⚠️ 以下所有内容总结都来自于 大语言模型的能力,如有错误,仅供参考,谨慎使用
🔴 请注意:千万不要用于严肃的学术场景,只能用于论文阅读前的初筛!
💗 如果您觉得我们的项目对您有帮助 ChatPaperFree ,还请您给我们一些鼓励!⭐️ HuggingFace免费体验
2025-04-24 更新
Rotational ultrasound and photoacoustic tomography of the human body
Authors:Yang Zhang, Shuai Na, Jonathan J. Russin, Karteekeya Sastry, Li Lin, Junfu Zheng, Yilin Luo, Xin Tong, Yujin An, Peng Hu, Konstantin Maslov, Tze-Woei Tan, Charles Y. Liu, Lihong V. Wang
Imaging the human body’s morphological and angiographic information is essential for diagnosing, monitoring, and treating medical conditions. Ultrasonography performs the morphological assessment of the soft tissue based on acoustic impedance variations, whereas photoacoustic tomography (PAT) can visualize blood vessels based on intrinsic hemoglobin absorption. Three-dimensional (3D) panoramic imaging of the vasculature is generally not practical in conventional ultrasonography with limited field-of-view (FOV) probes, and PAT does not provide sufficient scattering-based soft tissue morphological contrast. Complementing each other, fast panoramic rotational ultrasound tomography (RUST) and PAT are integrated for hybrid rotational ultrasound and photoacoustic tomography (RUS-PAT), which obtains 3D ultrasound structural and PAT angiographic images of the human body quasi-simultaneously. The RUST functionality is achieved in a cost-effective manner using a single-element ultrasonic transducer for ultrasound transmission and rotating arc-shaped arrays for 3D panoramic detection. RUST is superior to conventional ultrasonography, which either has a limited FOV with a