⚠️ 以下所有内容总结都来自于 大语言模型的能力,如有错误,仅供参考,谨慎使用
🔴 请注意:千万不要用于严肃的学术场景,只能用于论文阅读前的初筛!
💗 如果您觉得我们的项目对您有帮助 ChatPaperFree ,还请您给我们一些鼓励!⭐️ HuggingFace免费体验
2025-04-25 更新
OptimAI: Optimization from Natural Language Using LLM-Powered AI Agents
Authors:Raghav Thind, Youran Sun, Ling Liang, Haizhao Yang
Optimization plays a vital role in scientific research and practical applications, but formulating a concrete optimization problem described in natural language into a mathematical form and selecting a suitable solver to solve the problem requires substantial domain expertise. We introduce \textbf{OptimAI}, a framework for solving \underline{Optim}ization problems described in natural language by leveraging LLM-powered \underline{AI} agents, achieving superior performance over current state-of-the-art methods. Our framework is built upon four key roles: (1) a \emph{formulator} that translates natural language problem descriptions into precise mathematical formulations; (2) a \emph{planner} that constructs a high-level solution strategy prior to execution; and (3) a \emph{coder} and a \emph{code critic} capable of interacting with the environment and reflecting on outcomes to refine future actions. Ablation studies confirm that all roles are essential; removing the planner or code critic results in $5.8\times$ and $3.1\times$ drops in productivity, respectively. Furthermore, we introduce UCB-based debug scheduling to dynamically switch between alternative plans, yielding an additional $3.3\times$ productivity gain. Our design emphasizes multi-agent collaboration, allowing us to conveniently explore the synergistic effect of combining diverse models within a unified system. Our approach attains 88.1% accuracy on the NLP4LP dataset and 71.2% on the Optibench (non-linear w/o table) subset, reducing error rates by 58% and 50% respectively over prior best results.
优化在科学研究和实际应用中发挥着至关重要的作用,然而,将自然语言描述的优化问题转化为数学形式并选择适当的求解器来解决问题需要大量的专业知识。我们引入了OptimAI框架,该框架利用大型语言模型驱动的AI代理解决自然语言描述的优化问题,相较于当前最先进的方法,展现了卓越的性能。我们的框架基于四个关键角色:一是将自然语言问题描述翻译成精确数学形式的表述器;二是在执行前构建高级解决方案策略的规划器;三是能够与环境互动并反思结果以改进未来行动的编码者和代码评论家。消融研究证实,所有角色都是必不可少的;移除规划者或代码评论家分别会导致生产力下降$5.8\times$和$3.1\times$。此外,我们引入了基于UCB的调试调度来动态切换不同计划,产生了额外的$3.3\times$生产力增益。我们的设计强调多智能体协作,使我们能够方便地探索在统一系统中结合不同模型的协同作用。我们的方法在NLP4LP数据集上达到了88.1%的准确率,在Optibench(非线性无表)子集上达到了71.2%的准确率,相比之前的最佳结果,误差率分别降低了58%和50%。
