⚠️ 以下所有内容总结都来自于 大语言模型的能力,如有错误,仅供参考,谨慎使用
🔴 请注意:千万不要用于严肃的学术场景,只能用于论文阅读前的初筛!
💗 如果您觉得我们的项目对您有帮助 ChatPaperFree ,还请您给我们一些鼓励!⭐️ HuggingFace免费体验
2025-04-25 更新
SEGA: Drivable 3D Gaussian Head Avatar from a Single Image
Authors:Chen Guo, Zhuo Su, Jian Wang, Shuang Li, Xu Chang, Zhaohu Li, Yang Zhao, Guidong Wang, Ruqi Huang
Creating photorealistic 3D head avatars from limited input has become increasingly important for applications in virtual reality, telepresence, and digital entertainment. While recent advances like neural rendering and 3D Gaussian splatting have enabled high-quality digital human avatar creation and animation, most methods rely on multiple images or multi-view inputs, limiting their practicality for real-world use. In this paper, we propose SEGA, a novel approach for Single-imagE-based 3D drivable Gaussian head Avatar creation that combines generalized prior models with a new hierarchical UV-space Gaussian Splatting framework. SEGA seamlessly combines priors derived from large-scale 2D datasets with 3D priors learned from multi-view, multi-expression, and multi-ID data, achieving robust generalization to unseen identities while ensuring 3D consistency across novel viewpoints and expressions. We further present a hierarchical UV-space Gaussian Splatting framework that leverages FLAME-based structural priors and employs a dual-branch architecture to disentangle dynamic and static facial components effectively. The dynamic branch encodes expression-driven fine details, while the static branch focuses on expression-invariant regions, enabling efficient parameter inference and precomputation. This design maximizes the utility of limited 3D data and achieves real-time performance for animation and rendering. Additionally, SEGA performs person-specific fine-tuning to further enhance the fidelity and realism of the generated avatars. Experiments show our method outperforms state-of-the-art approaches in generalization ability, identity preservation, and expression realism, advancing one-shot avatar creation for practical applications.
