⚠️ 以下所有内容总结都来自于 大语言模型的能力,如有错误,仅供参考,谨慎使用
🔴 请注意:千万不要用于严肃的学术场景,只能用于论文阅读前的初筛!
💗 如果您觉得我们的项目对您有帮助 ChatPaperFree ,还请您给我们一些鼓励!⭐️ HuggingFace免费体验
2025-04-25 更新
VideoMark: A Distortion-Free Robust Watermarking Framework for Video Diffusion Models
Authors:Xuming Hu, Hanqian Li, Jungang Li, Aiwei Liu
This work presents VideoMark, a training-free robust watermarking framework for video diffusion models. As diffusion models advance in generating highly realistic videos, the need for reliable content attribution mechanisms has become critical. While watermarking techniques for image diffusion models have made progress, directly extending these methods to videos presents unique challenges due to variable video lengths and vulnerability to temporal attacks. VideoMark addresses these limitations through a frame-wise watermarking strategy using pseudorandom error correction (PRC) codes to embed watermark information during the generation process. Our method generates an extended watermark message sequence and randomly selects starting positions for each video, ensuring uniform noise distribution in the latent space and maintaining generation quality. For watermark extraction, we introduce a Temporal Matching Module (TMM) that uses edit distance to align decoded messages with the original watermark sequence, providing robustness against temporal attacks such as frame deletion. Experimental results demonstrate that VideoMark achieves higher decoding accuracy than existing methods while maintaining video quality on par with watermark-free generation. Importantly, our watermark remains undetectable to attackers without the secret key, ensuring strong imperceptibility compared to other watermarking frameworks. VideoMark provides a practical solution for content attribution in diffusion-based video generation without requiring additional training or compromising video quality. Our code and data are available at \href{https://github.com/KYRIE-LI11/VideoMark}{https://github.com/KYRIE-LI11/VideoMark}.
本文介绍了VideoMark,这是一种无需训练的视频扩散模型稳健水印框架。随着扩散模型在生成高度逼真视频方面的进展,对可靠的内容归属机制的需求变得至关重要。虽然图像扩散模型的水印技术已经取得了进展,但由于视频长度的可变性和对临时攻击的脆弱性,将这些方法直接扩展到视频上带来了独特的挑战。VideoMark通过基于伪随机纠错(PRC)代码的帧级水印策略来解决这些限制,在生成过程中嵌入水印信息。我们的方法生成扩展的水印消息序列,并随机选择每个视频的起始位置,确保潜在空间中的噪声分布均匀,同时保持生成质量。对于水印提取,我们引入了时序匹配模块(TMM),该模块使用编辑距离来对齐解码消息与原始水印序列,为对抗如帧删除等临时攻击提供了稳健性。实验结果表明,VideoMark在保持视频质量与无水印生成相媲美的同时,实现了比现有方法更高的解码精度。重要的是,没有秘密密钥的攻击者无法检测到我们的水印,与其他水印框架相比,确保了更强的隐蔽性。VideoMark为扩散式视频生成中的内容归属提供了实用解决方案,无需额外的训练或牺牲视频质量。我们的代码和数据可在https://github.com/KYRIE-LI11/VideoMark上找到。
论文及项目相关链接
Summary
