⚠️ 以下所有内容总结都来自于 大语言模型的能力,如有错误,仅供参考,谨慎使用
🔴 请注意:千万不要用于严肃的学术场景,只能用于论文阅读前的初筛!
💗 如果您觉得我们的项目对您有帮助 ChatPaperFree ,还请您给我们一些鼓励!⭐️ HuggingFace免费体验
2025-04-26 更新
MCAF: Efficient Agent-based Video Understanding Framework through Multimodal Coarse-to-Fine Attention Focusing
Authors:Shiwen Cao, Zhaoxing Zhang, Junming Jiao, Juyi Qiao, Guowen Song, Rong Shen
Even in the era of rapid advances in large models, video understanding, particularly long videos, remains highly challenging. Compared with textual or image-based information, videos commonly contain more information with redundancy, requiring large models to strategically allocate attention at a global level for accurate comprehension. To address this, we propose MCAF, an agent-based, training-free framework perform video understanding through Multimodal Coarse-to-fine Attention Focusing. The key innovation lies in its ability to sense and prioritize segments of the video that are highly relevant to the understanding task. First, MCAF hierarchically concentrates on highly relevant frames through multimodal information, enhancing the correlation between the acquired contextual information and the query. Second, it employs a dilated temporal expansion mechanism to mitigate the risk of missing crucial details when extracting information from these concentrated frames. In addition, our framework incorporates a self-reflection mechanism utilizing the confidence level of the model’s responses as feedback. By iteratively applying these two creative focusing strategies, it adaptively adjusts attention to capture highly query-connected context and thus improves response accuracy. MCAF outperforms comparable state-of-the-art methods on average. On the EgoSchema dataset, it achieves a remarkable 5% performance gain over the leading approach. Meanwhile, on Next-QA and IntentQA datasets, it outperforms the current state-of-the-art standard