⚠️ 以下所有内容总结都来自于 大语言模型的能力,如有错误,仅供参考,谨慎使用
🔴 请注意:千万不要用于严肃的学术场景,只能用于论文阅读前的初筛!
💗 如果您觉得我们的项目对您有帮助 ChatPaperFree ,还请您给我们一些鼓励!⭐️ HuggingFace免费体验
2025-04-25 更新
FREAK: Frequency-modulated High-fidelity and Real-time Audio-driven Talking Portrait Synthesis
Authors:Ziqi Ni, Ao Fu, Yi Zhou
Achieving high-fidelity lip-speech synchronization in audio-driven talking portrait synthesis remains challenging. While multi-stage pipelines or diffusion models yield high-quality results, they suffer from high computational costs. Some approaches perform well on specific individuals with low resources, yet still exhibit mismatched lip movements. The aforementioned methods are modeled in the pixel domain. We observed that there are noticeable discrepancies in the frequency domain between the synthesized talking videos and natural videos. Currently, no research on talking portrait synthesis has considered this aspect. To address this, we propose a FREquency-modulated, high-fidelity, and real-time Audio-driven talKing portrait synthesis framework, named FREAK, which models talking portraits from the frequency domain perspective, enhancing the fidelity and naturalness of the synthesized portraits. FREAK introduces two novel frequency-based modules: 1) the Visual Encoding Frequency Modulator (VEFM) to couple multi-scale visual features in the frequency domain, better preserving visual frequency information and reducing the gap in the frequency spectrum between synthesized and natural frames. and 2) the Audio Visual Frequency Modulator (AVFM) to help the model learn the talking pattern in the frequency domain and improve audio-visual synchronization. Additionally, we optimize the model in both pixel domain and frequency domain jointly. Furthermore, FREAK supports seamless switching between one-shot and video dubbing settings, offering enhanced flexibility. Due to its superior performance, it can simultaneously support high-resolution video results and real-time inference. Extensive experiments