论文及项目相关链接
摘要
优化在自然语言描述和数学形式转换以及选择合适的求解器解决实际问题中起到关键作用。我们介绍了OptimAI框架,利用LLM驱动的AI代理解决自然语言描述的优化问题,实现优于现有最新方法的性能。该框架建立在四个关键角色上:问题表述器、规划器、编码器和代码评论家。这些角色对于框架的性能至关重要,缺少规划器或代码评论家将导致生产力下降5.8倍和3.1倍。此外,我们引入了基于UCB的调试调度策略来动态切换不同计划,带来了额外的3.3倍生产力增长。该设计强调多代理协作,能够在统一系统中方便地探索不同模型的协同作用效果。我们的方法在NLP4LP数据集上达到88.1%的准确率,在Optibench(非线性不含表格)子集上达到71.2%的准确率,相比之前最佳结果降低了错误率分别高达58%和50%。框架凭借先进的多层次智能决策和精细化协作机制展现了巨大的潜力,可为各类优化问题提供高效解决方案。
关键见解
- OptimAI框架利用自然语言描述解决优化问题,展示了卓越性能。
- 框架建立在四个关键角色上:问题表述器、规划器、编码器和代码评论家,每个角色都至关重要。
- 基于UCB的调试调度策略提高了生产力。
- 多代理协作设计便于在统一系统中探索不同模型的协同作用。
- 在NLP4LP和Optibench数据集上的准确率高于先前最佳结果。
- 框架为各类优化问题提供了高效解决方案的潜力。
点此查看论文截图
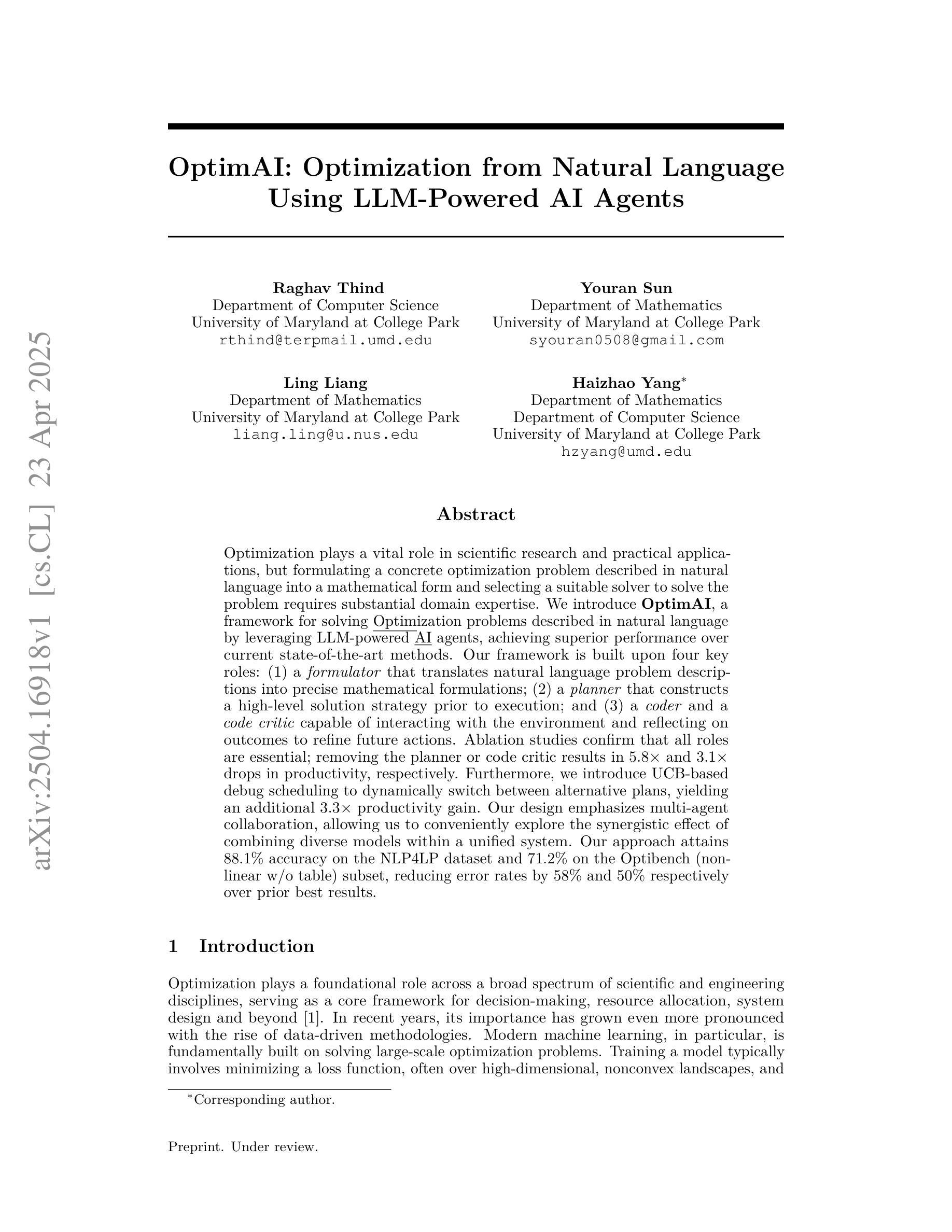
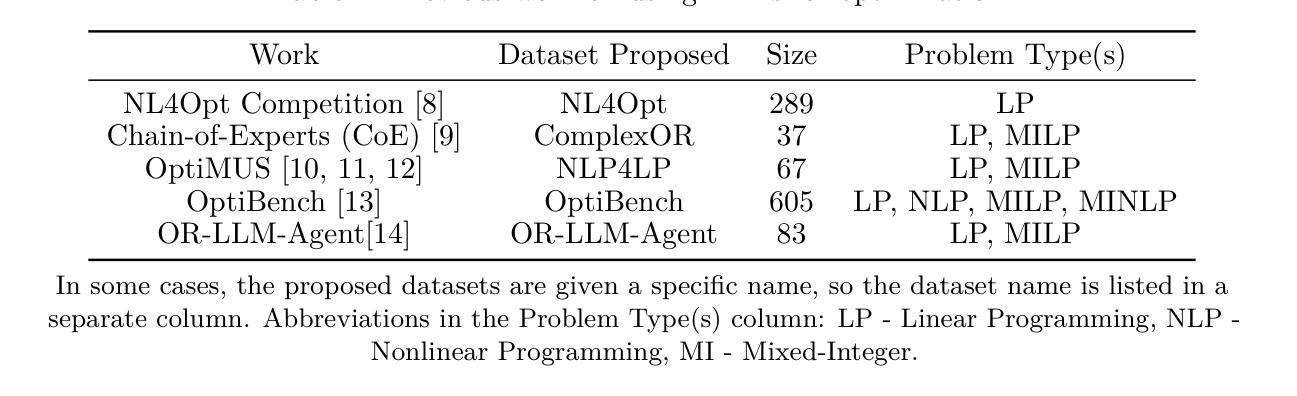
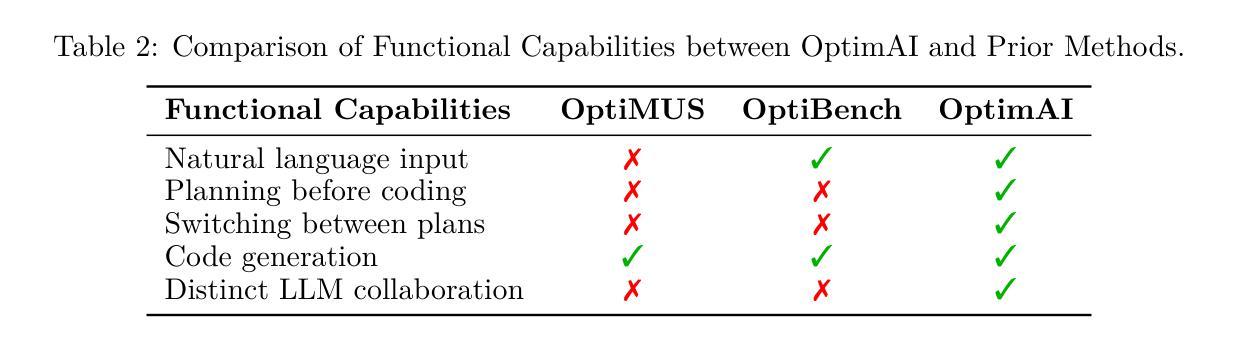
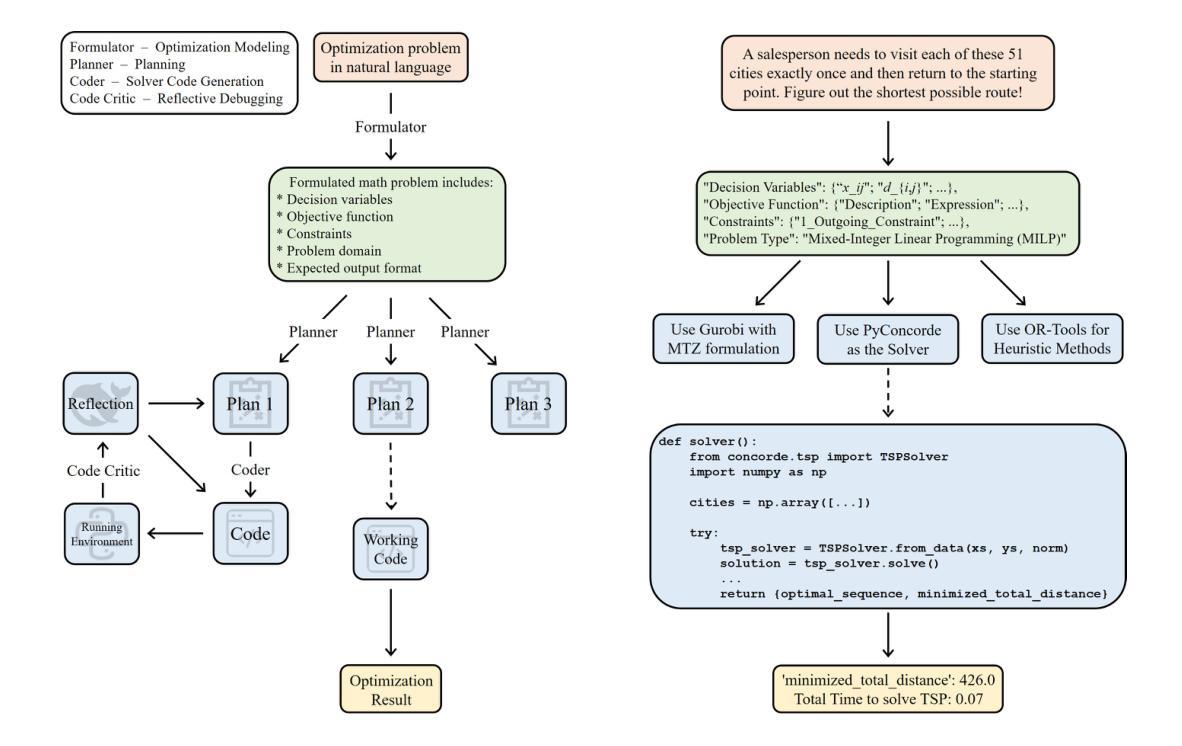
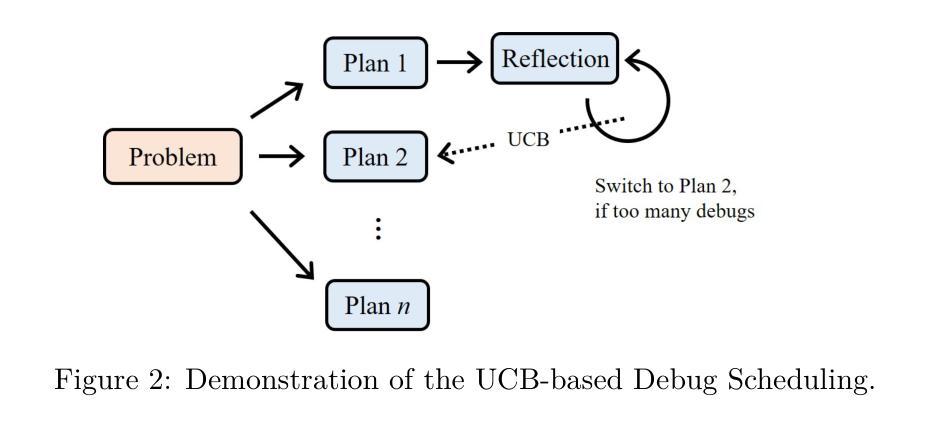
Building A Secure Agentic AI Application Leveraging A2A Protocol
Authors:Idan Habler, Ken Huang, Vineeth Sai Narajala, Prashant Kulkarni
As Agentic AI systems evolve from basic workflows to complex multi agent collaboration, robust protocols such as Google’s Agent2Agent (A2A) become essential enablers. To foster secure adoption and ensure the reliability of these complex interactions, understanding the secure implementation of A2A is essential. This paper addresses this goal by providing a comprehensive security analysis centered on the A2A protocol. We examine its fundamental elements and operational dynamics, situating it within the framework of agent communication development. Utilizing the MAESTRO framework, specifically designed for AI risks, we apply proactive threat modeling to assess potential security issues in A2A deployments, focusing on aspects such as Agent Card management, task execution integrity, and authentication methodologies. Based on these insights, we recommend practical secure development methodologies and architectural best practices designed to build resilient and effective A2A systems. Our analysis also explores how the synergy between A2A and the Model Context Protocol (MCP) can further enhance secure interoperability. This paper equips developers and architects with the knowledge and practical guidance needed to confidently leverage the A2A protocol for building robust and secure next generation agentic applications.
