⚠️ 以下所有内容总结都来自于 大语言模型的能力,如有错误,仅供参考,谨慎使用
🔴 请注意:千万不要用于严肃的学术场景,只能用于论文阅读前的初筛!
💗 如果您觉得我们的项目对您有帮助 ChatPaperFree ,还请您给我们一些鼓励!⭐️ HuggingFace免费体验
2025-04-25 更新
Compositional 4D Dynamic Scenes Understanding with Physics Priors for Video Question Answering
Authors:Xingrui Wang, Wufei Ma, Angtian Wang, Shuo Chen, Adam Kortylewski, Alan Yuille
For vision-language models (VLMs), understanding the dynamic properties of objects and their interactions in 3D scenes from videos is crucial for effective reasoning about high-level temporal and action semantics. Although humans are adept at understanding these properties by constructing 3D and temporal (4D) representations of the world, current video understanding models struggle to extract these dynamic semantics, arguably because these models use cross-frame reasoning without underlying knowledge of the 3D/4D scenes. In this work, we introduce DynSuperCLEVR, the first video question answering dataset that focuses on language understanding of the dynamic properties of 3D objects. We concentrate on three physical concepts – velocity, acceleration, and collisions within 4D scenes. We further generate three types of questions, including factual queries, future predictions, and counterfactual reasoning that involve different aspects of reasoning about these 4D dynamic properties. To further demonstrate the importance of explicit scene representations in answering these 4D dynamics questions, we propose NS-4DPhysics, a Neural-Symbolic VideoQA model integrating Physics prior for 4D dynamic properties with explicit scene representation of videos. Instead of answering the questions directly from the video text input, our method first estimates the 4D world states with a 3D generative model powered by physical priors, and then uses neural