⚠️ 以下所有内容总结都来自于 大语言模型的能力,如有错误,仅供参考,谨慎使用
🔴 请注意:千万不要用于严肃的学术场景,只能用于论文阅读前的初筛!
💗 如果您觉得我们的项目对您有帮助 ChatPaperFree ,还请您给我们一些鼓励!⭐️ HuggingFace免费体验
2025-04-25 更新
Gaussian Splatting is an Effective Data Generator for 3D Object Detection
Authors:Farhad G. Zanjani, Davide Abati, Auke Wiggers, Dimitris Kalatzis, Jens Petersen, Hong Cai, Amirhossein Habibian
We investigate data augmentation for 3D object detection in autonomous driving. We utilize recent advancements in 3D reconstruction based on Gaussian Splatting for 3D object placement in driving scenes. Unlike existing diffusion-based methods that synthesize images conditioned on BEV layouts, our approach places 3D objects directly in the reconstructed 3D space with explicitly imposed geometric transformations. This ensures both the physical plausibility of object placement and highly accurate 3D pose and position annotations. Our experiments demonstrate that even by integrating a limited number of external 3D objects into real scenes, the augmented data significantly enhances 3D object detection performance and outperforms existing diffusion-based 3D augmentation for object detection. Extensive testing on the nuScenes dataset reveals that imposing high geometric diversity in object placement has a greater impact compared to the appearance diversity of objects. Additionally, we show