by 0.2% and 0.3% respectively. On the Video-MME dataset, which features videos averaging nearly an hour in length, MCAF also outperforms other agent-based methods.
即使在大型模型迅猛发展的时代,视频理解,尤其是长视频理解,仍然极具挑战性。与文本或图像信息相比,视频通常包含更多冗余信息,需要大型模型在全局层面策略性地分配注意力以实现准确理解。为了解决这一问题,我们提出了MCAF(基于注意力集中因子的视频理解框架),这是一种基于主体且无需训练的框架,可通过多模态从粗到细注意力集中机制执行视频理解任务。其关键创新之处在于能够感知并优先处理与理解任务高度相关的视频片段。首先,MCAF通过多模态信息层次性地关注高度相关的帧,增强获取上下文信息与查询之间的相关性。其次,它采用膨胀的时间扩展机制,以减少从集中帧中提取信息时遗漏关键细节的风险。此外,我们的框架还采用了一种自我反馈机制,利用模型响应的置信度作为反馈。通过迭代应用这两种创新的聚焦策略,它能够自适应地调整注意力以捕获与查询高度相关的上下文,从而提高响应准确性。MCAF在平均水平上优于其他最先进的方法。在EgoSchema数据集上,它比领先的方法取得了显著的5%的性能提升。同时,在Next-QA和IntentQA数据集上,它的性能分别超出了当前最新标准0.2%和0.3%。在平均时长接近一小时的视频数据集Video-MME上,MCAF也优于其他基于主体的方法。
论文及项目相关链接
Summary
视频理解,尤其是长视频的理解,仍然极具挑战性。针对该问题,提出了MCAF框架,一种基于多模态粗到细注意力聚焦的无训练视频理解框架。该框架能感知并优先处理与理解任务高度相关的视频片段,通过分层的方式集中在高度相关的帧上,并增强获取上下文信息与查询之间的相关性。此外,它采用膨胀的时间扩展机制,并融入模型的响应置信度作为反馈,自适应地调整注意力以捕捉与查询高度相关的上下文,从而提高响应的准确性。MCAF在多个数据集上的表现均优于当前先进方法。
Key Takeaways