随着Agentic AI系统从基本工作流程发展到复杂的跨代理协作,像Google的Agent2Agent(A2A)这样的稳健协议变得至关重要。为了促进安全采用并确保这些复杂交互的可靠性,理解A2A的安全实施至关重要。本文旨在通过围绕A2A协议进行全面安全分析来实现这一目标。我们研究了其基础要素和操作动态,将其置于代理通信发展的框架内。我们利用专门为人工智能风险设计的MAESTRO框架,采用主动威胁建模来评估A2A部署中的潜在安全问题,重点关注代理卡管理、任务执行完整性和认证方法等方面。基于这些见解,我们提出了实用的安全开发方法和架构最佳实践,旨在构建具有弹性和有效的A2A系统。我们的分析还探讨了A2A与模型上下文协议(MCP)之间的协同作用如何进一步增强安全互操作性。本文旨在为开发人员和架构师提供知识和实践指导,使他们能够有信心地利用A2A协议构建强大而安全的下一代Agentic应用程序。
论文及项目相关链接
PDF 13 pages, 4 figures, 1 table, Authors contributed equally to this work
Summary
随着Agentic AI系统从基本工作流程演变到复杂的多智能体协作,诸如Google的Agent2Agent(A2A)等稳健的协议变得至关重要。本文旨在通过全面分析A2A协议的安全实施,促进安全采用和确保这些复杂交互的可靠性。我们利用专为人工智能风险设计的MAESTRO框架,采用主动威胁建模评估A2A部署中的潜在安全问题。基于这些见解,我们提出了实用的安全开发方法和架构最佳实践,以构建具有弹性和有效的A2A系统。本文的分析还探讨了A2A与模型上下文协议(MCP)之间的协同作用如何进一步提高安全互操作性。
Key Takeaways
- Agent2Agent (A2A) 协议在多智能体协作中扮演关键角色。
- A2A协议的安全实施对于确保智能体系统的可靠和复杂交互至关重要。
- 利用MAESTRO框架进行主动威胁建模,以评估A2A协议的安全问题。
- A2A协议的关键要素包括Agent Card管理、任务执行完整性和认证方法。
- 开发者需掌握安全开发方法和架构最佳实践来构建稳健和有效的A2A系统。
- A2A协议与模型上下文协议(MCP)的结合可提高安全互操作性。
点此查看论文截图
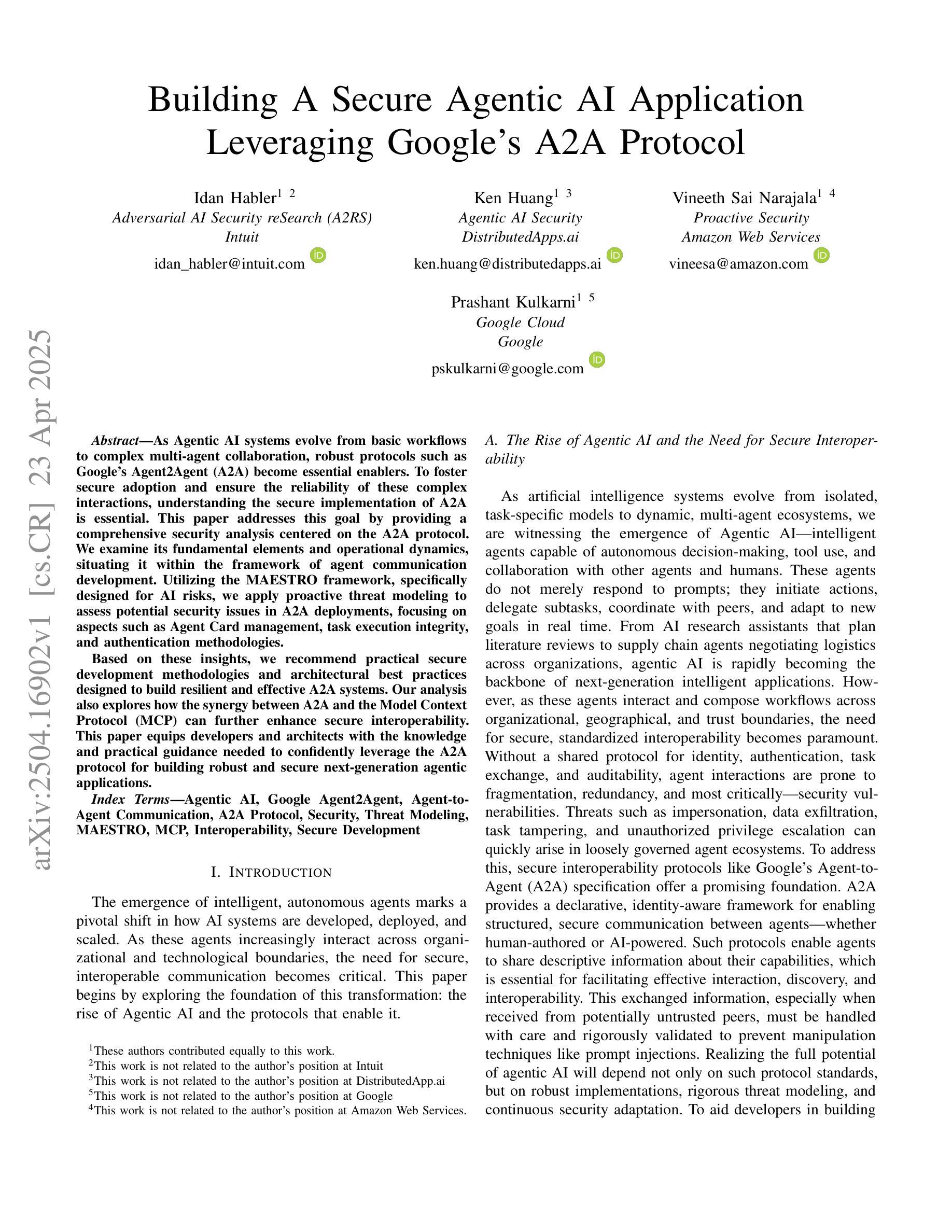
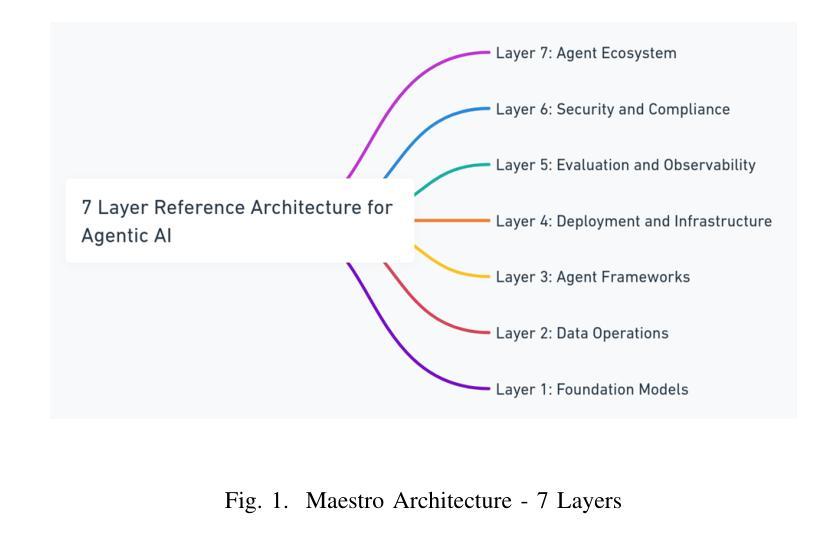
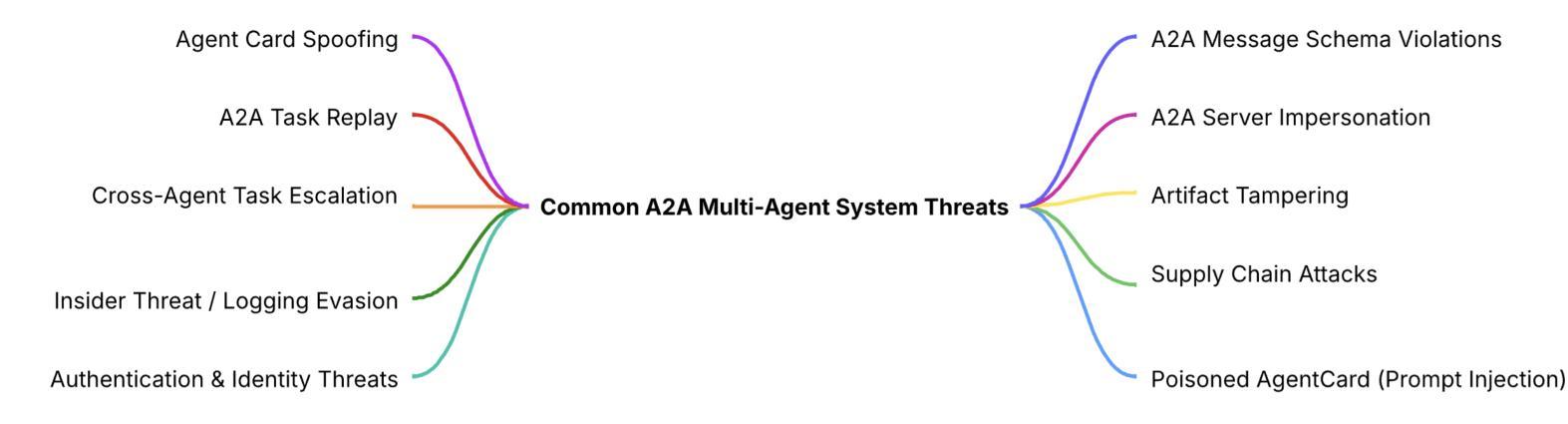
Enhancing LLM-Based Agents via Global Planning and Hierarchical Execution
Authors:Junjie Chen, Haitao Li, Jingli Yang, Yiqun Liu, Qingyao Ai
Intelligent agent systems based on Large Language Models (LLMs) have shown great potential in real-world applications. However, existing agent frameworks still face critical limitations in task planning and execution, restricting their effectiveness and generalizability. Specifically, current planning methods often lack clear