本文介绍了VideoMark,一种无需训练的视频扩散模型稳健水印框架。该框架采用伪随机纠错码策略,在生成过程中嵌入水印信息,解决了视频水印面临的挑战,如可变视频长度和易受到时间攻击的问题。VideoMark提供了对内容归属性的实际解决方案,可在无需额外训练的情况下维护视频质量和水印的不可察觉性。其代码和数据集可通过相应链接获取。
Key Takeaways
- VideoMark是一个无需训练的视频扩散模型水印框架,旨在解决视频内容归属权的问题。
- VideoMark采用伪随机纠错码策略嵌入水印信息,确保水印信息的稳定性和鲁棒性。
- VideoMark通过帧级水印策略解决了视频长度可变性和易受时间攻击的问题。
- Temporal Matching Module (TMM)提供了对水印的可靠提取和定位机制,使其对时间攻击具有鲁棒性。
- VideoMark的实验结果证明其解码准确性较高,同时保持了视频质量,并与其他框架相比表现出强不可察觉性。
- VideoMark提供了一个适用于扩散型视频生成的实际解决方案,不需要额外的训练即可实现水印嵌入。
点此查看论文截图
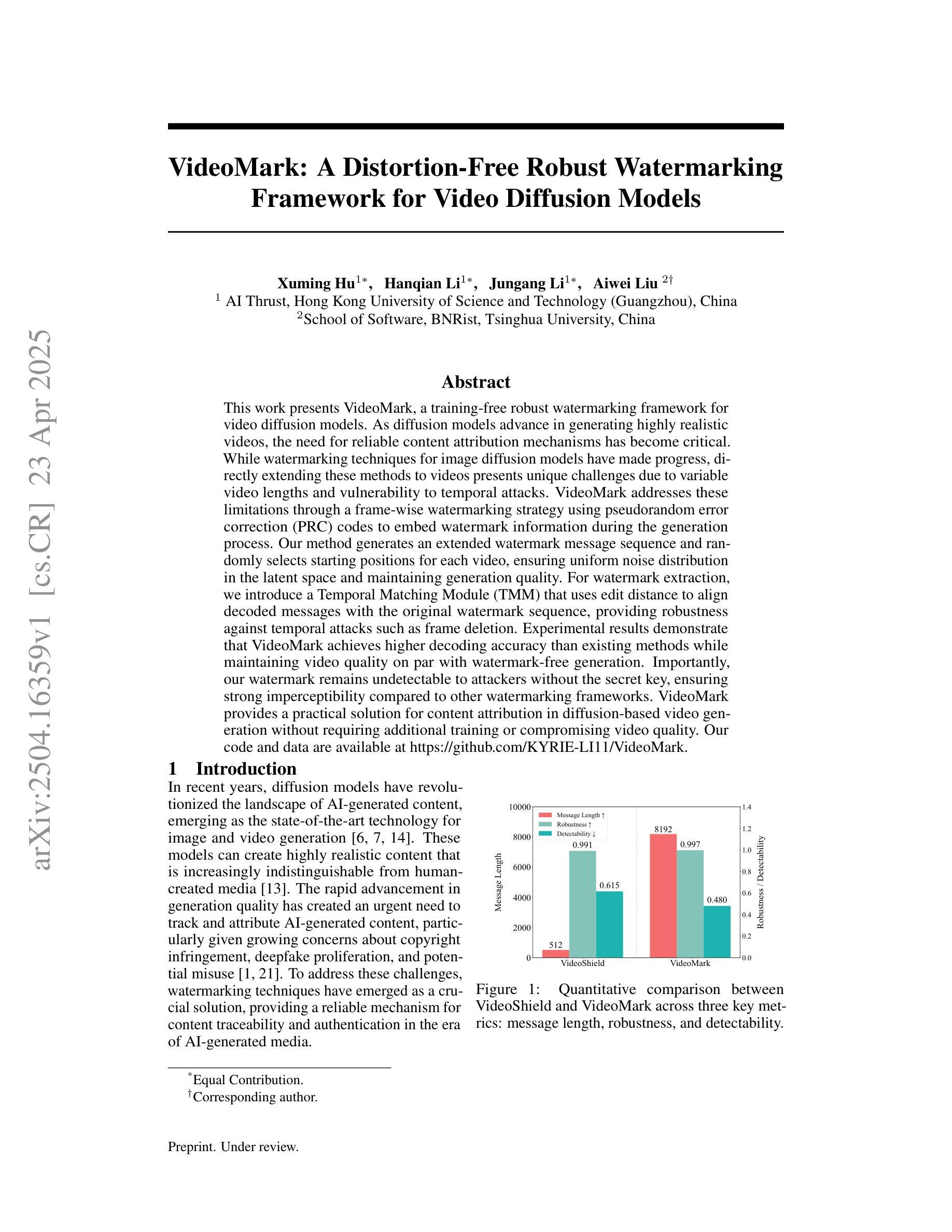
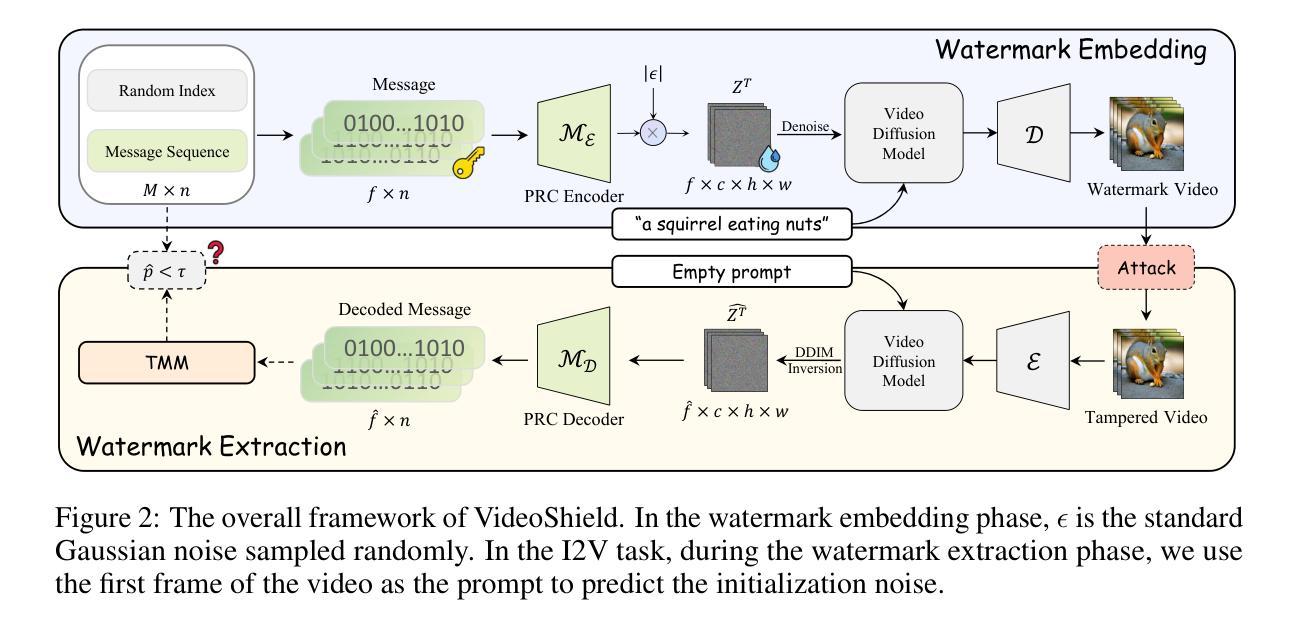
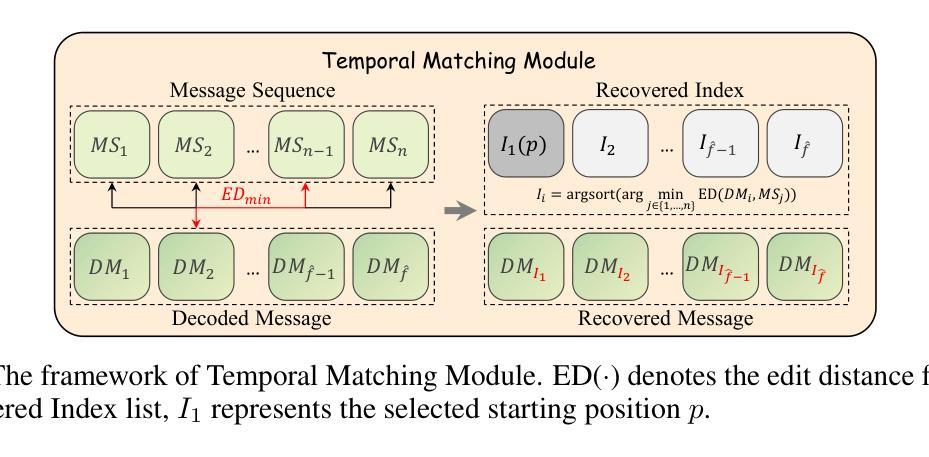
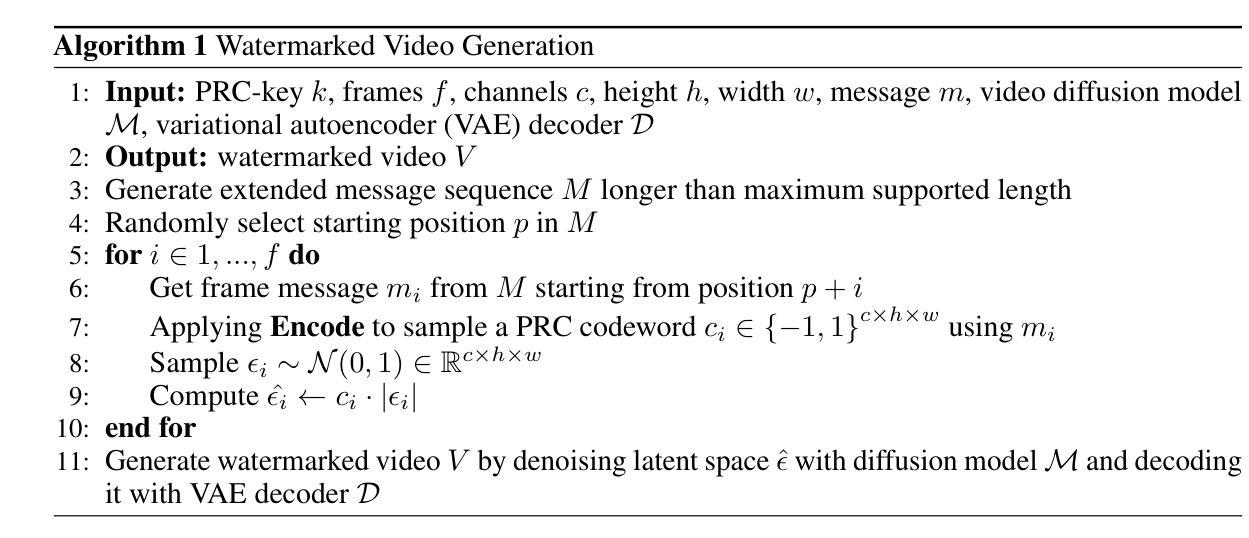
DreamID: High-Fidelity and Fast diffusion-based Face Swapping via Triplet ID Group Learning
Authors:Fulong Ye, Miao Hua, Pengze Zhang, Xinghui Li, Qichao Sun, Songtao Zhao, Qian He, Xinglong Wu
In this paper, we introduce DreamID, a diffusion-based face swapping model that achieves high levels of ID similarity, attribute preservation, image fidelity, and fast inference speed. Unlike the typical face swapping training process, which often relies on implicit supervision and struggles to achieve satisfactory results. DreamID establishes explicit supervision for face swapping by constructing Triplet ID Group data, significantly enhancing identity similarity and attribute preservation. The iterative nature of diffusion models poses challenges for utilizing efficient image-space loss functions, as performing time-consuming multi-step sampling to obtain the generated image during training is impractical. To address this issue, we leverage the accelerated diffusion model SD Turbo, reducing the inference steps to a single iteration, enabling efficient pixel-level end-to-end training with explicit Triplet ID Group supervision. Additionally, we propose an improved diffusion-based model architecture comprising SwapNet, FaceNet, and ID Adapter. This robust architecture fully unlocks the power of the Triplet ID Group explicit supervision. Finally, to further extend our method, we explicitly modify the Triplet ID Group data during training to fine-tune and preserve specific attributes, such as glasses and face shape. Extensive experiments demonstrate that DreamID outperforms state-of-the-art methods in terms of identity similarity, pose and expression preservation, and image fidelity. Overall, DreamID achieves high-quality face swapping results at 512*512 resolution in just 0.6 seconds and performs exceptionally well in challenging scenarios such as complex lighting, large angles, and occlusions.
