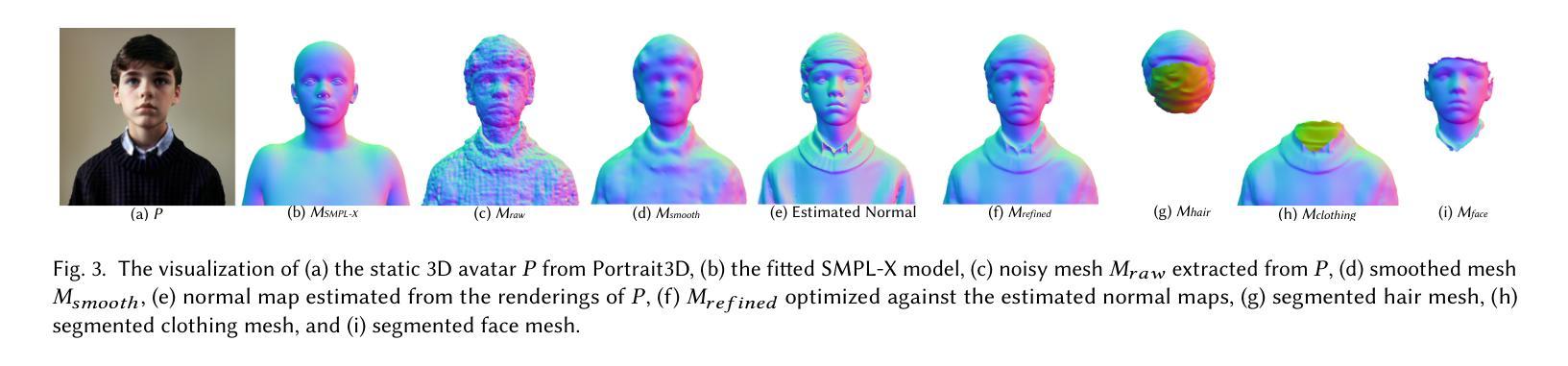
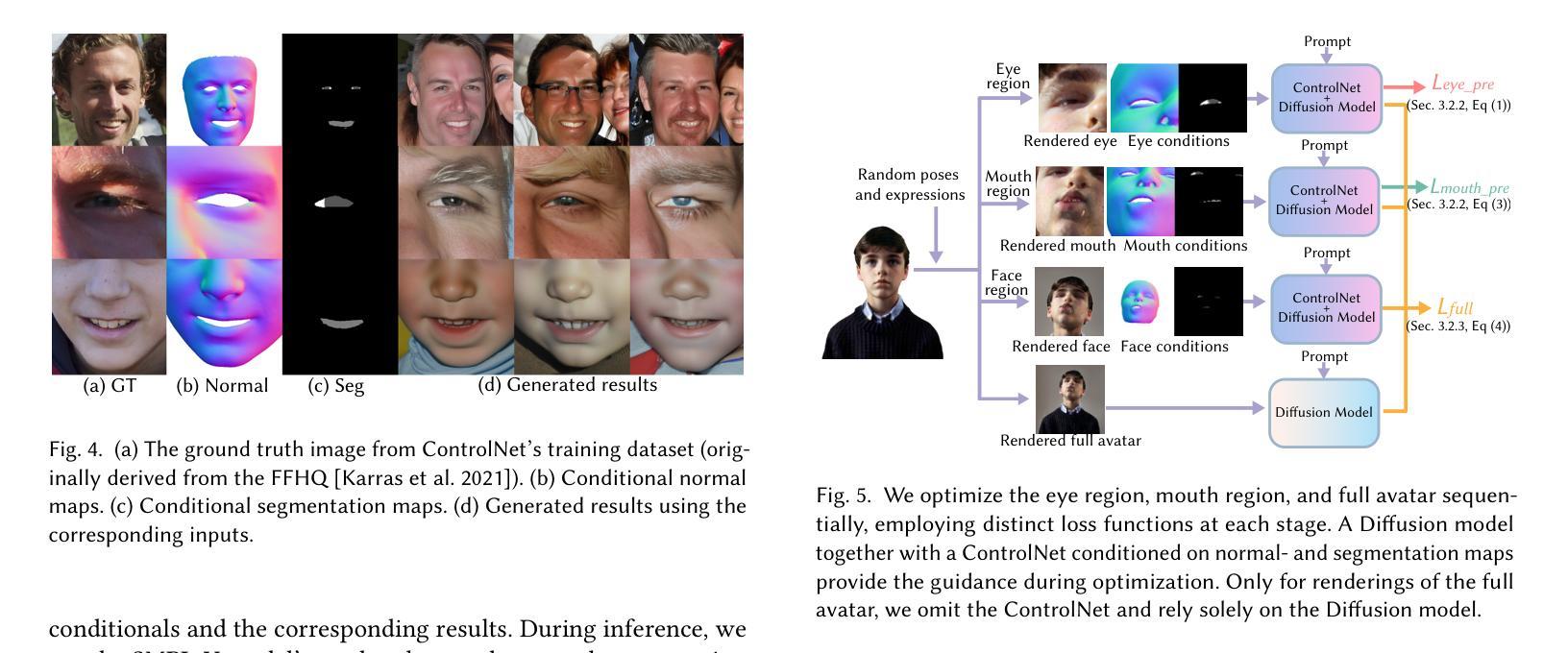
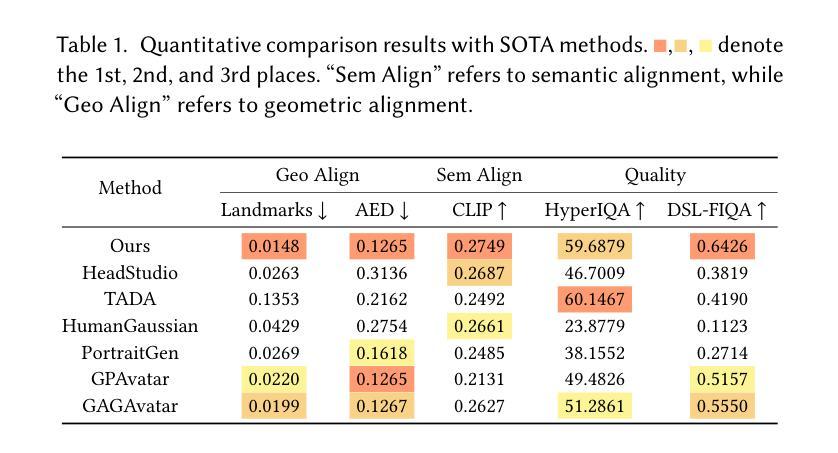
HEMGS: A Hybrid Entropy Model for 3D Gaussian Splatting Data Compression
Authors:Lei Liu, Zhenghao Chen, Wei Jiang, Wei Wang, Dong Xu
In this work, we propose a novel compression framework for 3D Gaussian Splatting (3DGS) data. Building on anchor-based 3DGS methodologies, our approach compresses all attributes within each anchor by introducing a novel Hybrid Entropy Model for 3D Gaussian Splatting (HEMGS) to achieve hybrid lossy-lossless compression. It consists of three main components: a variable-rate predictor, a hyperprior network, and an autoregressive network. First, unlike previous methods that adopt multiple models to achieve multi-rate lossy compression, thereby increasing training overhead, our variable-rate predictor enables variable-rate compression with a single model and a hyperparameter $\lambda$ by producing a learned Quantization Step feature for versatile lossy compression. Second, to improve lossless compression, the hyperprior network captures both scene-agnostic and scene-specific features to generate a prior feature, while the autoregressive network employs an adaptive context selection algorithm with flexible receptive fields to produce a contextual feature. By integrating these two features, HEMGS can accurately estimate the distribution of the current coding element within each attribute, enabling improved entropy coding and reduced storage. We integrate HEMGS into a compression framework, and experimental results on four benchmarks indicate that HEMGS achieves about a 40% average reduction in size while maintaining rendering quality over baseline methods and achieving state-of-the-art compression results.