demonstrate that our method synthesizes high-fidelity talking portraits with detailed facial textures and precise lip synchronization in real-time, outperforming state-of-the-art methods.
实现音频驱动的肖像谈话合成中的高保真唇语音同步仍然是一个挑战。虽然多阶段管道或扩散模型能产生高质量的结果,但它们面临着计算成本高的困境。一些方法在低资源条件下对特定个体表现良好,但仍会出现唇部动作不匹配的问题。上述方法都是在像素域进行建模的。我们观察到,合成对话视频和自然视频在频率域存在明显的差异。目前,尚无关于肖像谈话合成的研究考虑这一因素。为了解决这一问题,我们提出了一个名为FREAK的频率调制、高保真、实时音频驱动肖像谈话合成框架,该框架从频率域的角度对肖像进行建模,提高了合成肖像的保真度和自然度。FREAK引入了两个新颖的频率模块:1)视觉编码频率调制器(VEFM),用于在频率域中结合多尺度视觉特征,更好地保留视觉频率信息,减少合成帧和自然帧之间的频谱差距;2)视听频率调制器(AVFM),帮助模型学习频率域的说话模式,提高视听同步性。此外,我们在像素域和频率域中联合优化模型。而且,FREAK支持在一键式和视频配音设置之间进行无缝切换,提供了增强的灵活性。凭借其卓越性能,它可以同时支持高分辨率视频结果和实时推理。大量实验表明,我们的方法在实时合成高保真谈话肖像方面表现出色,具有详细的面部纹理和精确的唇同步,超越了现有最先进的方法。
论文及项目相关链接
PDF Accepted by ICMR 2025
摘要
实现高保真度的音频驱动说话肖像合成中的唇语音同步仍然具有挑战性。当前的方法大多在像素域建模,存在计算成本高、特定个体资源匹配不佳等问题。本文提出一种频率调制、高保真、实时音频驱动说话肖像合成框架——FREAK,从频率域角度建模说话肖像,提高合成肖像的保真度和自然度。FREAK引入两个新型频率基础模块:视觉编码频率调制器(VEFM)和音频视觉频率调制器(AVFM),以改善合成视频的频率域特性,提高音频与视觉的同步效果。通过像素域和频率域的联合优化,FREAK支持在一击和影片配音设置之间的无缝切换,提供增强的灵活性。实验表明,该方法能实时合成高保真度的说话肖像,具有详细的面部纹理和精确的唇同步效果,优于现有技术。