本文介绍了DreamID,这是一款基于扩散的面貌替换模型,实现了高水平的身份相似性、属性保留、图像保真度和快速推理速度。不同于典型的面貌替换训练过程,通常依赖于隐式监督并难以取得令人满意的结果。DreamID通过构建Triplet ID Group数据实现对面貌替换的显式监督,从而显著提高身份相似性和属性保留。扩散模型的迭代性质给利用高效的图像空间损失函数带来了挑战,因为在训练期间通过耗时多步采样获得生成图像是不切实际的。为了解决这个问题,我们利用了加速扩散模型SD Turbo,将推理步骤减少到单次迭代,能够在显式Triplet ID Group监督下进行高效的像素级端到端训练。此外,我们提出了一种改进的基于扩散的模型架构,包括SwapNet、FaceNet和ID Adapter。这一稳健的架构充分释放了Triplet ID Group显式监督的威力。最后,为了进一步完善我们的方法,我们在训练过程中显式修改了Triplet ID Group数据,以微调并保留特定属性,如眼镜和脸型。大量实验表明,DreamID在身份相似性、姿势和表情保留以及图像保真度方面均优于最先进的方法。总的来说,DreamID在512*512分辨率下实现了高质量的面貌替换结果,仅需0.6秒,且在复杂光照、大角度和遮挡等挑战场景下表现尤为出色。
论文及项目相关链接
PDF Project: https://superhero-7.github.io/DreamID/
Summary
本文介绍了基于扩散模型的DreamID面部换脸技术,具备高身份识别相似度、属性保留、图像保真度和快速推理速度等优点。它采用显式监督方法实现面部换脸,构建Triplet ID Group数据,提高了身份相似度和属性保留效果。借助加速扩散模型SD Turbo,实现了单迭代推理,可进行高效的像素级端到端训练。提出的改进扩散模型架构包括SwapNet、FaceNet和ID Adapter,充分利用了Triplet ID Group的显式监督。此外,通过对训练过程中的Triplet ID Group数据进行显式修改,实现对特定属性的微调保留,如眼镜和脸型。实验表明,DreamID在身份识别相似度、姿势和表情保留以及图像保真度方面均优于现有技术,并在复杂光照、大角度和遮挡等场景下表现优异。
Key Takeaways
- DreamID是一个基于扩散模型的面部换脸技术,具有高身份识别相似度、属性保留和图像保真度等特点。
- 采用显式监督方法实现面部换脸,构建Triplet ID Group数据增强身份相似度和属性保留。
- 利用加速扩散模型SD Turbo实现单迭代推理,支持高效像素级端到端训练。
- 改进扩散模型架构包括SwapNet、FaceNet和ID Adapter,充分利用显式监督的优势。
- 通过修改训练过程中的Triplet ID Group数据,实现对特定属性的微调保留。
- DreamID在多项实验中表现出优异的性能,尤其是在复杂场景下的面部换脸任务。
点此查看论文截图
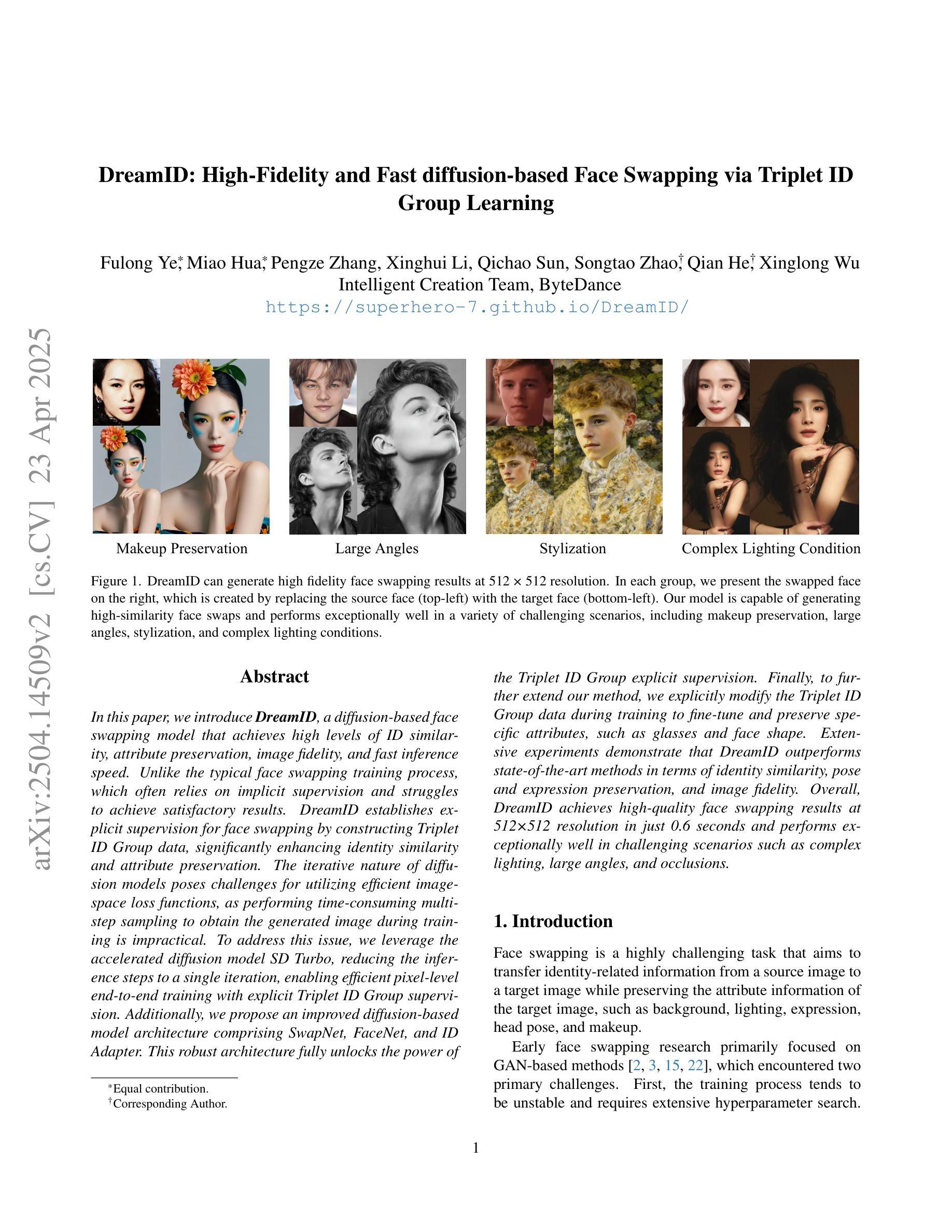
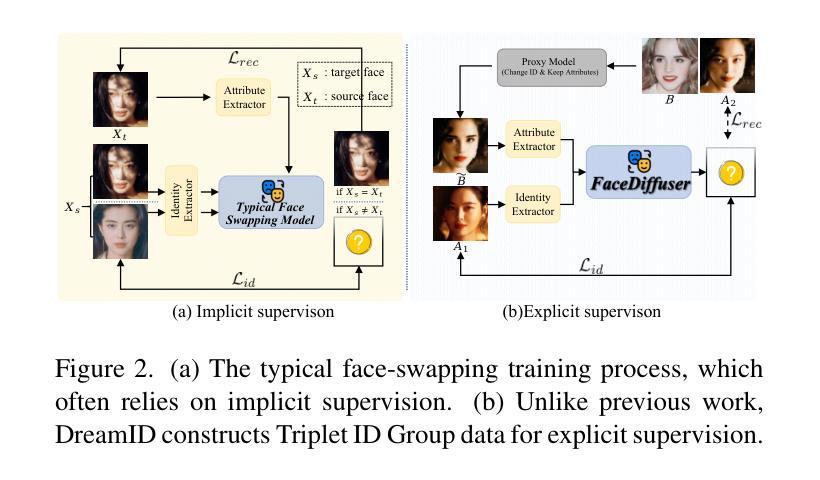
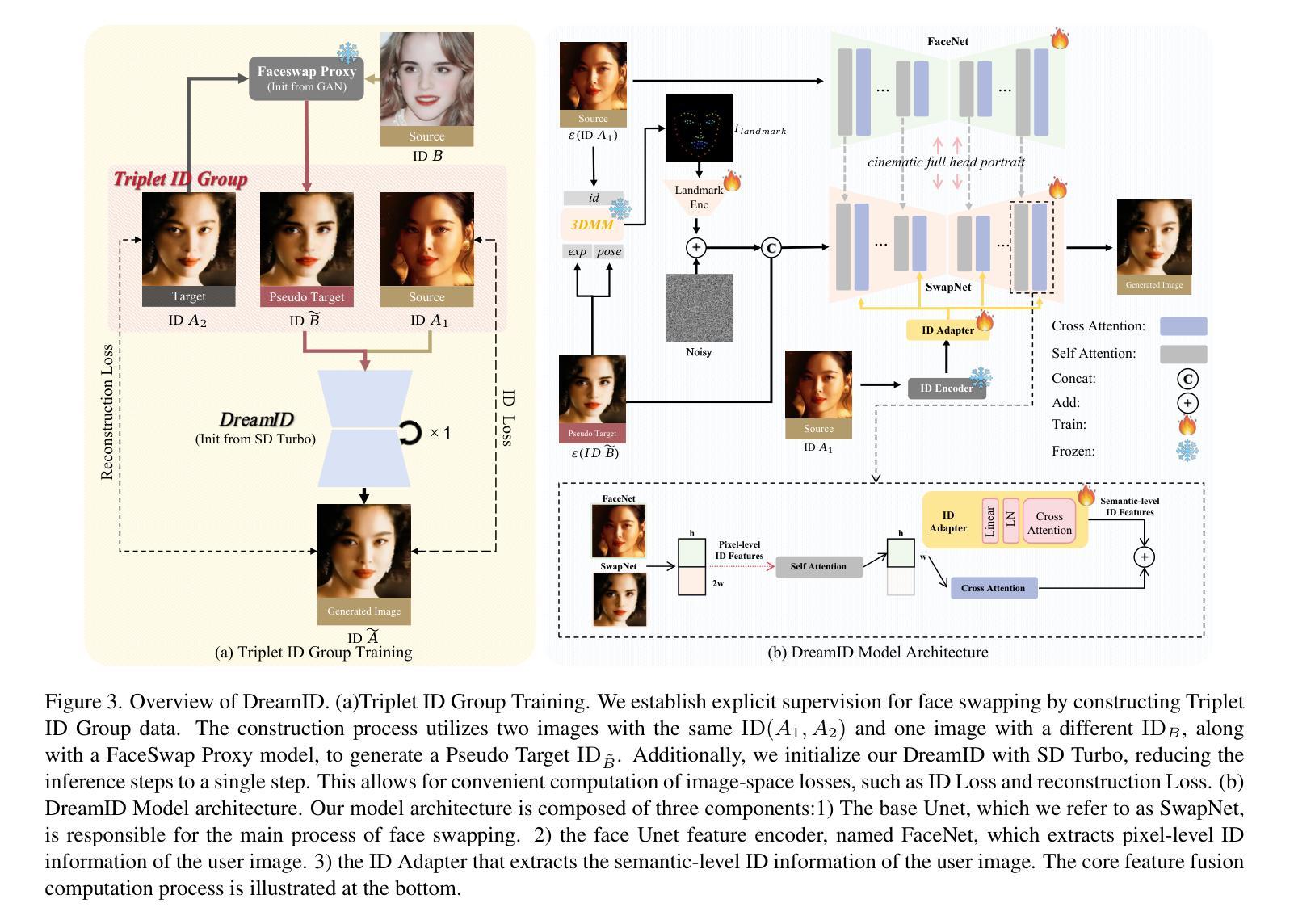
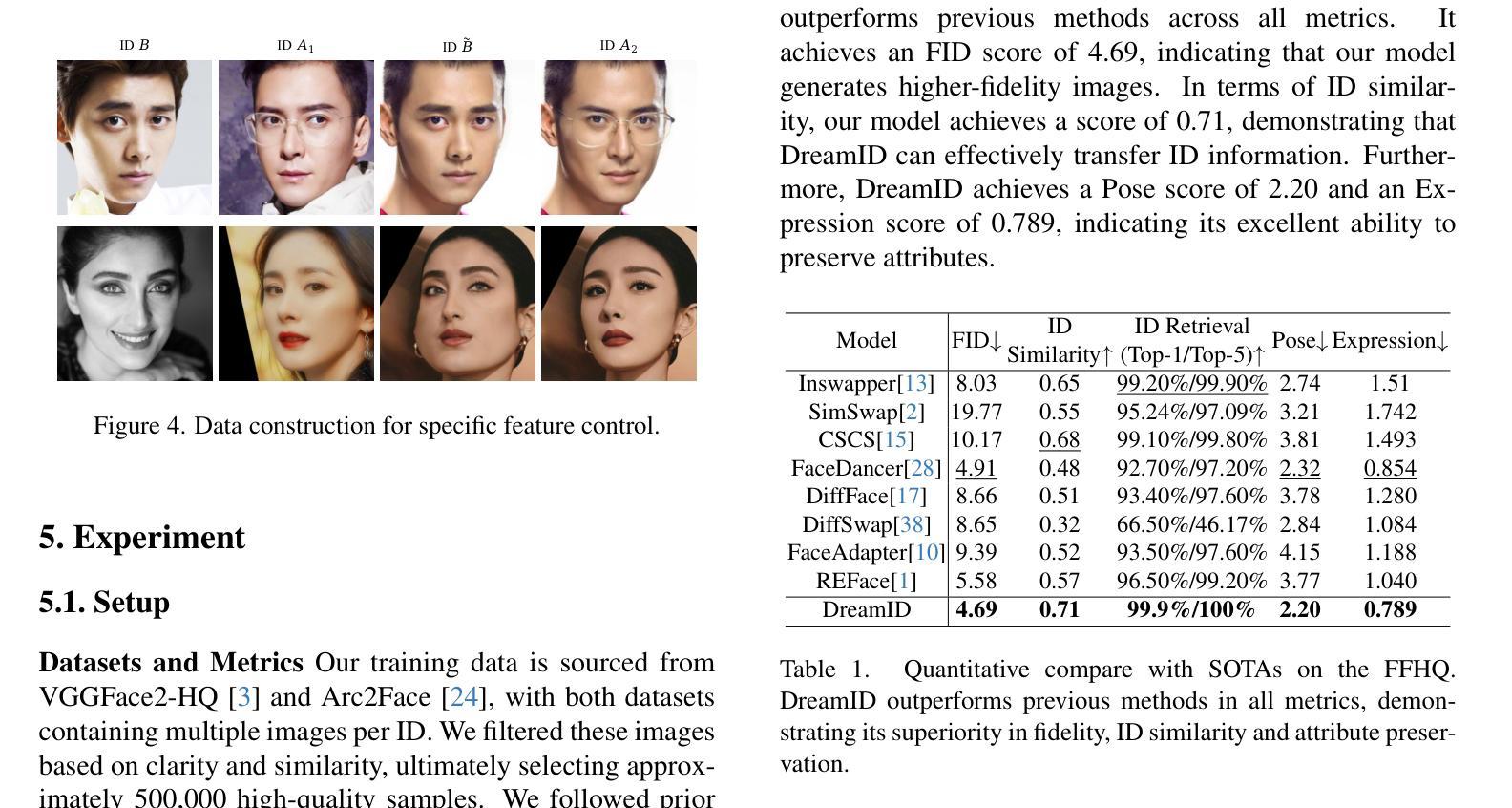
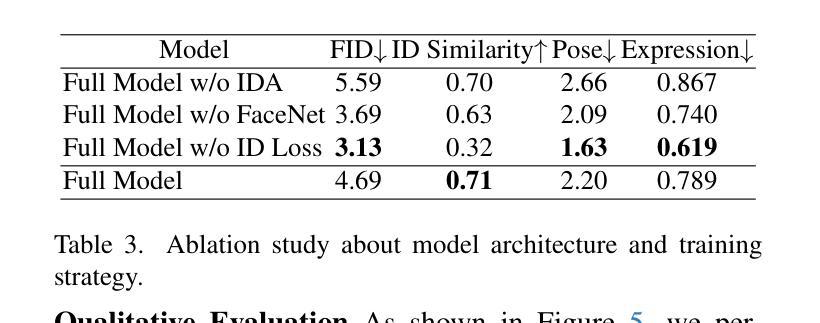
Decoding Vision Transformers: the Diffusion Steering Lens
Authors:Ryota Takatsuki, Sonia Joseph, Ippei Fujisawa, Ryota Kanai
Logit Lens is a widely adopted method for mechanistic interpretability of transformer-based language models, enabling the analysis of how internal representations evolve across layers by projecting them into the output vocabulary space. Although applying Logit Lens to Vision Transformers (ViTs) is technically straightforward, its direct use faces limitations in capturing the richness of visual representations. Building on the work of Toker et al. (2024)~\cite{Toker2024-ve}, who introduced Diffusion Lens to visualize intermediate representations in the text encoders of text-to-image diffusion models, we demonstrate that while Diffusion Lens can effectively visualize residual stream representations in image encoders, it fails to capture the direct contributions of individual submodules. To overcome this limitation, we propose \textbf{Diffusion Steering Lens} (DSL), a novel, training-free approach that steers submodule outputs and patches subsequent indirect contributions. We validate our method through interventional studies, showing that DSL provides an intuitive and reliable interpretation of the internal processing in ViTs.