在这项工作中,我们针对3D高斯混合(3DGS)数据提出了一种新颖的压缩框架。我们的方法基于锚点(anchor-based)的3DGS方法,通过引入用于3D高斯混合的混合熵模型(HEMGS),对锚点内的所有属性进行压缩,以实现混合有损-无损压缩。它主要包括三个主要组件:可变率预测器、超先验网络和自回归网络。首先,与先前采用多个模型实现多速率有损压缩的方法不同,这些方法增加了训练开销,我们的可变率预测器使用单个模型和超参数λ,通过生成学习的量化步幅特征来实现可变率压缩,用于通用的有损压缩。其次,为了改进无损压缩,超先验网络捕获场景无关和场景特定的特征以生成先验特征,同时自回归网络采用具有灵活感受野的自适应上下文选择算法来生成上下文特征。通过集成这两个特征,HEMGS可以准确估计每个属性内当前编码元素的分布,从而提高熵编码并减少存储。我们将HEMGS集成到压缩框架中,四项基准测试的实验结果表明,HEMGS在保持渲染质量的同时,平均大小减少了约40%,并实现了最先进的压缩结果。
论文及项目相关链接
Summary
该研究提出了一种针对3D高斯喷溅(3DGS)数据的新型压缩框架。该框架基于锚点技术的3DGS方法,通过引入混合熵模型(HEMGS)来压缩每个锚点的所有属性,实现了混合有损-无损压缩。主要组件包括可变率预测器、超先验网络和自回归网络。该研究实现了单一模型下的可变率压缩,并通过超参数λ产生学习的量化步长特征,以进行多功能有损压缩。超先验网络捕获场景无关和场景特定特征以生成先验特征,而自回归网络采用自适应上下文选择算法和灵活的感受野来产生上下文特征。这些特征集成使HEMGS能够准确估计每个属性的当前编码元素的分布,从而提高熵编码的效率和存储的减少。实验结果表明,与基准方法和最新压缩技术相比,HEMGS在保持渲染质量的同时,平均减少了约40%的大小。
Key Takeaways
- 提出了针对3D高斯喷溅(3DGS)数据的新型压缩框架。
- 引入混合熵模型(HEMGS)以压缩每个锚点的所有属性,实现混合有损-无损压缩。
- 通过单一模型实现可变率压缩,并通过超参数调整量化步长以实现多功能有损压缩。