- 视频理解仍是挑战,需处理大量冗余信息,需要模型全局地分配注意力。
- MCAF是一个无训练的视频理解框架,基于多模态信息实现粗到细的注意力聚焦。
- MCAF能感知并优先处理与理解任务高度相关的视频片段。
- 通过分层方式集中在高度相关的帧上,增强上下文与查询的相关性。
- 采用膨胀的时间扩展机制,减少提取信息时错过关键细节的风险。
- 融入模型的响应置信度作为反馈,提高响应的准确性。
点此查看论文截图
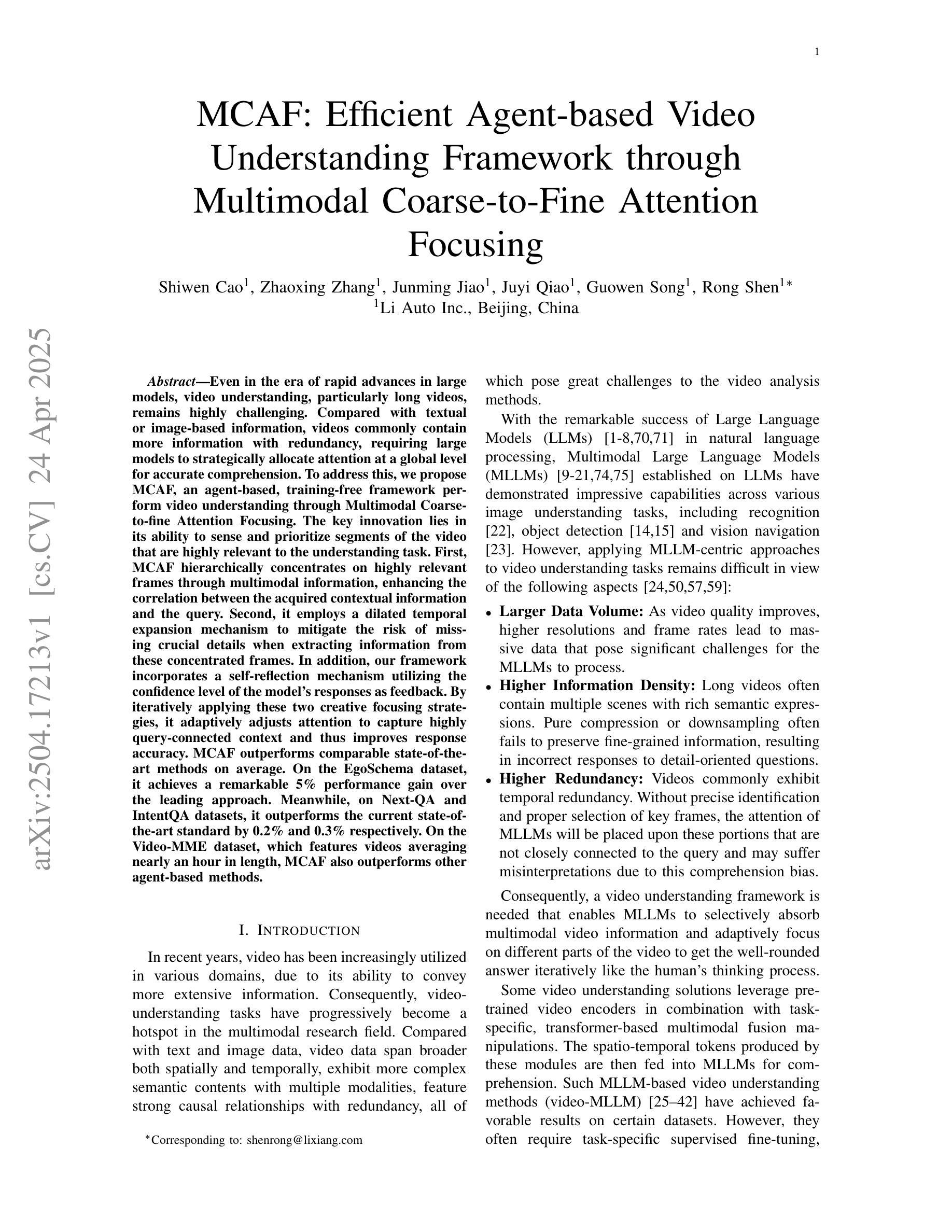
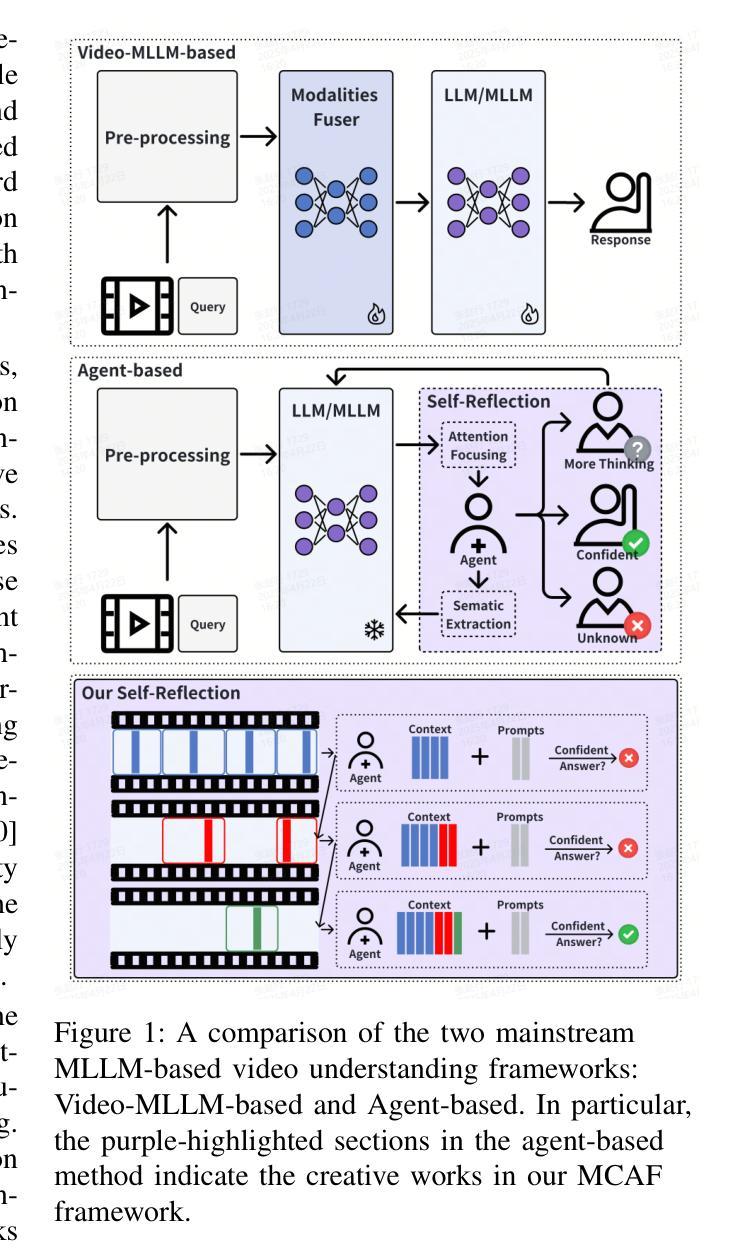
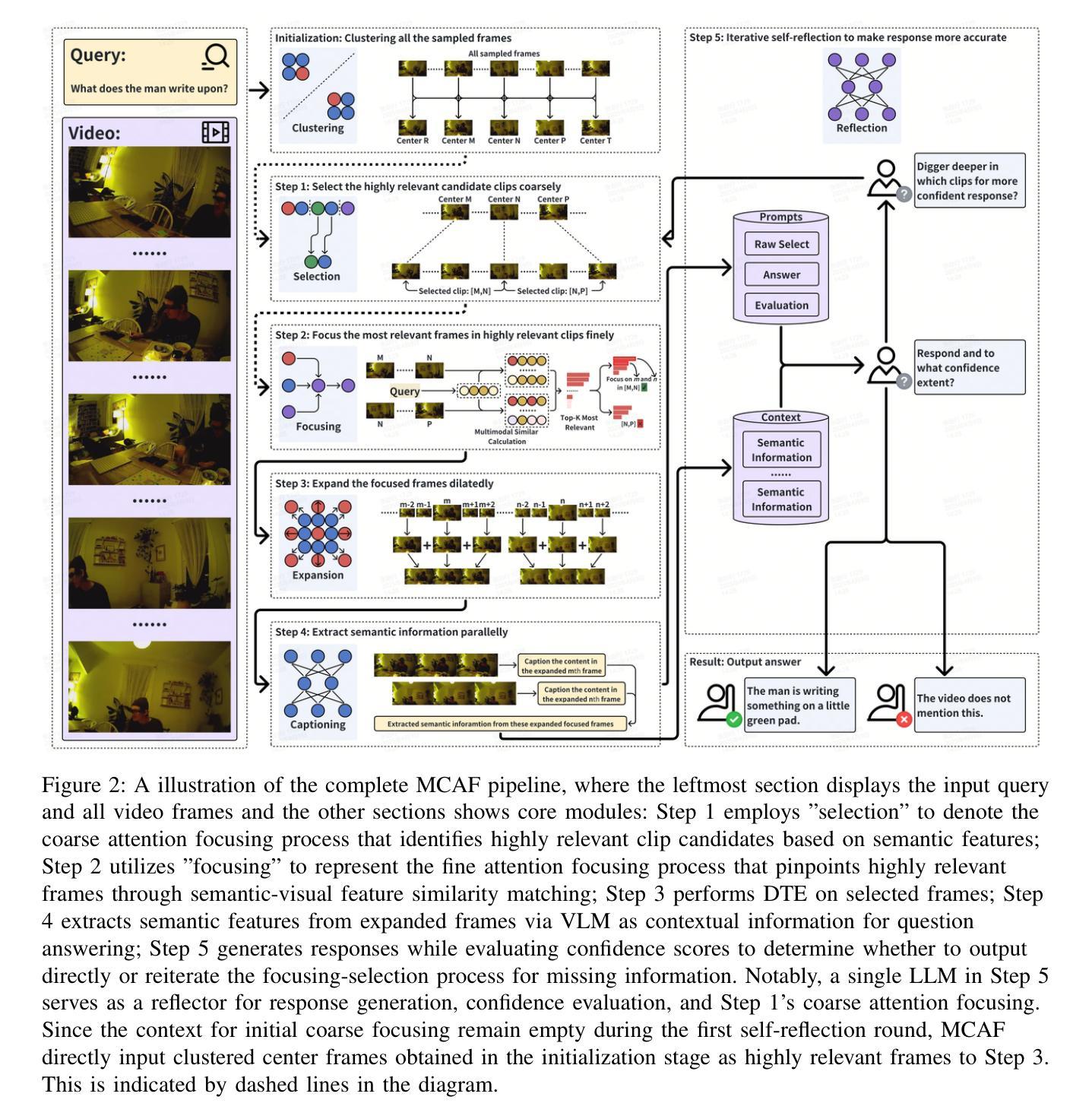
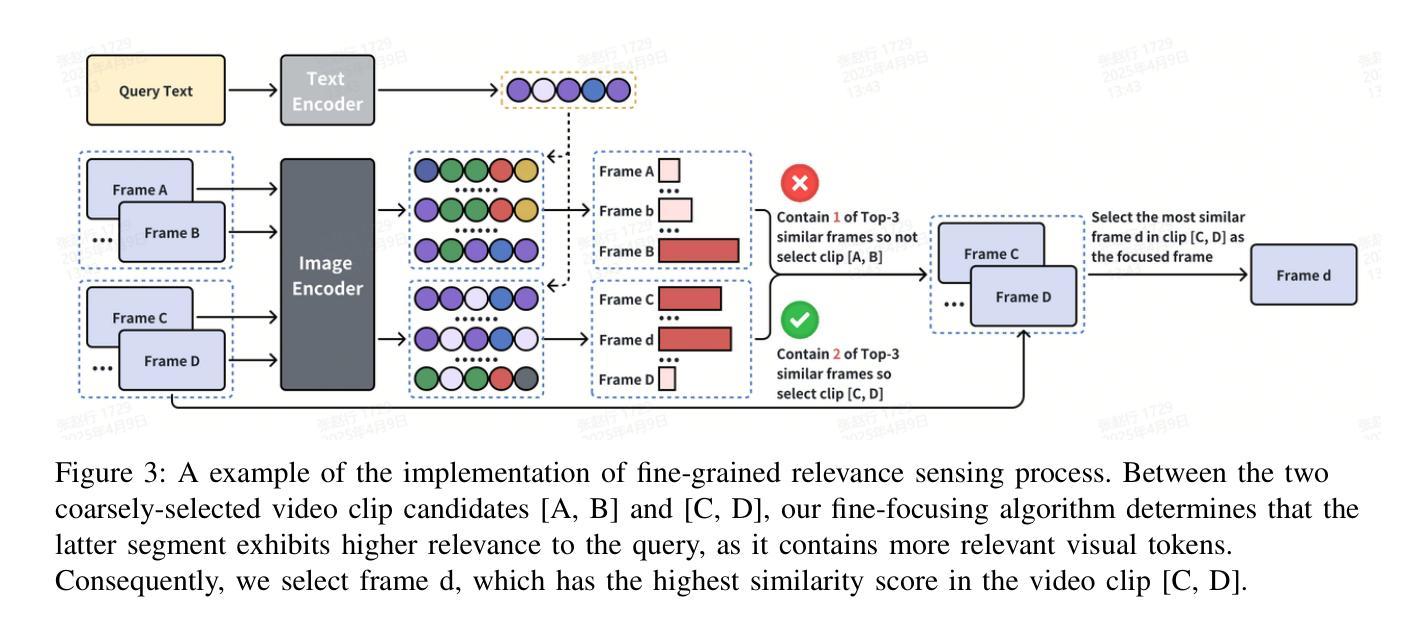
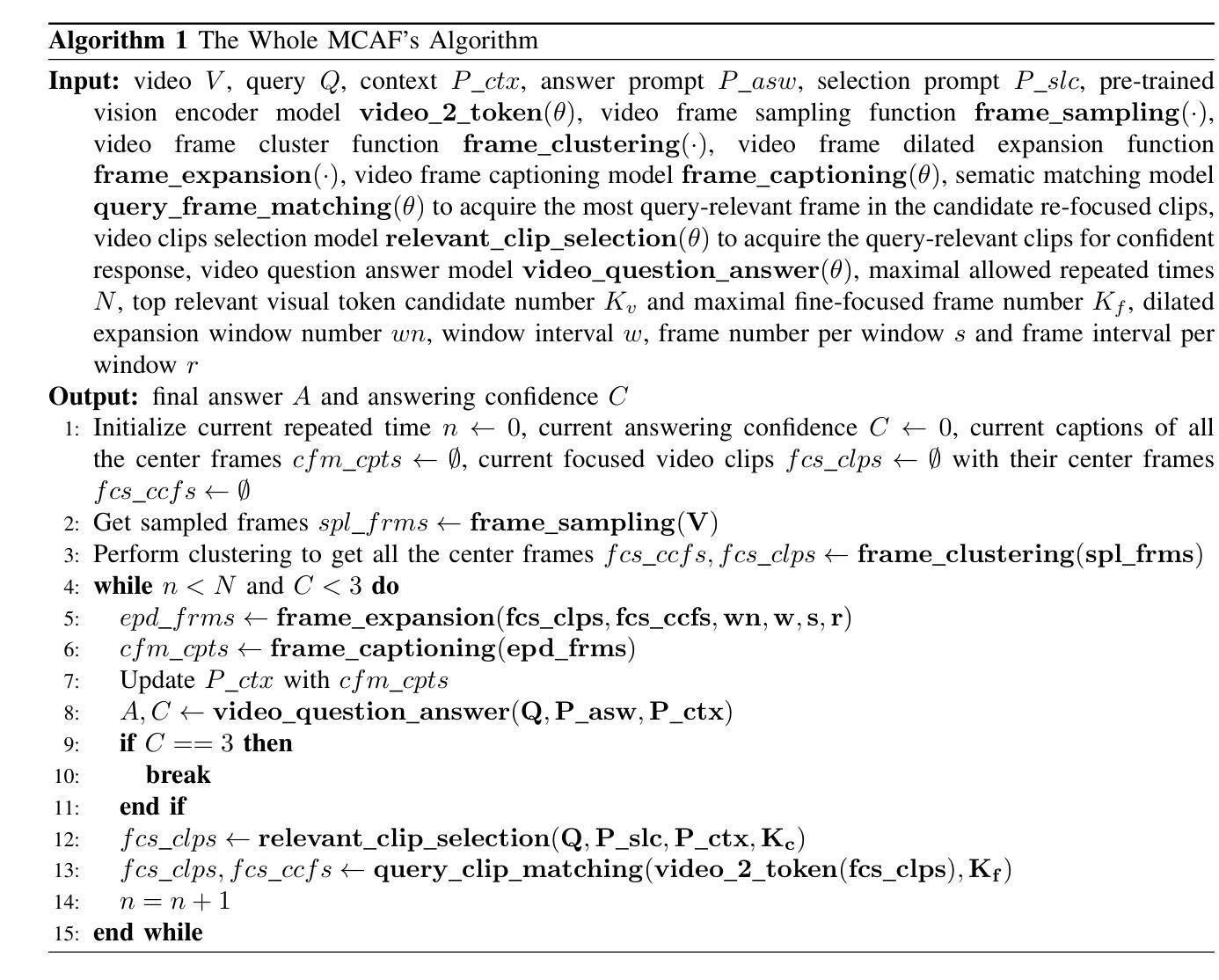
A RAG-Based Multi-Agent LLM System for Natural Hazard Resilience and Adaptation
Authors:Yangxinyu Xie, Bowen Jiang, Tanwi Mallick, Joshua David Bergerson, John K. Hutchison, Duane R. Verner, Jordan Branham, M. Ross Alexander, Robert B. Ross, Yan Feng, Leslie-Anne Levy, Weijie Su, Camillo J. Taylor
Large language models (LLMs) are a transformational capability at the frontier of artificial intelligence and machine learning that can support decision-makers in addressing pressing societal challenges such as extreme natural hazard events. As generalized models, LLMs often struggle to provide context-specific information, particularly in areas requiring specialized knowledge. In this work we propose a retrieval-augmented generation (RAG)-based multi-agent LLM system to support analysis and decision-making in the context of natural hazards and extreme weather events. As a proof of concept, we present WildfireGPT, a specialized system focused on wildfire hazards. The architecture employs a user-centered, multi-agent design to deliver tailored risk insights across diverse stakeholder groups. By integrating natural hazard and extreme weather projection data, observational datasets, and scientific literature through an RAG framework, the system ensures both the accuracy and contextual relevance of the information it provides. Evaluation across ten expert-led case studies demonstrates that WildfireGPT significantly outperforms existing LLM-based solutions for decision support.