symbolic reasoning to answer the questions based on the 4D world states. Our evaluation on all three types of questions in DynSuperCLEVR shows that previous video question answering models and large multimodal models struggle with questions about 4D dynamics, while our NS-4DPhysics significantly outperforms previous state-of-the-art models. Our code and data are released in https://xingruiwang.github.io/projects/DynSuperCLEVR/.
对于视觉语言模型(VLMs)而言,从视频中理解物体的动态属性及其在三维场景中的交互对于有效推理高级时间动作语义至关重要。尽管人类通过构建世界的三维和时间(四维)表示来擅长理解这些属性,但当前的视频理解模型在提取这些动态语义方面存在困难,这可能是因为这些模型使用跨帧推理而没有关于三维或四维场景的底层知识。在这项工作中,我们引入了DynSuperCLEVR,这是第一个专注于三维物体动态属性的语言理解的视频问答数据集。我们集中在三个物理概念上——速度、加速度和四维场景内的碰撞。我们还生成了三种类型的问题,包括事实查询、未来预测和涉及这些四维动态属性的不同方面的反事实推理。为了进一步证明明确的场景表示在回答这些四维动态问题中的重要性,我们提出了NS-4DPhysics,这是一个结合了物理先验的神经网络视频问答模型,用于根据视频的明确场景表示进行四维动态属性的语言理解。我们的方法不是直接从视频文本输入中回答问题,而是首先使用物理先验驱动的三维生成模型估计四维世界状态,然后使用神经网络符号推理基于四维世界状态回答问题。