关键见解
- 实现音频驱动的说话肖像合成中的唇语音同步是一项挑战。
- 当前方法在像素域建模,存在计算成本高和特定个体资源匹配问题。
- FREAK框架从频率域角度建模说话肖像,提高合成肖像的保真度和自然度。
- FREAK引入视觉编码频率调制器(VEFM)和音频视觉频率调制器(AVFM)改善合成视频的频率域特性。
- FREAK支持像素域和频率域的联合优化,实现无缝切换不同设置。
- FREAK具有实时合成高保真说话肖像的能力,具有详细的面部纹理和精确的唇同步效果。
点此查看论文截图
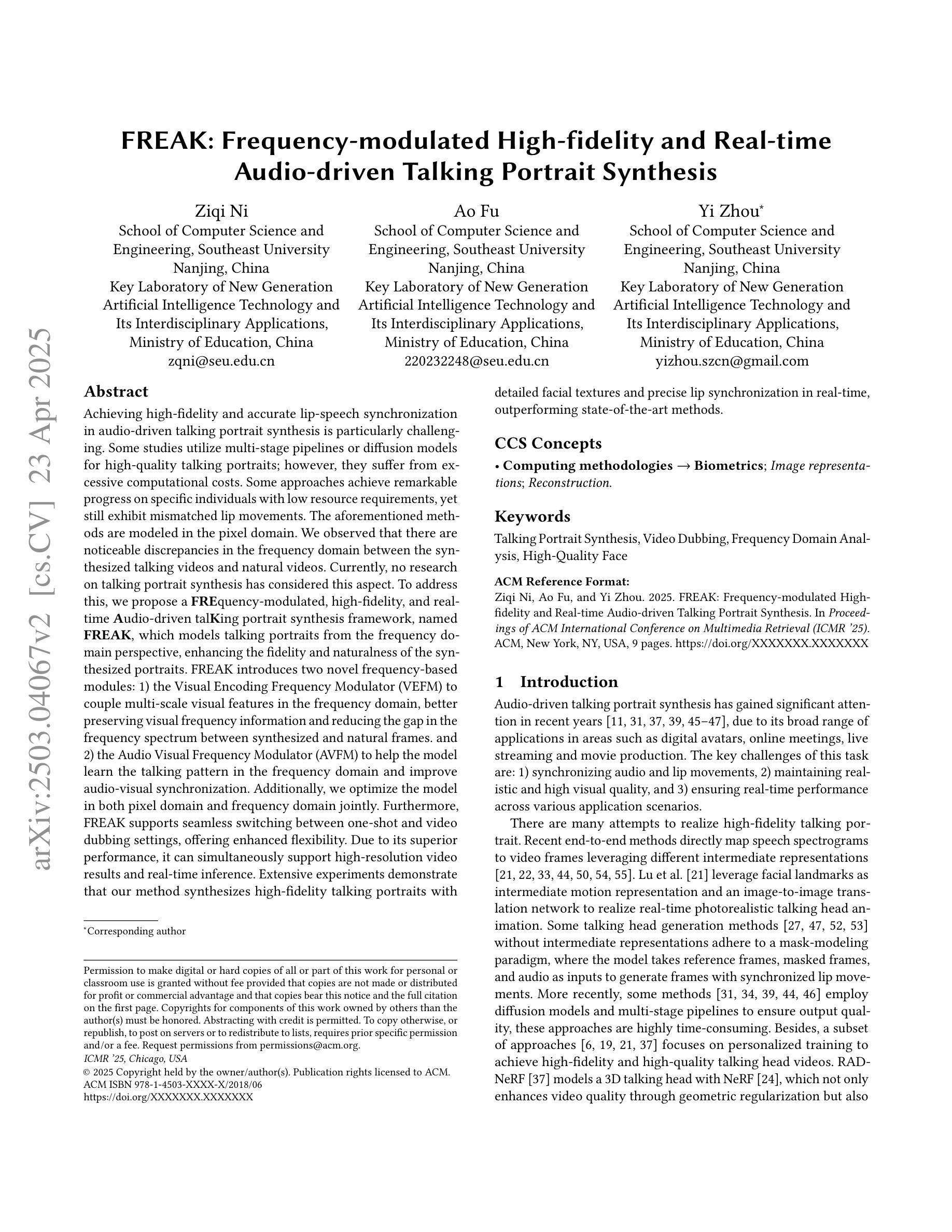
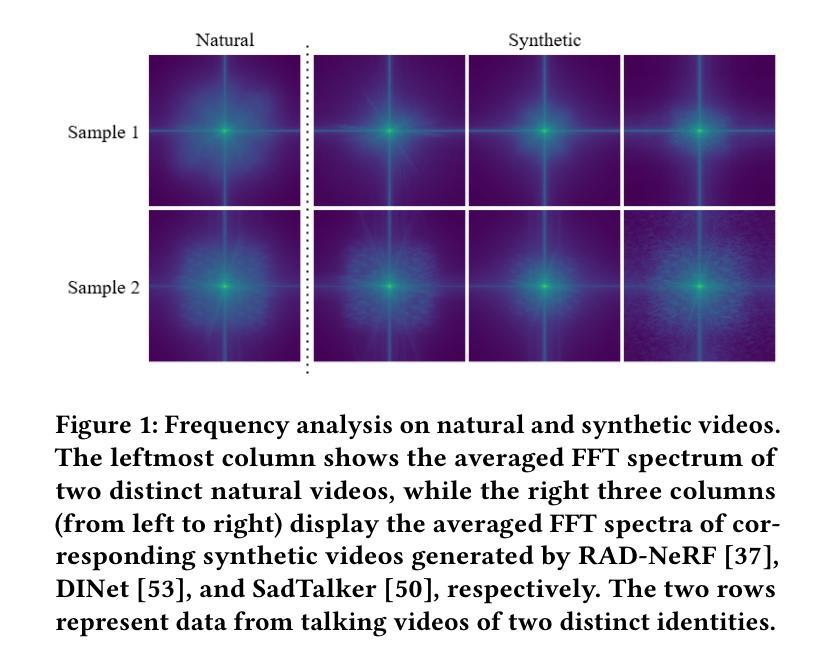
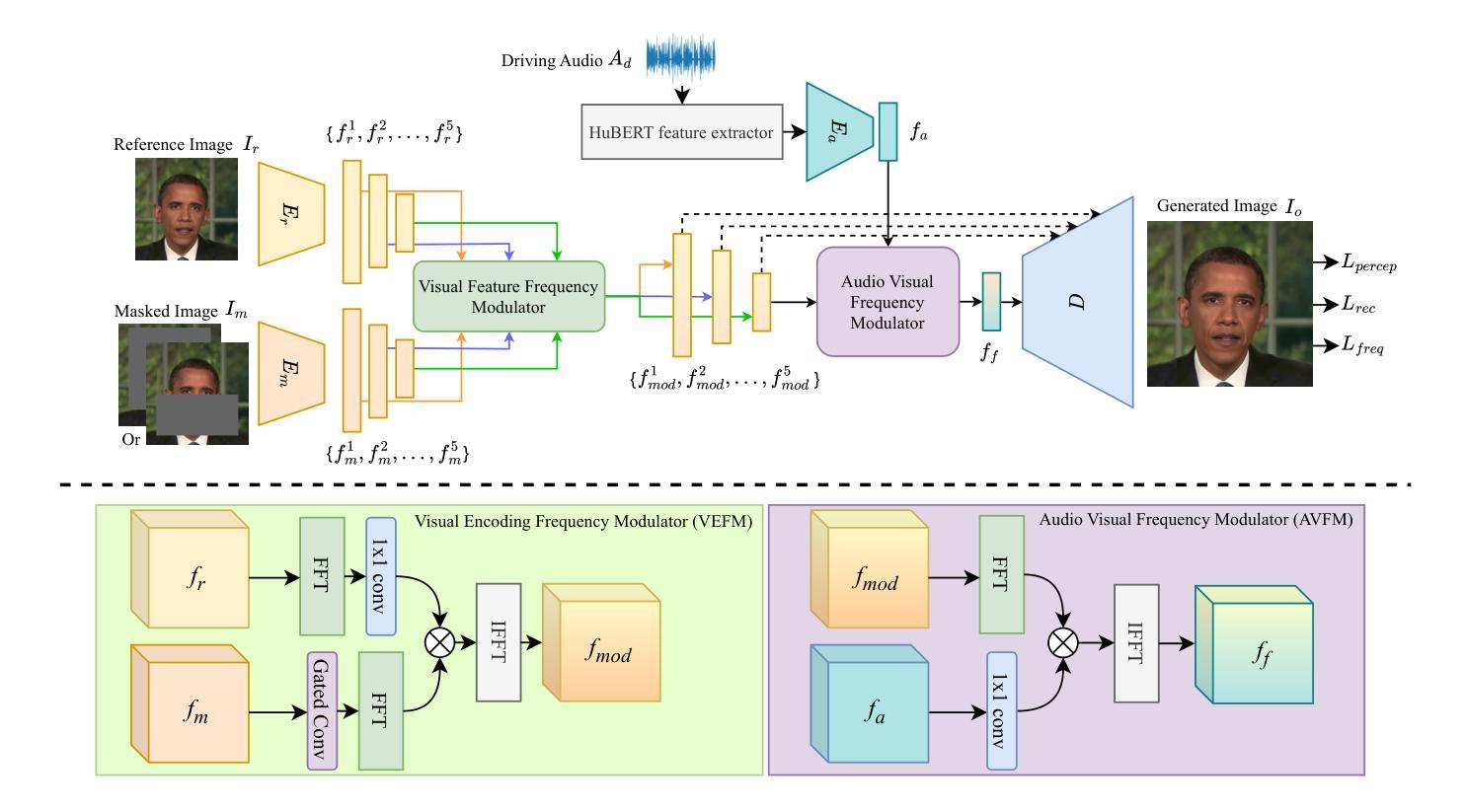
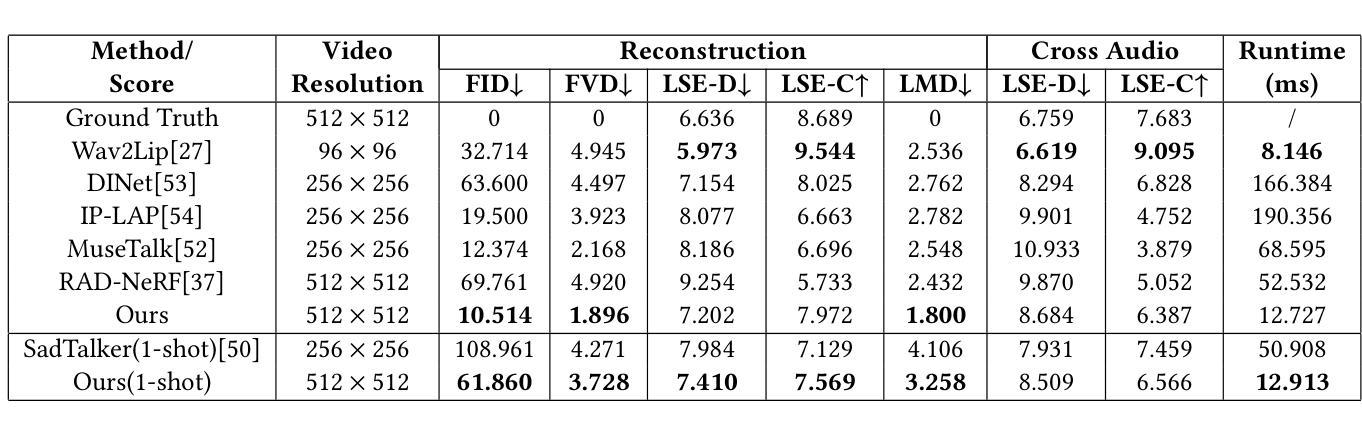
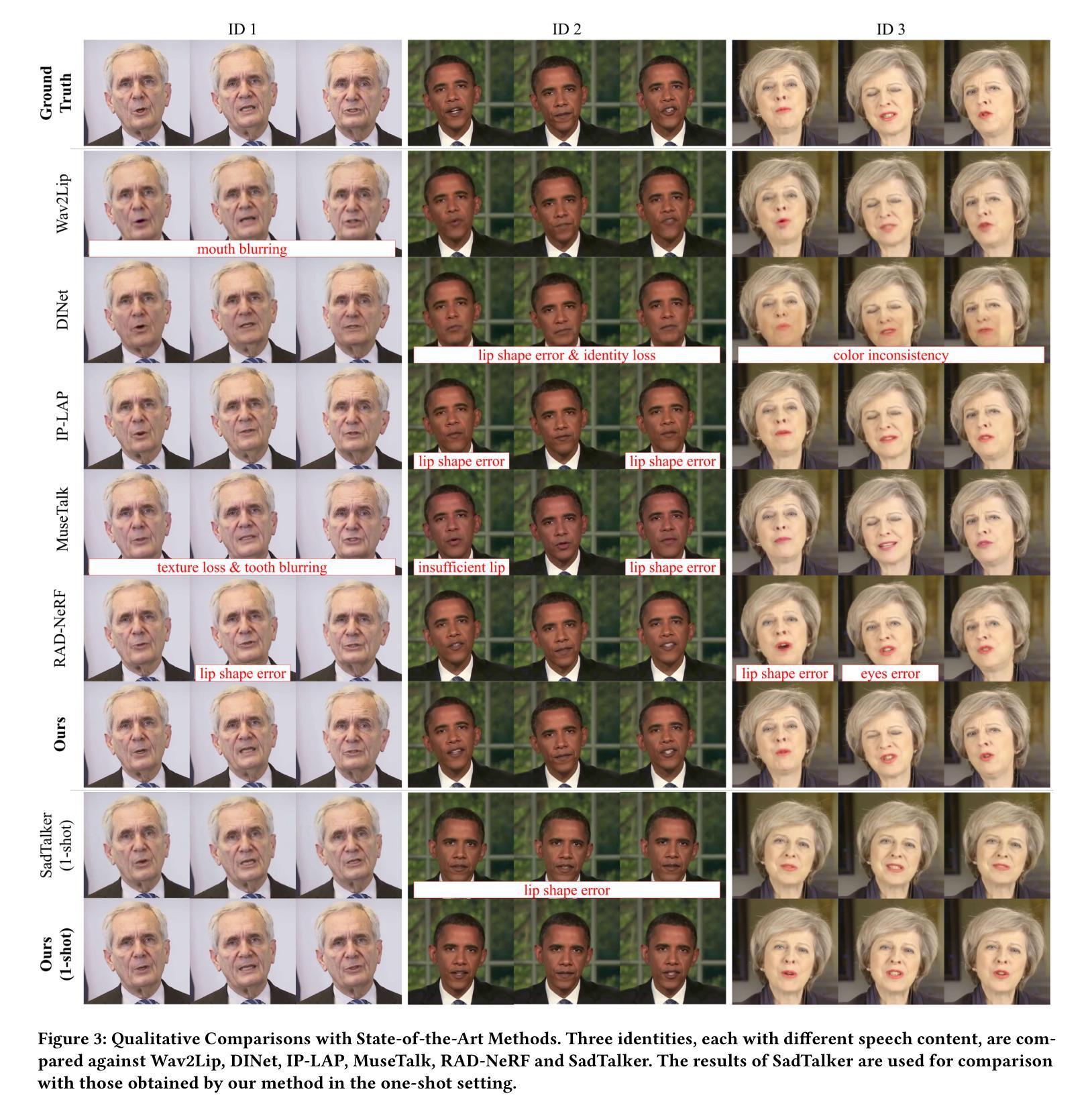
FLAP: Fully-controllable Audio-driven Portrait Video Generation through 3D head conditioned diffusion model
Authors:Lingzhou Mu, Baiji Liu, Ruonan Zhang, Guiming Mo, Jiawei Jin, Kai Zhang, Haozhi Huang
Diffusion-based video generation techniques have significantly improved zero-shot talking-head avatar generation, enhancing the naturalness of both head motion and facial expressions. However, existing methods suffer from poor controllability, making them less applicable to real-world scenarios such as filmmaking and live streaming for e-commerce. To address this limitation, we propose FLAP, a novel approach that integrates explicit 3D intermediate parameters (head poses and facial expressions) into the diffusion model for end-to-end generation of realistic portrait videos. The proposed architecture allows the model to generate vivid portrait videos from audio while simultaneously incorporating additional control signals, such as head rotation angles and eye-blinking frequency. Furthermore, the decoupling of head pose and facial expression allows for independent control of each, offering precise manipulation of both the avatar’s pose and facial expressions. We also demonstrate its flexibility in integrating with existing 3D head generation methods, bridging the gap between 3D model-based approaches and end-to-end diffusion techniques. Extensive experiments show that our method outperforms recent audio-driven portrait video models in both naturalness and controllability.