linear array or is high-cost with a hemispherical array that requires both transmission and receiving. By switching the acoustic source to a light source, the system is conveniently converted to PAT mode to acquire angiographic images in the same region. Using RUS-PAT, we have successfully imaged the human head, breast, hand, and foot with a 10 cm diameter FOV, submillimeter isotropic resolution, and 10 s imaging time for each modality. The 3D RUS-PAT is a powerful tool for high-speed, 3D, dual-contrast imaging of the human body with potential for rapid clinical translation.
在诊断、监测和治疗医疗状况时,获取人体形态学和血管造影信息至关重要。超声通过基于声阻抗变化的软组织形态评估发挥作用,而光声断层扫描(PAT)则能基于血红蛋白的固有吸收来可视化血管。在常规超声中,由于视野有限的探针,通常无法实现对血管的的三维全景成像(3D),而PAT并不提供足够的基于散射的软组织形态对比。超声旋转断层扫描(RUST)和PAT相互补充,将其整合为混合旋转超声和光声断层扫描(RUS-PAT),可同时获取人体的三维超声结构和PAT血管造影图像。RUST的功能以具有成本效益的方式实现,采用单元素超声换能器进行超声传输,并围绕三维全景检测旋转弧形阵列。与传统的超声相比,RUST具有优势,传统的超声要么视野有限使用线性阵列,要么使用需要传输和接收的半球形阵列成本较高。通过将声源切换为光源,系统可轻松转换为PAT模式以获取同一区域的血管造影图像。使用RUS-PAT,我们成功地对人头、乳房、手和脚进行了成像,成像区域直径为10厘米,具有亚毫米各向同性分辨率以及每模态的成像时间为10秒。三维RUS-PAT是一个强大的工具,能够以高速对人体进行三维双对比度成像,具有迅速进入临床应用的潜力。
论文及项目相关链接
Summary
结合超声与光声成像技术,实现人体形态学与血管造影信息的三维全景成像。采用快速旋转超声断层扫描技术与光声成像技术相结合,以低成本的方式获得三维超声结构和光声血管造影图像。此技术有望迅速应用于临床。
Key Takeaways
- 超声成像与光声成像技术结合可实现人体形态与血管造影信息的三维全景成像。
- 快速旋转超声断层扫描技术(RUST)可以实现低成本的三维全景检测。
- RUST技术相较于传统超声技术具有更广的视野和更高的成本效益。
- 通过将声源切换为光源,系统可便捷地转换为光声成像模式。
- RUS-PAT技术已成功应用于人体头部、胸部、手部及足部的成像。
- 该技术具备高速度、高分辨率及潜在的临床应用前景。
点此查看论文截图
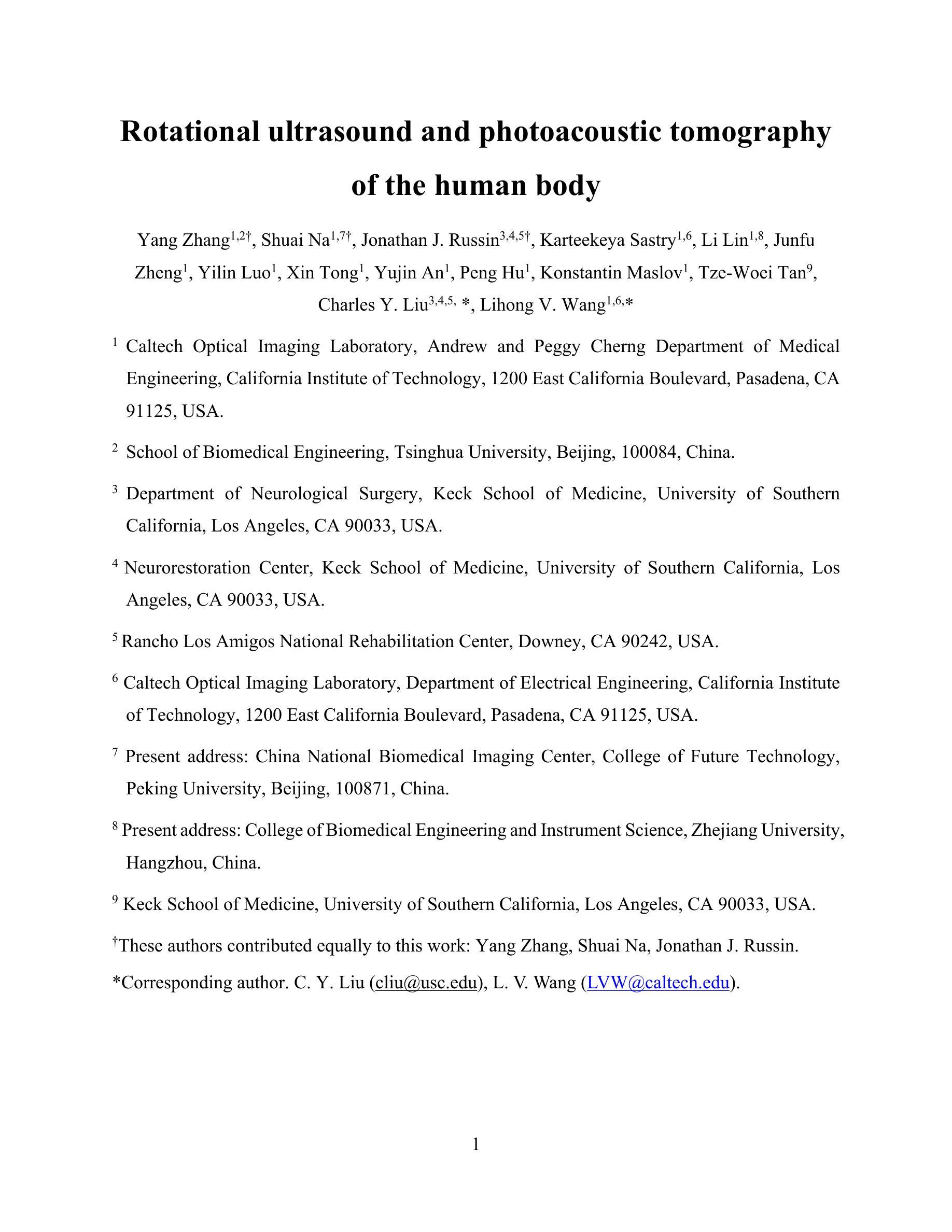
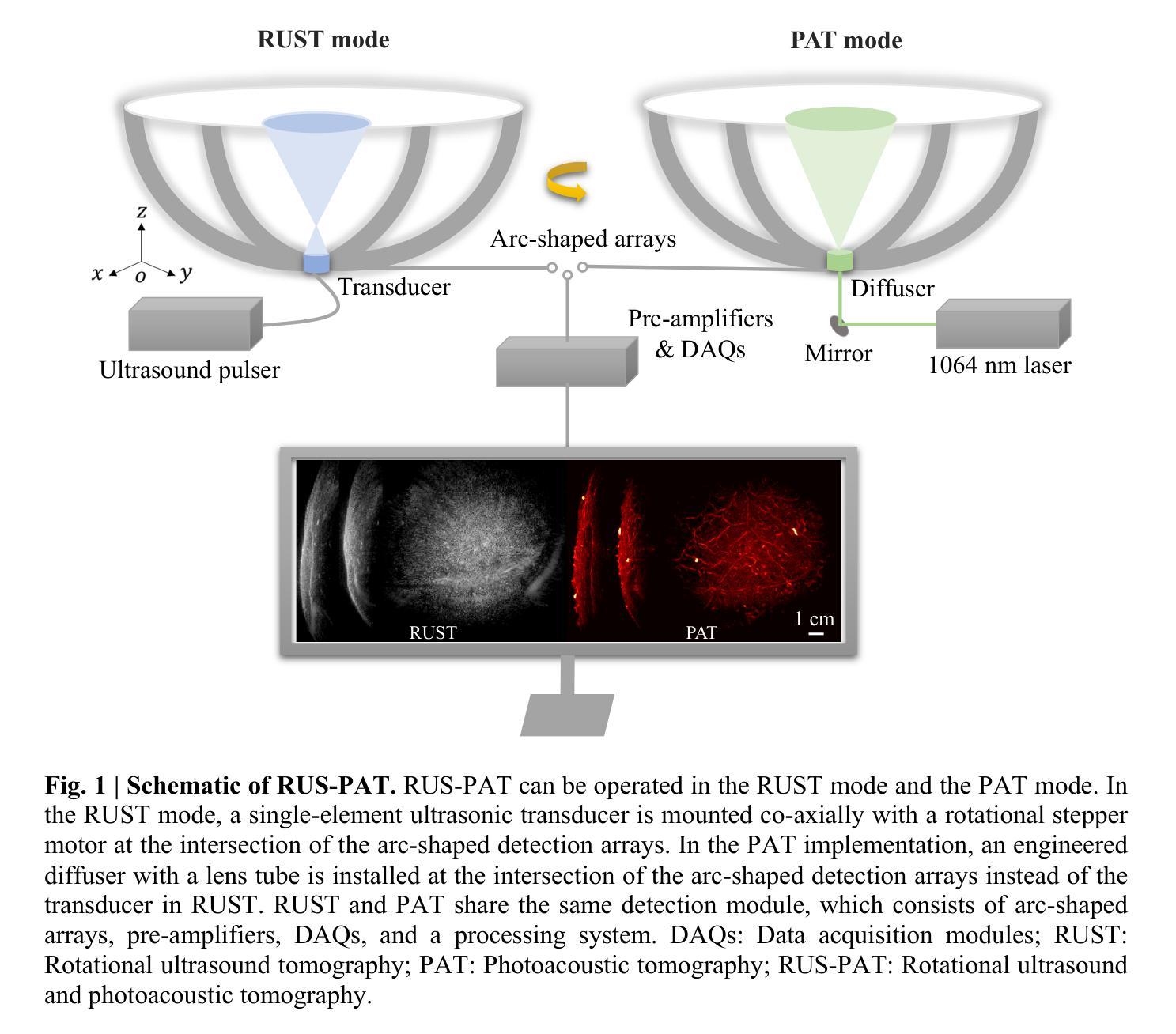
Exploring Cognitive and Aesthetic Causality for Multimodal Aspect-Based Sentiment Analysis