Logit Lens是广泛应用于基于转换器的语言模型的机械解释性的方法,它通过投影到输出词汇空间来分析内部表示如何在各层中演变。虽然将Logit Lens应用于视觉转换器(ViTs)在技术上很直接,但其直接使用在捕捉视觉表示的丰富性方面存在局限性。基于Toker等人(2024)的工作,他们引入了Diffusion Lens来可视化文本到图像扩散模型的文本编码器的中间表示,我们证明虽然Diffusion Lens可以有效地可视化图像编码器的剩余流表示,但它无法捕捉单个子模块的直接贡献。为了克服这一局限性,我们提出了Diffusion Steering Lens(DSL),这是一种新型的无训练方法,用于引导子模块输出并修补随后的间接贡献。我们通过干预研究验证了我们的方法,表明DSL为ViTs的内部处理提供了直观和可靠的解释。
论文及项目相关链接
PDF 12 pages, 17 figures. Accepted to the CVPR 2025 Workshop on Mechanistic Interpretability for Vision (MIV)
Summary
本文介绍了Logit Lens方法在基于转换器的语言模型中的广泛应用,它通过投影到输出词汇空间来分析内部表示如何在各层中演变。尽管将Logit Lens应用于视觉转换器(ViTs)在技术上很直观,但其直接使用在捕捉视觉表示的丰富性方面存在局限性。基于Toker等人(2024)的工作,本文引入了Diffusion Lens来可视化文本到图像扩散模型的文本编码器的中间表示。为了解决Diffusion Lens无法捕捉图像编码器中单个子模块的直接贡献的问题,本文提出了训练无关的**Diffusion Steering Lens (DSL)**方法,该方法可以引导子模块输出并修复随后的间接贡献。通过干预性研究验证了DSL方法能够直观可靠地解释ViTs的内部处理过程。
Key Takeaways
- Logit Lens广泛用于解释基于转换器的语言模型的内部机制,通过投影到输出词汇空间分析内部表示的演变。
- 虽然Logit Lens在视觉转换器(ViTs)中的应用是技术上的直观,但在捕捉视觉表示的丰富性方面存在局限性。
- Diffusion Lens能可视化文本到图像扩散模型的文本编码器的中间表示,但在捕捉图像编码器中子模块的直接贡献方面存在不足。
- 提出了训练无关的Diffusion Steering Lens (DSL)方法,旨在解决Diffusion Lens的局限性。
- DSL方法可以引导子模块输出并修复随后的间接贡献,提供更直观和可靠的内部处理解释。
- 通过干预性研究验证了DSL在ViTs内部处理过程中的解释能力。
点此查看论文截图
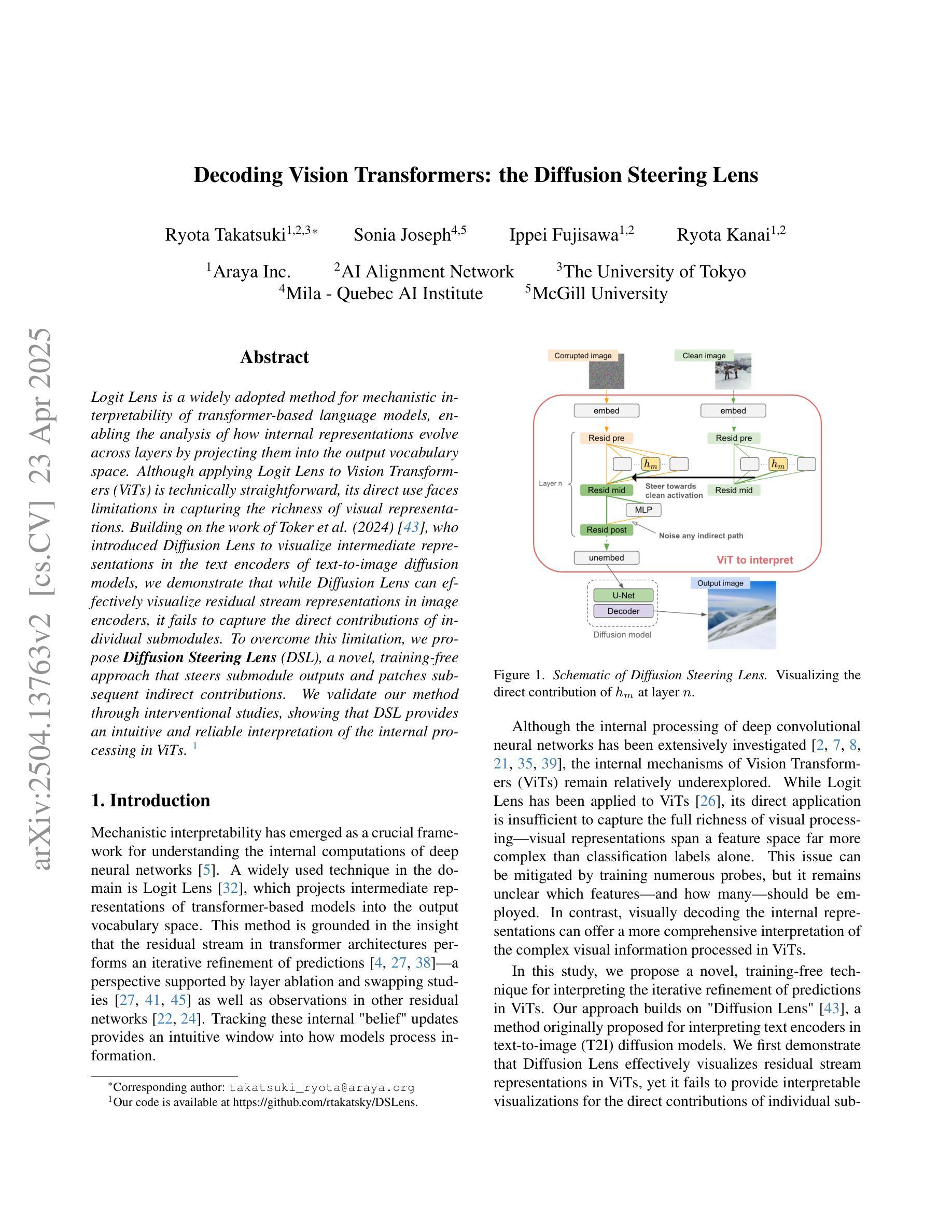
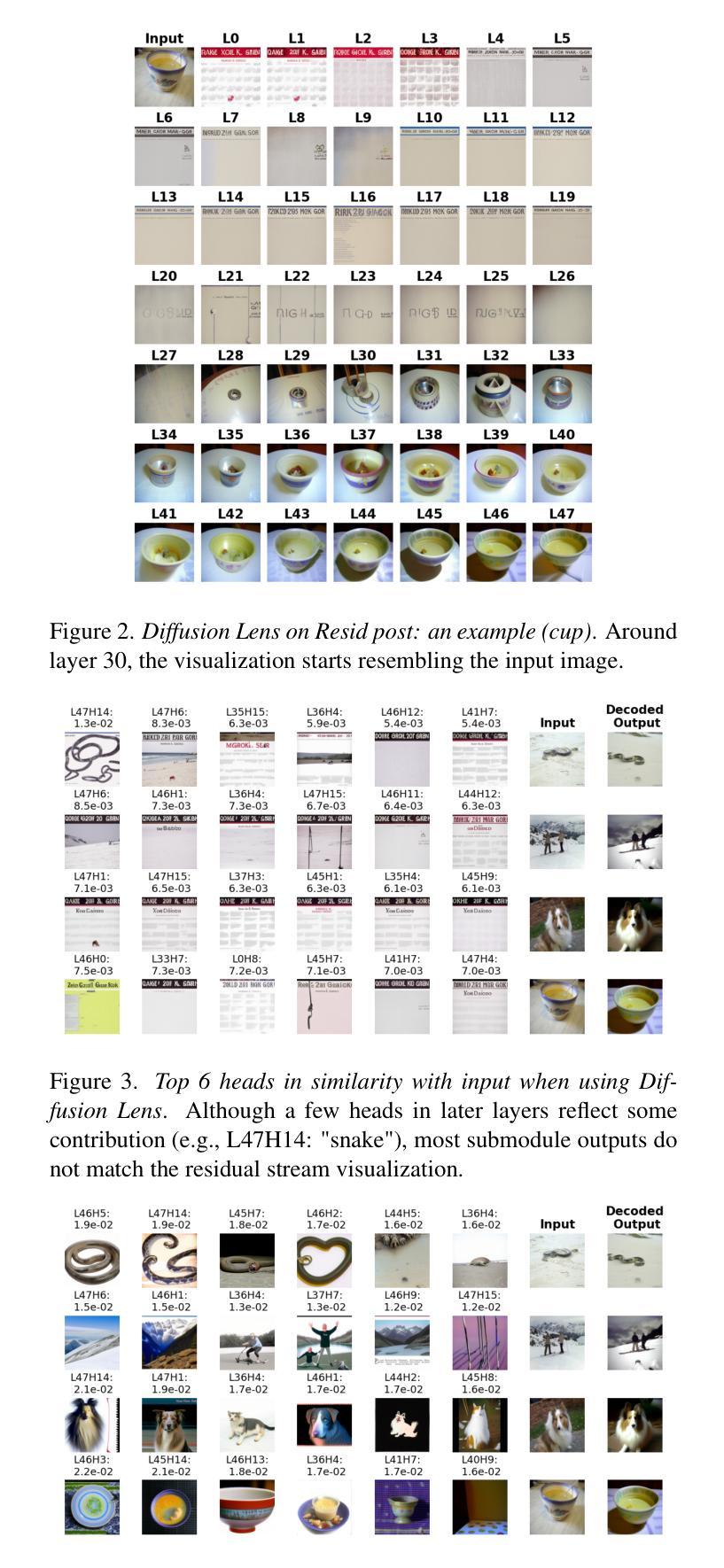
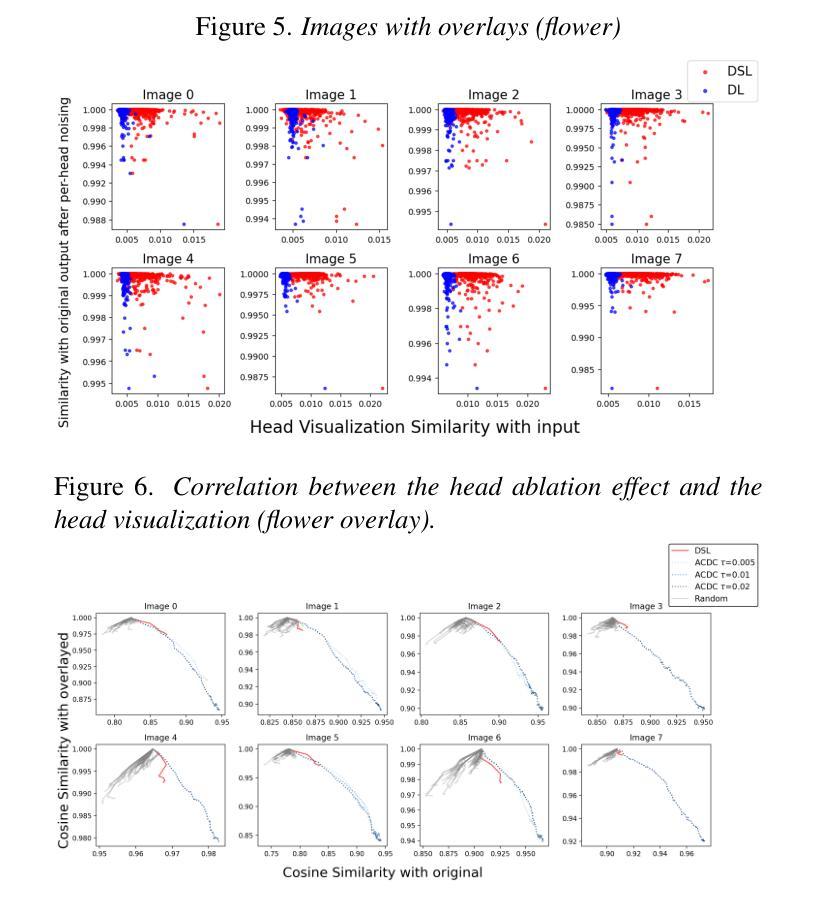
Anti-Aesthetics: Protecting Facial Privacy against Customized Text-to-Image Synthesis