基于扩散的视频生成技术极大地改进了零样本说话人头像生成,提高了头部运动和面部表情的自然性。然而,现有方法存在可控性差的问题,使得它们不适用于电影制作和电子商务直播等现实世界场景。为了解决这一局限性,我们提出了FLAP,这是一种将明确的3D中间参数(头部姿势和面部表情)集成到扩散模型中的新方法,以进行逼真的肖像视频端到端生成。该架构允许模型从音频生成生动肖像视频,同时融入额外的控制信号,如头部旋转角度和眨眼频率。此外,头部姿势和面部表情的解耦使得两者能够独立控制,实现对头像姿势和面部表情的精确操作。我们还展示了它与现有3D头像生成方法的灵活性,缩小了基于3D模型的方法和端到端扩散技术之间的差距。大量实验表明,我们的方法在自然性和可控性方面优于最新的音频驱动肖像视频模型。
论文及项目相关链接
Summary
在基于扩散的视频生成技术中,零起点说话人头像生成得到显著改善,头部运动和面部表情的自然性得到提高。然而,现有方法可控性较差,难以适用于电影制作和电商直播等现实场景。为解决此问题,我们提出FLAP方法,将明确的3D中间参数(头部姿态和面部表情)整合到扩散模型中,实现逼真肖像视频的端到端生成。该方法可从音频生成生动肖像视频,同时纳入额外的控制信号,如头部旋转角度和眨眼频率。头部姿态和面部表情的解耦,使得两者可独立控制,实现对虚拟角色姿态和表情的精准操控。此外,该方法还展示了与现有3D头像生成方法的灵活集成,缩小了基于3D模型的方法和端到端的扩散技术之间的差距。实验证明,我们的方法在音频驱动的肖像视频模型中的自然性和可控性方面均表现出优异性能。
