⚠️ 以下所有内容总结都来自于 大语言模型的能力,如有错误,仅供参考,谨慎使用
🔴 请注意:千万不要用于严肃的学术场景,只能用于论文阅读前的初筛!
💗 如果您觉得我们的项目对您有帮助 ChatPaperFree ,还请您给我们一些鼓励!⭐️ HuggingFace免费体验
2025-04-25 更新
Pix2Next: Leveraging Vision Foundation Models for RGB to NIR Image Translation
Authors:Youngwan Jin, Incheol Park, Hanbin Song, Hyeongjin Ju, Yagiz Nalcakan, Shiho Kim
This paper proposes Pix2Next, a novel image-to-image translation framework designed to address the challenge of generating high-quality Near-Infrared (NIR) images from RGB inputs. Our approach leverages a state-of-the-art Vision Foundation Model (VFM) within an encoder-decoder architecture, incorporating cross-attention mechanisms to enhance feature integration. This design captures detailed global representations and preserves essential spectral characteristics, treating RGB-to-NIR translation as more than a simple domain transfer problem. A multi-scale PatchGAN discriminator ensures realistic image generation at various detail levels, while carefully designed loss functions couple global context understanding with local feature preservation. We performed experiments on the RANUS dataset to demonstrate Pix2Next’s advantages in quantitative