Authors:Songping Wang, Yueming Lyu, Shiqi Liu, Ning Li, Tong Tong, Hao Sun, Caifeng Shan
The rise of customized diffusion models has spurred a boom in personalized visual content creation, but also poses risks of malicious misuse, severely threatening personal privacy and copyright protection. Some studies show that the aesthetic properties of images are highly positively correlated with human perception of image quality. Inspired by this, we approach the problem from a novel and intriguing aesthetic perspective to degrade the generation quality of maliciously customized models, thereby achieving better protection of facial identity. Specifically, we propose a Hierarchical Anti-Aesthetic (HAA) framework to fully explore aesthetic cues, which consists of two key branches: 1) Global Anti-Aesthetics: By establishing a global anti-aesthetic reward mechanism and a global anti-aesthetic loss, it can degrade the overall aesthetics of the generated content; 2) Local Anti-Aesthetics: A local anti-aesthetic reward mechanism and a local anti-aesthetic loss are designed to guide adversarial perturbations to disrupt local facial identity. By seamlessly integrating both branches, our HAA effectively achieves the goal of anti-aesthetics from a global to a local level during customized generation. Extensive experiments show that HAA outperforms existing SOTA methods largely in identity removal, providing a powerful tool for protecting facial privacy and copyright.
定制扩散模型的兴起促进了个性化视觉内容创作的繁荣,但同时也带来了恶意滥用的风险,严重威胁个人隐私和版权保护。一些研究表明,图像的美学属性与人们对图像质量的感知高度正相关。受此启发,我们从一个全新且有趣的美学角度来解决这一问题,以降低恶意定制模型的生成质量,从而实现更好的面部身份保护。具体来说,我们提出了分层反美学(HAA)框架,以充分探索美学线索,该框架包含两个关键分支:1)全局反美学:通过建立全局反美学奖励机制和全局反美学损失,它可以降低生成内容的整体美观度;2)局部反美学:设计局部反美学奖励机制和局部反美学损失,以引导对抗性扰动破坏局部面部身份。通过无缝集成这两个分支,我们的HAA有效地实现了从全局到局部的反美学目标。大量实验表明,在身份去除方面,HAA大大优于现有最先进的方法,为保护面部隐私和版权提供了有力的工具。
论文及项目相关链接
PDF After the submission of the paper, we realized that the study still has room for expansion. In order to make the research findings more profound and comprehensive, we have decided to withdraw the paper so that we can conduct further research and expansion
Summary
本文提出一种基于美学视角的个性化扩散模型保护策略。通过构建全局和局部反美学奖励机制和损失函数,实现对生成内容的整体和局部美学的削弱,以规避恶意定制化模型的风险,加强个人隐私与版权保护。实验证明,该方法在身份去除方面大幅超越现有最先进方法,为面部隐私和版权保护提供有力工具。
Key Takeaways
- 定制扩散模型的出现促进了个性化视觉内容的创建,但也带来了恶意滥用的风险,威胁个人隐私和版权保护。
- 美学属性与人类对图像质量的感知有高度正相关,因此从美学角度来解决恶意定制模型的问题是一种新颖且有效的方法。
- 提出了一个分层的反美学(HAA)框架,该框架包括两个关键分支:全局反美学和局部反美学。
- 全局反美学通过建立全局反美学奖励机制和损失来降低生成内容的整体美学。
- 局部反美学设计局部反美学奖励机制和损失,以引导对抗性扰动破坏局部面部身份。
- HAA框架将两者无缝结合,实现从全局到局部的抗美学目标。
点此查看论文截图
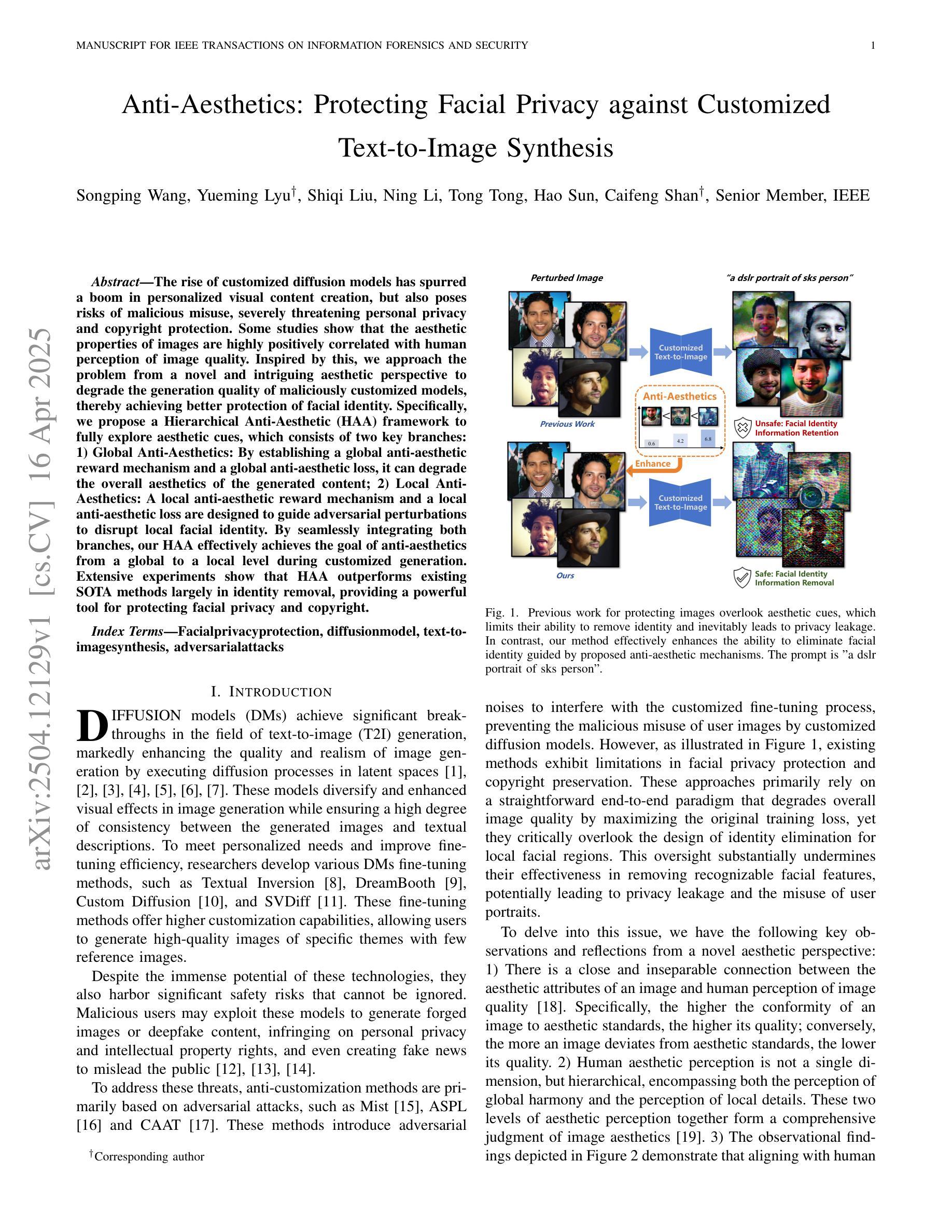
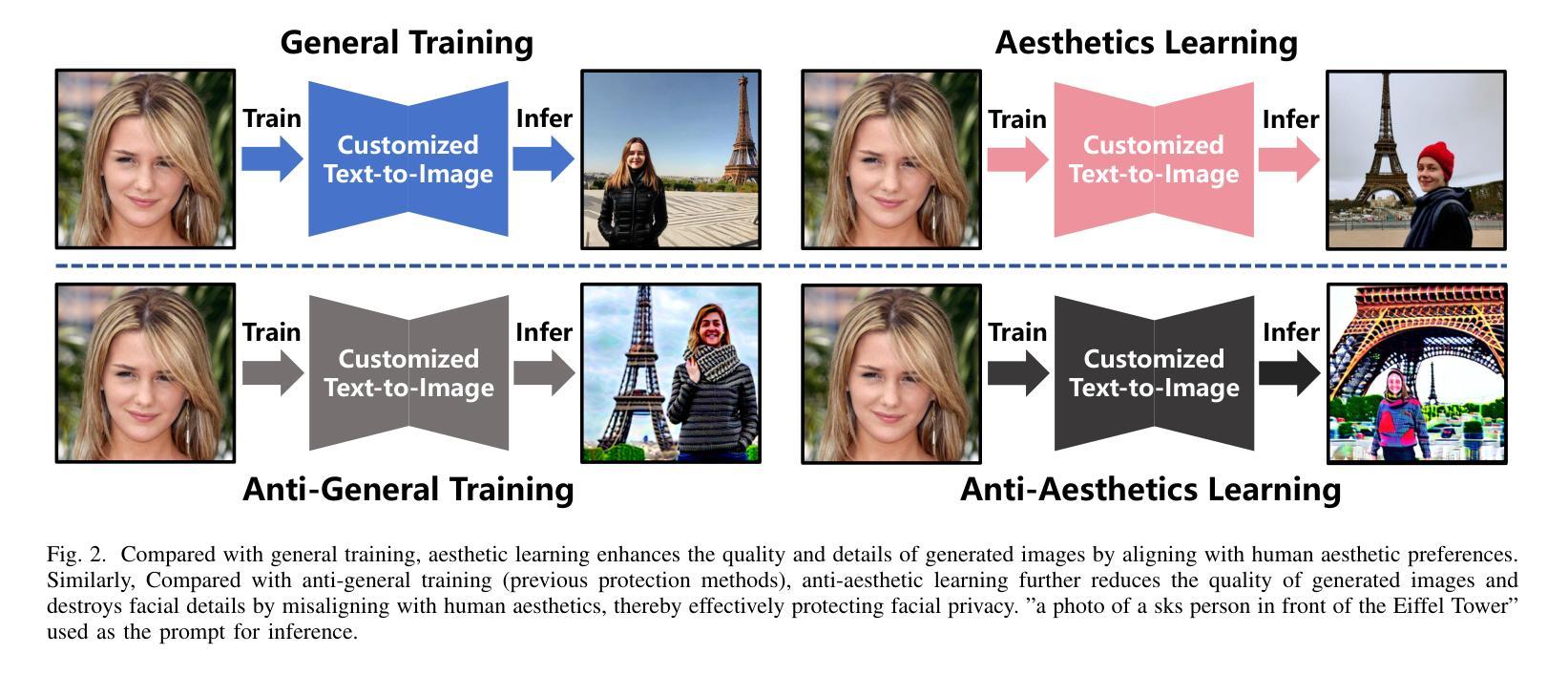
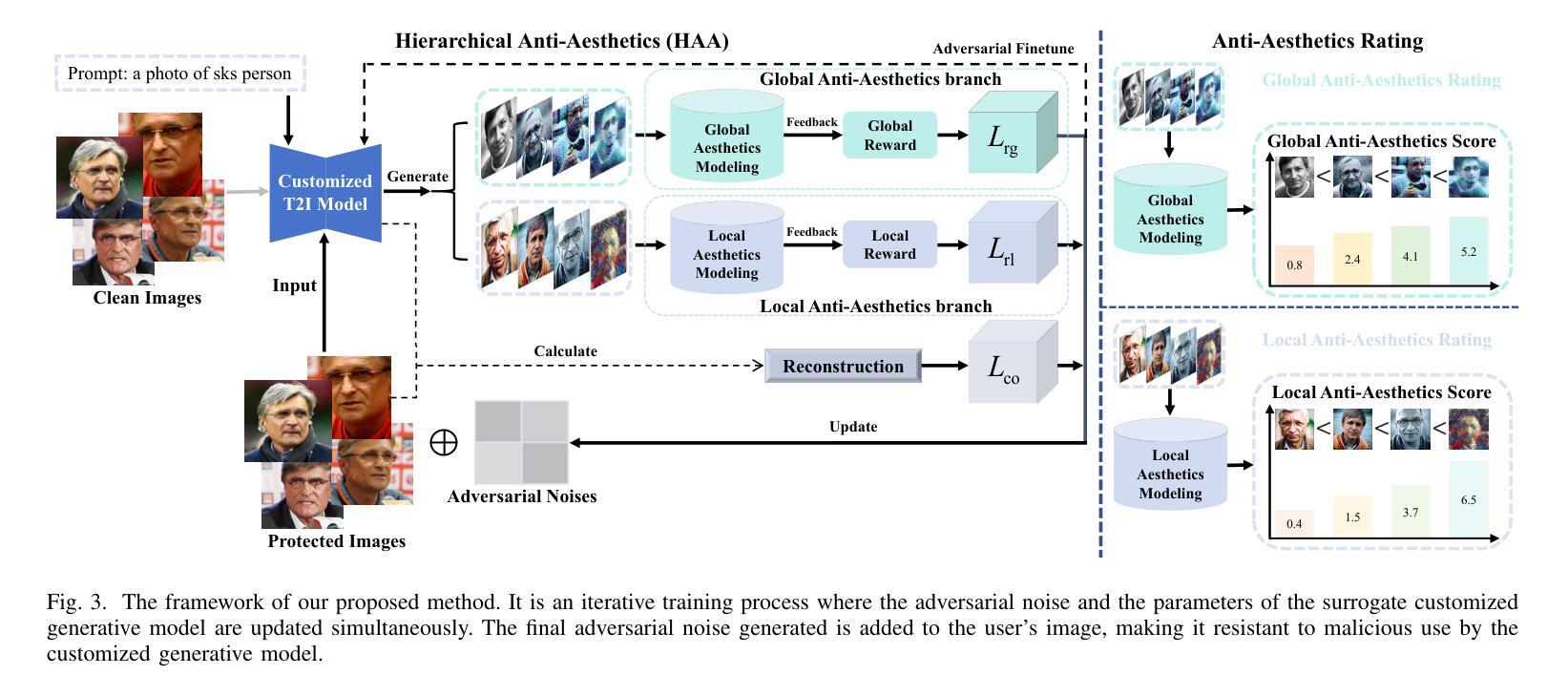
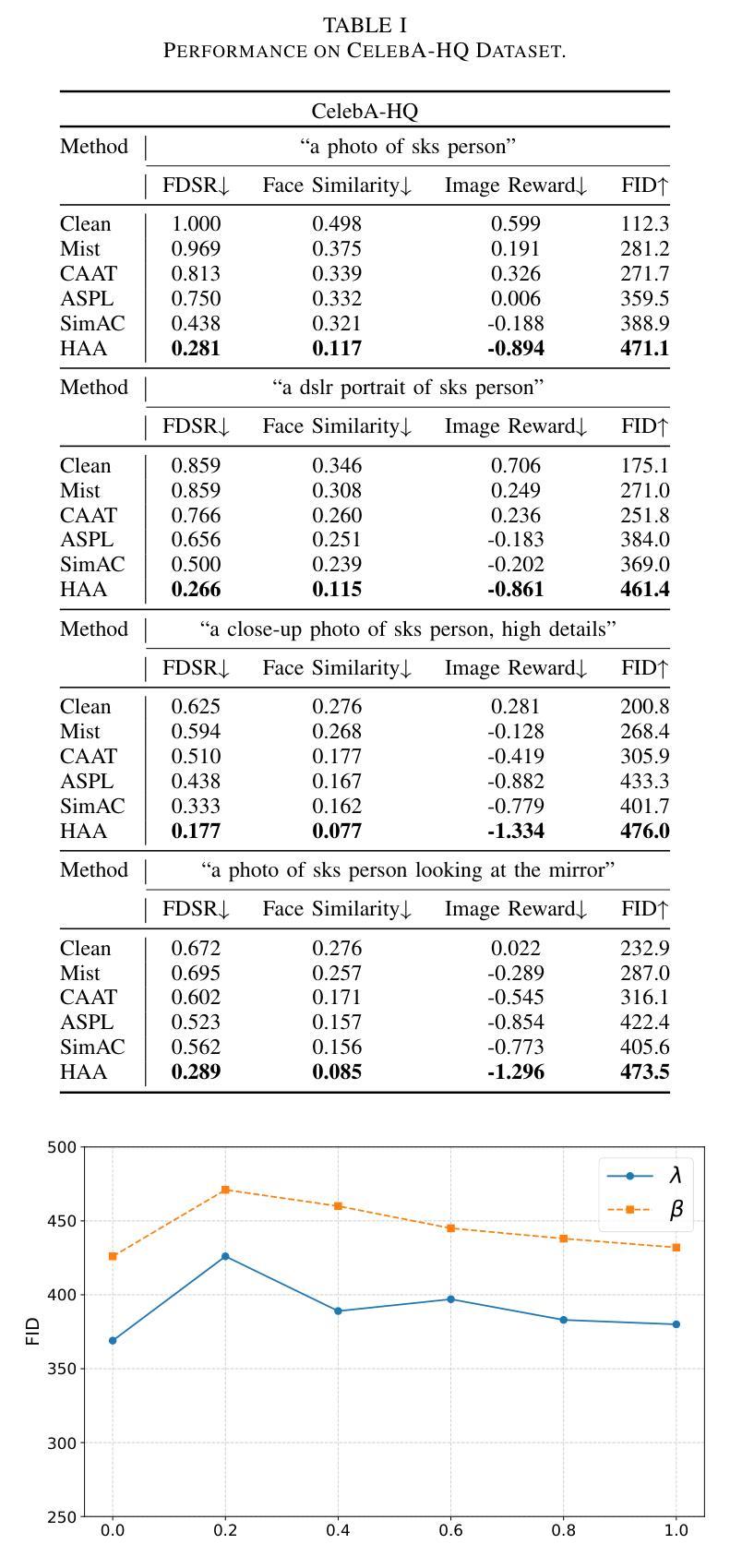
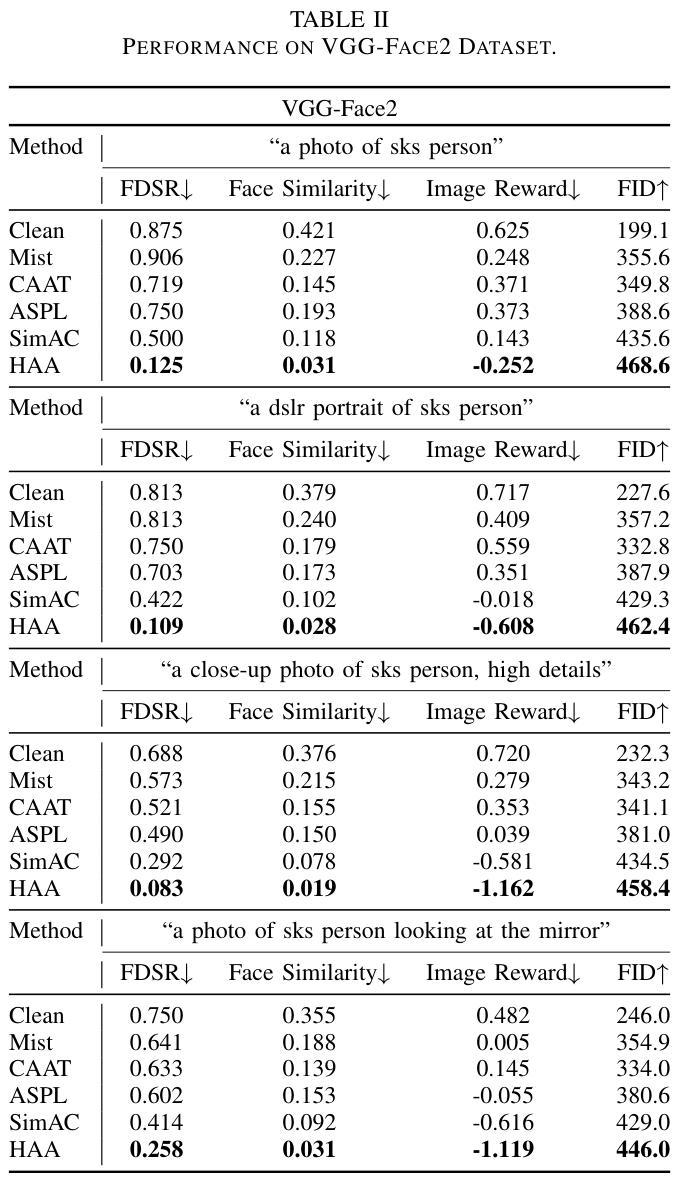