global goals, leading agents to get stuck in local branches, or produce non-executable plans. Meanwhile, existing execution mechanisms struggle to balance complexity and stability, and their limited action space restricts their ability to handle diverse real-world tasks. To address these limitations, we propose GoalAct, a novel agent framework that introduces a continuously updated global planning mechanism and integrates a hierarchical execution strategy. GoalAct decomposes task execution into high-level skills, including searching, coding, writing and more, thereby reducing planning complexity while enhancing the agents’ adaptability across diverse task scenarios. We evaluate GoalAct on LegalAgentBench, a benchmark with multiple types of legal tasks that require the use of multiple types of tools. Experimental results demonstrate that GoalAct achieves state-of-the-art (SOTA) performance, with an average improvement of 12.22% in success rate. These findings highlight GoalAct’s potential to drive the development of more advanced intelligent agent systems, making them more effective across complex real-world applications. Our code can be found at https://github.com/cjj826/GoalAct.
基于大型语言模型(LLM)的智能代理系统在实际应用中显示出巨大潜力。然而,现有的代理框架在任务规划和执行方面仍存在关键限制,限制了其有效性和通用性。具体来说,当前的规划方法通常缺乏明确的全球目标,导致代理陷入局部分支,或产生不可执行的计划。同时,现有的执行机制在平衡复杂性和稳定性方面面临困难,其有限的行动空间限制了其处理各种实际任务的能力。为了解决这些限制,我们提出了GoalAct,这是一种新的代理框架,它引入了一种持续更新的全球规划机制,并集成了一种分层执行策略。GoalAct将任务执行分解为高级技能,包括搜索、编码、写作等,从而降低了规划复杂性,同时提高了代理在不同任务场景中的适应性。我们在LegalAgentBench上评估了GoalAct,这是一个需要使用多种工具完成多种类型法律任务的基准测试。实验结果表明,GoalAct达到了最先进的性能,成功率平均提高了12.22%。这些发现突出了GoalAct在推动更先进智能代理系统发展方面的潜力,使其能够在复杂的实际应用中更加有效地发挥作用。我们的代码可在https://github.com/cjj826/GoalAct找到。
