⚠️ 以下所有内容总结都来自于 大语言模型的能力,如有错误,仅供参考,谨慎使用
🔴 请注意:千万不要用于严肃的学术场景,只能用于论文阅读前的初筛!
💗 如果您觉得我们的项目对您有帮助 ChatPaperFree ,还请您给我们一些鼓励!⭐️ HuggingFace免费体验
2025-04-26 更新
Scene-Aware Location Modeling for Data Augmentation in Automotive Object Detection
Authors:Jens Petersen, Davide Abati, Amirhossein Habibian, Auke Wiggers
Generative image models are increasingly being used for training data augmentation in vision tasks. In the context of automotive object detection, methods usually focus on producing augmented frames that look as realistic as possible, for example by replacing real objects with generated ones. Others try to maximize the diversity of augmented frames, for example by pasting lots of generated objects onto existing backgrounds. Both perspectives pay little attention to the locations of objects in the scene. Frame layouts are either reused with little or no modification, or they are random and disregard realism entirely. In this work, we argue that optimal data augmentation should also include realistic augmentation of layouts. We introduce a scene-aware probabilistic location model that predicts where new objects can realistically be placed in an existing scene. By then inpainting objects in these locations with a generative model, we obtain much stronger augmentation performance than existing approaches. We set a new