Authors:Luwei Xiao, Rui Mao, Shuai Zhao, Qika Lin, Yanhao Jia, Liang He, Erik Cambria
Multimodal aspect-based sentiment classification (MASC) is an emerging task due to an increase in user-generated multimodal content on social platforms, aimed at predicting sentiment polarity toward specific aspect targets (i.e., entities or attributes explicitly mentioned in text-image pairs). Despite extensive efforts and significant achievements in existing MASC, substantial gaps remain in understanding fine-grained visual content and the cognitive rationales derived from semantic content and impressions (cognitive interpretations of emotions evoked by image content). In this study, we present Chimera: a cognitive and aesthetic sentiment causality understanding framework to derive fine-grained holistic features of aspects and infer the fundamental drivers of sentiment expression from both semantic perspectives and affective-cognitive resonance (the synergistic effect between emotional responses and cognitive interpretations). Specifically, this framework first incorporates visual patch features for patch-word alignment. Meanwhile, it extracts coarse-grained visual features (e.g., overall image representation) and fine-grained visual regions (e.g., aspect-related regions) and translates them into corresponding textual descriptions (e.g., facial, aesthetic). Finally, we leverage the sentimental causes and impressions generated by a large language model (LLM) to enhance the model’s awareness of sentimental cues evoked by semantic content and affective-cognitive resonance. Experimental