⚠️ 以下所有内容总结都来自于 大语言模型的能力,如有错误,仅供参考,谨慎使用
🔴 请注意:千万不要用于严肃的学术场景,只能用于论文阅读前的初筛!
💗 如果您觉得我们的项目对您有帮助 ChatPaperFree ,还请您给我们一些鼓励!⭐️ HuggingFace免费体验
2025-04-24 更新
Parallel Corpora for Machine Translation in Low-resource Indic Languages: A Comprehensive Review
Authors:Rahul Raja, Arpita Vats
Parallel corpora play an important role in training machine translation (MT) models, particularly for low-resource languages where high-quality bilingual data is scarce. This review provides a comprehensive overview of available parallel corpora for Indic languages, which span diverse linguistic families, scripts, and regional variations. We categorize these corpora into text-to-text, code-switched, and various categories of multimodal datasets, highlighting their significance in the development of robust multilingual MT systems. Beyond resource enumeration, we critically examine the challenges faced in corpus creation, including linguistic diversity, script variation, data scarcity, and the prevalence of informal textual content.We also discuss and evaluate these corpora in various terms such as alignment quality and domain representativeness. Furthermore, we address open challenges such as data imbalance across Indic languages, the trade-off between quality and quantity, and the impact of noisy, informal, and dialectal data on MT performance. Finally, we outline future directions, including leveraging cross-lingual transfer learning, expanding multilingual datasets, and integrating multimodal resources to enhance translation quality. To the best of our knowledge, this paper presents the first comprehensive review of parallel corpora specifically tailored for low-resource Indic languages in the context of machine translation.
并行语料库在训练机器翻译(MT)模型方面发挥着重要作用,特别是在高质量双语数据稀缺的低资源语言领域。本文全面概述了印度语言可用的并行语料库,这些语料库涵盖了不同的语言家族、脚本和地区变体。我们将这些语料库分为文本到文本、代码切换和多种模态数据集的不同类别,并强调它们在开发稳健的多语种机器翻译系统中的重要性。除了资源枚举,我们还对语料库创建面临的挑战进行了批判性审视,包括语言多样性、脚本变化、数据稀缺以及非正式文本内容的普及。