state of the art for generative data augmentation on two automotive object detection tasks, achieving up to $2.8\times$ higher gains than the best competing approach ($+1.4$ vs. $+0.5$ mAP boost). We also demonstrate significant improvements for instance segmentation.
图像生成模型在视觉任务中越来越常用于训练数据增强。在汽车目标检测的背景下,方法通常侧重于生成尽可能逼真的增强帧,例如通过用生成的目标替换真实目标。其他人则试图最大化增强帧的多样性,例如通过将许多生成的目标粘贴到现有背景上。这两种方法都很少关注场景中物体的位置。帧布局要么稍作修改或不加修改地重复使用,要么完全随机且不注重真实性。在这项工作中,我们认为最佳数据增强还应包括布局的真实性增强。我们引入了一个场景感知的概率位置模型,该模型可以预测新物体可以真实放置在现有场景中的位置。然后,在这些位置使用生成模型进行对象填充,我们获得了比现有方法更强的增强性能。我们在两个汽车目标检测任务上为生成数据增强设置了新的技术状态,与最佳竞争方法相比,我们实现了高达2.8倍的更高收益(+1.4 vs. +0.5 mAP提升)。我们还证明了实例分割的显著改善。
论文及项目相关链接
Summary
在汽车目标检测中,传统数据增强方法往往忽视场景布局的真实性和多样性。本文提出了一种场景感知的概率位置模型,预测新对象在现有场景中的合理放置位置,并利用生成模型在这些位置进行对象填充,实现了强大的数据增强效果,达到了最新的目标检测水平。
Key Takeaways
- 生成图像模型在视觉任务中用于训练数据增强。
- 传统的数据增强方法在汽车目标检测中主要关注对象的真实性或多样性,但忽略了场景中对象的位置。
- 本文提出了一种场景感知的概率位置模型,该模型能预测新对象在现有场景中的合理放置位置。