论文及项目相关链接
Summary
基于大型语言模型(LLM)的智能代理系统在实际应用中显示出巨大潜力。然而,现有代理框架在任务规划和执行方面仍存在关键限制,限制了其有效性和通用性。为解决这些问题,本文提出GoalAct框架,引入持续更新的全局规划机制,并整合分层执行策略。GoalAct将任务执行分解为高级技能,包括搜索、编码、写作等,降低规划复杂性,提高代理在不同任务场景中的适应性。在LegalAgentBench基准测试上,GoalAct取得了最新技术成果,成功率平均提高12.22%。
Key Takeaways
- 智能代理系统在实际应用中具有巨大潜力,但现有框架在任务规划和执行方面存在限制。
- 当前规划方法缺乏明确的全局目标,导致代理陷入局部分支或产生不可执行的计划。
- 现有执行机制在平衡复杂性和稳定性方面存在困难,有限的行动空间限制了处理多样化现实任务的能力。
- GoalAct框架引入持续更新的全局规划机制和分层执行策略,以提高代理的适应性和效率。
- GoalAct将任务执行分解为高级技能,包括搜索、编码、写作等,降低规划复杂性。
- 在LegalAgentBench基准测试上,GoalAct取得了最新技术成果,成功率有所提高。
点此查看论文截图
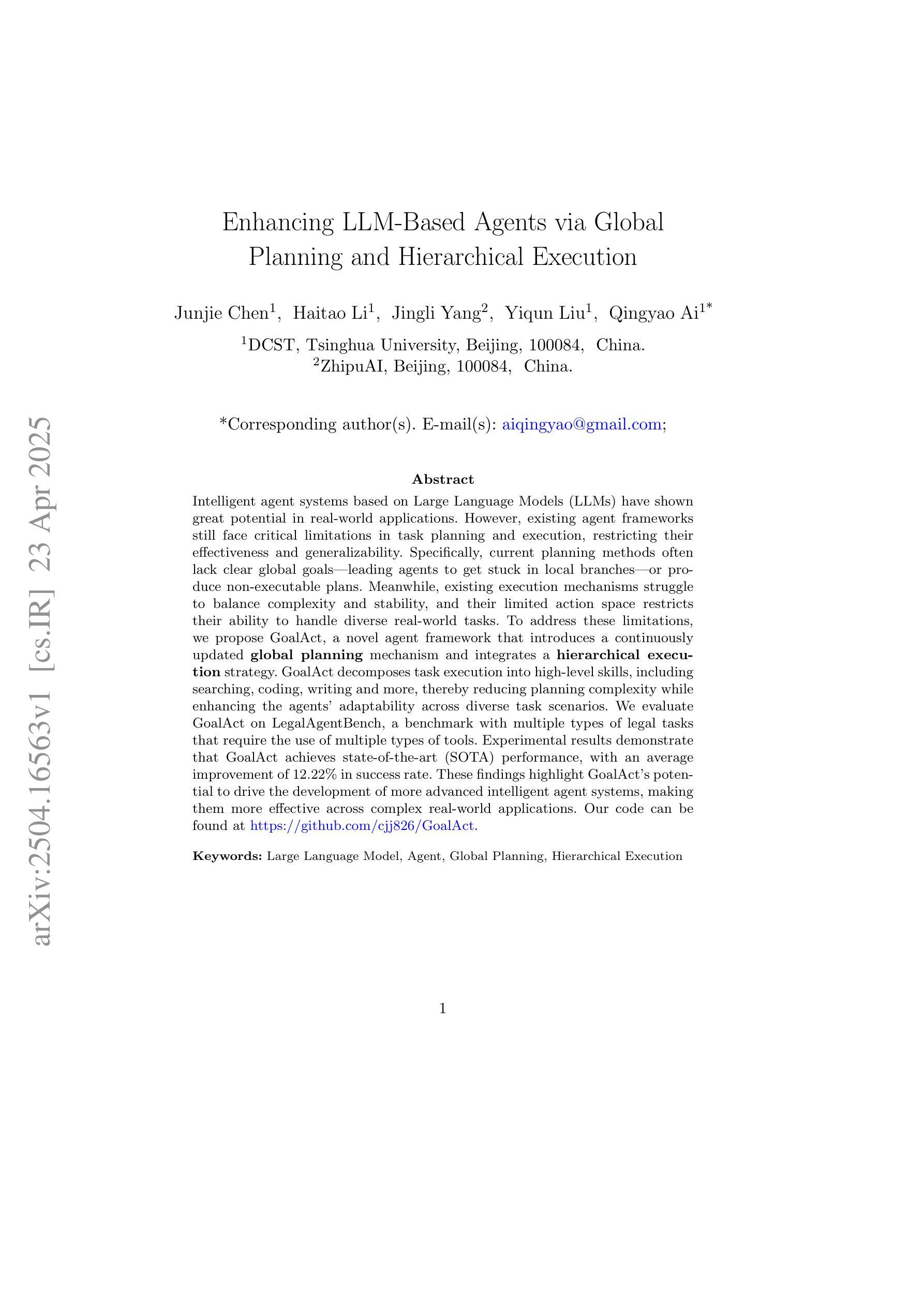
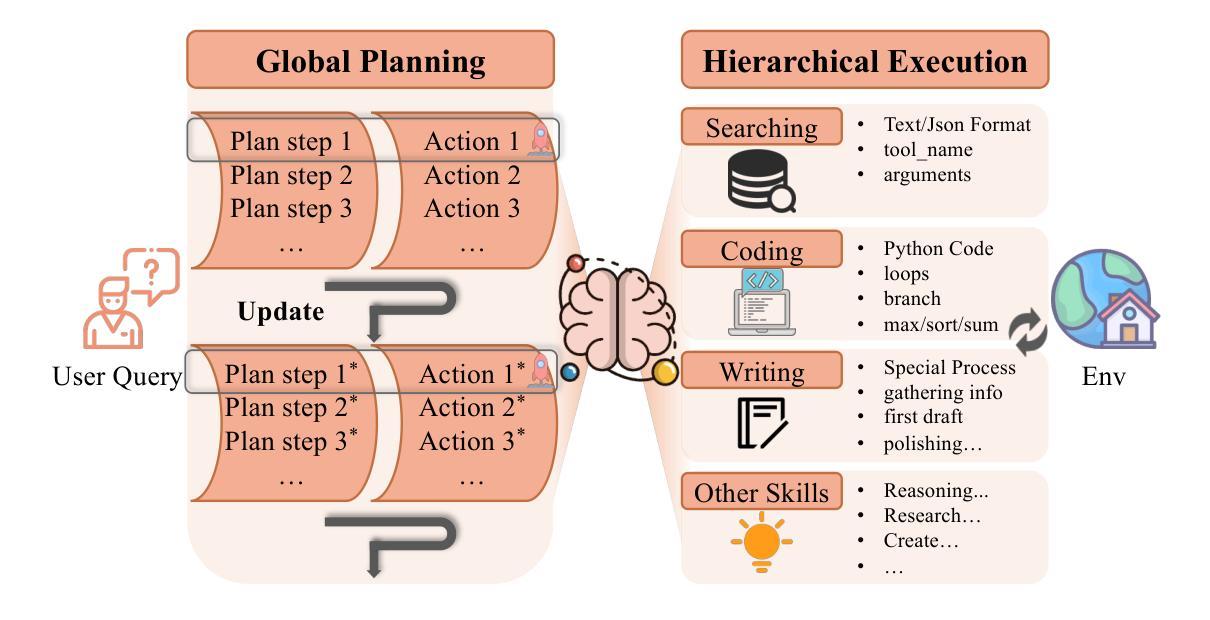
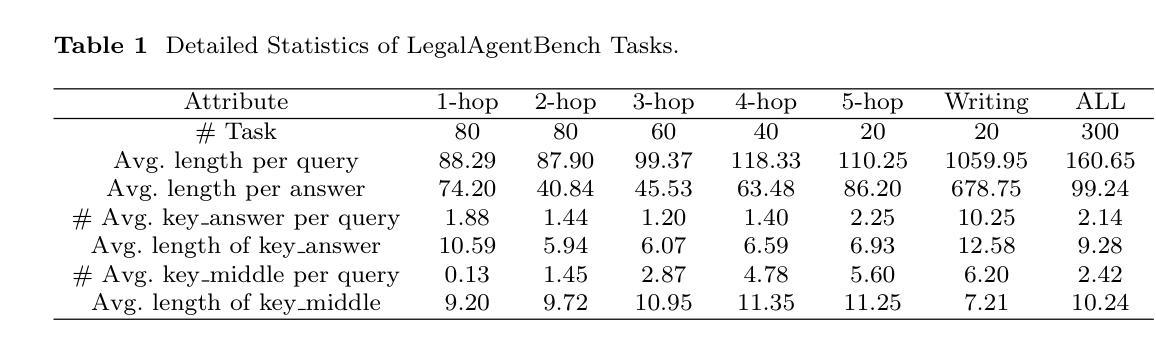
A Survey of Foundation Model-Powered Recommender Systems: From Feature-Based, Generative to Agentic Paradigms
Authors:Chengkai Huang, Hongtao Huang, Tong Yu, Kaige Xie, Junda Wu, Shuai Zhang, Julian Mcauley, Dietmar Jannach, Lina Yao
Recommender systems (RS) have become essential in filtering information and personalizing content for users. RS techniques have traditionally relied on modeling interactions between users and items as well as the features of content using models specific to each task. The emergence of foundation models (FMs), large scale models trained on vast amounts of data such as GPT, LLaMA and CLIP, is reshaping the recommendation paradigm. This survey provides a comprehensive overview of the Foundation Models for Recommender Systems (FM4RecSys), covering their integration in three