我们在DynSuperCLEVR中所有三种类型的问题上的评估表明,之前的视频问答模型和多模态模型在处理关于四维动态的问题时遇到困难,而我们的NS-4DPhysics显著优于之前的最先进模型。我们的代码和数据已在https://xingruiwang.github.io/projects/DynSuperCLEVR/中发布。
论文及项目相关链接
PDF ICLR 2025 accepted paper. Project url: https://xingruiwang.github.io/projects/DynSuperCLEVR/
Summary
针对视觉语言模型(VLMs),理解和模拟视频中的物体动态特性及其交互作用对高级时序和动作语义的有效推理至关重要。为应对此挑战,DynSuperCLEVR数据集应运而生,专注视频中的动态语言理解,涵盖速度、加速度和碰撞等物理概念。同时推出NS-4DPhysics模型,整合物理先验,建立视频的明确场景表示。方法上估计4D世界状态后结合神经网络进行问答推理。在DynSuperCLEVR上的评估显示,我们的模型在三种类型问题上优于之前视频问答模型和大型多模态模型。
Key Takeaways
- 理解视频中的物体动态特性和交互作用对于高级时序和动作语义推理至关重要。
- DynSuperCLEVR数据集专注于视频语言理解,侧重于物体的速度、加速度和碰撞等物理概念。
- 当前视频理解模型难以提取动态语义,因为它们缺乏关于3D/4D场景的底层知识。
- NS-4DPhysics模型结合了物理先验知识,用于构建视频的明确场景表示。
- 该模型通过估计4D世界状态并结合神经网络进行问答推理。
点此查看论文截图
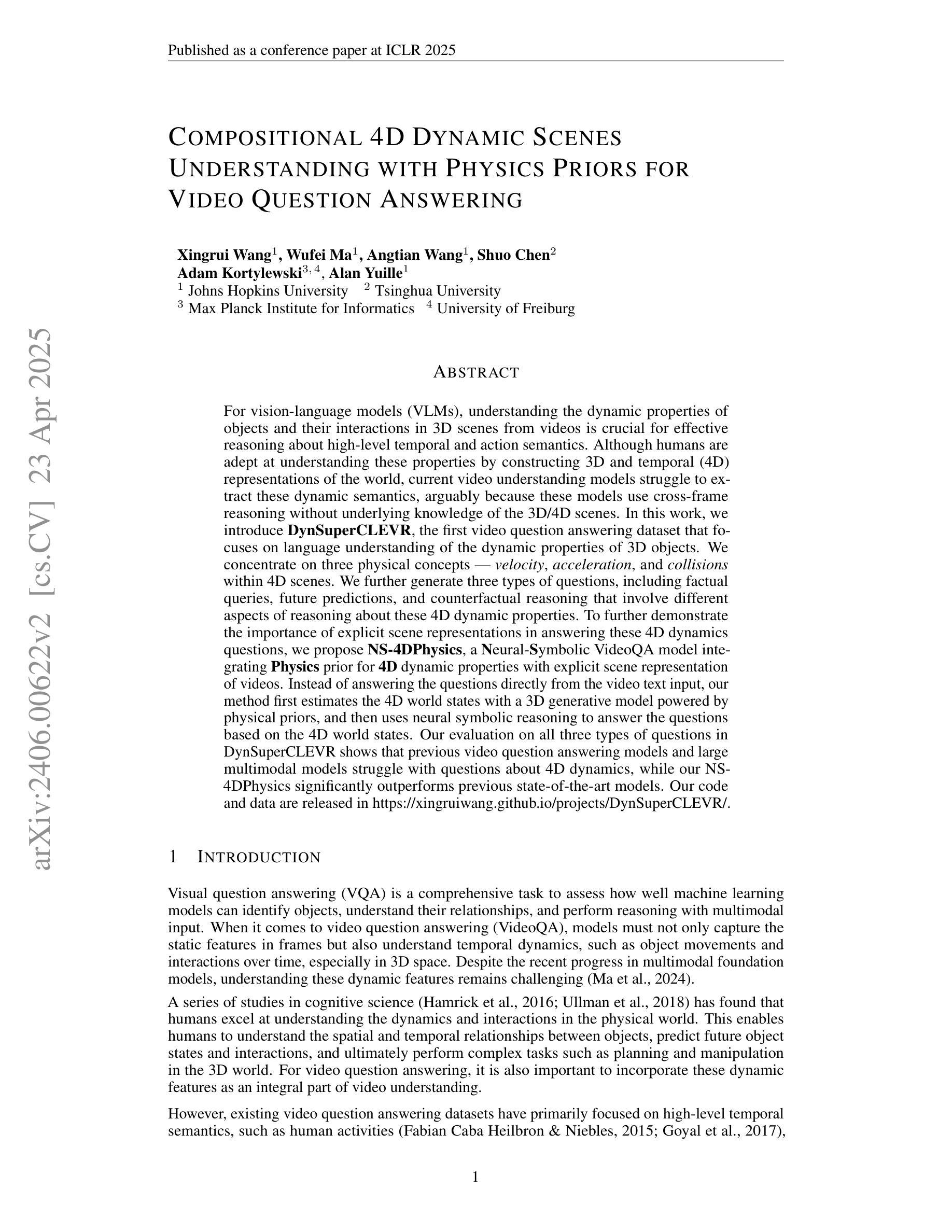
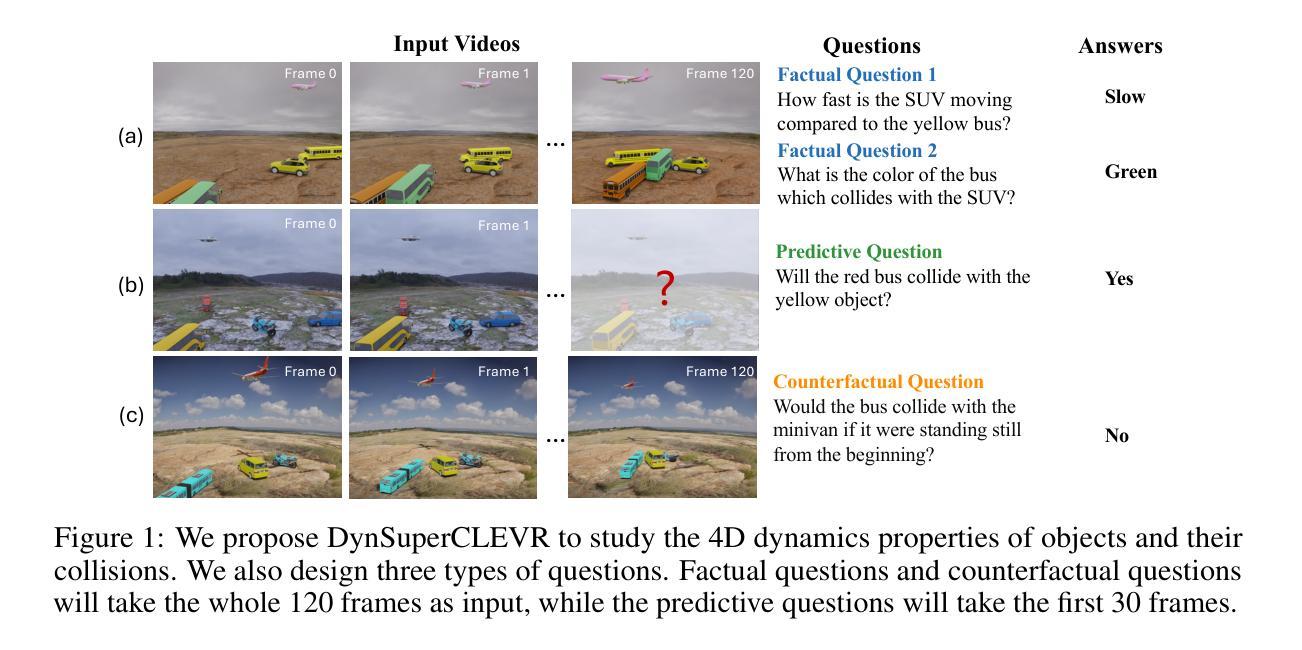
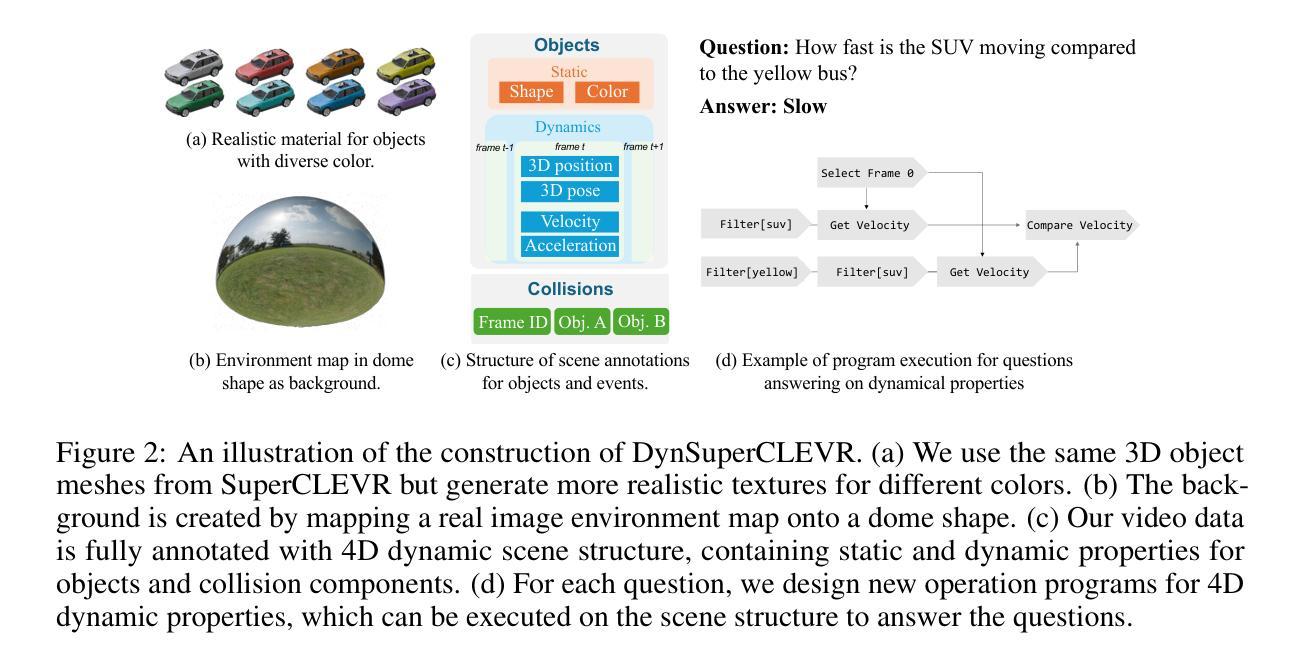
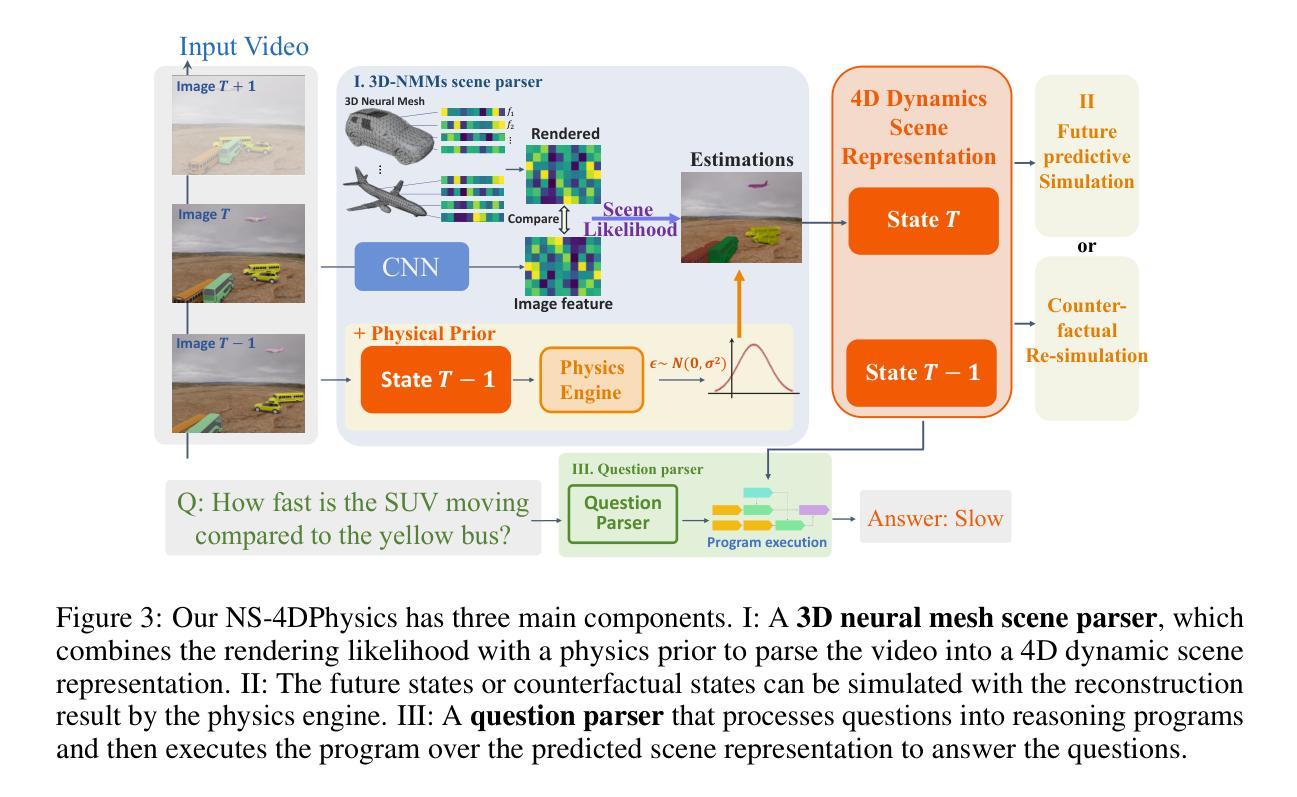