大型语言模型(LLM)是人工智能和机器学习领域的前沿变革性技术,能够支持决策者应对极端自然灾害等紧迫的社会挑战。作为通用模型,LLM在提供特定上下文信息方面往往存在困难,特别是在需要专业知识领域。在这项工作中,我们提出了一种基于检索增强生成(RAG)的多智能体LLM系统,以支持自然灾害和极端天气事件背景下的分析和决策。作为概念验证,我们推出了专注于野火危害的WildfireGPT系统。该系统采用以用户为中心的多智能体设计,为不同的利益相关方提供量身定制的风险见解。通过整合自然灾害和极端天气预测数据、观测数据集和科学文献的RAG框架,该系统确保其提供的信息既准确又具有上下文相关性。在十个专家主导的案例研究中的评估表明,WildfireGPT在决策支持方面显著优于现有的LLM解决方案。
论文及项目相关链接
Summary
大型语言模型(LLM)在人工智能和机器学习领域具有变革性能力,能支持决策者应对极端自然灾害等社会挑战。然而,LLM作为通用模型,在需要专业知识领域的上下文信息提供方面存在困难。本研究提出了一种基于检索增强生成(RAG)的多智能体LLM系统,用于支持自然灾害和极端天气事件的分析和决策。以野火危害为重点的专用系统WildfireGPT作为概念验证被提出。该系统采用以用户为中心的多智能体设计,为不同利益相关方提供量身定制的风险洞察。通过整合自然灾害和极端天气预测数据、观测数据集和科学文献的RAG框架,确保所提供信息的准确性和上下文相关性。专家进行的十项案例研究表明,WildfireGPT在决策支持方面显著优于现有LLM解决方案。
Key Takeaways
- 大型语言模型(LLM)在人工智能和机器学习领域具有重要的作用,尤其在应对社会挑战如极端自然灾害方面。