paradigms: (1) Feature-Based augmentation of representations, (2) Generative recommendation approaches, and (3) Agentic interactive systems. We first review the data foundations of RS, from traditional explicit or implicit feedback to multimodal content sources. We then introduce FMs and their capabilities for representation learning, natural language understanding, and multi-modal reasoning in RS contexts. The core of the survey discusses how FMs enhance RS under different paradigms. Afterward, we examine FM applications in various recommendation tasks. Through an analysis of recent research, we highlight key opportunities that have been realized as well as challenges encountered. Finally, we outline open research directions and technical challenges for next-generation FM4RecSys. This survey not only reviews the state-of-the-art methods but also provides a critical analysis of the trade-offs among the feature-based, the generative, and the agentic paradigms, outlining key open issues and future research directions.
推荐系统(RS)在信息过滤和用户内容个性化方面已成为不可或缺的技术。传统的推荐系统技术主要依赖于对用户与项目之间的交互以及内容特征的建模,并使用了针对每项任务特有的模型。基础模型(FMs)的出现,如GPT、LLaMA和CLIP等大型模型,在大量数据上进行训练,正在重塑推荐系统的格局。本文全面概述了用于推荐系统的基础模型(FM4RecSys),涵盖了它们在三种范式中的集成:(1)基于特征的表示增强,(2)生成式推荐方法,(3)智能交互系统。首先,我们回顾了推荐系统的数据基础,从传统的显式或隐式反馈到多模态内容源。然后,我们介绍了基础模型及其在推荐系统中的表示学习、自然语言理解和多模态推理的能力。本文的核心是讨论不同范式下基础模型如何增强推荐系统。之后,我们分析了基础模型在各种推荐任务中的应用。通过对最近的研究进行分析,我们强调了已实现的关键机遇以及遇到的挑战。最后,我们概述了下一代FM4RecSys的开放研究方向和技术挑战。本文不仅回顾了最新方法,还深入分析了基于特征、生成和智能范式之间的权衡,并指出了关键开放问题和未来研究方向。
论文及项目相关链接
Summary