- 该模型结合生成模型和场景感知的概率位置模型进行对象填充,实现了强大的数据增强效果。
- 该方法在两个汽车目标检测任务上达到了最新的水平,相较于最佳竞争方法,增益高达2.8倍(mAP提升+1.4 vs +0.5)。
- 除了目标检测,该方法在实例分割上也表现出了显著的改进。
- 该方法的优点在于结合了真实性和场景感知,从而提高了数据增强的效果。
点此查看论文截图
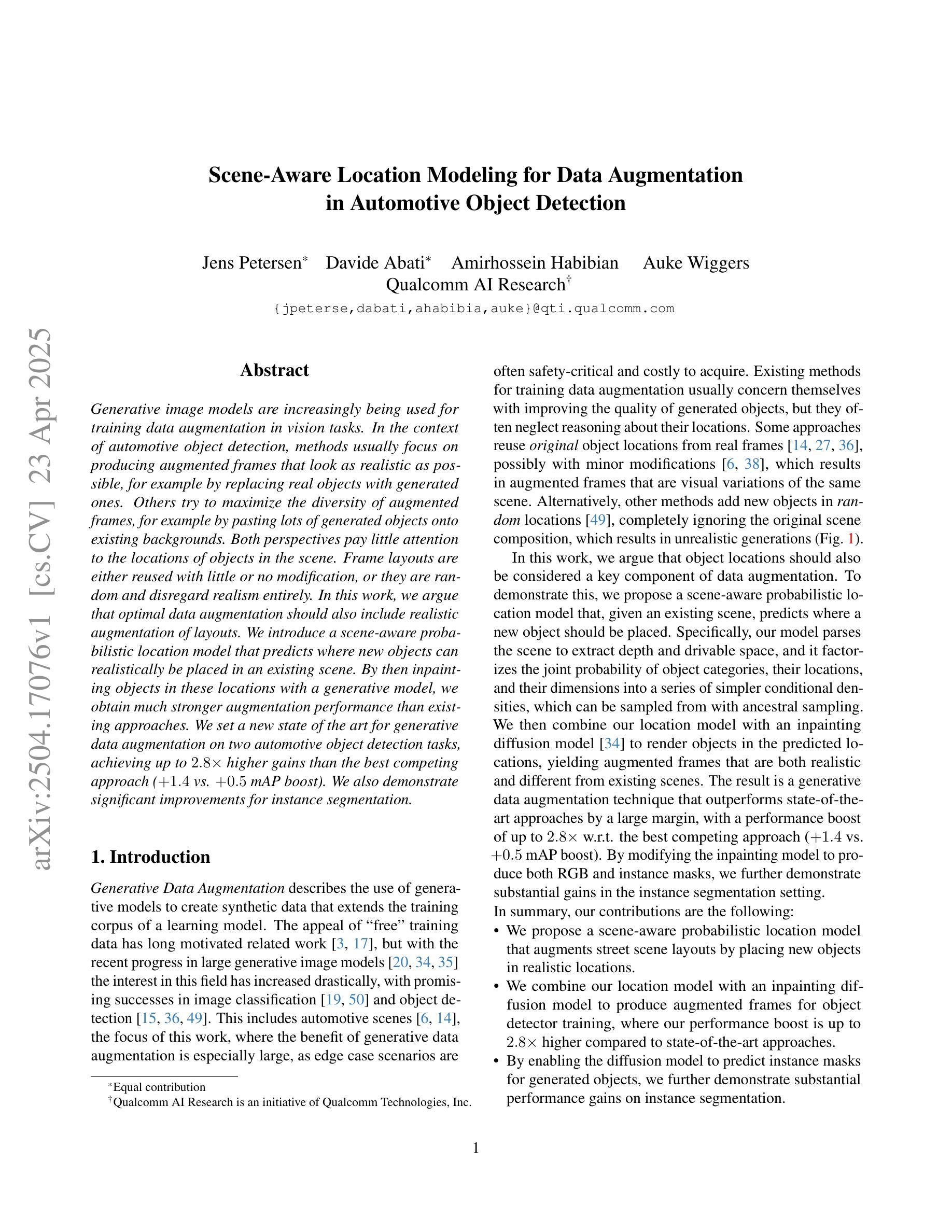
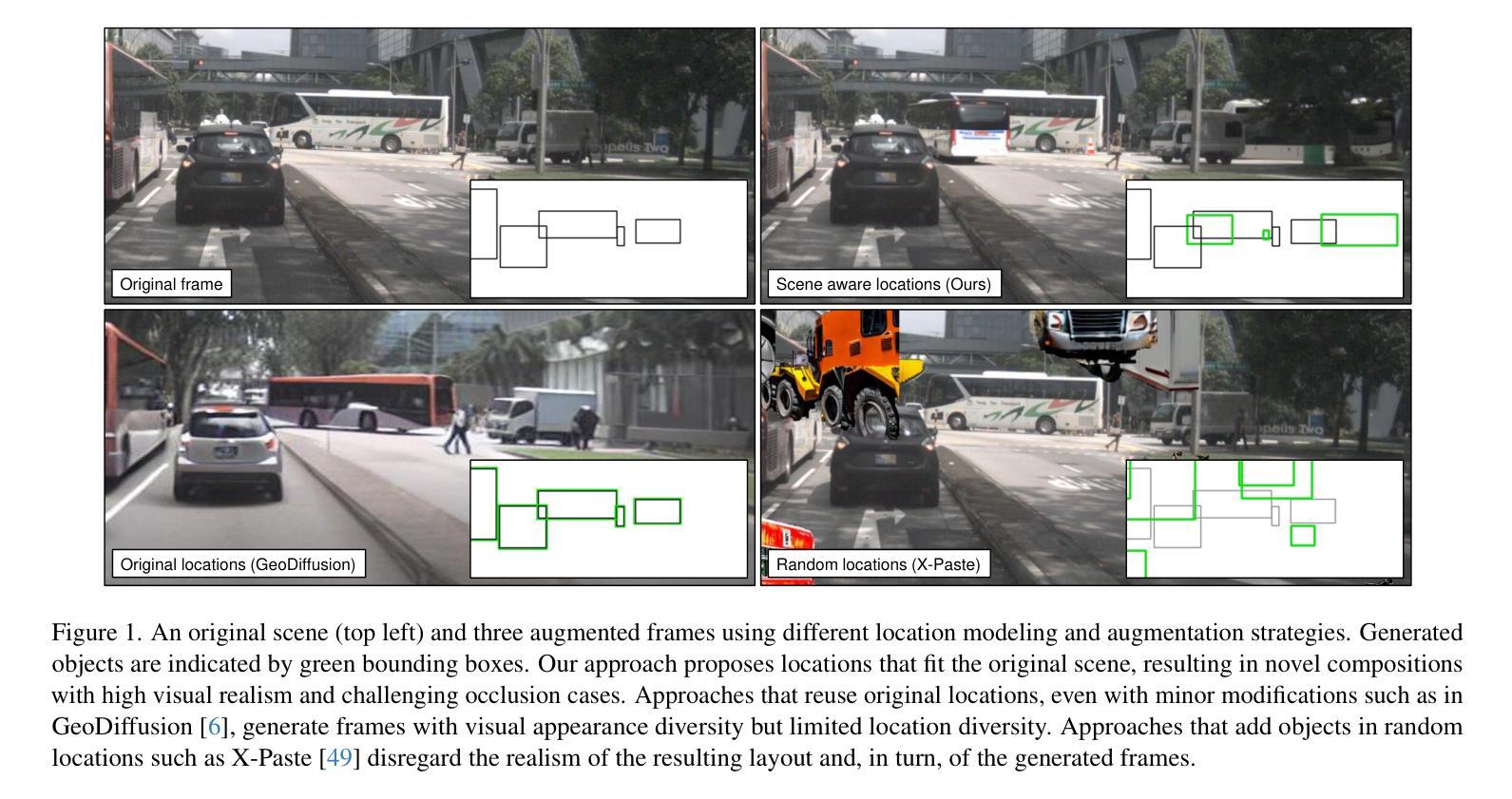
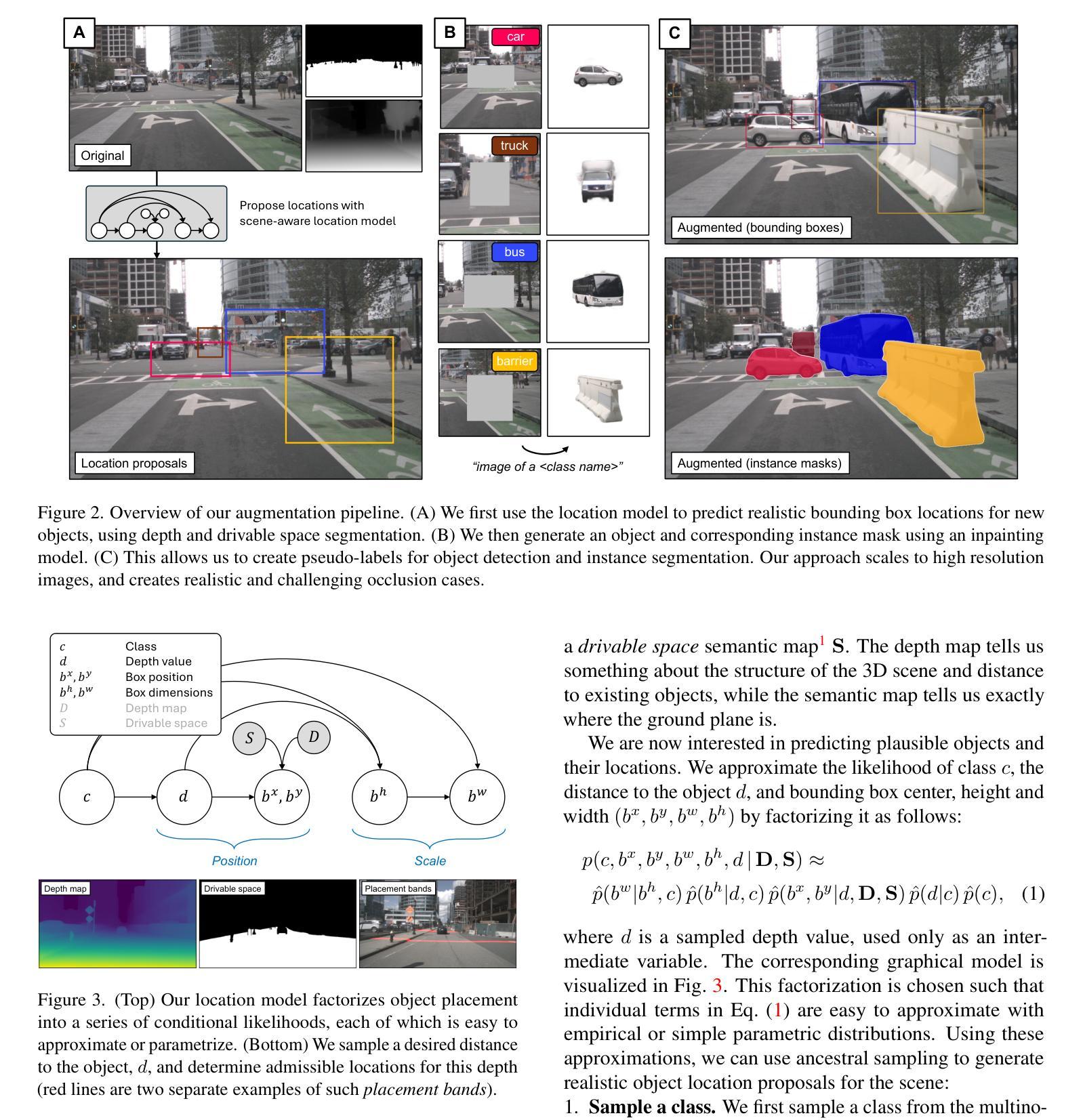
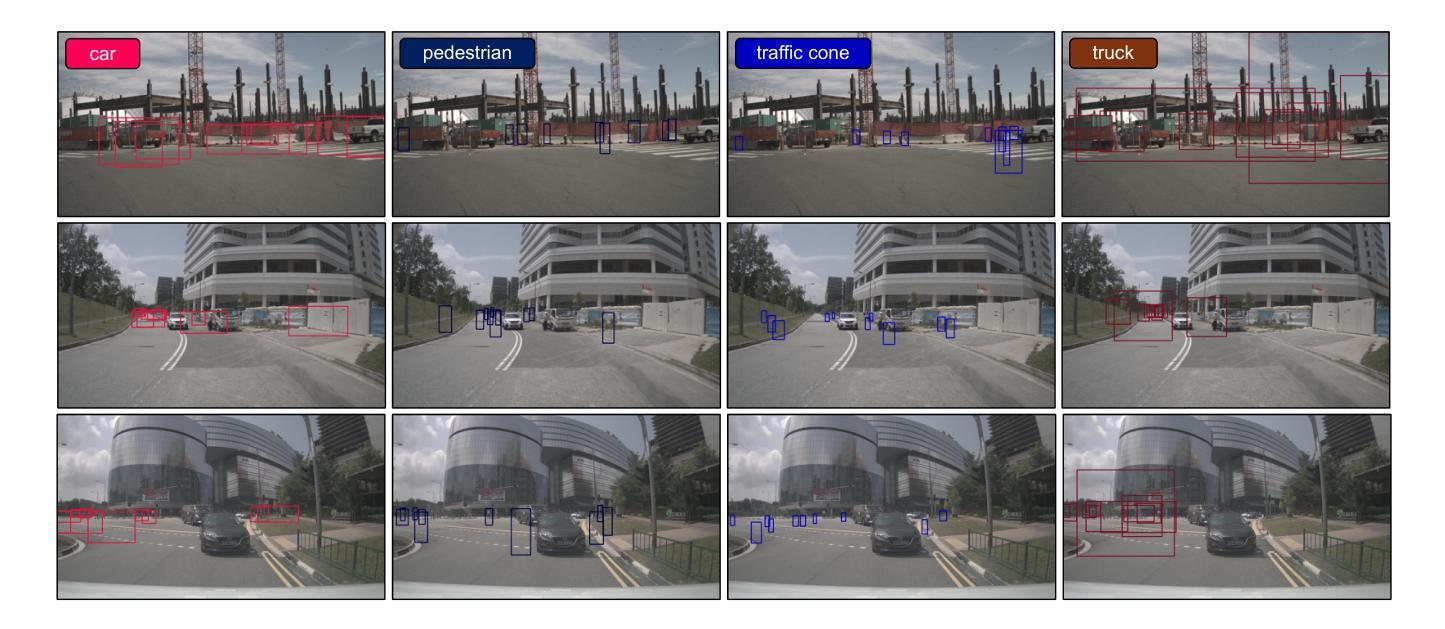
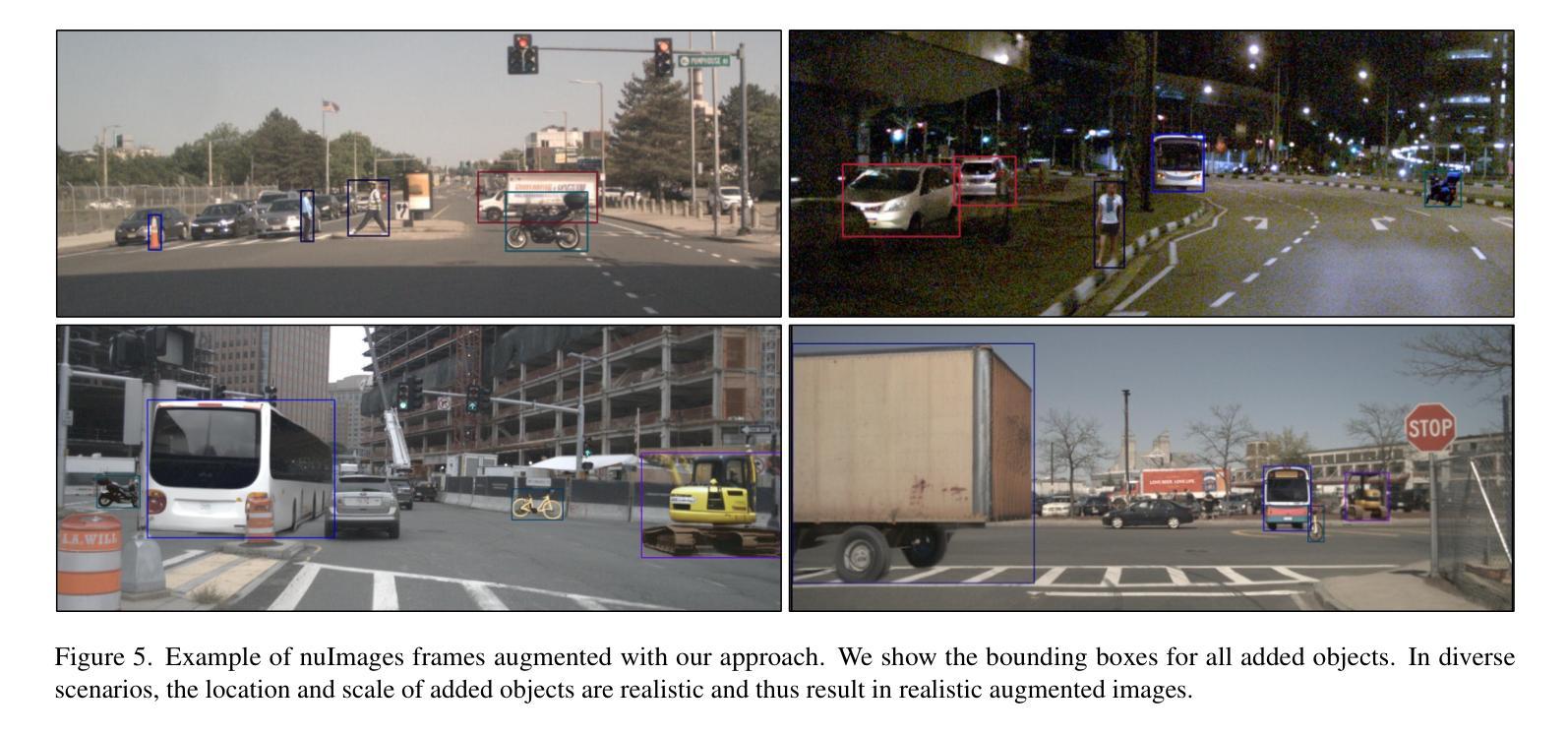
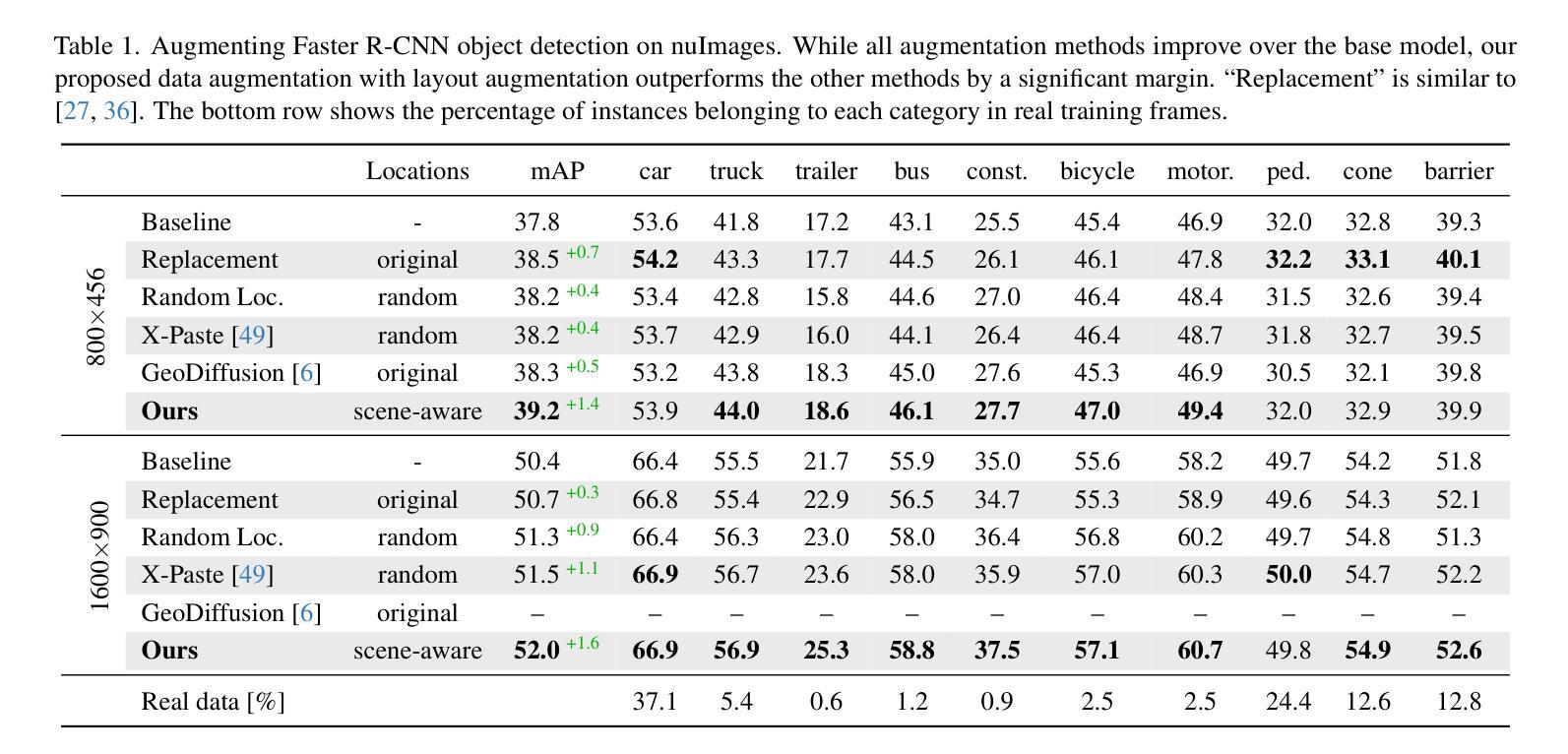