results on standard MASC datasets demonstrate the effectiveness of the proposed model, which also exhibits greater flexibility to MASC compared to LLMs such as GPT-4o. We have publicly released the complete implementation and dataset at https://github.com/Xillv/Chimera
基于多模态的情感分类(MASC)是一项新兴的任务,这是由于社交平台上的用户生成的多模态内容不断增加所导致的。它的目标是预测对特定方面目标(即文本图像对中明确提及的实体或属性)的情感倾向。尽管现有的MASC领域已经付出了巨大的努力并取得了显著的成就,但在理解精细粒度的视觉内容以及从语义内容和印象中得出的认知理由方面仍存在很大差距(即对图像内容引发的情感的认知解读)。在这项研究中,我们提出了Chimera:一个认知和审美情感因果理解框架,用于推导方面的精细粒度整体特征,并从语义视角和情感认知共振(情感反应和认知解读之间的协同作用)推断情感表达的基本驱动因素。具体来说,该框架首先结合视觉补丁特征进行补丁词对齐。同时,它提取粗粒度的视觉特征(例如整体图像表示)和细粒度的视觉区域(例如与方面相关的区域),并将它们转化为相应的文本描述(例如面部、美学)。最后,我们利用大型语言模型(LLM)产生的情感因素和印象,增强模型对语义内容和情感认知共振所引发的情感线索的认识。在标准MASC数据集上的实验结果表明,所提出模型的有效性,与GPT-4o等LLM相比,该模型在MASC方面表现出更大的灵活性。我们已在https://github.com/Xillv/ Chimera上公开发布了完整的实现和数据集。