- 超先验网络用于捕获场景无关和场景特定特征,生成先验特征以改善无损压缩。
- 自回归网络采用自适应上下文选择算法和灵活感受野,产生上下文特征以提高熵编码效率。
- 集成HEMGS的压缩框架在四个基准测试上实现了平均约40%的大小减少,同时保持渲染质量。
点此查看论文截图
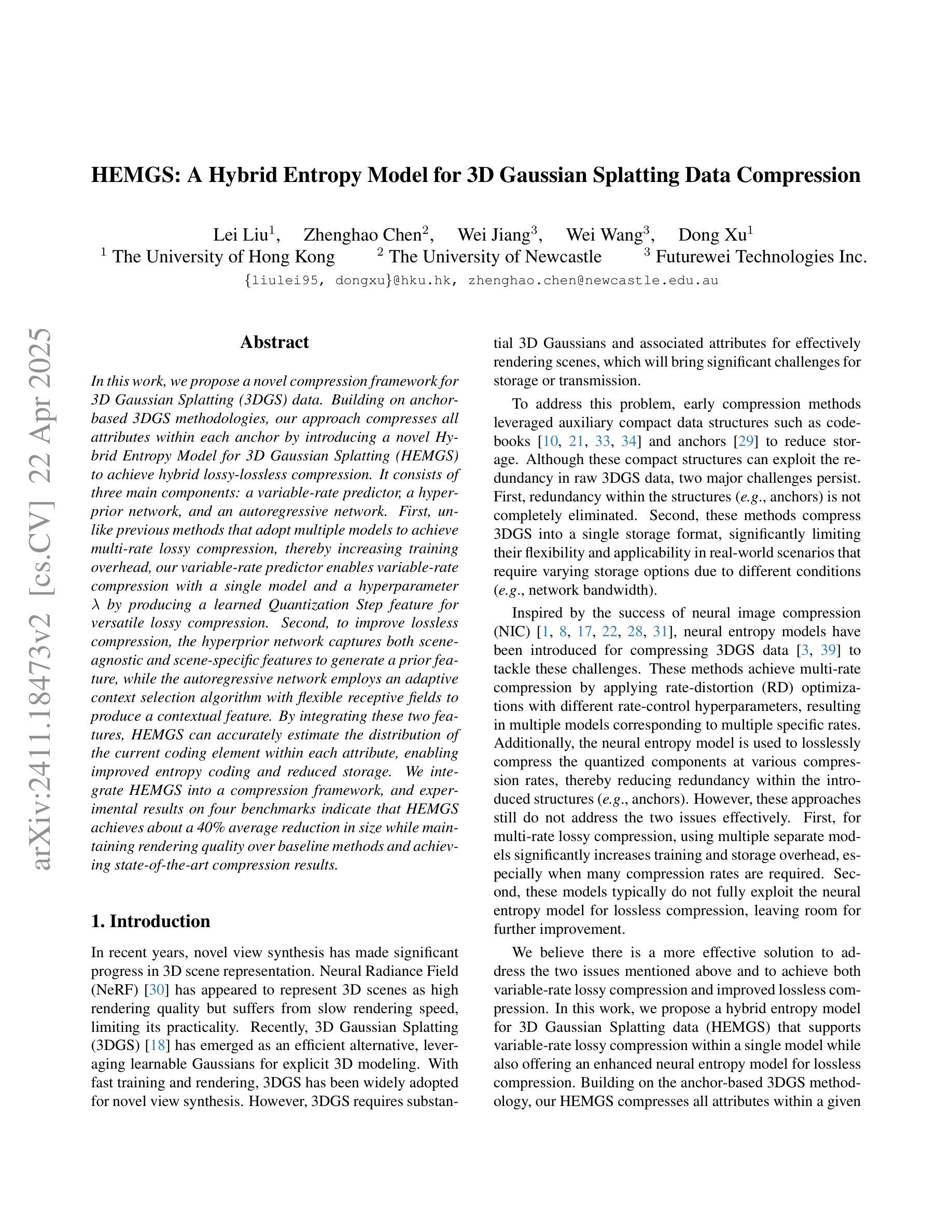
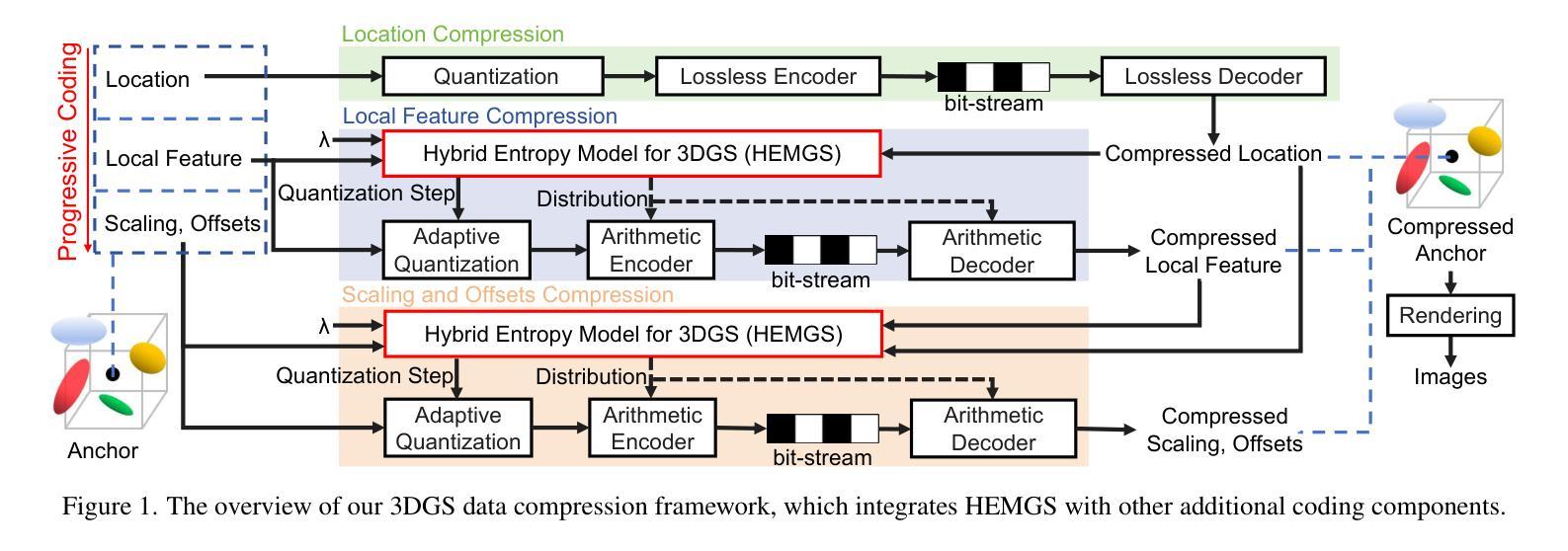
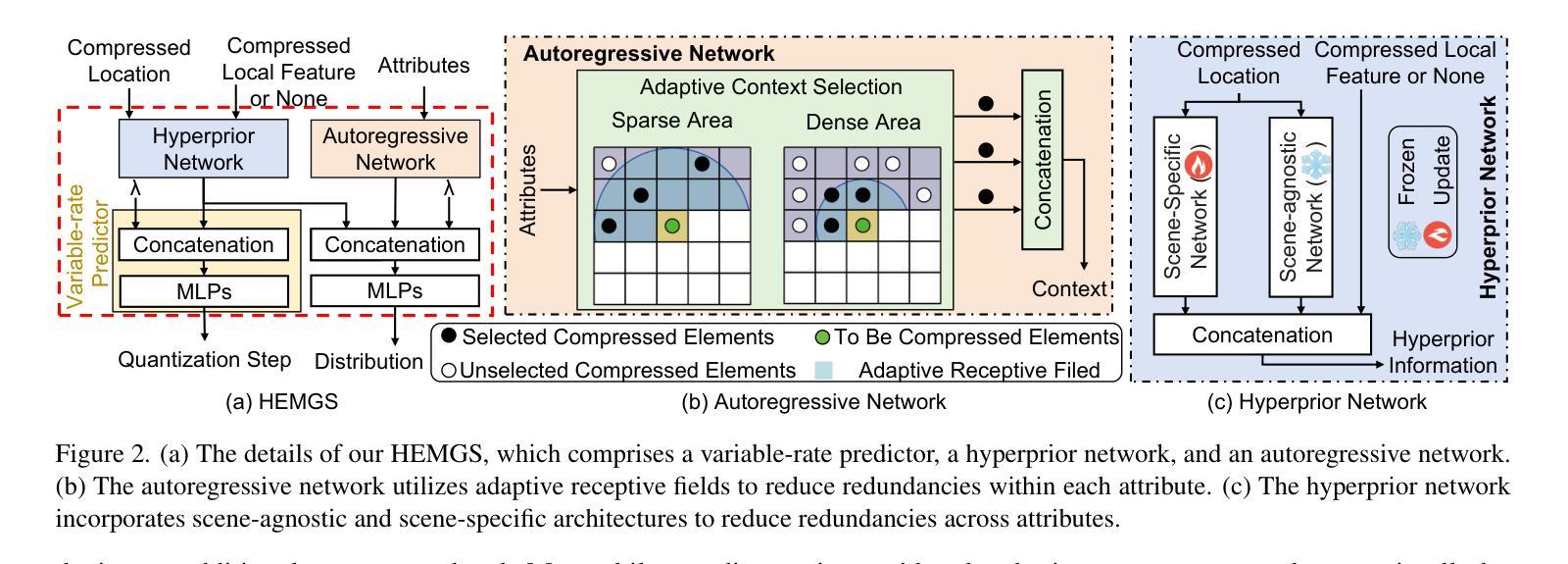
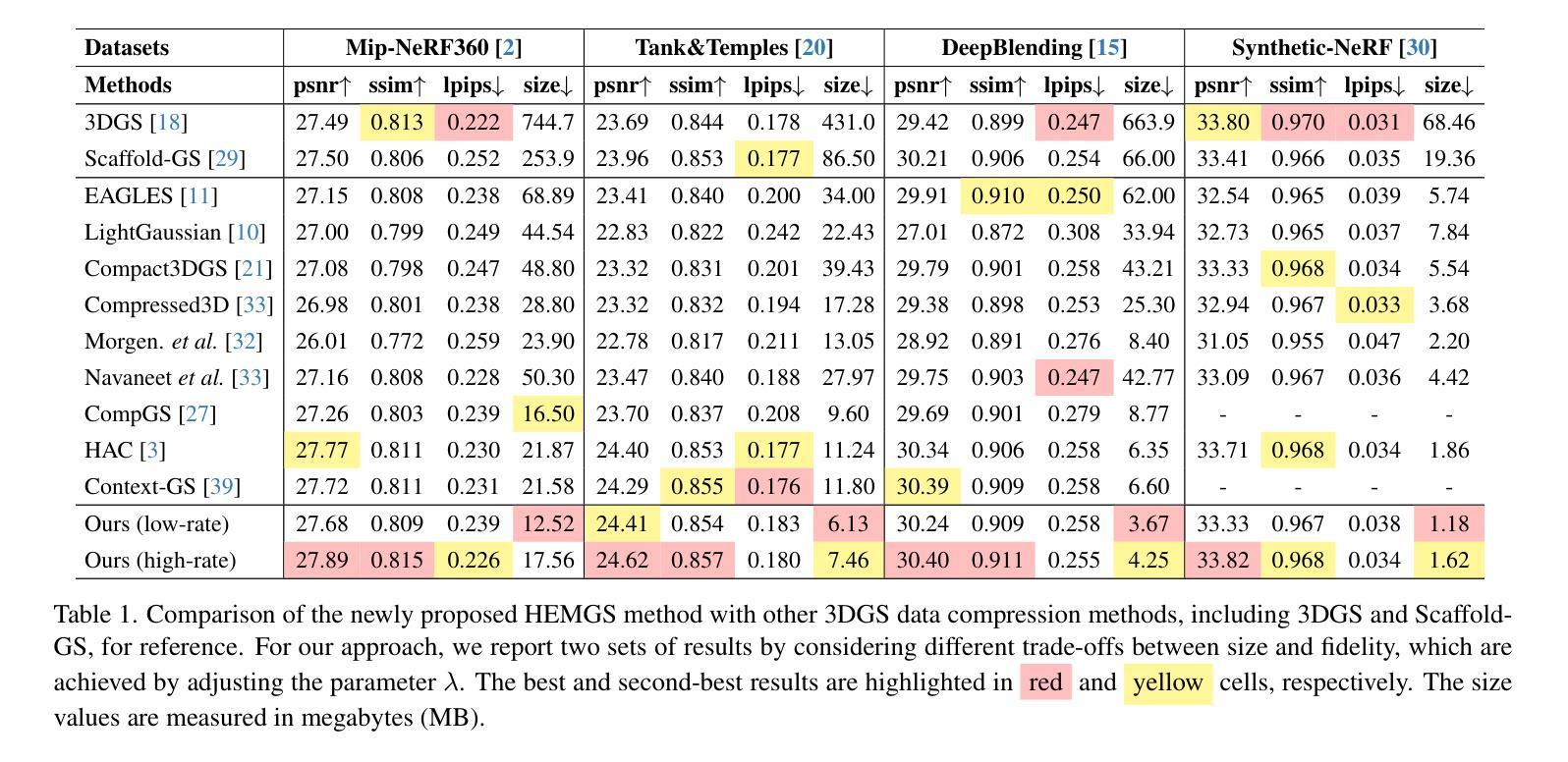
ThermalGaussian: Thermal 3D Gaussian Splatting
Authors:Rongfeng Lu, Hangyu Chen, Zunjie Zhu, Yuhang Qin, Ming Lu, Le Zhang, Chenggang Yan, Anke Xue
Thermography is especially valuable for the military and other users of surveillance cameras. Some recent methods based on Neural Radiance Fields (NeRF) are proposed to reconstruct the thermal scenes in 3D from a set of thermal and RGB images. However, unlike NeRF, 3D Gaussian splatting (3DGS) prevails due to its rapid training and real-time rendering. In this work, we propose ThermalGaussian, the first thermal 3DGS approach capable of rendering high-quality images in RGB and thermal modalities. We first calibrate the RGB camera and the thermal camera to ensure that both modalities are accurately aligned. Subsequently, we use the registered images to learn the multimodal 3D Gaussians. To prevent the overfitting of any single modality, we introduce several multimodal regularization constraints. We also develop smoothing constraints tailored to the physical characteristics of the thermal modality. Besides, we contribute a real-world dataset named RGBT-Scenes, captured by a hand-hold thermal-infrared camera, facilitating future research on thermal scene reconstruction. We conduct comprehensive experiments to show that ThermalGaussian achieves photorealistic rendering of thermal images and improves the rendering quality of RGB images. With the proposed multimodal regularization constraints, we also reduced the model’s storage cost by 90%. Our project page is at https://thermalgaussian.github.io/.