创建具有真实感的3D头像化身从有限的输入对于虚拟现实、远程存在和数字娱乐等应用变得越来越重要。尽管最近的神经渲染和3D高斯拼贴等技术进步已实现了高质量的数字人类化身创建和动画,但大多数方法仍依赖于多张图像或多视角输入,限制了它们在现实世界应用中的实用性。在本文中,我们提出了SEGA,这是一种基于单图像的新型可驱动的高斯头像素化身创建方法,它结合了广义先验模型和新层次化的UV空间高斯拼贴框架。SEGA无缝结合了从大规模二维数据集得出的先验知识和从多视角、多表情和多身份数据中学习的三维先验知识,实现了对未见身份的稳健泛化,同时确保在不同视角和表情下的三维一致性。我们还提出了一种层次化的UV空间高斯拼贴框架,它利用基于FLAME的结构先验知识,并采用双分支架构有效地分离动态和静态面部组件。动态分支编码表情驱动的精细细节,而静态分支则专注于表情不变的区域,从而实现高效的参数推断和预计算。这种设计最大限度地提高了有限三维数据的实用性,实现了动画和渲染的实时性能。此外,SEGA还执行针对个人的微调,以进一步提高生成的化身的真实感和逼真度。实验表明,我们的方法在泛化能力、身份保留和表情逼真度方面优于最先进的方法,推动了单次拍摄化身创建的实际应用。
论文及项目相关链接
Summary
本文提出了一种基于单张图像创建3D可驱动高斯头像的方法SEGA,该方法结合了通用先验模型和新层次UV空间高斯平铺框架。SEGA无缝结合大规模二维数据集导出的先验信息和从多角度、多表情和多身份数据中学习的三维先验信息,实现了对未见身份的稳健泛化,同时确保在新视角和表情下的三维一致性。此外,SEGA采用层次UV空间高斯平铺框架,利用基于FLAME的结构先验信息,并采用双分支架构有效分离动态和静态面部组件。实验表明,该方法在泛化能力、身份保留和表情真实性方面优于现有技术,推动了基于单张图像的一键式头像创建技术在现实应用中的发展。
Key Takeaways
- SEGA方法实现了基于单张图像创建3D可驱动的高斯头像。
- SEGA结合了通用先验模型与新的层次UV空间高斯平铺框架。
- SEGA能够无缝结合二维和三维数据,实现对未见身份的稳健泛化。
- 采用层次UV空间高斯平铺框架提高参数推断和预计算效率。
- SEGA实现了实时动画和渲染性能。
- SEGA可以进行个性化微调,提高头像的逼真度。
点此查看论文截图
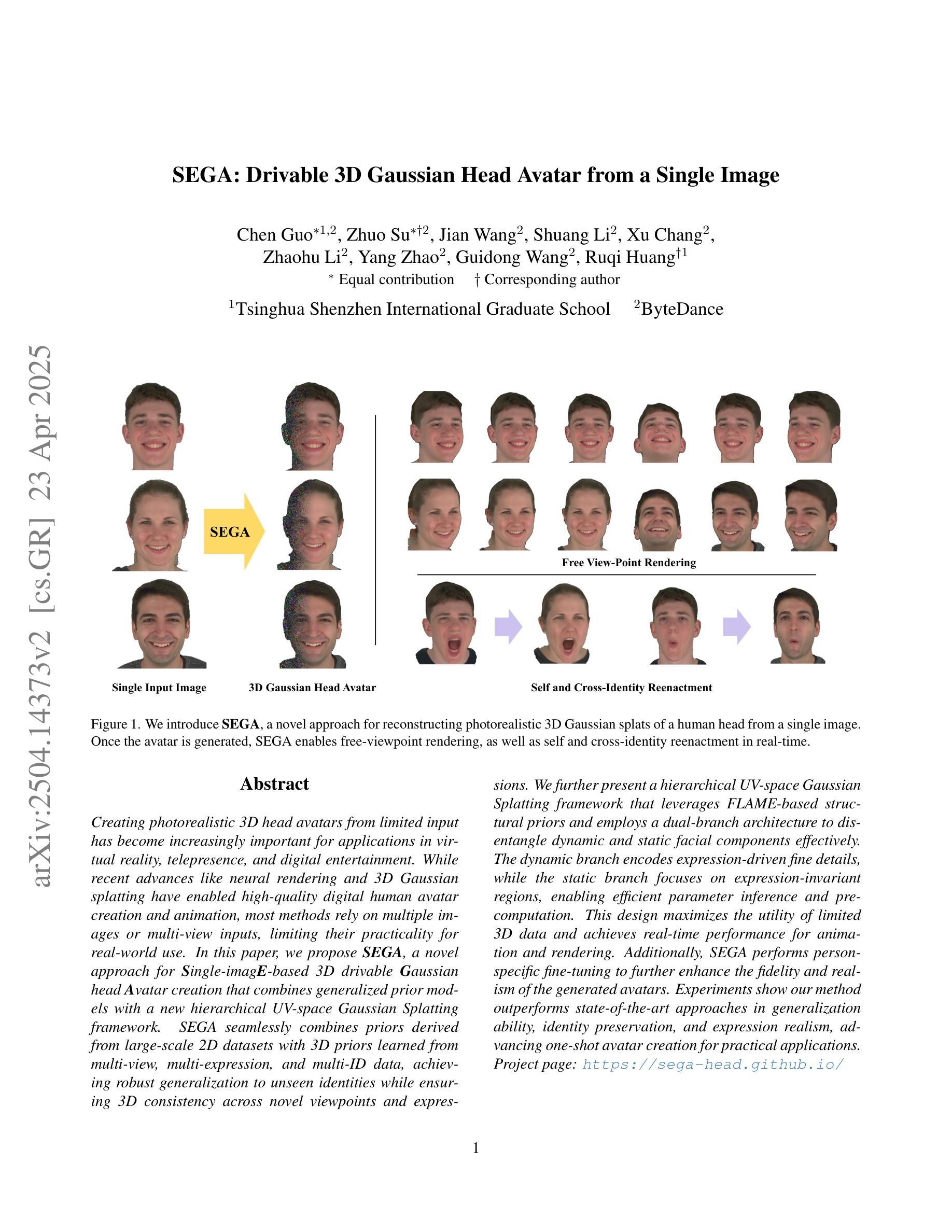
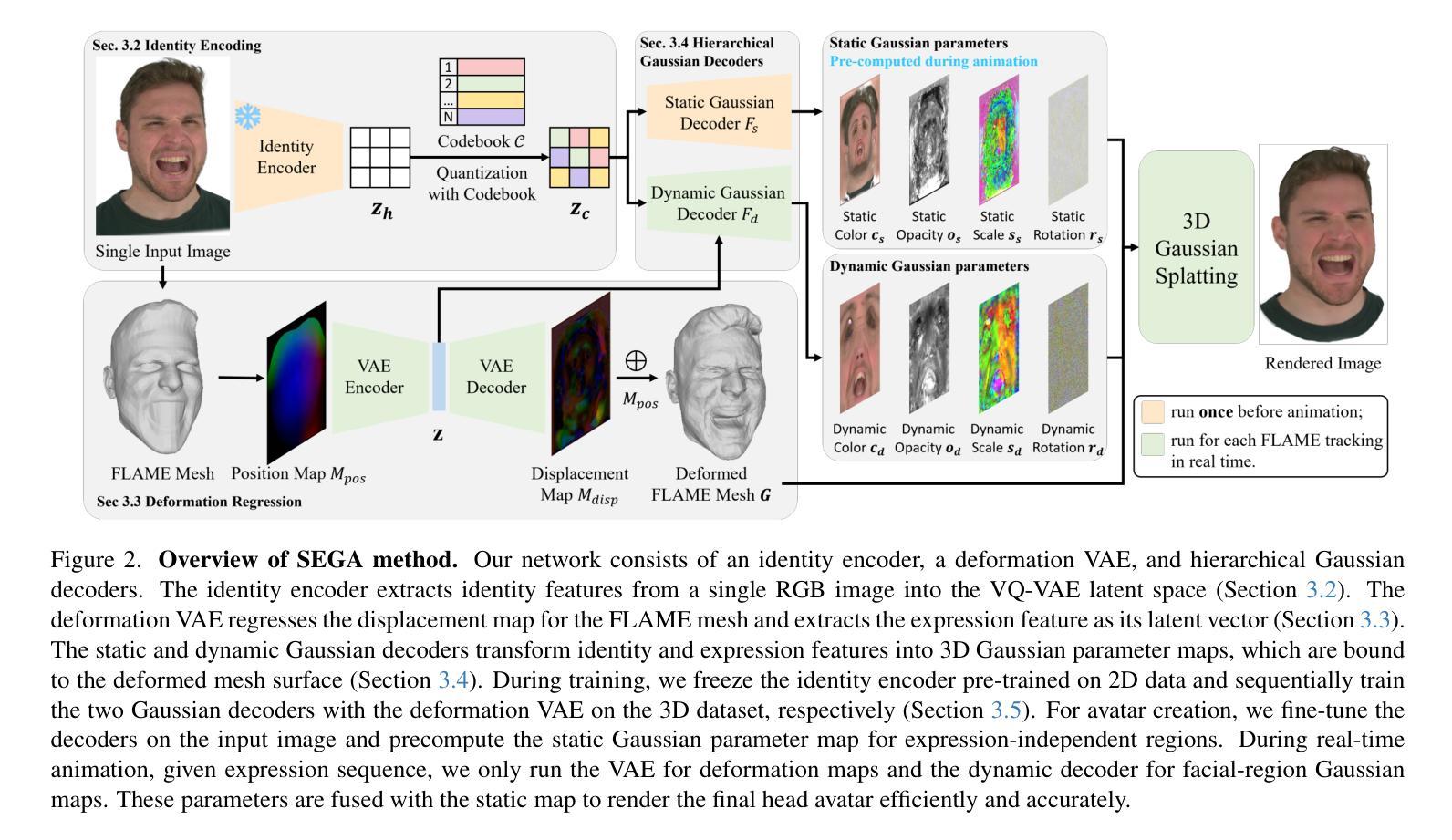