metrics and visual quality, improving the FID score by 34.81% compared to existing methods. Furthermore, we demonstrate the practical utility of Pix2Next by showing improved performance on a downstream object detection task using generated NIR data to augment limited real NIR datasets. The proposed approach enables the scaling up of NIR datasets without additional data acquisition or annotation efforts, potentially accelerating advancements in NIR-based computer vision applications.
本文提出了Pix2Next这一新型图像到图像的翻译框架,旨在解决从RGB输入生成高质量近红外(NIR)图像的挑战。我们的方法采用最先进的视觉基础模型(VFM)在编码器-解码器架构中,融入交叉注意力机制以增强特征融合。这种设计捕捉详细的全球代表性特征并保留关键光谱特性,将RGB到NIR的翻译视为不仅仅是简单的域转移问题。多尺度PatchGAN鉴别器确保在各种细节级别上生成逼真的图像,而精心设计的损失函数将全局上下文理解与局部特征保留相结合。我们在RANUS数据集上进行了实验,以展示Pix2Next在定量指标和视觉质量方面的优势,与现有方法相比,FID得分提高了34.81%。此外,我们通过下游对象检测任务展示了Pix2Next的实际效用,使用生成的NIR数据增强有限的真实NIR数据集。所提出的方法能够在不获取或标注额外数据的情况下扩大NIR数据集,可能加速基于NIR的计算机视觉应用的进步。
论文及项目相关链接
PDF 19 pages,12 figures
Summary
Pix2Next是一个用于生成高质量近红外图像的新型图像到图像翻译框架。它采用先进的视觉基础模型,结合编码器-解码器架构和跨注意力机制,旨在捕捉全局详细表示并保留关键光谱特征。通过多尺度PatchGAN鉴别器和精心设计的损失函数,确保在不同细节级别生成逼真的图像。在RANUS数据集上的实验证明了Pix2Next在定量指标和视觉质量上的优势,相较于现有方法,FID得分提高了34.81%。此外,Pix2Next在实践应用中展现出卓越性能,例如在下游对象检测任务中,利用生成的NIR数据增强有限的真实NIR数据集,证明了其能够扩大NIR数据集规模,无需额外的数据采集或标注工作,有望加快NIR计算机视觉应用的进步。