热成像技术对于军事和其他监控相机使用者来说尤其有价值。最近有一些基于神经辐射场(NeRF)的方法被提出来从一组热图和RGB图像中重建三维热场景。然而,不同于NeRF,3D高斯拼贴(3DGS)由于其快速训练和实时渲染而更受欢迎。在这项工作中,我们提出了ThermalGaussian,这是第一种能够在RGB和热成像模式下呈现高质量图像的热3DGS方法。我们首先校准RGB相机和热成像相机,以确保两种模式能够准确对齐。随后,我们使用已注册的图像来学习多模态三维高斯分布。为了防止单一模态过度拟合,我们引入了多种多模态正则化约束。我们还针对热成像的物理特性开发了定制的光滑约束。此外,我们还贡献了一个名为RGBT-Scenes的真实世界数据集,该数据集由手持热红外相机拍摄,有助于未来热场景重建的研究。我们进行了全面的实验,以证明ThermalGaussian能够实现热图像的光照现实渲染,并提高了RGB图像的渲染质量。通过提出的多模态正则化约束,我们还降低了模型90%的存储成本。我们的项目页面是:[https://thermalgaussian.github.io/] 。
论文及项目相关链接
PDF 10 pages, 7 figures
Summary
本文提出了基于热成像技术的3D高斯喷射(ThermalGaussian)方法,利用RGB和红外图像重建三维场景。该方法快速训练,能实时渲染高质量图像,并具有模态间的正则化约束和针对热模态物理特性的平滑约束。此外,还贡献了一个名为RGBT-Scenes的真实世界数据集,促进了热成像场景重建的研究。
Key Takeaways
- 介绍了热成像技术在军事和其他监控领域的重要性。
- 基于神经网络辐射场(NeRF)的方法被用于重建三维热场景,但3D高斯喷射(3DGS)因其快速训练和实时渲染而更受欢迎。
- 提出了ThermalGaussian方法,是首个能渲染RGB和红外模态高质量图像的热成像3DGS方法。