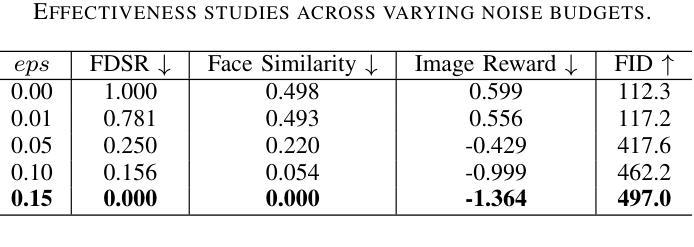
OSDFace: One-Step Diffusion Model for Face Restoration
Authors:Jingkai Wang, Jue Gong, Lin Zhang, Zheng Chen, Xing Liu, Hong Gu, Yutong Liu, Yulun Zhang, Xiaokang Yang
Diffusion models have demonstrated impressive performance in face restoration. Yet, their multi-step inference process remains computationally intensive, limiting their applicability in real-world scenarios. Moreover, existing methods often struggle to generate face images that are harmonious, realistic, and consistent with the subject’s identity. In this work, we propose OSDFace, a novel one-step diffusion model for face restoration. Specifically, we propose a visual representation embedder (VRE) to better capture prior information and understand the input face. In VRE, low-quality faces are processed by a visual tokenizer and subsequently embedded with a vector-quantized dictionary to generate visual prompts. Additionally, we incorporate a facial identity loss derived from face recognition to further ensure identity consistency. We further employ a generative adversarial network (GAN) as a guidance model to encourage distribution alignment between the restored face and the ground truth. Experimental results demonstrate that OSDFace surpasses current state-of-the-art (SOTA) methods in both visual quality and quantitative metrics, generating high-fidelity, natural face images with high identity consistency. The code and model will be released at https://github.com/jkwang28/OSDFace.