Key Takeaways
- Pix2Next是一个图像到图像翻译框架,旨在生成高质量的近红外图像。
- 采用先进的视觉基础模型,结合编码器-解码器架构和跨注意力机制,捕捉全局详细表示并保留关键光谱特征。
- 多尺度PatchGAN鉴别器确保在不同细节级别生成逼真的图像。
- 精心设计的损失函数结合了全局上下文理解和局部特征保留。
- 在RANUS数据集上进行的实验证明了Pix2Next在定量指标和视觉质量上的优势。
- Pix2Next能够利用生成的NIR数据增强有限的真实NIR数据集,提高下游任务性能。
点此查看论文截图
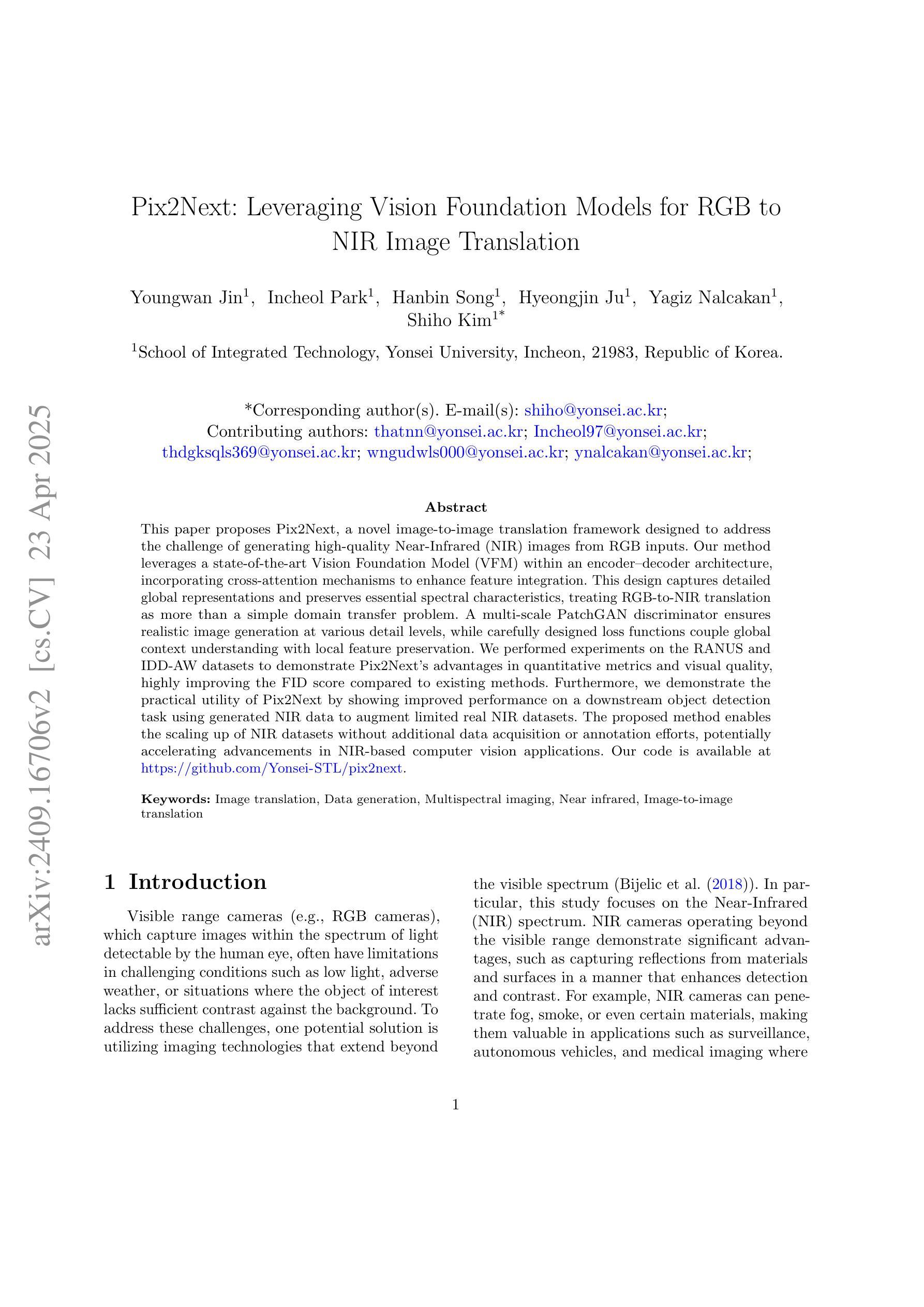
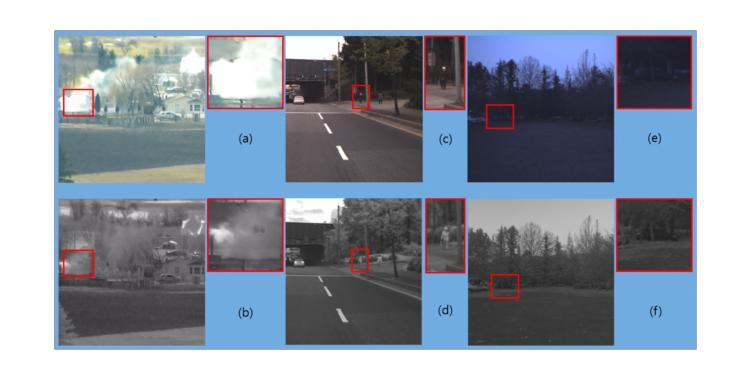
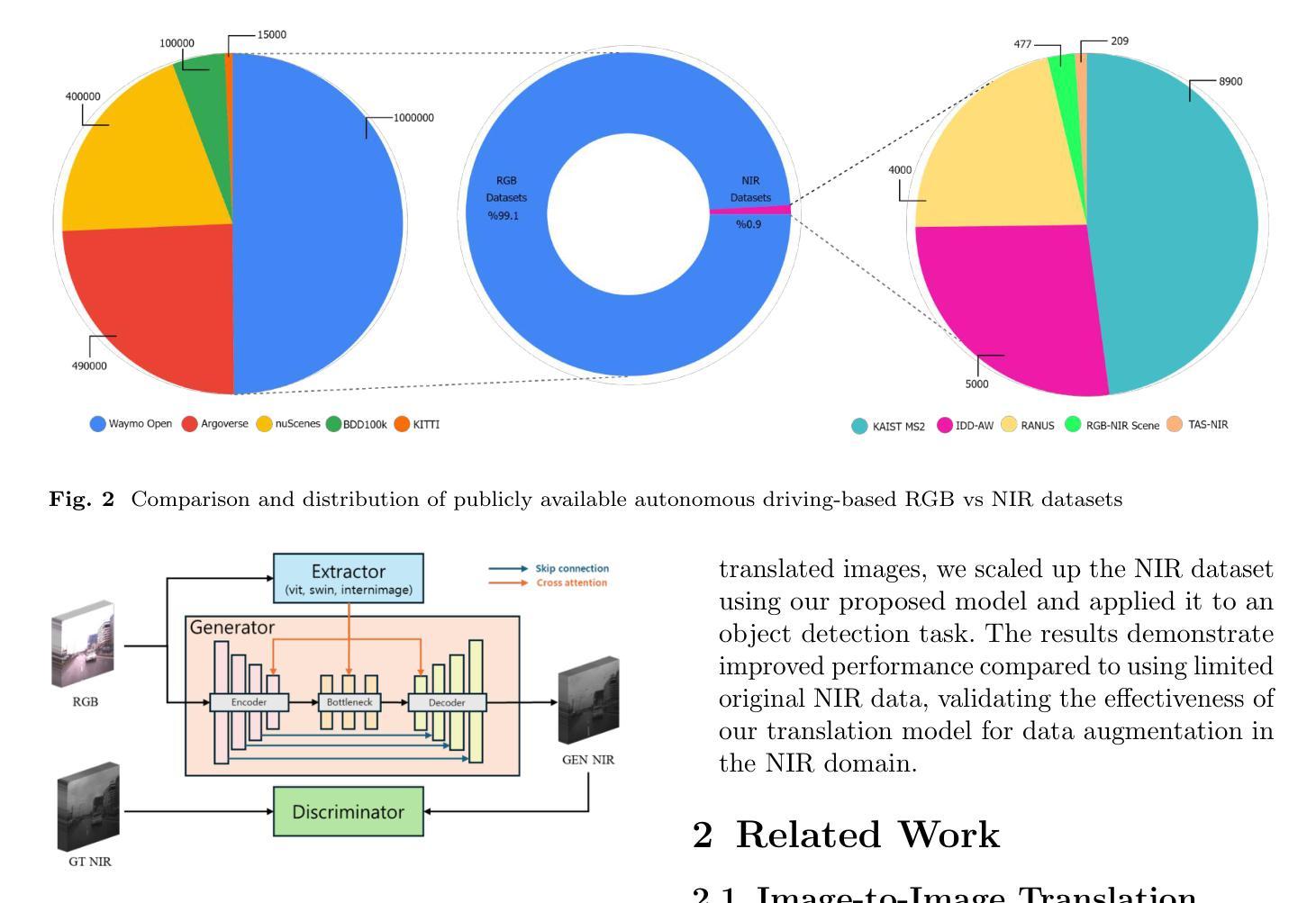
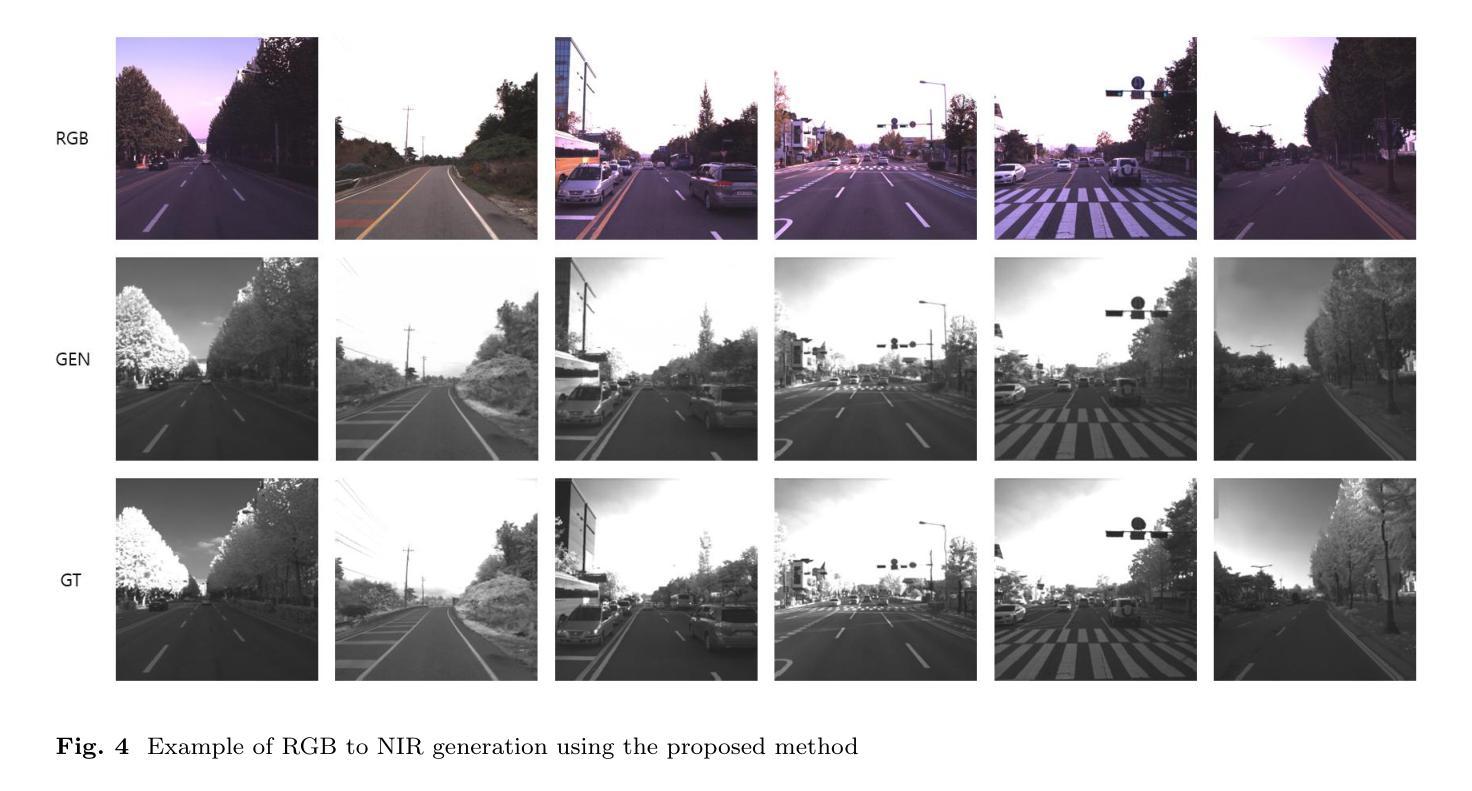
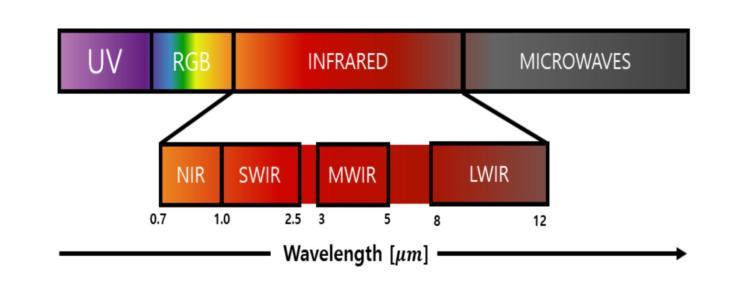
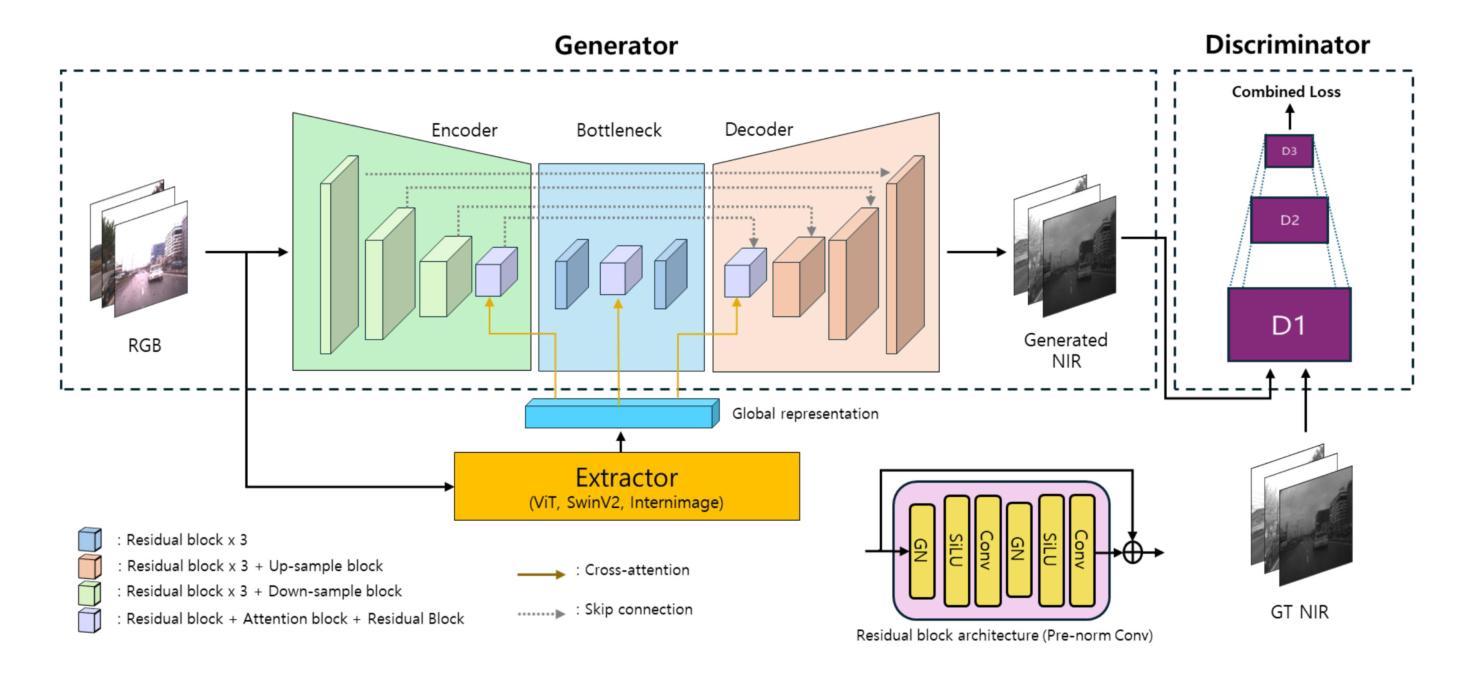