我们还根据对齐质量和领域代表性等不同标准对这些语料库进行了讨论和评估。此外,我们还探讨了当前面临的挑战,如印度语言之间的数据不平衡、质量与数量之间的权衡,以及嘈杂、非正式和方言数据对机器翻译性能的影响。最后,我们概述了未来的发展方向,包括利用跨语言迁移学习、扩展多语种数据集和整合多模态资源以提高翻译质量。据我们所知,本文是在机器翻译的语境下,专门针对低资源印度语言的首个全面回顾平行语料库的论文。
论文及项目相关链接
PDF Accepted in NACCL
Summary
本文综述了可用于训练机器翻译模型的印地语平行语料库,特别关注低资源语言的高质量双语数据的稀缺问题。文章详细介绍了印地语语料库的类别,如文本到文本、代码转换和多种模态数据集,并强调了它们在开发稳健的多语种机器翻译系统中的重要性。文章还批判性地探讨了语料库创建面临的挑战,包括语言多样性、脚本变化、数据稀缺以及非正式文本内容的普及。此外,本文还讨论了这些语料库的对齐质量和领域代表性等评估指标,并探讨了数据不平衡、质量与数量之间的权衡以及噪声、非正式和方言数据对机器翻译性能的影响。最后,本文展望了未来的研究方向,包括利用跨语言迁移学习、扩展多语种数据集和整合多模态资源来提高翻译质量。据我们所知,本文是对低资源印地语平行语料库在机器翻译领域的首次全面综述。
Key Takeaways
- 平行语料库在训练机器翻译模型方面发挥着重要作用,特别是对于低资源语言而言。
- 印地语平行语料库分为文本到文本、代码转换和多种模态数据集等类别。
- 语料库创建面临诸多挑战,如语言多样性、脚本变化、数据稀缺和非正式文本内容的普及。
- 对语料库的对齐质量和领域代表性等评估指标进行了讨论。
- 数据不平衡、质量与数量之间的权衡以及噪声、非正式和方言数据对机器翻译性能有影响。
- 未来的研究方向包括利用跨语言迁移学习、扩展多语种数据集和整合多模态资源来提高翻译质量。
点此查看论文截图
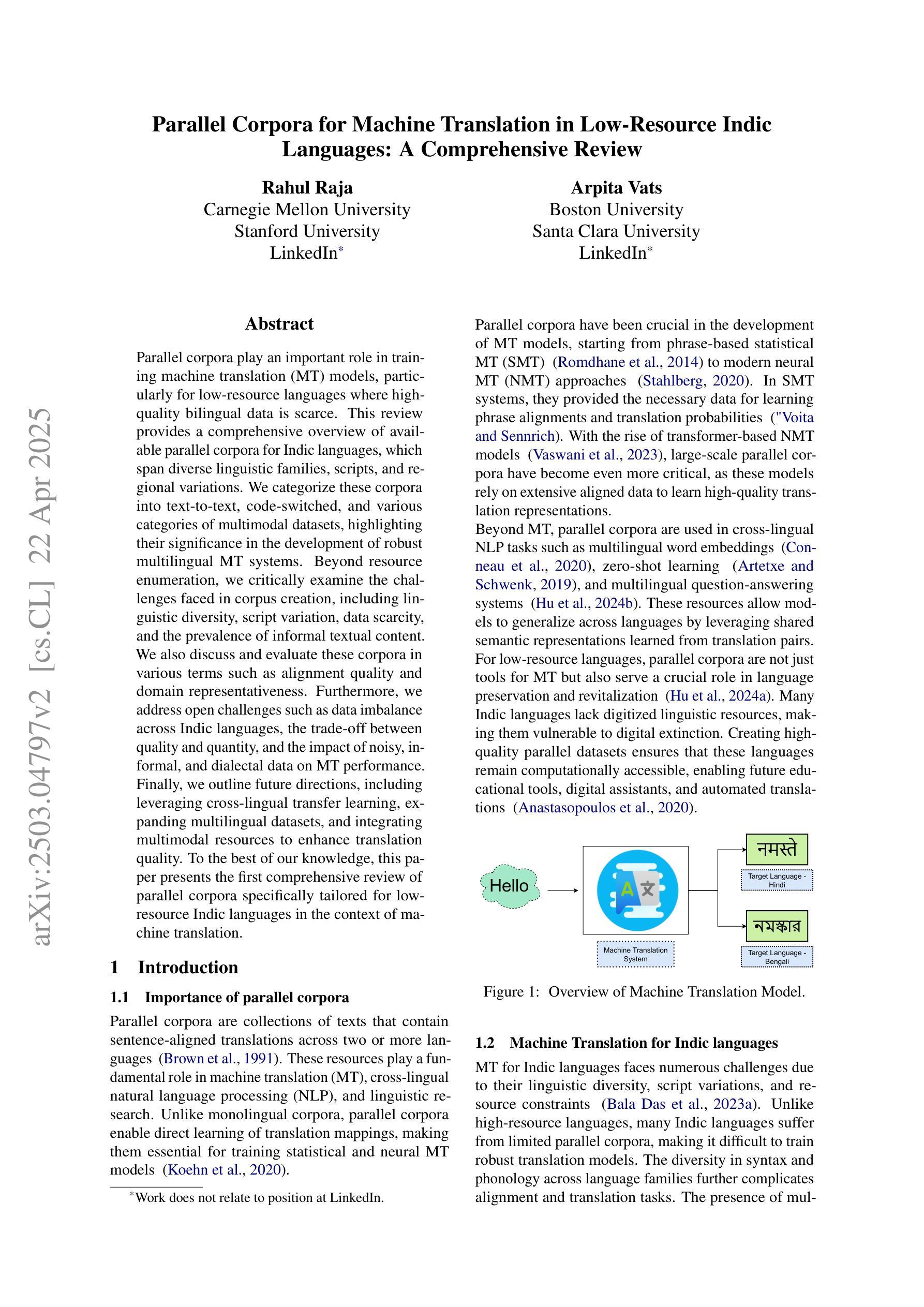
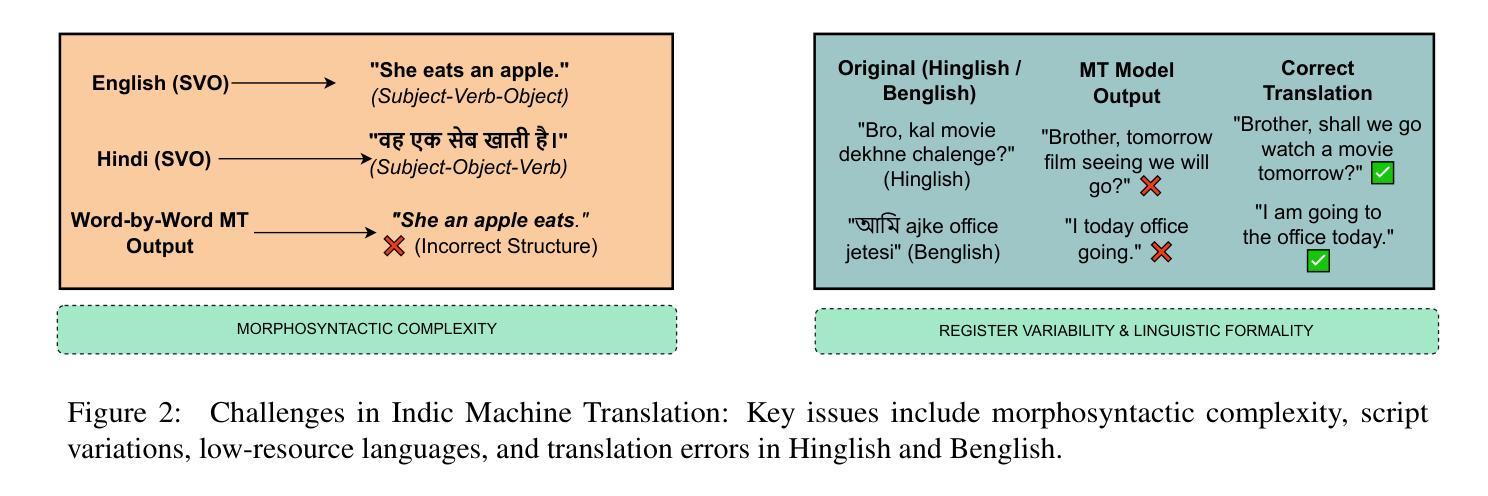
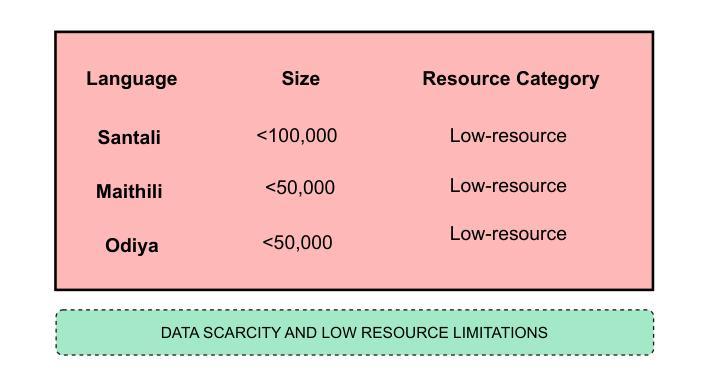