- 通过校准RGB相机和红外相机,确保两种模态的准确对齐。
- 引入多模态正则化约束,防止单一模态的过拟合。
- 针对热模态的物理特性开发了平滑约束。
点此查看论文截图
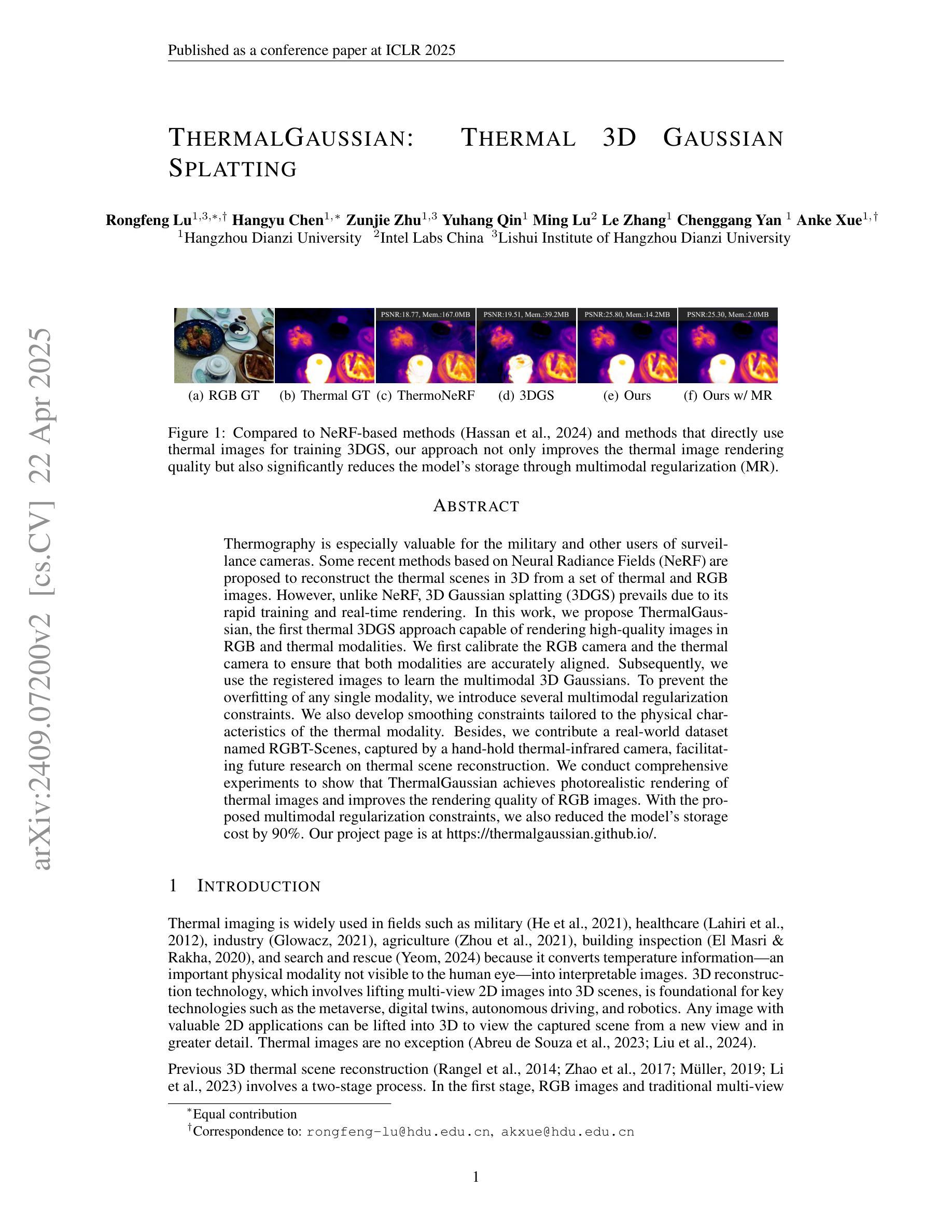
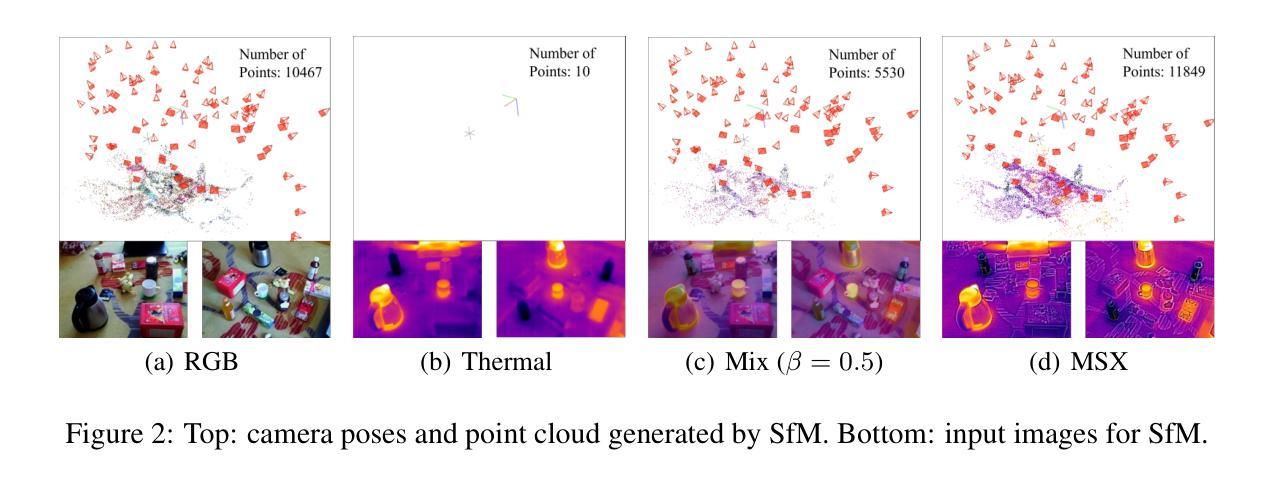
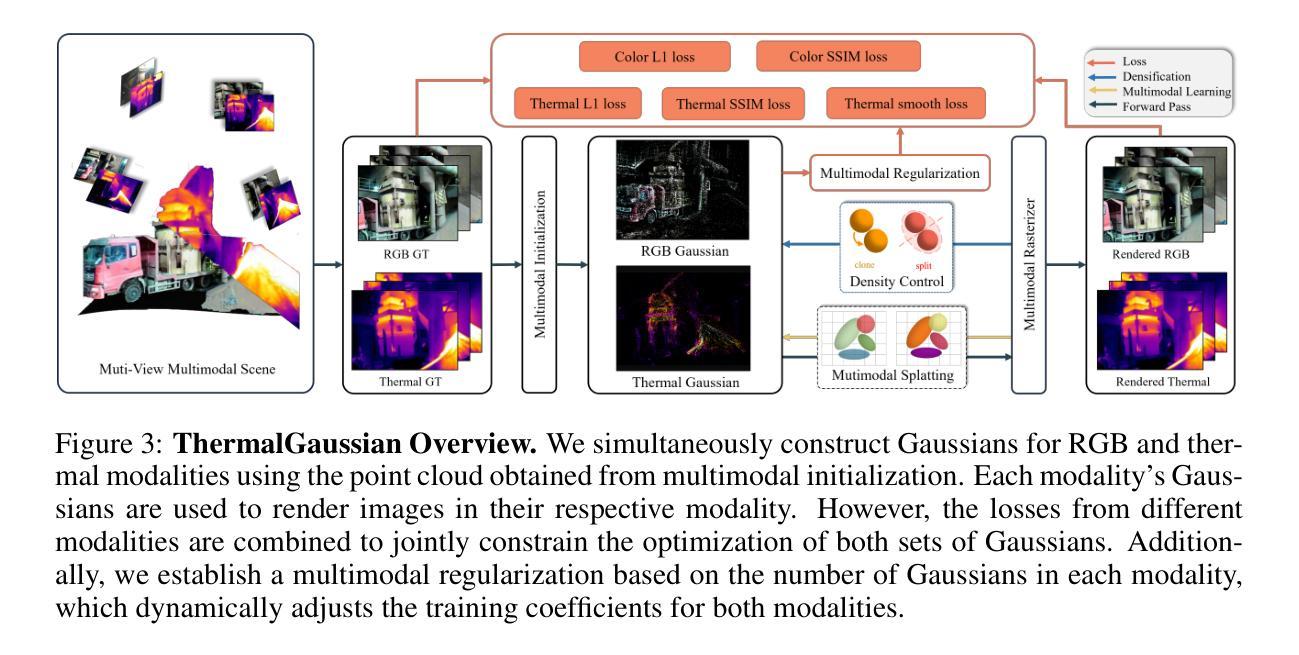
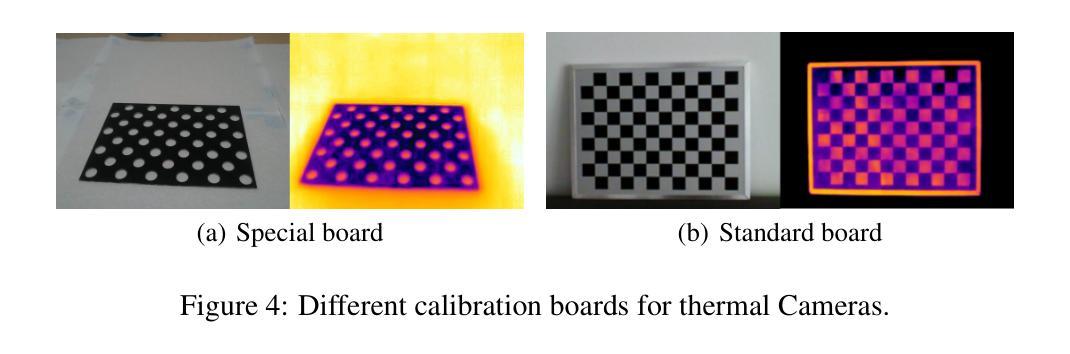
3DGR-CT: Sparse-View CT Reconstruction with a 3D Gaussian Representation
Authors:Yingtai Li, Xueming Fu, Han Li, Shang Zhao, Ruiyang Jin, S. Kevin Zhou
Sparse-view computed tomography (CT) reduces radiation exposure by acquiring fewer projections, making it a valuable tool in clinical scenarios where low-dose radiation is essential. However, this often results in increased noise and artifacts due to limited data. In this paper we propose a novel 3D Gaussian representation (3DGR) based method for sparse-view CT reconstruction. Inspired by recent success in novel view synthesis driven