基于推荐系统的重要性以及其在过滤信息、个性化内容方面的应用,本文调查了推荐系统的现状。传统推荐系统技术主要依赖于针对每个任务特定建模的用户与物品之间的交互以及内容特征。随着大型模型的兴起,如GPT、LLaMA和CLIP等,推荐系统的范式正在发生变革。本文全面概述了推荐系统的基石模型(FM4RecSys),探讨了其在特征基于的表示、生成推荐方法和代理交互系统三种范式中的集成。文章首先回顾了推荐系统的数据基础,从传统的显式或隐式反馈到多模态内容源。然后介绍了基石模型及其在推荐系统上下文中的表示学习、自然语言理解和多模态推理的能力。文章的核心部分讨论了不同范式下FM如何增强推荐系统的功能。最后,我们通过分析最近的研究,指出了应用中的关键机会和所遇到的挑战,并概述了下一代FM4RecSys开放的研究方向和技术挑战。本文不仅回顾了最新方法,还对特征基于的、生成的和代理的范式进行了关键分析,指出了开放问题和未来的研究方向。
Key Takeaways
- 推荐系统已经成为过滤信息和个性化内容的重要工具。
- 传统推荐系统技术主要依赖于针对每个任务的特定模型。
- 基石模型(FMs)的出现正在改变推荐系统的范式。
- FMs在推荐系统中的集成包括特征基于的表示、生成推荐方法和代理交互系统三种范式。
- FMs通过不同的范式增强了推荐系统的功能。
- 最近的研究指出了推荐系统应用中的关键机会和挑战。
点此查看论文截图
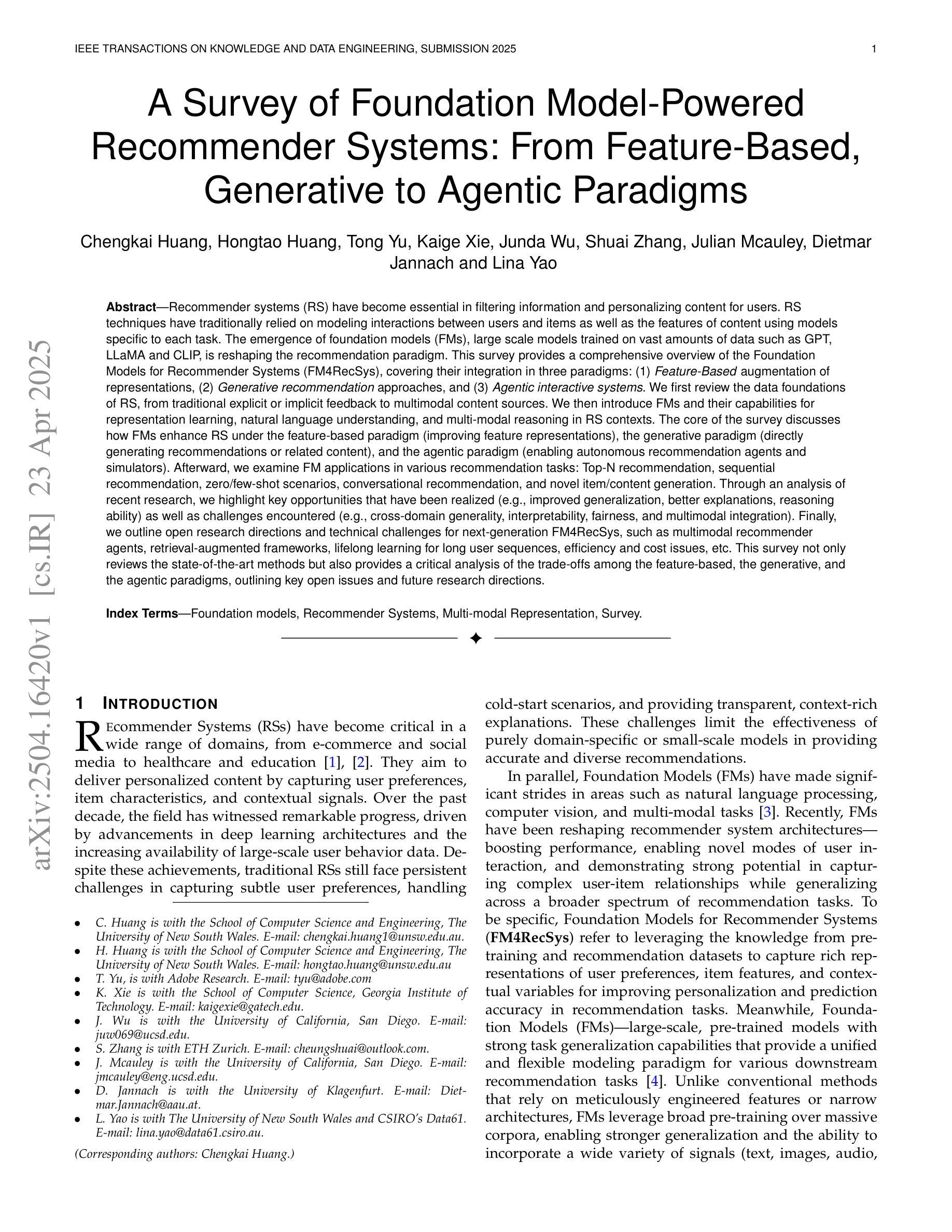
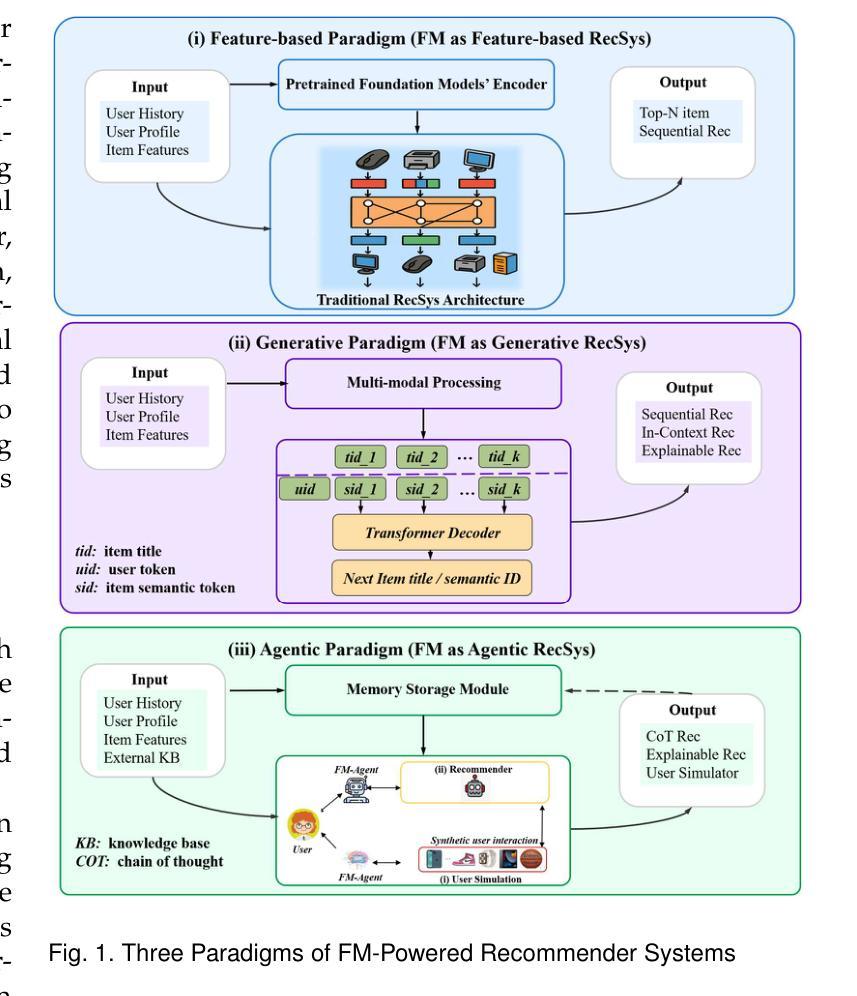
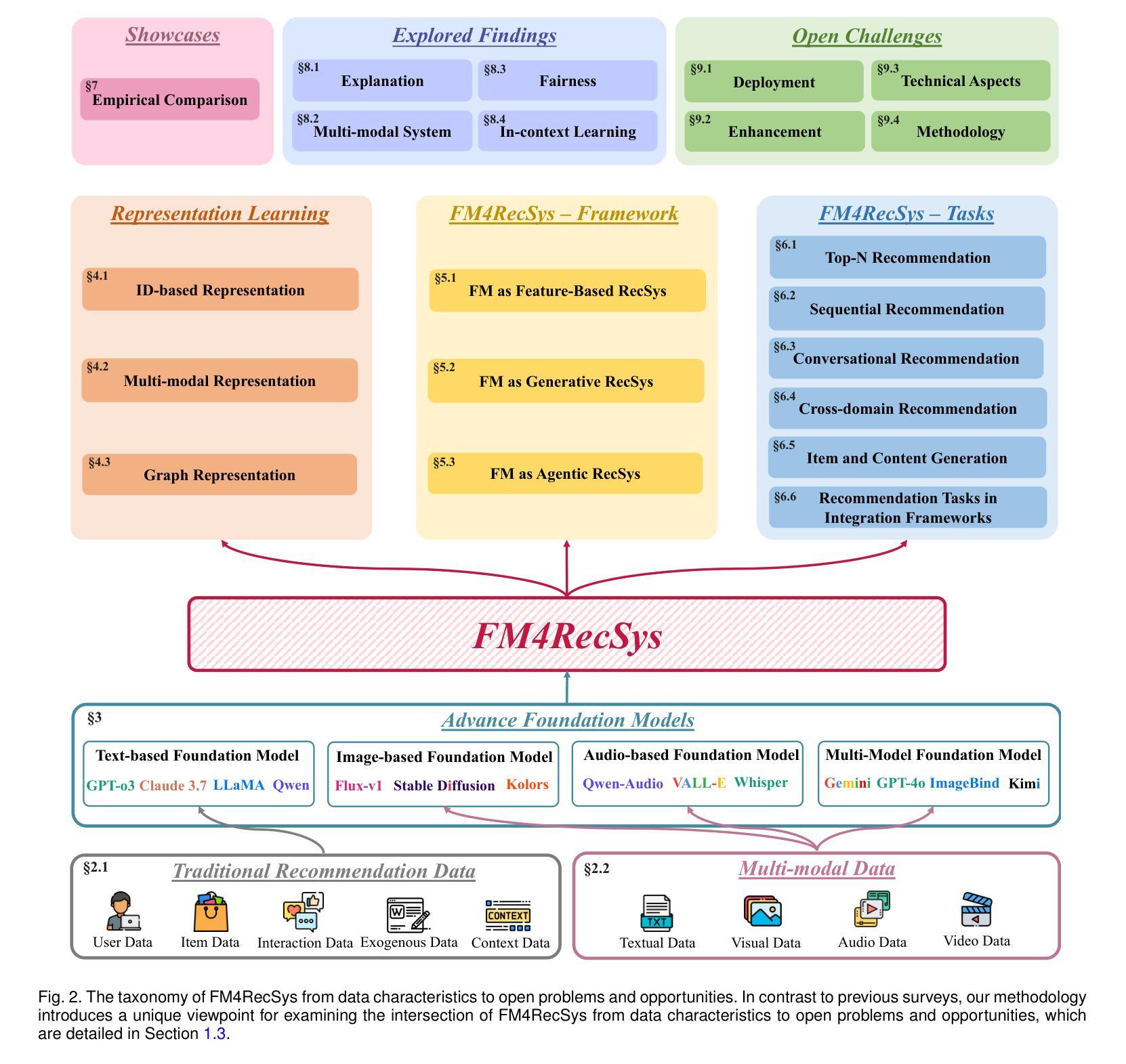
Less is More: Enhancing Structured Multi-Agent Reasoning via Quality-Guided Distillation
Authors:Jiahao Yuan, Xingzhe Sun, Xing Yu, Jingwen Wang, Dehui Du, Zhiqing Cui, Zixiang Di
The XLLM@ACL2025 Shared Task-III formulates a low-resource structural reasoning task that challenges LLMs to generate interpretable, step-by-step rationales with minimal labeled data. We present Less is More, the third-place winning approach in the XLLM@ACL2025 Shared Task-III, which focuses on structured reasoning from only 24 labeled examples. Our approach leverages a multi-agent framework with reverse-prompt induction, retrieval-augmented reasoning synthesis via GPT-4o, and dual-stage reward-guided filtering to distill high-quality supervision across three subtasks: question parsing, CoT parsing, and step-level verification. All modules are fine-tuned from Meta-Llama-3-8B-Instruct under a unified LoRA+ setup. By combining