that generating hard examples, either by maximizing detection loss or imposing high visual occlusion in camera images, does not lead to more efficient 3D data augmentation for camera-based 3D object detection in autonomous driving.
我们研究了自动驾驶中3D对象检测的数据增强技术。我们利用基于高斯涂抹的3D重建的最新进展,将3D对象放置在驾驶场景中。与现有基于扩散的方法不同,这些方法根据BEV布局合成图像,我们的方法直接在重建的3D空间中放置3D对象,并明确施加几何变换。这确保了对象放置的物理可行性以及高度准确的3D姿势和位置注释。我们的实验表明,即使只将有限数量的外部3D对象集成到真实场景中,增强数据也能显著提高3D对象检测性能,并优于现有的基于扩散的3D对象检测数据增强方法。在nuScenes数据集上的广泛测试表明,在对象放置中施加高几何多样性比对象的外观多样性具有更大的影响。此外,我们还表明,通过最大化检测损失或在相机图像中施加高视觉遮挡来生成困难样本,并不会导致用于自动驾驶中基于相机的3D对象检测的更有效的3D数据增强。
论文及项目相关链接
Summary
本文探讨了自动驾驶中3D目标检测的数据增强问题。研究团队利用基于高斯涂斑技术的最新3D重建方法,在驾驶场景中直接放置3D目标,并进行明确的几何变换。此方法确保了目标放置的物理合理性,并提供了精确的3D姿态和位置标注。实验表明,即使在真实场景中添加少量外部3D目标,增强数据也能显著提高3D目标检测性能,并优于现有的基于扩散的3D数据增强方法。对nuScenes数据集的大量测试表明,在目标放置上施加高几何多样性比目标的外观多样性具有更大的影响。
Key Takeaways
- 研究团队利用基于高斯涂斑的3D重建技术,进行自动驾驶中的3D目标放置。
- 与现有基于扩散的方法不同,该方法直接在重建的3D空间中放置目标,并进行几何变换。
- 方法确保了目标放置的物理合理性,并提供精确标注。
- 增强数据通过添加少量外部3D目标显著提高真实场景的3D目标检测性能。
- 在nuScenes数据集上的测试表明,高几何多样性的目标放置对检测性能的影响更大。
- 生成困难样本(通过最大化检测损失或在相机图像中施加高视觉遮挡)并不适用于相机基于的自动驾驶中的3D目标检测的数据增强。
- 该研究为自动驾驶中的3D目标检测提供了新的数据增强方法和见解。
点此查看论文截图
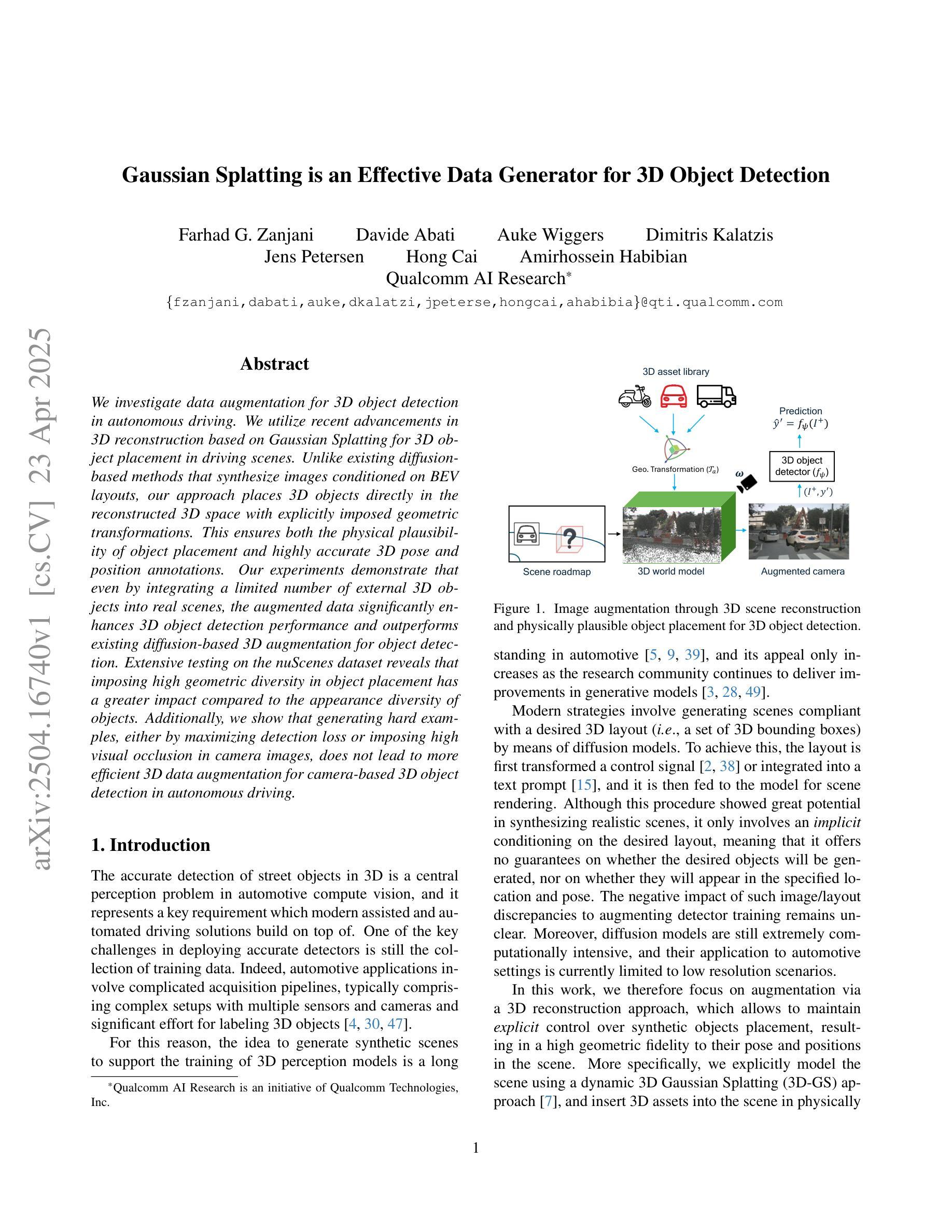
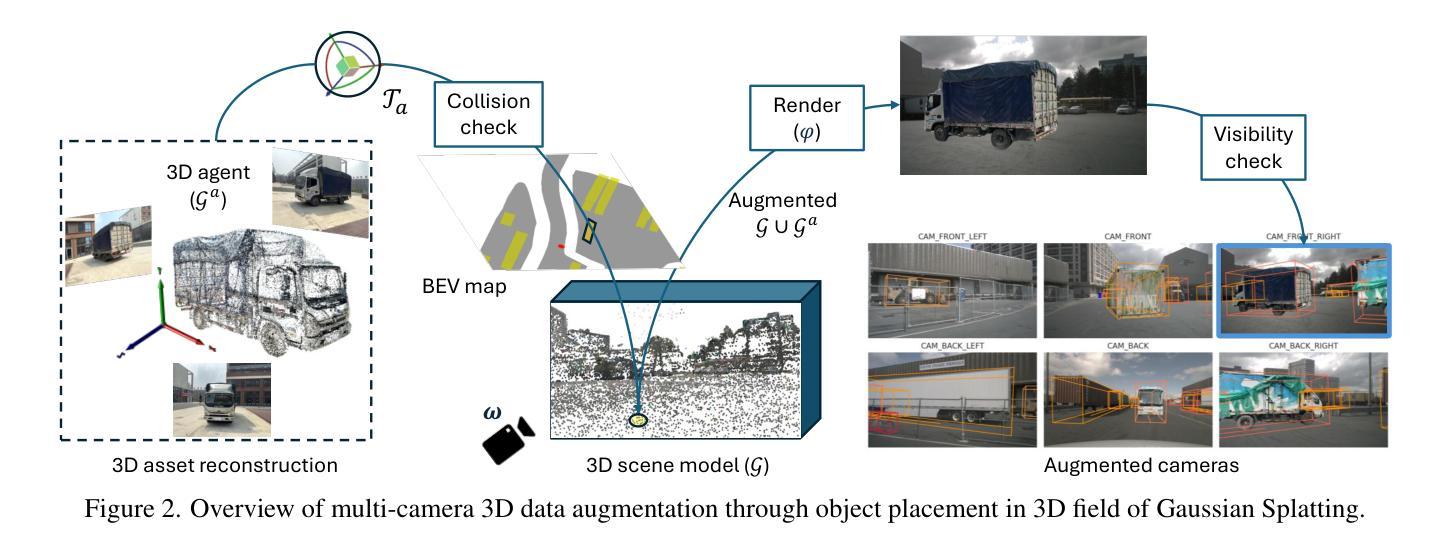
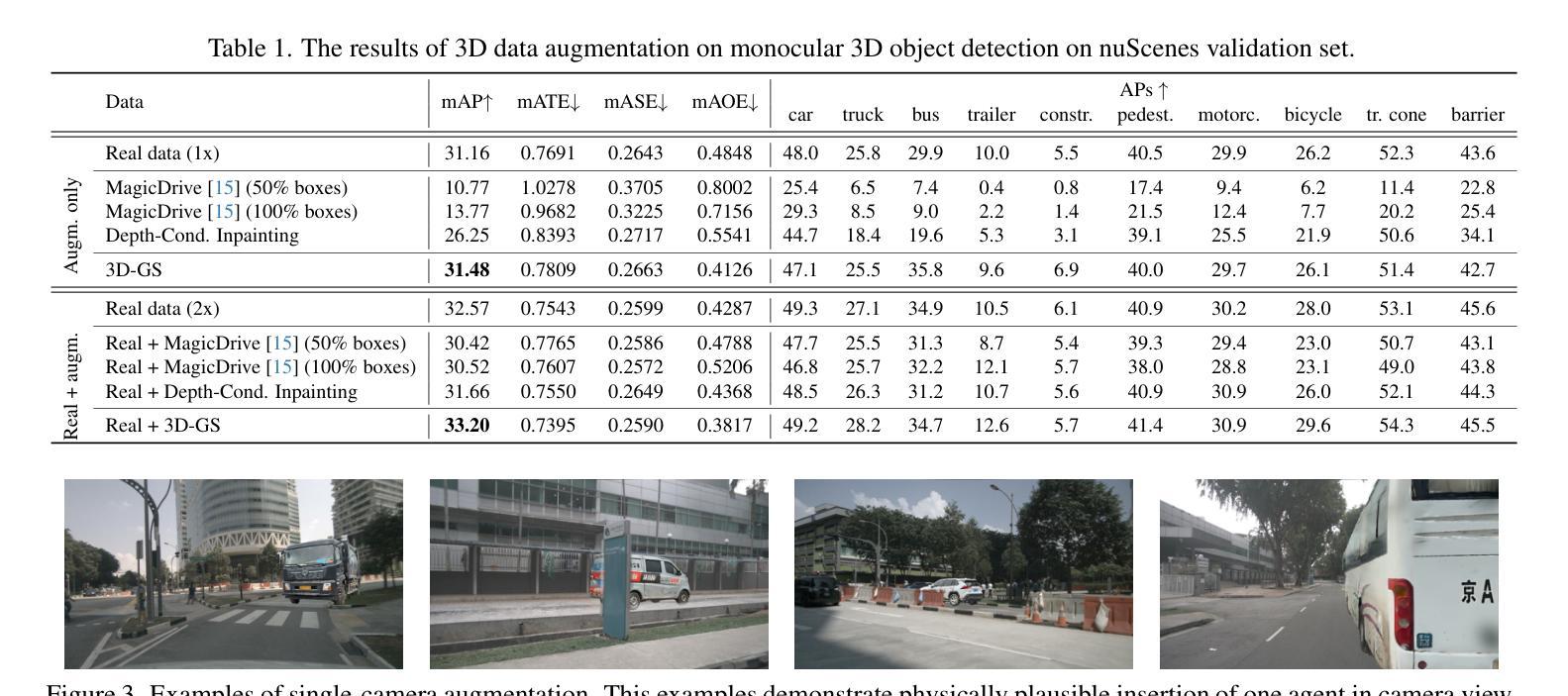
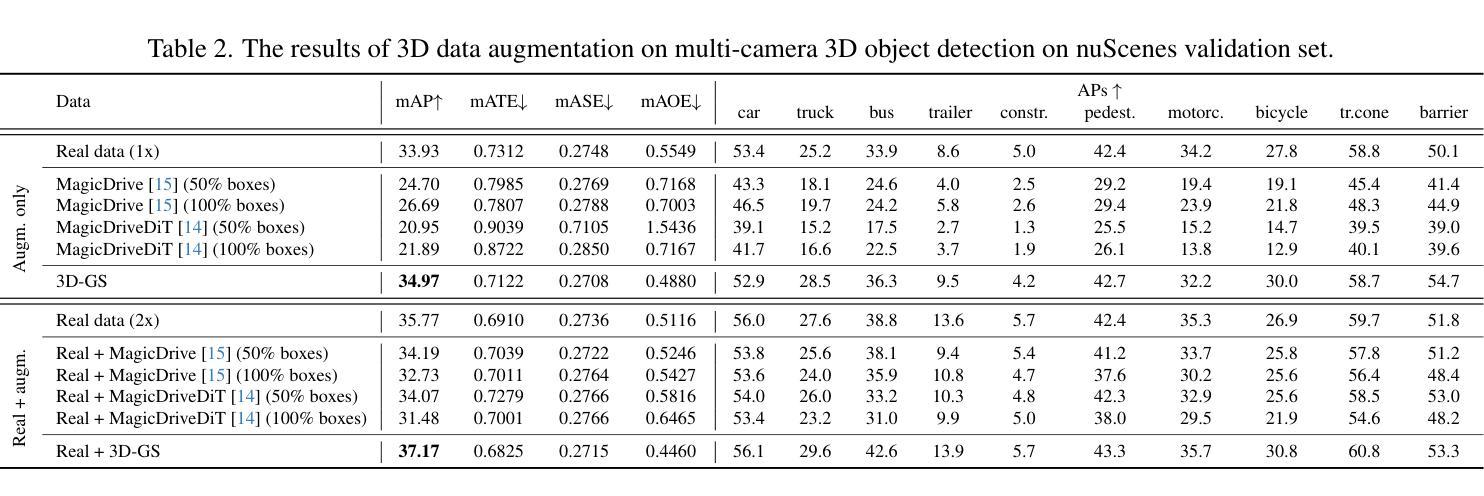
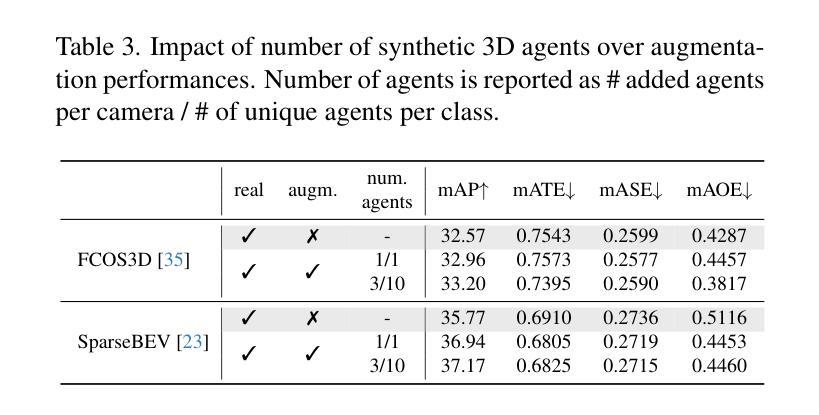