by 3D Gaussian splatting, we leverage the efficiency and expressiveness of 3D Gaussian representation as an alternative to implicit neural representation. To unleash the potential of 3DGR for CT imaging scenario, we propose two key innovations: (i) FBP-image-guided Guassian initialization and (ii) efficient integration with a differentiable CT projector. Extensive experiments and ablations on diverse datasets demonstrate the proposed 3DGR-CT consistently outperforms state-of-the-art counterpart methods, achieving higher reconstruction accuracy with faster convergence. Furthermore, we showcase the potential of 3DGR-CT for real-time physical simulation, which holds important clinical applications while challenging for implicit neural representations.
稀疏视图计算机断层扫描(CT)通过获取较少的投影来减少辐射暴露,使其成为在临床场景中低剂量辐射至关重要的有价值工具。然而,这通常由于数据有限而导致噪声和伪影增加。在本文中,我们提出了一种基于新型三维高斯表示(3DGR)的稀疏视图CT重建方法。受最近由三维高斯喷射驱动的新型视图合成的成功启发,我们利用三维高斯表示的效率和表现力,作为一种替代隐神经表示的方法。为了释放3DGR在CT成像场景中的潜力,我们提出了两项关键创新:(i)FBP图像引导的Guassian初始化,以及(ii)与可微分CT投影仪的有效集成。在多种数据集上的广泛实验和剔除研究证明,所提出的3DGR-CT持续优于最先进的对比方法,以更高的重建精度和更快的收敛速度实现了优异表现。此外,我们展示了3DGR-CT在实时物理模拟中的潜力,这在临床应用中具有重要意义,而对隐神经表示来说则是一项挑战。
论文及项目相关链接
Summary
本文研究了稀疏视角计算机断层扫描(CT)技术中的噪声和伪影问题。为解决此问题,提出了一种基于三维高斯表示(3DGR)的方法用于稀疏视角CT重建。该方法结合了FBP图像引导和可微CT投影器的效率,实现更高重建精度和更快收敛速度。同时展示了该技术在实时物理模拟方面的潜力,具有临床应用的巨大价值。
Key Takeaways
- 稀疏视角CT技术通过减少投影数量降低辐射暴露,但会导致噪声和伪影问题。
- 提出了基于三维高斯表示(3DGR)的稀疏视角CT重建方法。
- 借助FBP图像引导高斯初始化,提高重建准确性。
- 与可微CT投影器结合,实现高效集成。
- 该方法相较于现有技术,展现出更高的重建精度和更快的收敛速度。
- 展示了该方法在实时物理模拟方面的潜力,适用于临床应用。
点此查看论文截图
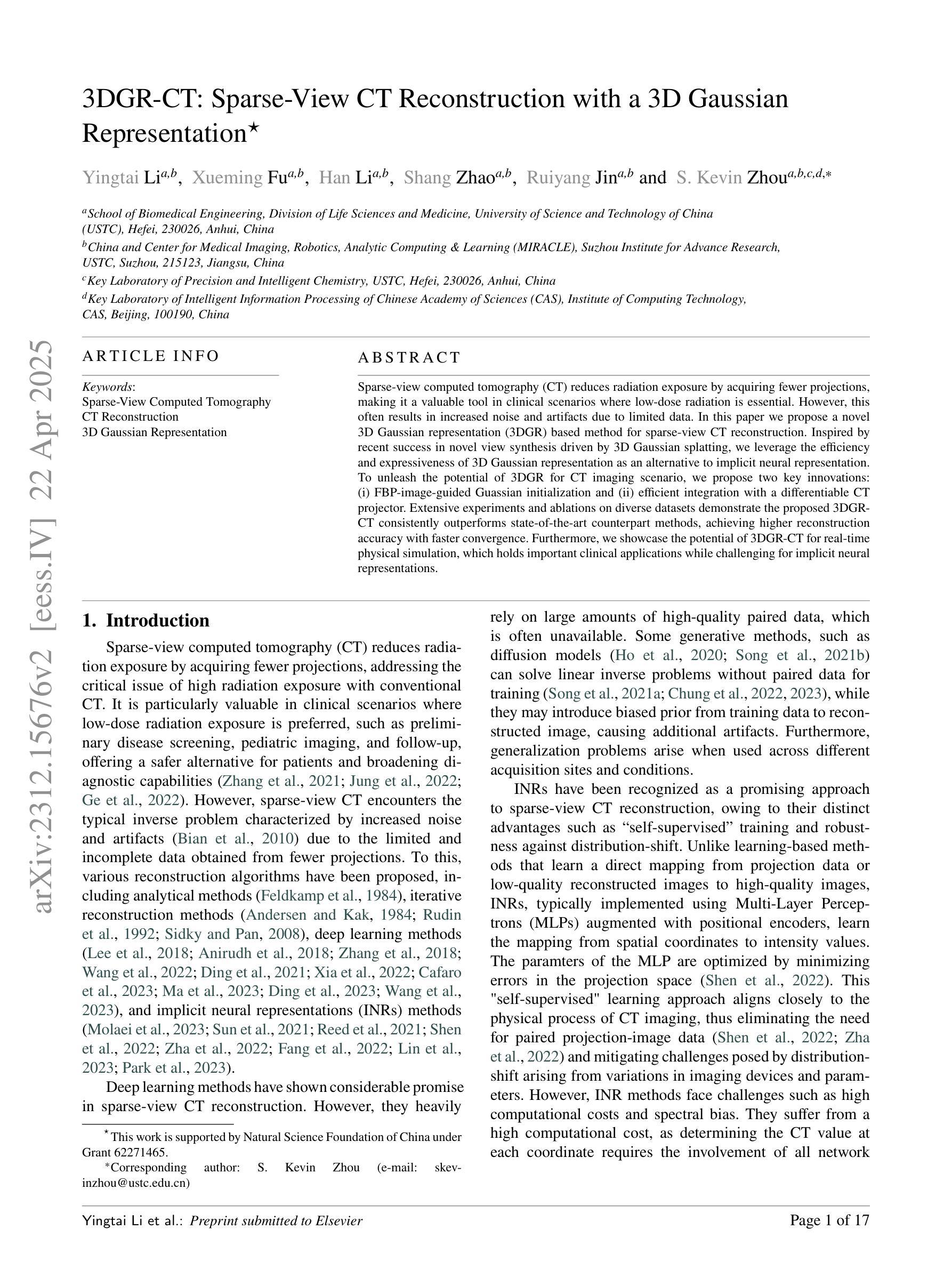
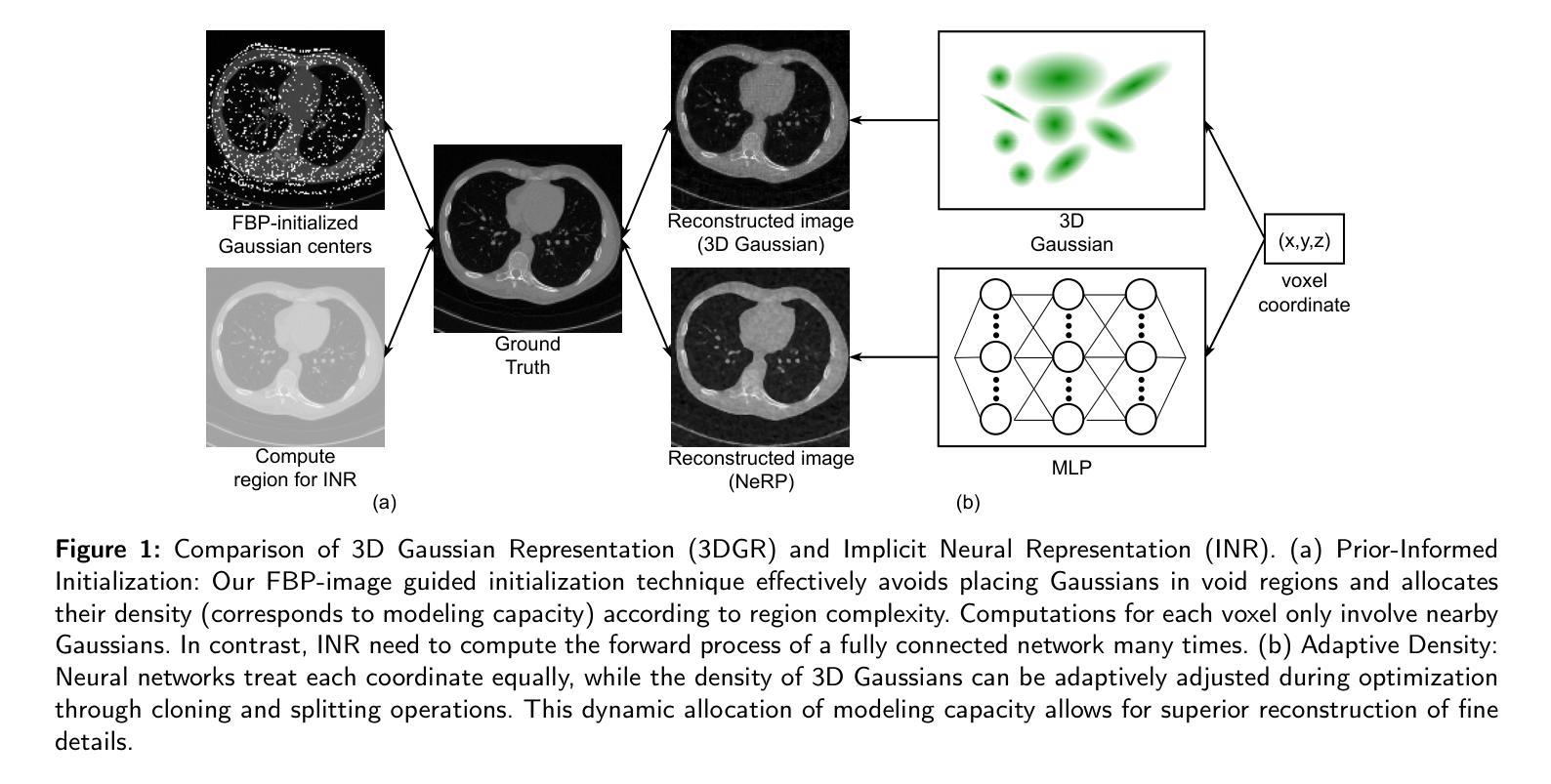
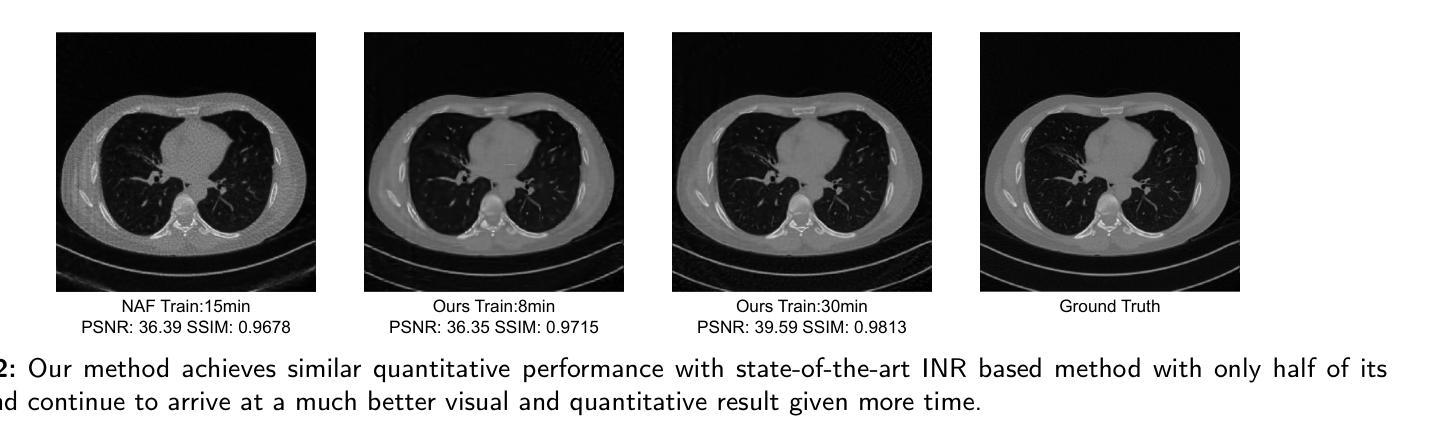
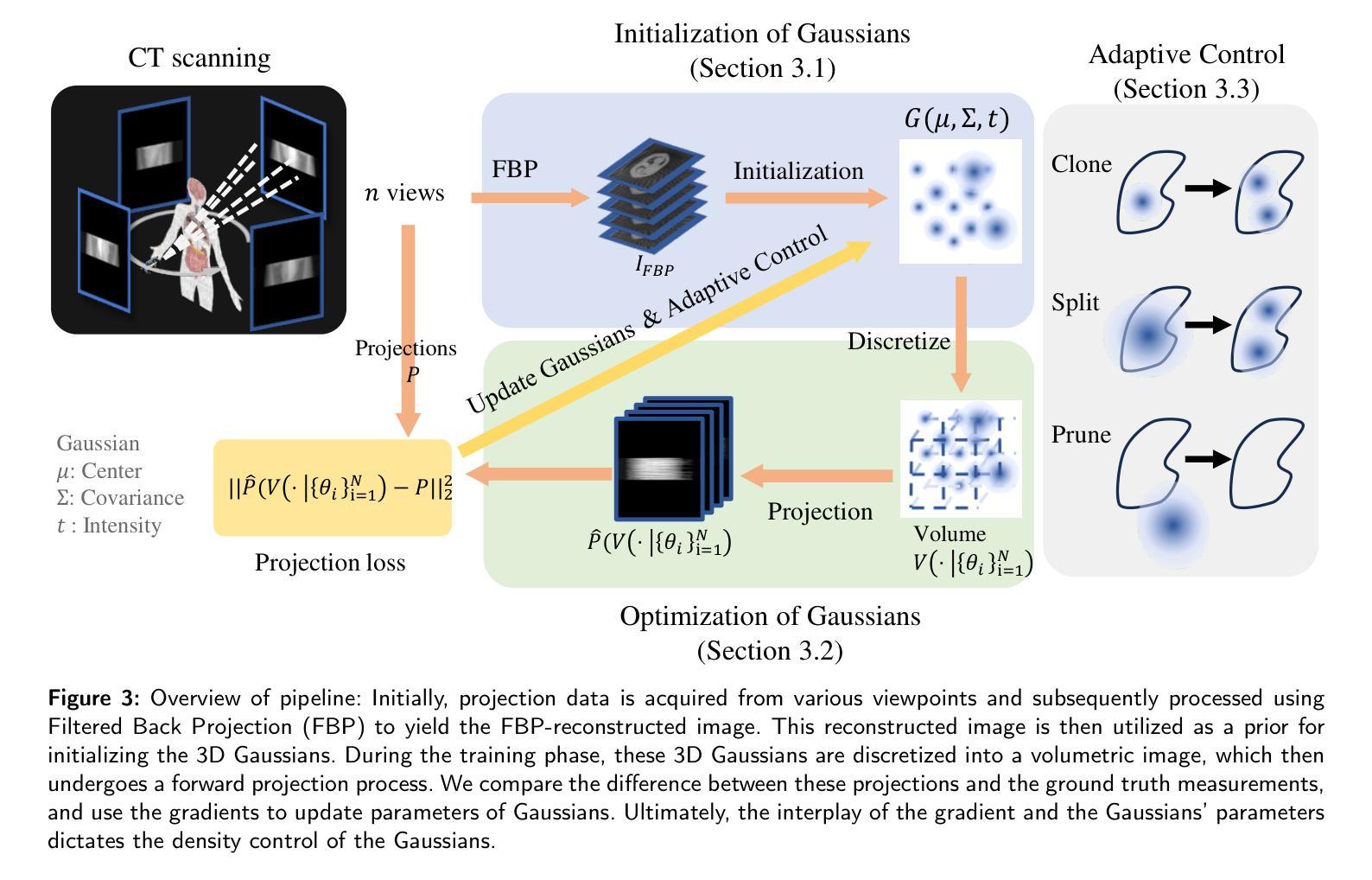