structure validation with reward filtering across few-shot and zero-shot prompts, our pipeline consistently improves structure reasoning quality. These results underscore the value of controllable data distillation in enhancing structured inference under low-resource constraints. Our code is available at https://github.com/Jiahao-Yuan/Less-is-More.
XLLM@ACL2025共享任务III设定了一个低资源结构推理任务,该任务挑战了大型语言模型在少量标注数据的情况下生成可解释的、逐步的理性推理。我们提出了”少即是多”的方法,这是XLLM@ACL2025共享任务III的季军获奖方案,它专注于仅使用24个标注示例进行结构化推理。我们的方法利用多智能体框架进行逆向提示归纳,通过GPT-4o增强推理合成,以及双阶段奖励引导过滤,以提炼三个子任务的高质量监督:问题解析、CoT解析和步骤级验证。所有模块都是在统一的LoRA+设置下,基于Meta-Llama-3-8B-Instruct进行微调。通过结合结构验证和奖励过滤在少数和零样本提示中,我们的管道在结构推理质量上始终有所提高。这些结果强调了可控数据蒸馏在增强低资源约束下的结构化推理中的价值。我们的代码可在https://github.com/Jiahao-Yuan/Less-is-More找到。
论文及项目相关链接
Summary
本文介绍了在XLLM@ACL2025共享任务III中提出的挑战,即低资源结构推理任务。文章重点介绍了在该任务中获得第三名的“Less is More”方法,该方法仅使用24个标注样本进行结构化推理。该方法采用多智能体框架、反向提示归纳、基于GPT-4o的检索增强推理合成以及两阶段奖励引导过滤等技术,提高监督质量。所有模块都在统一的LoRA+设置下,基于Meta-Llama-3-8B-Instruct进行微调。通过结合结构验证和奖励过滤,在少量样本和零样本提示下,该管道持续提高结构推理质量。该研究突显了可控数据蒸馏在增强低资源约束下的结构化推理中的价值。
Key Takeaways
- XLLM@ACL2025 Shared Task-III 面向低资源结构推理任务,需要生成具有可解释性的、逐步的理性依据,且仅使用少量标注数据。
- “Less is More”方法在该任务中获得第三名,仅使用24个标注样本进行结构化推理。
- 该方法采用多智能体框架,结合反向提示归纳、检索增强推理合成及两阶段奖励引导过滤等技术,以提高监督质量。
- 方法结合了结构验证和奖励过滤,提高了结构推理质量,特别是在少量样本和零样本提示下。
- 所有模块在统一的LoRA+设置下基于Meta-Llama-3-8B-Instruct微调。
- 研究强调了可控数据蒸馏在增强结构化推理中的价值,特别是在低资源约束条件下。
点此查看论文截图
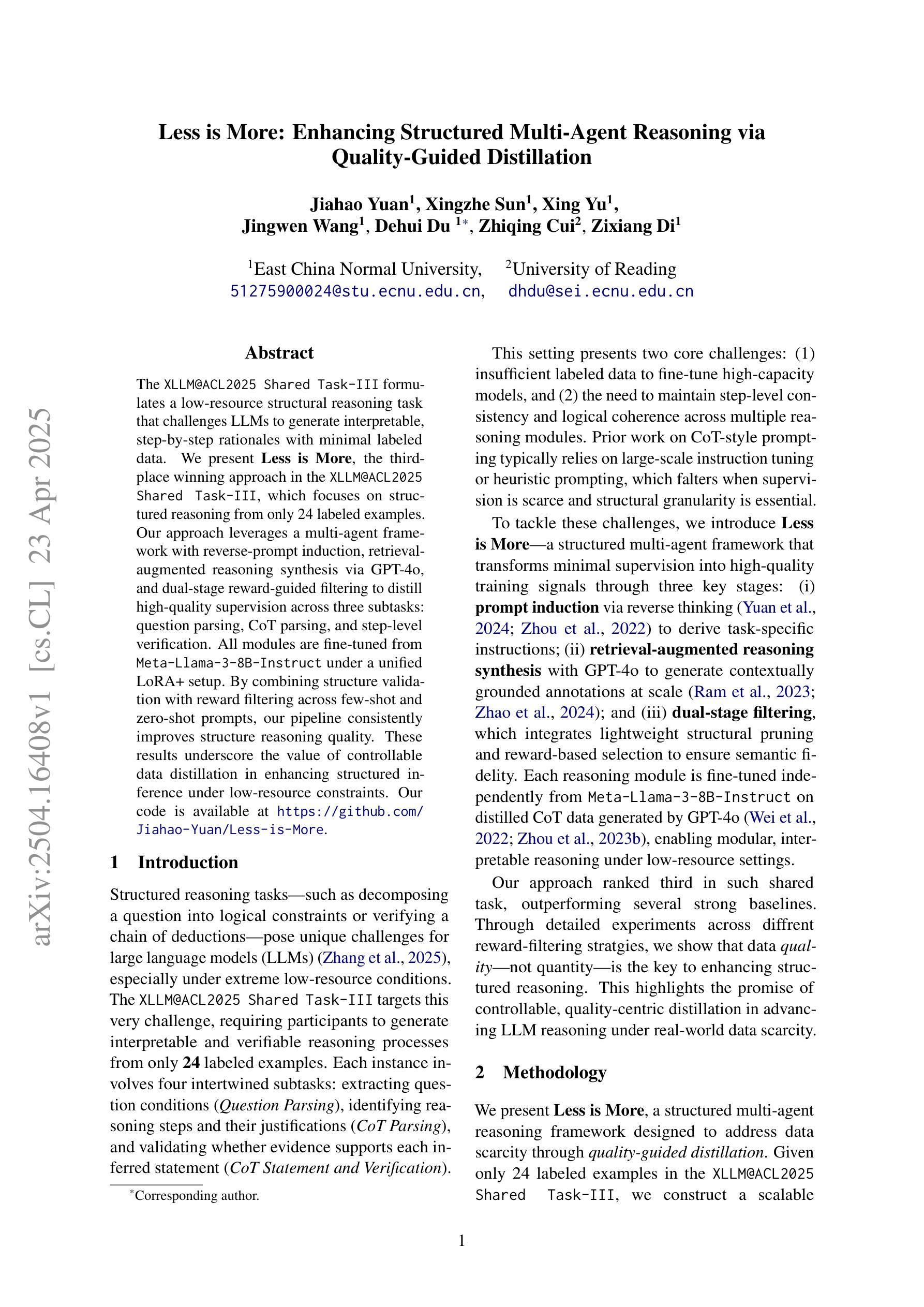
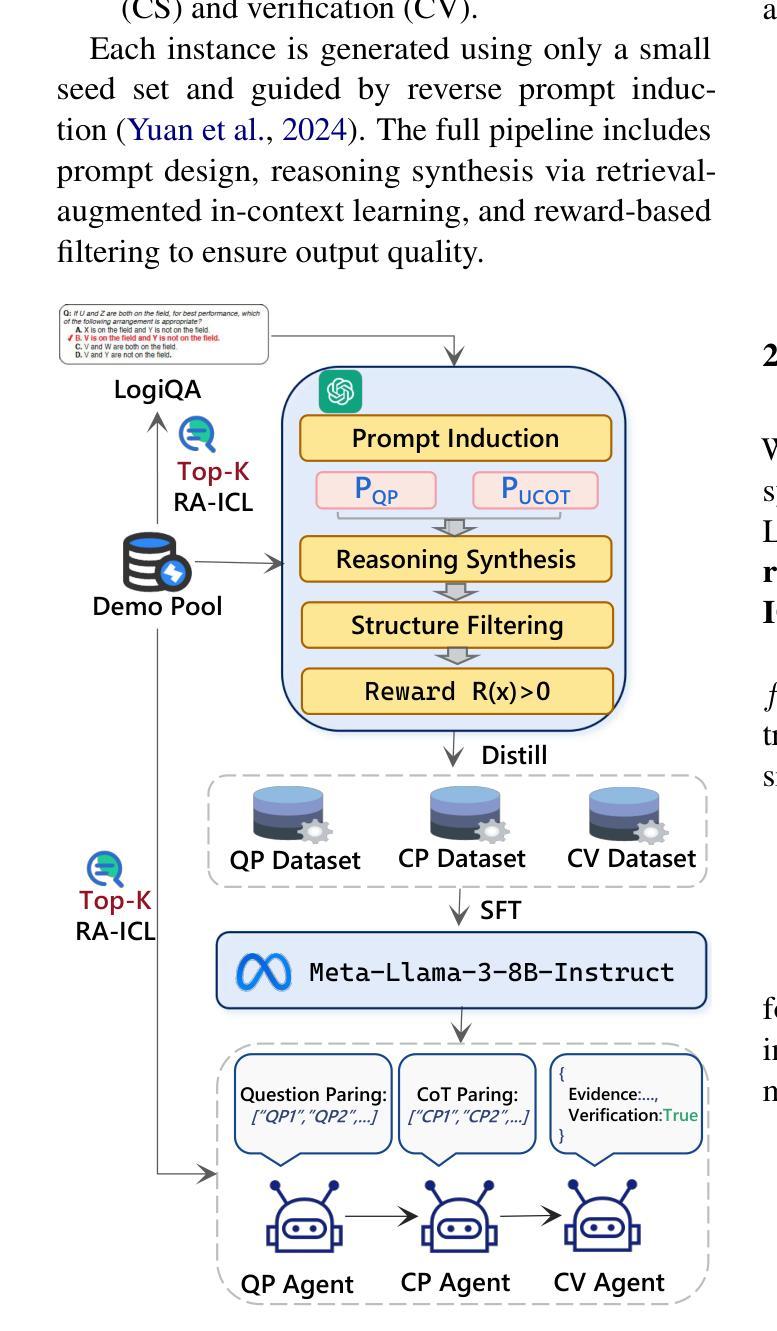
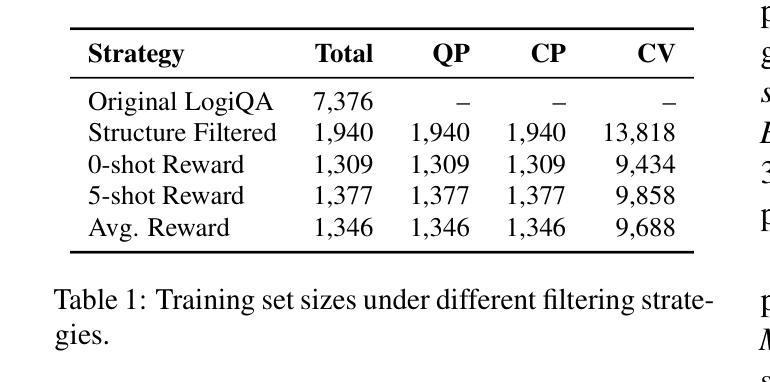
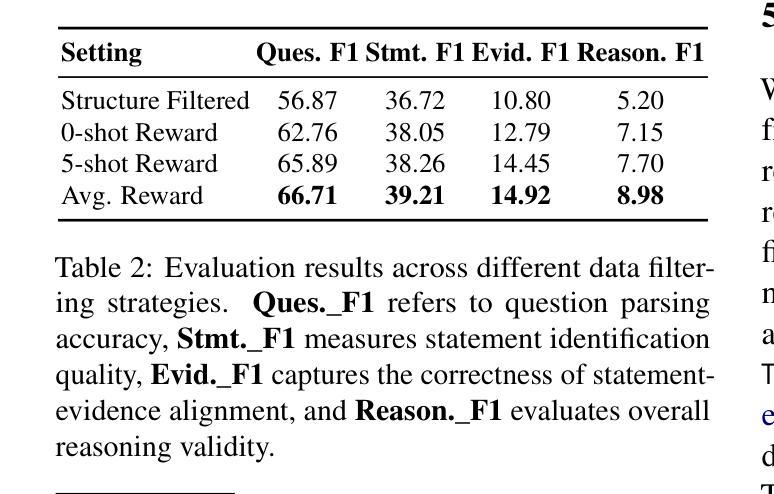
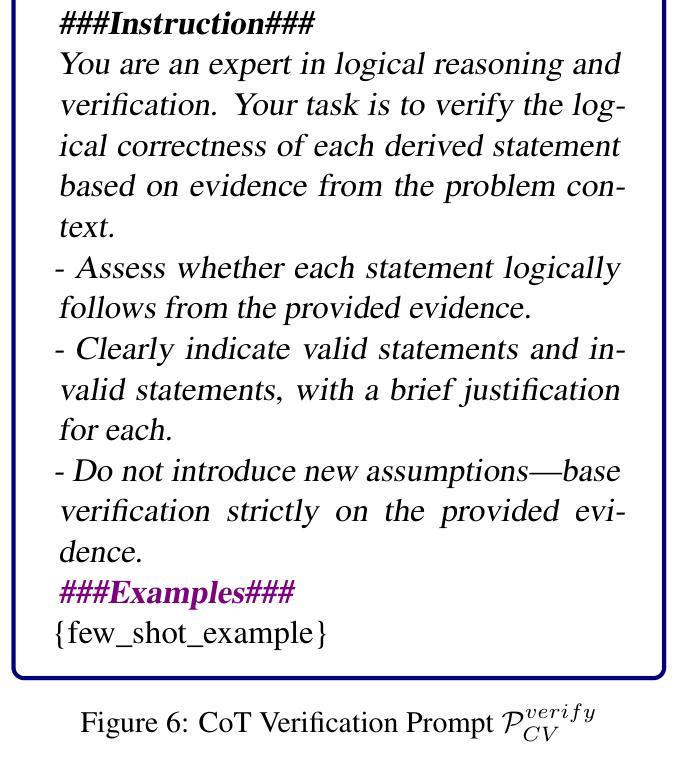