Key Takeaways
- 扩散模型技术已显著改善零起点说话人头像生成的自然性,包括头部运动和面部表情。
- 现有方法缺乏可控性,限制了其在现实场景中的应用。
- FLAP方法整合3D中间参数到扩散模型中,实现肖像视频的端到端生成。
- FLAP方法可从音频生成生动肖像视频,并纳入额外的控制信号。
- 头部姿态和面部表情的解耦允许独立控制,提高虚拟角色的精准操控。
- FLAP方法与现有3D头像生成方法可灵活集成,缩小技术差距。
点此查看论文截图
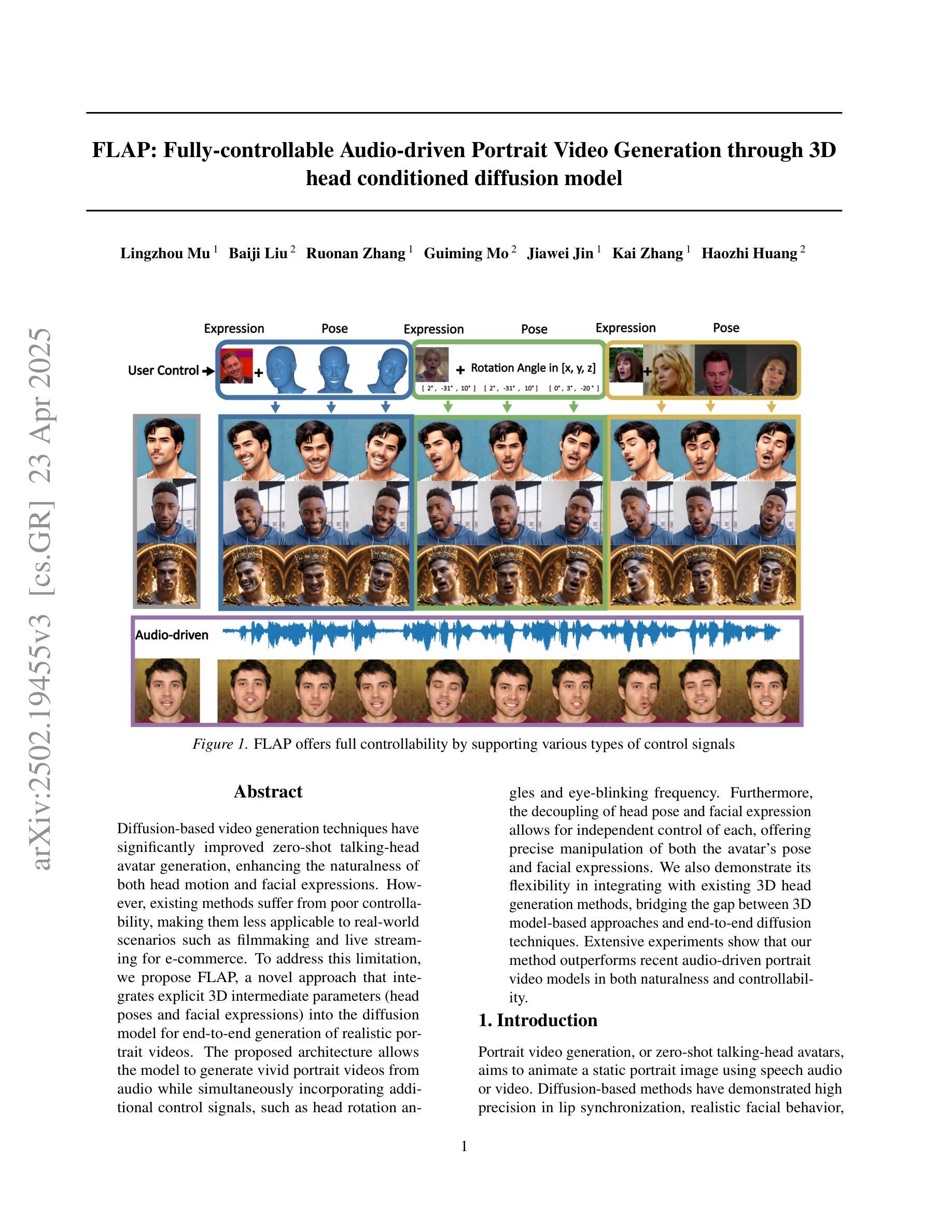
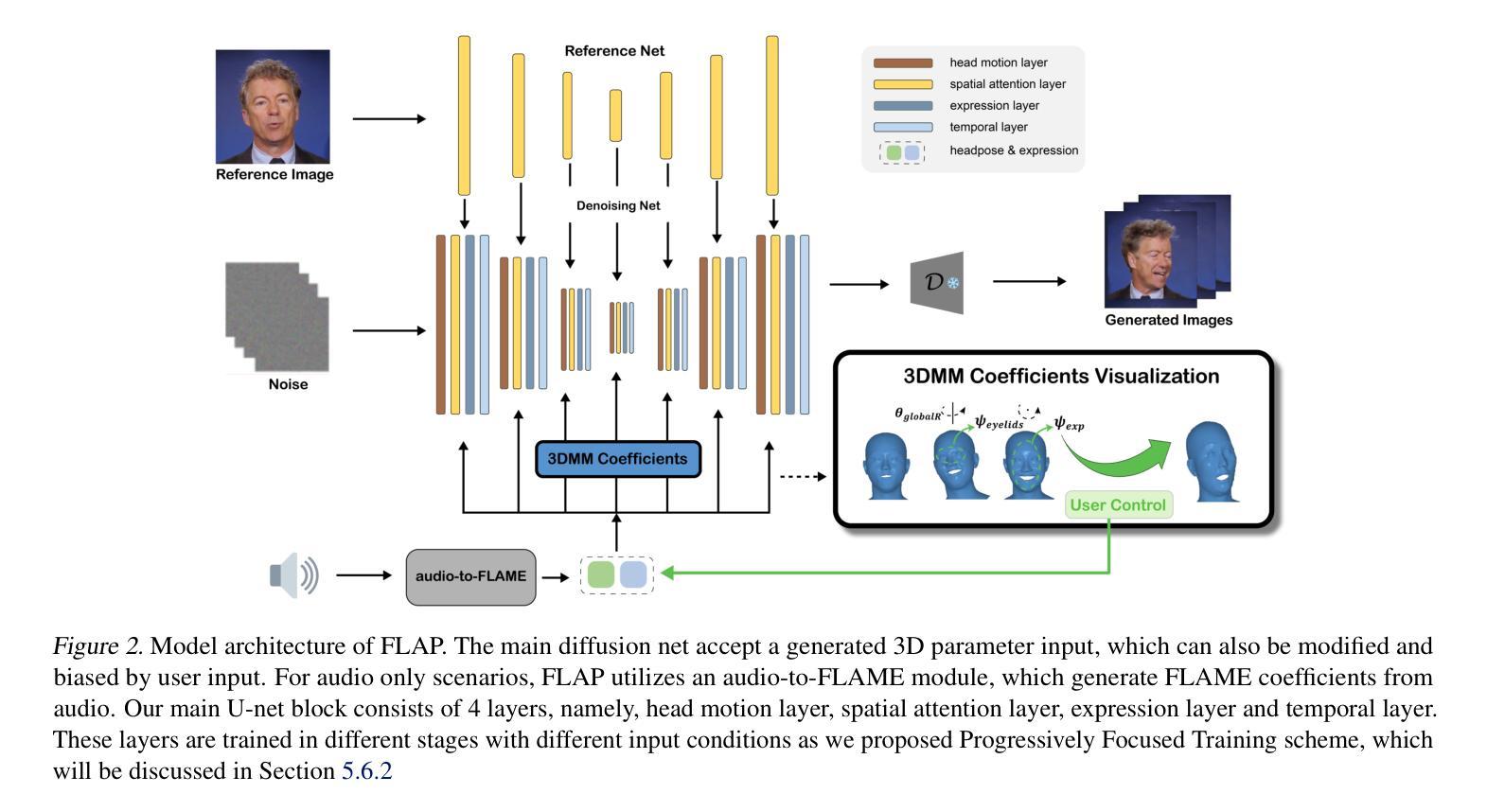
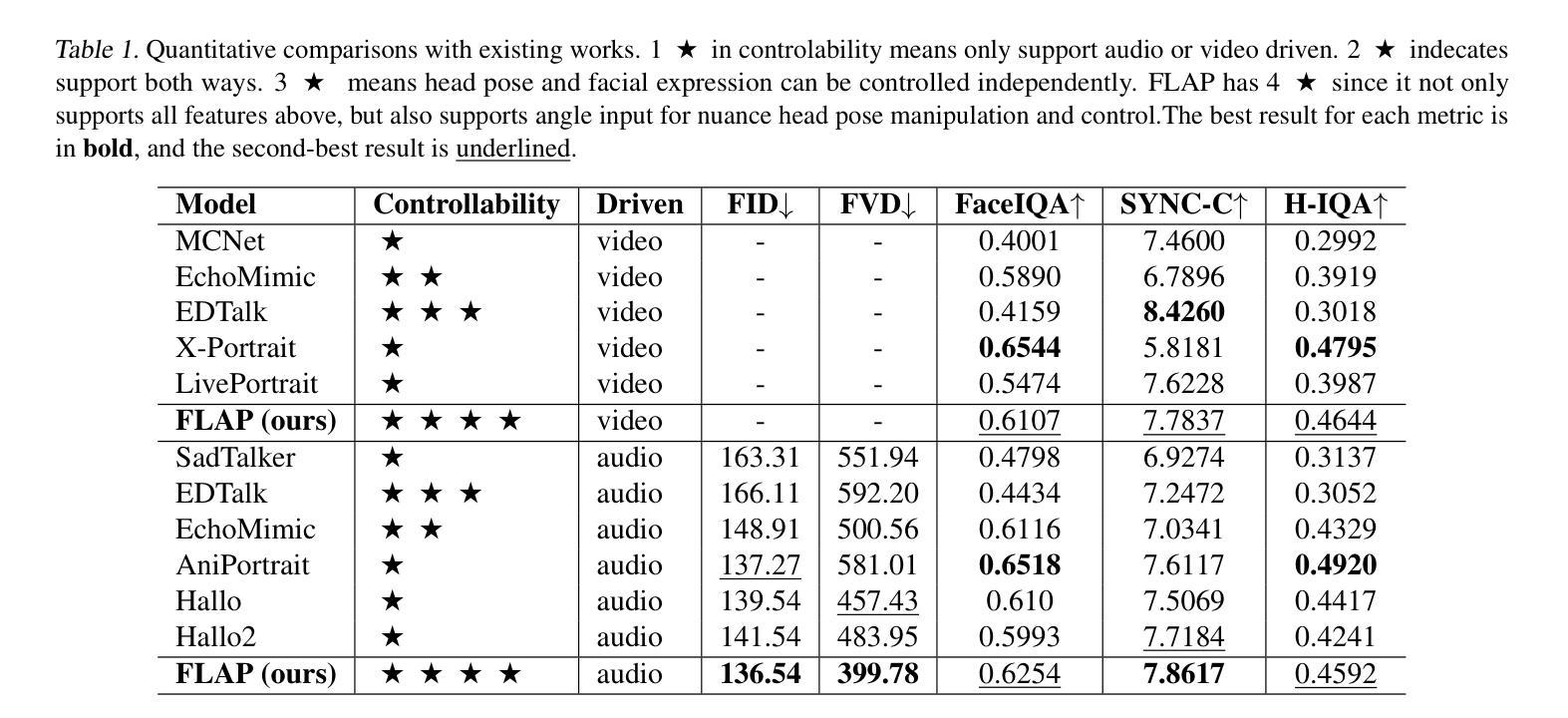