论文及项目相关链接
PDF Accepted by TAFFC 2025
Summary
多模态方面情感分类(MASC)是预测针对特定方面目标的情感极性(即文本图像对中明确提及的实体或属性)的新兴任务。由于社交平台上用户生成的多模态内容的增加,尽管已有大量研究和显著成果,但在理解精细视觉内容和从语义内容和印象派生的认知理由方面仍存在差距。本研究提出了Chimera框架,该框架结合了认知和情感因果理解,以获取方面的精细整体特征,并从语义和认知情感共振的角度推断情感表达的基本驱动因素。实验结果表明,该模型在标准MASC数据集上表现出有效性,并且与GPT-4等大语言模型相比具有更大的灵活性。
Key Takeaways
- 多模态方面情感分类(MASC)是预测针对特定方面目标的情感极性的新兴任务。
- MASC面临理解精细视觉内容和认知理由方面的挑战。
- Chimera框架结合了认知和情感因果理解,旨在解决这些问题。
- Chimera框架实现了视觉补丁特征和补丁词的匹配。
- 该框架区分了粗粒度视觉特征和精细视觉区域并将其翻译成相应的文本描述。
- 使用大型语言模型(LLM)产生的情感因素和印象增强模型的认知和情感共鸣。
点此查看论文截图
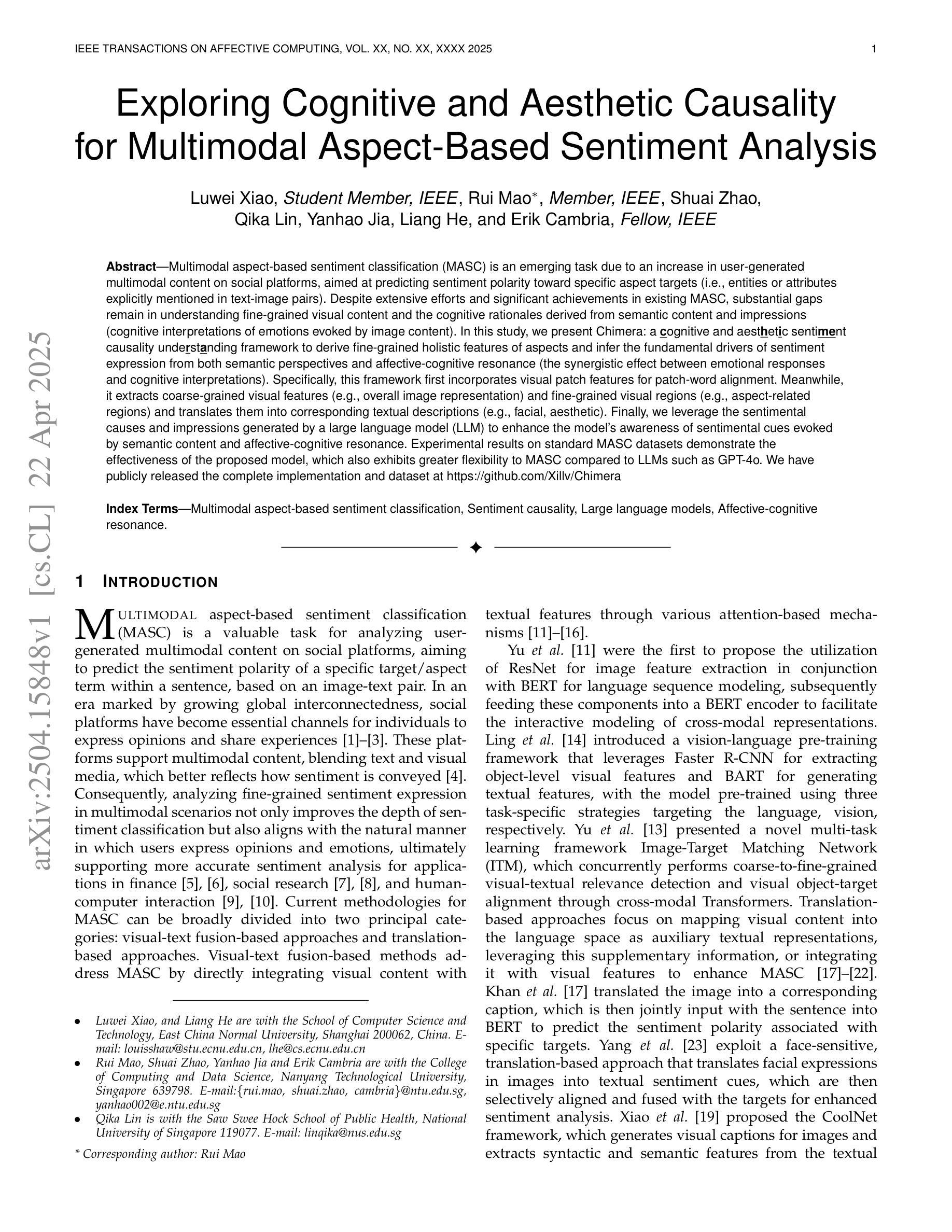
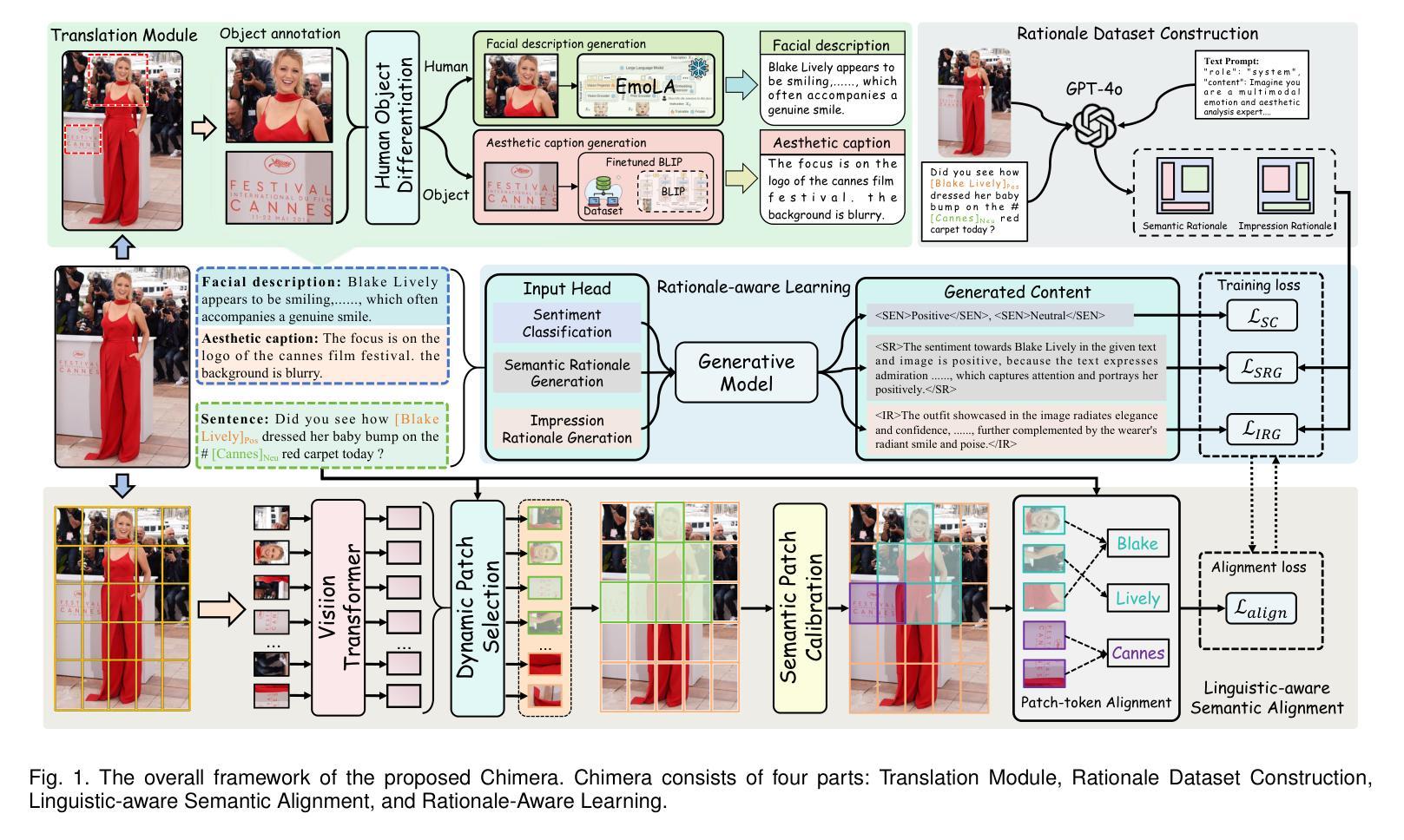
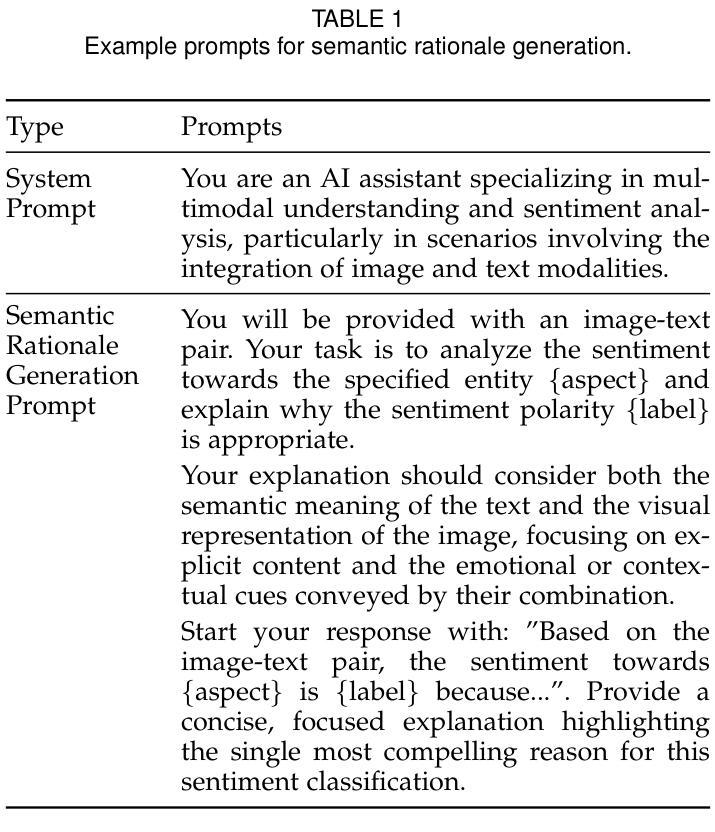
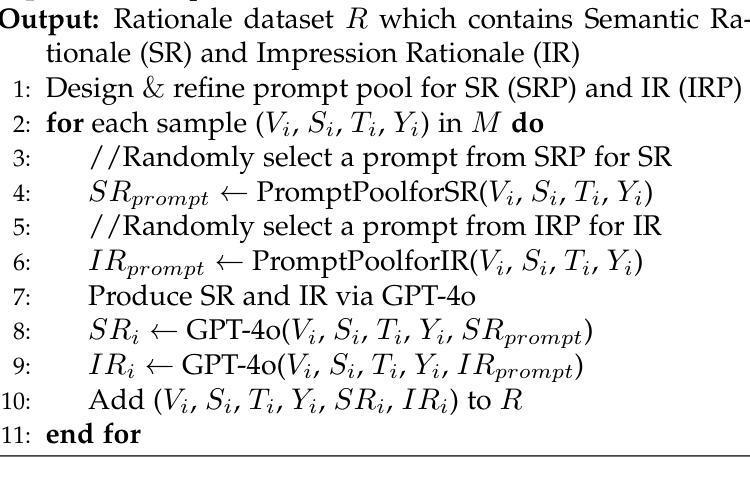
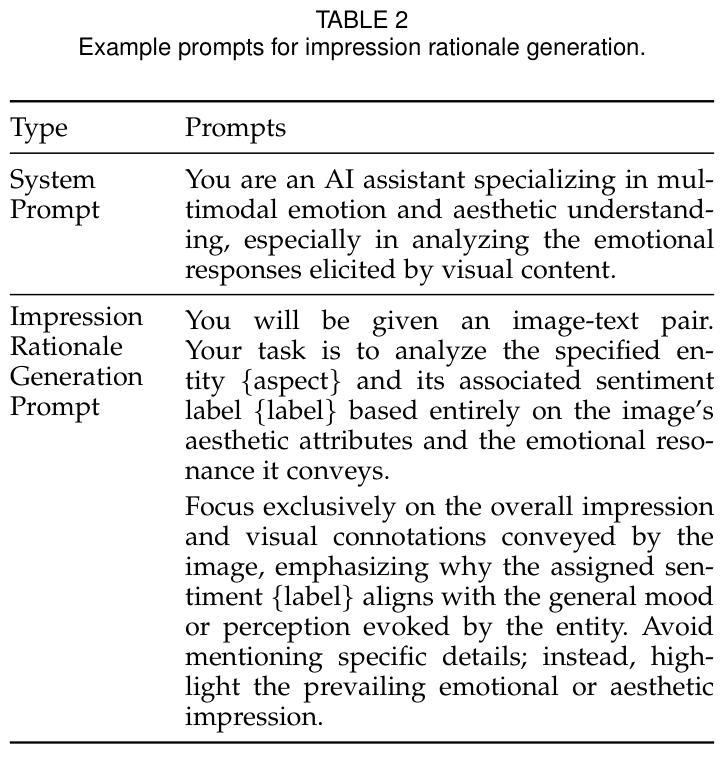
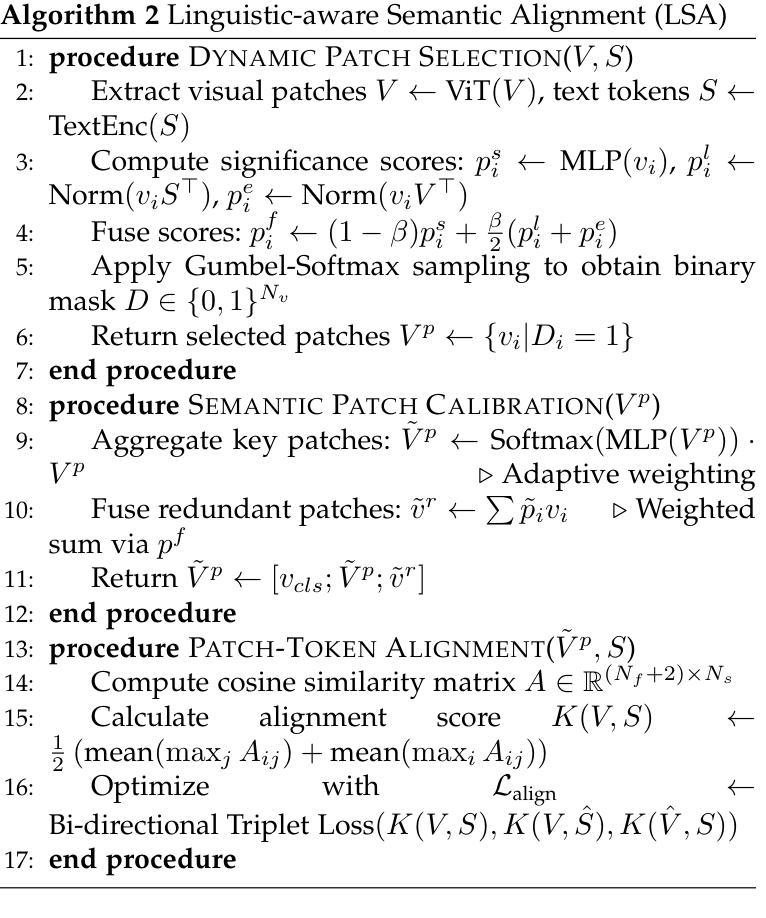