扩散模型在人脸修复方面表现出了令人印象深刻的性能。然而,其多步推理过程仍然计算密集,限制了其在现实场景中的应用。此外,现有方法往往难以生成和谐、逼真且与人脸身份一致的人脸图像。在这项工作中,我们提出了OSDFace,这是一种用于人脸修复的新型一步扩散模型。具体来说,我们提出了一种视觉表示嵌入器(VRE)来更好地捕获先验信息并理解输入的人脸。在VRE中,低质量的人脸通过视觉标记器进行处理,然后通过向量量化字典嵌入以生成视觉提示。此外,我们结合人脸识别中得出的面部身份损失来进一步确保身份一致性。我们还采用生成对抗网络(GAN)作为指导模型,以鼓励恢复的人脸与真实数据之间的分布对齐。实验结果表明,OSDFace在视觉质量和定量指标上均超过了当前先进技术(SOTA),生成了高保真、自然的人脸图像,具有高度的身份一致性。代码和模型将在https://github.com/jkwang28/OSDFace上发布。
论文及项目相关链接
PDF Accepted to CVPR 2025. The code and model will be available at https://github.com/jkwang28/OSDFace
摘要
扩散模型在面部修复方面展现出令人印象深刻的性能,但其多步推理过程计算量大,限制了其在现实场景中的应用。本文提出OSDFace,一种用于面部修复的新型一步扩散模型。通过视觉表示嵌入器(VRE)更好地捕获先验信息并理解输入面部。借助视觉标记器和向量量化字典生成视觉提示。还结合面部识别衍生的面部身份损失,以确保身份一致性。采用生成对抗网络(GAN)作为指导模型,使修复后的面部与真实面部的分布对齐。OSDFace在视觉质量和定量指标上均超越了当前最先进的方法,能生成高保真、自然的面部图像,具有很高的身份一致性。
关键见解
- 扩散模型在面部修复中表现出卓越性能,但存在计算量大和生成面部图像和谐性、真实性以及身份一致性方面的挑战。
- 提出OSDFace,一种新型一步扩散模型,用于面部修复。
- 引入视觉表示嵌入器(VRE)以捕获先验信息和理解输入面部。
- 使用视觉标记器和向量量化字典生成视觉提示。
- 结合面部识别技术,通过面部身份损失确保身份一致性。
- 采用生成对抗网络(GAN)作为指导模型,实现修复面部与真实面部的分布对齐。
- OSDFace在视觉质量和定量指标上超越当前最先进方法,能生成高保真、自然且具有高度身份一致性的面部图像。
点此查看论文截图
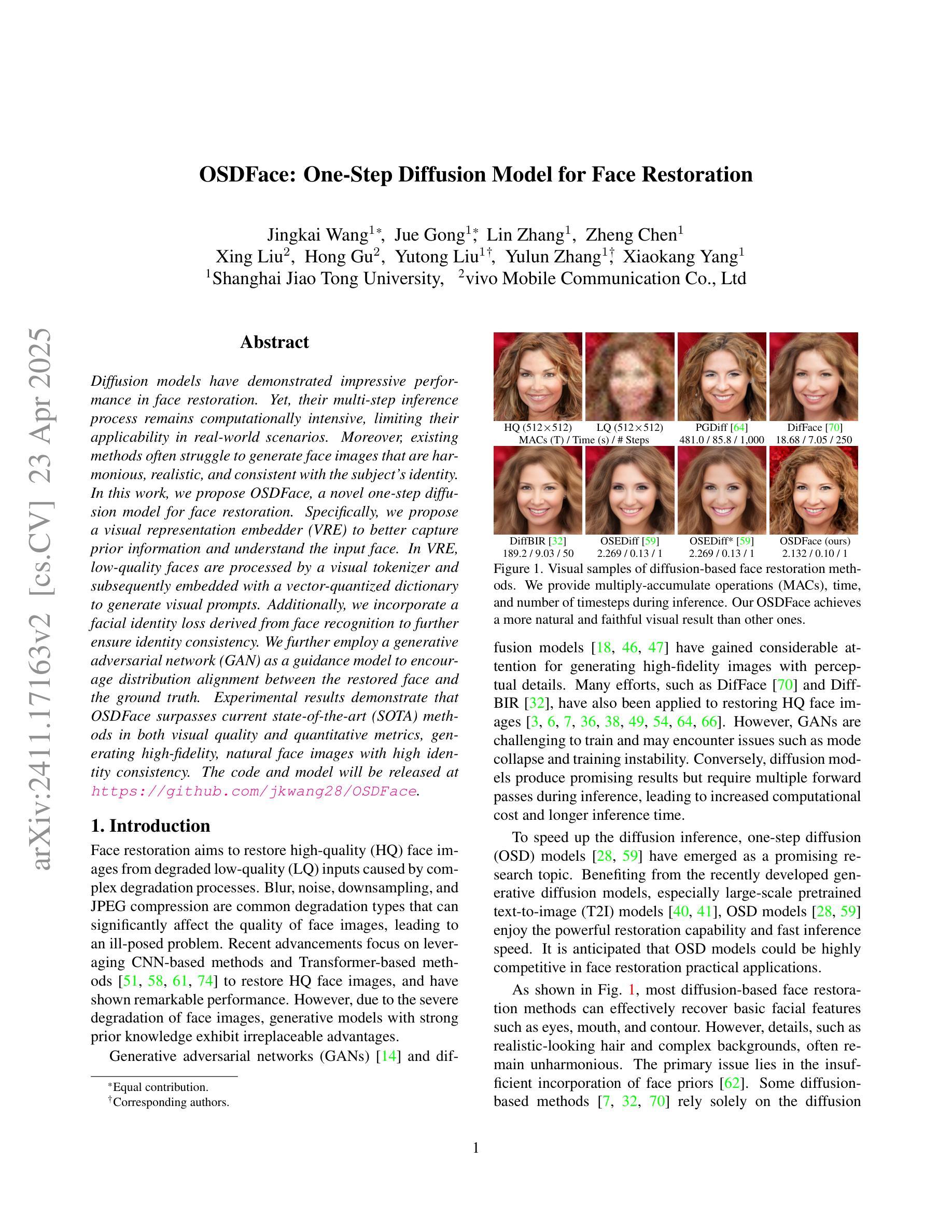
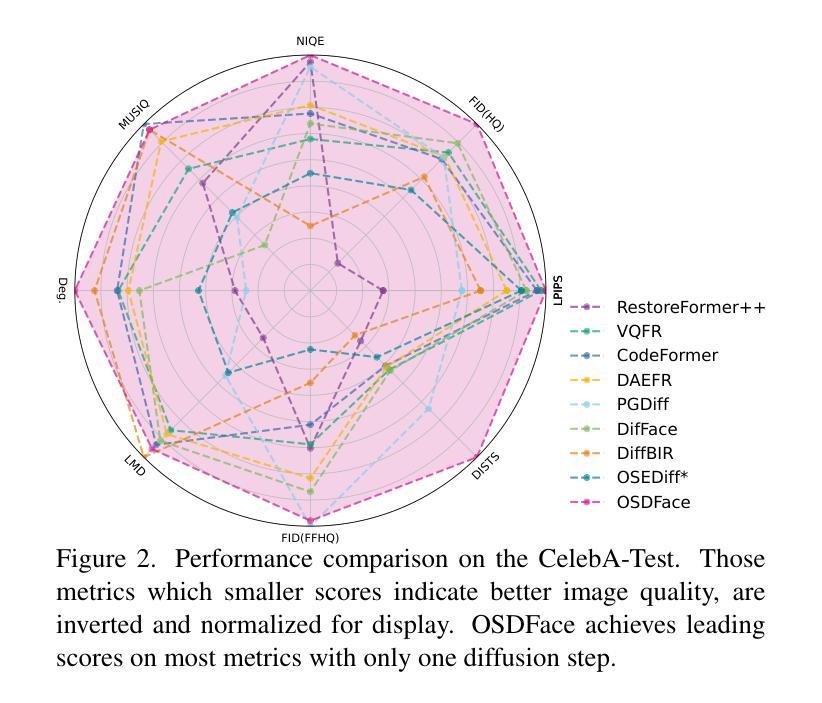
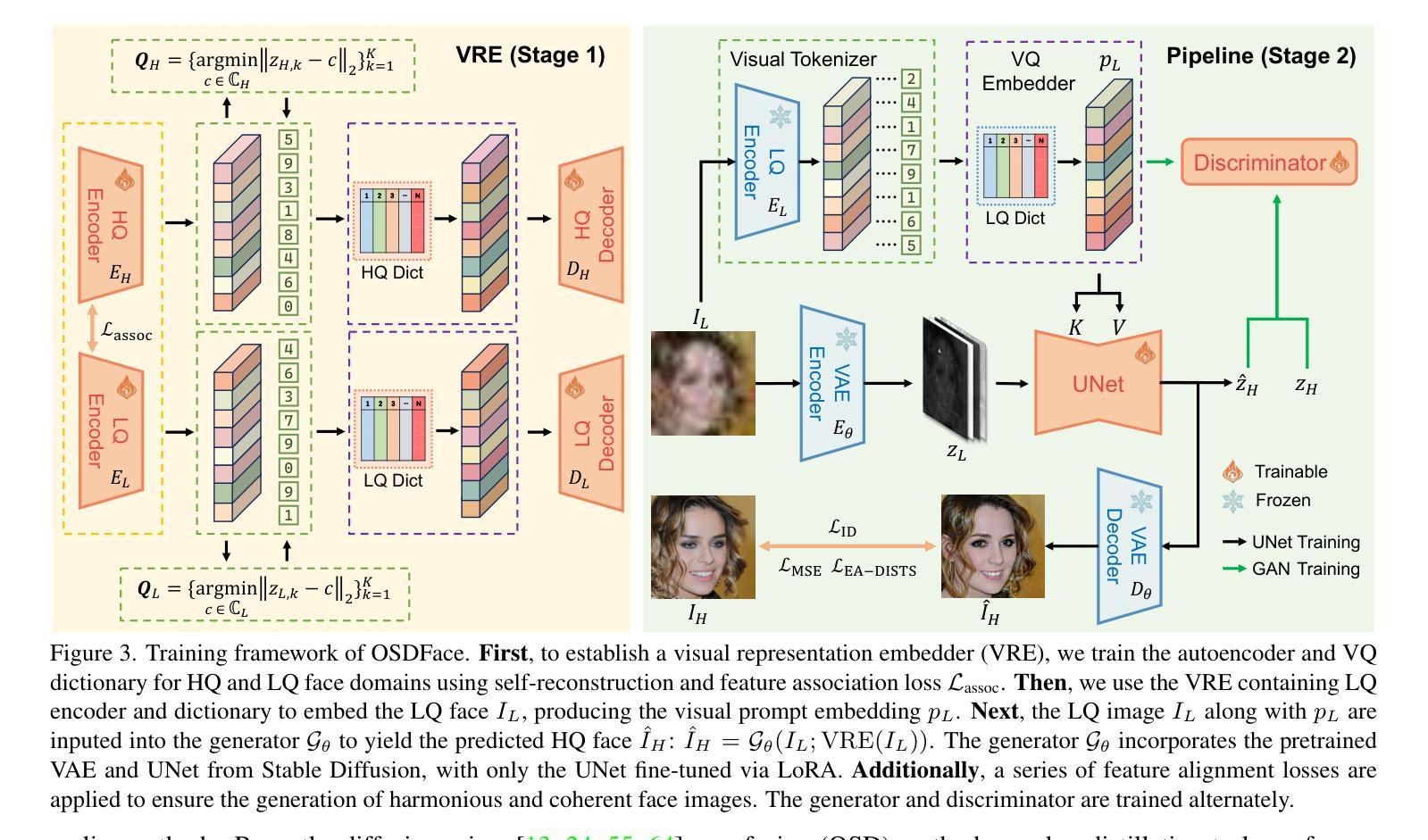
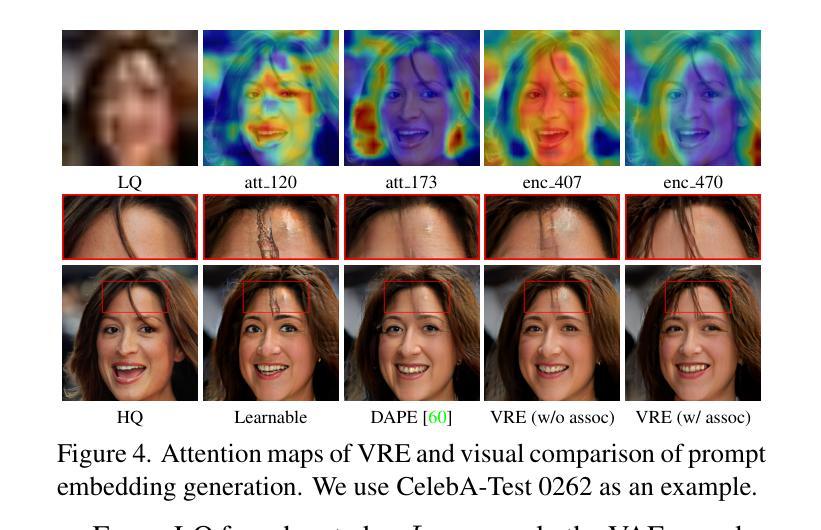
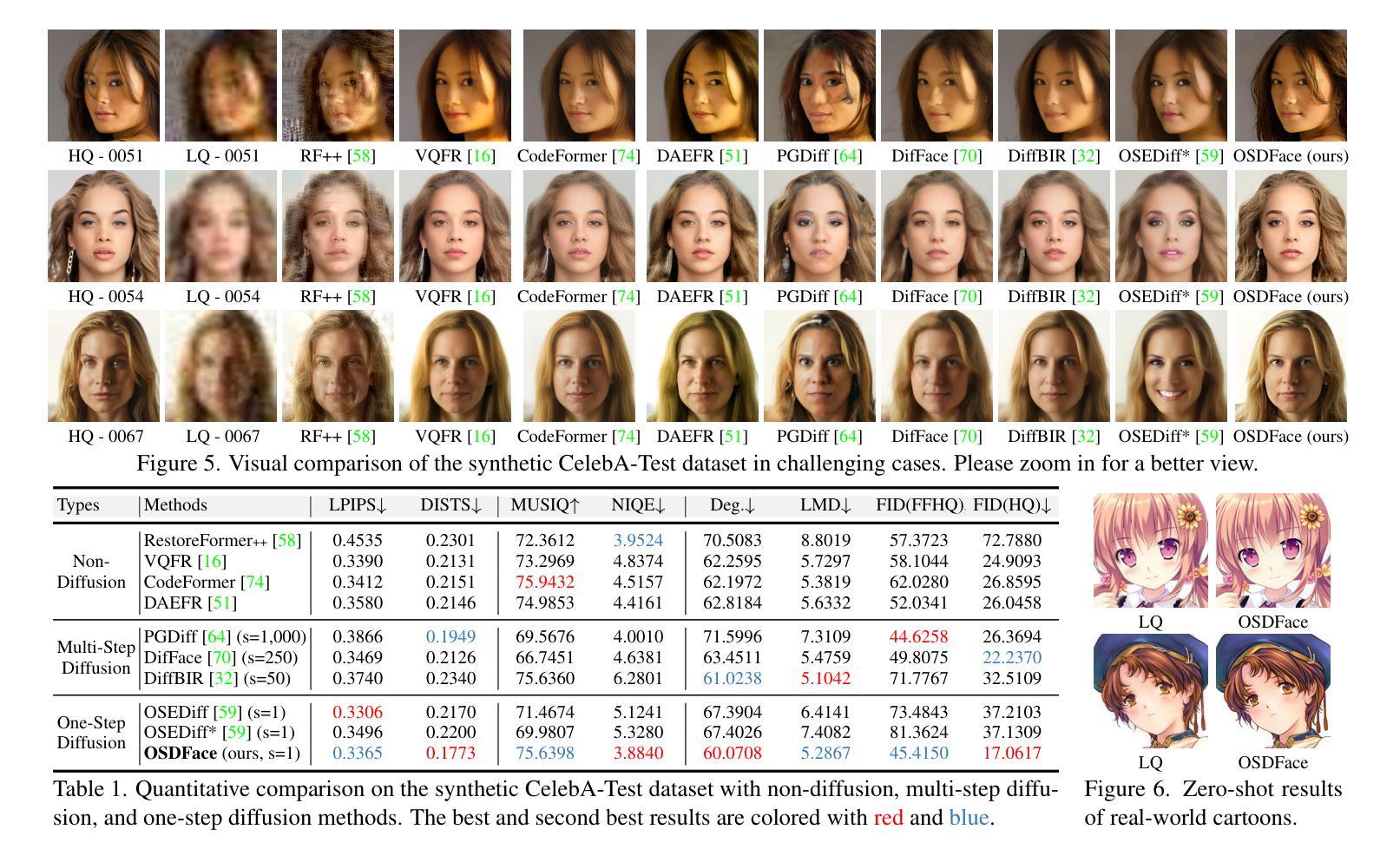