- LLM作为通用模型在提供上下文特定信息方面存在局限性,尤其在需要专业知识领域。
- 提出了一种基于检索增强生成(RAG)的多智能体LLM系统,以改进LLM在特定领域内的表现。
- WildfireGPT是一个专注于野火危害的专用系统,作为该概念验证的实例被展示。
- WildfireGPT采用多智能体设计,以用户为中心,为不同利益相关方提供量身定制的风险洞察。
- 该系统通过整合各种数据和信息源,包括自然灾害和极端天气预测数据、观测数据集和科学文献,确保提供信息的准确性和上下文相关性。
点此查看论文截图
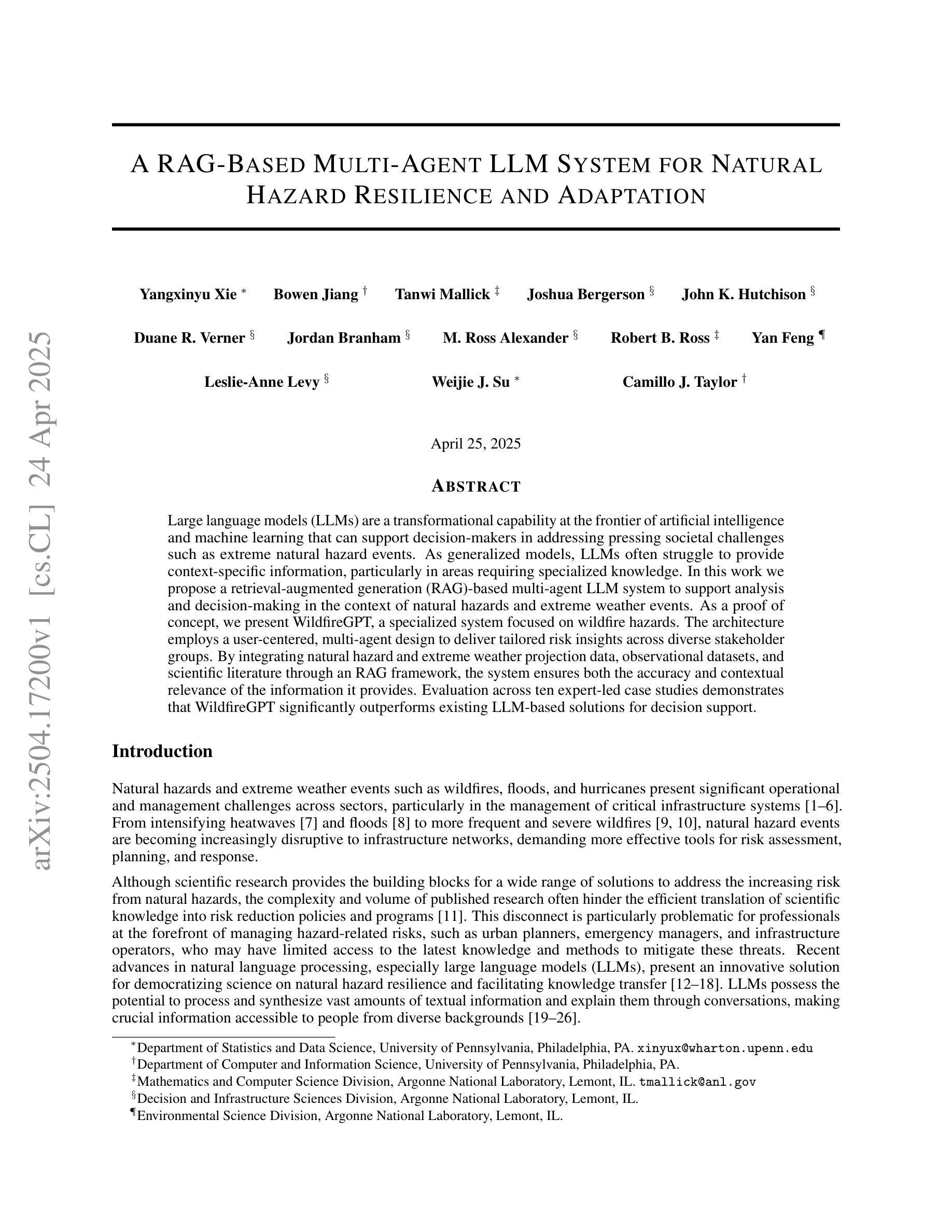
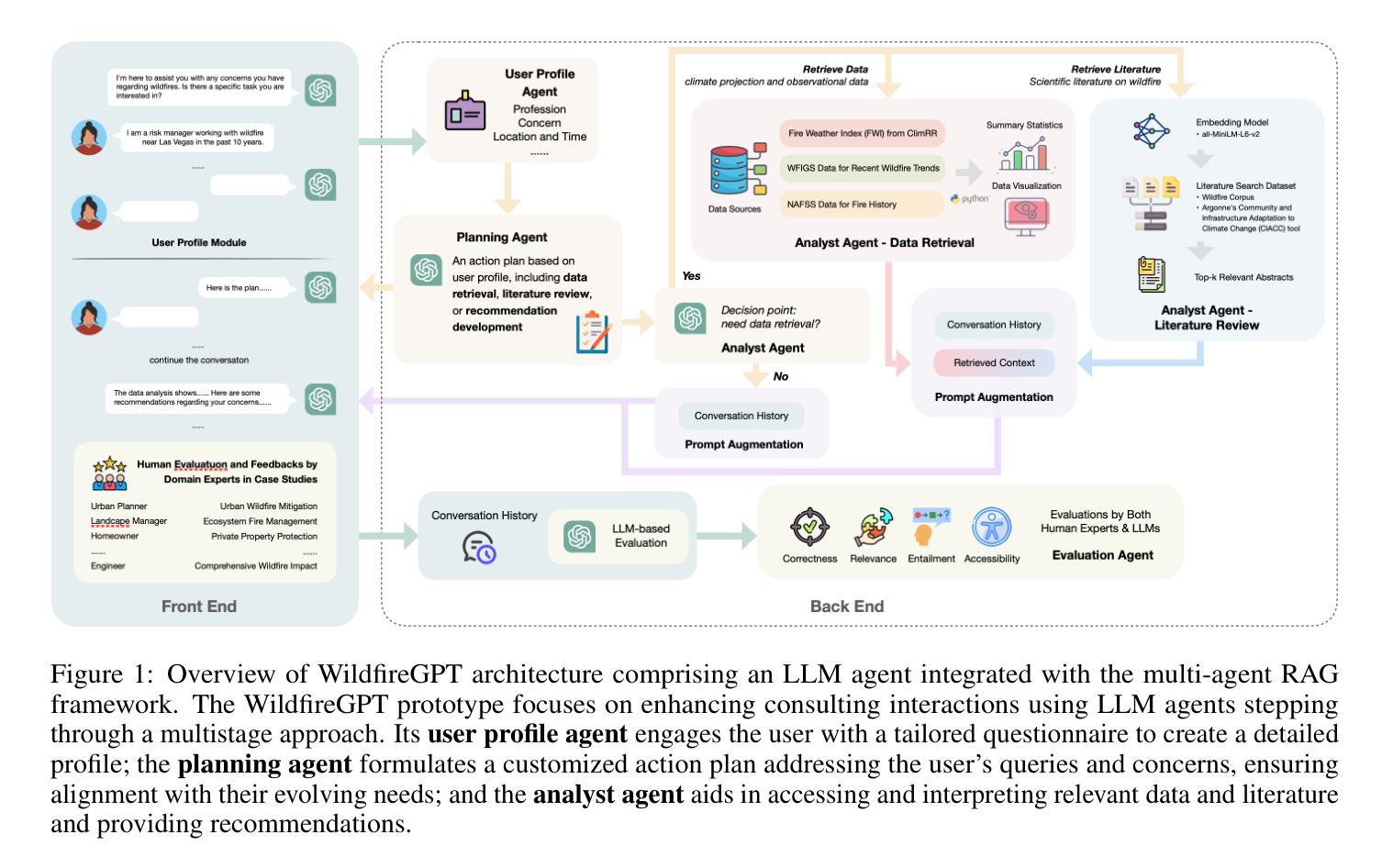
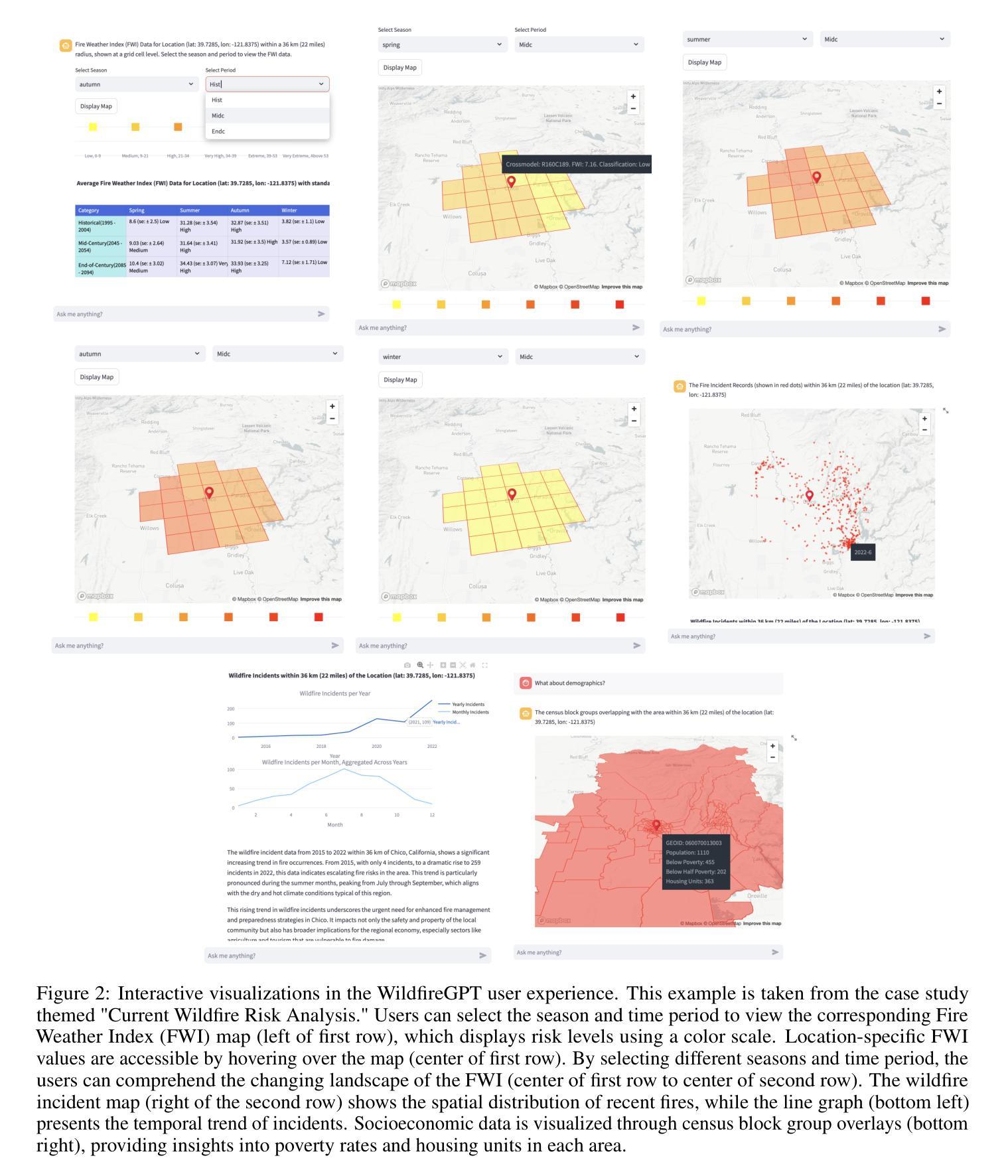
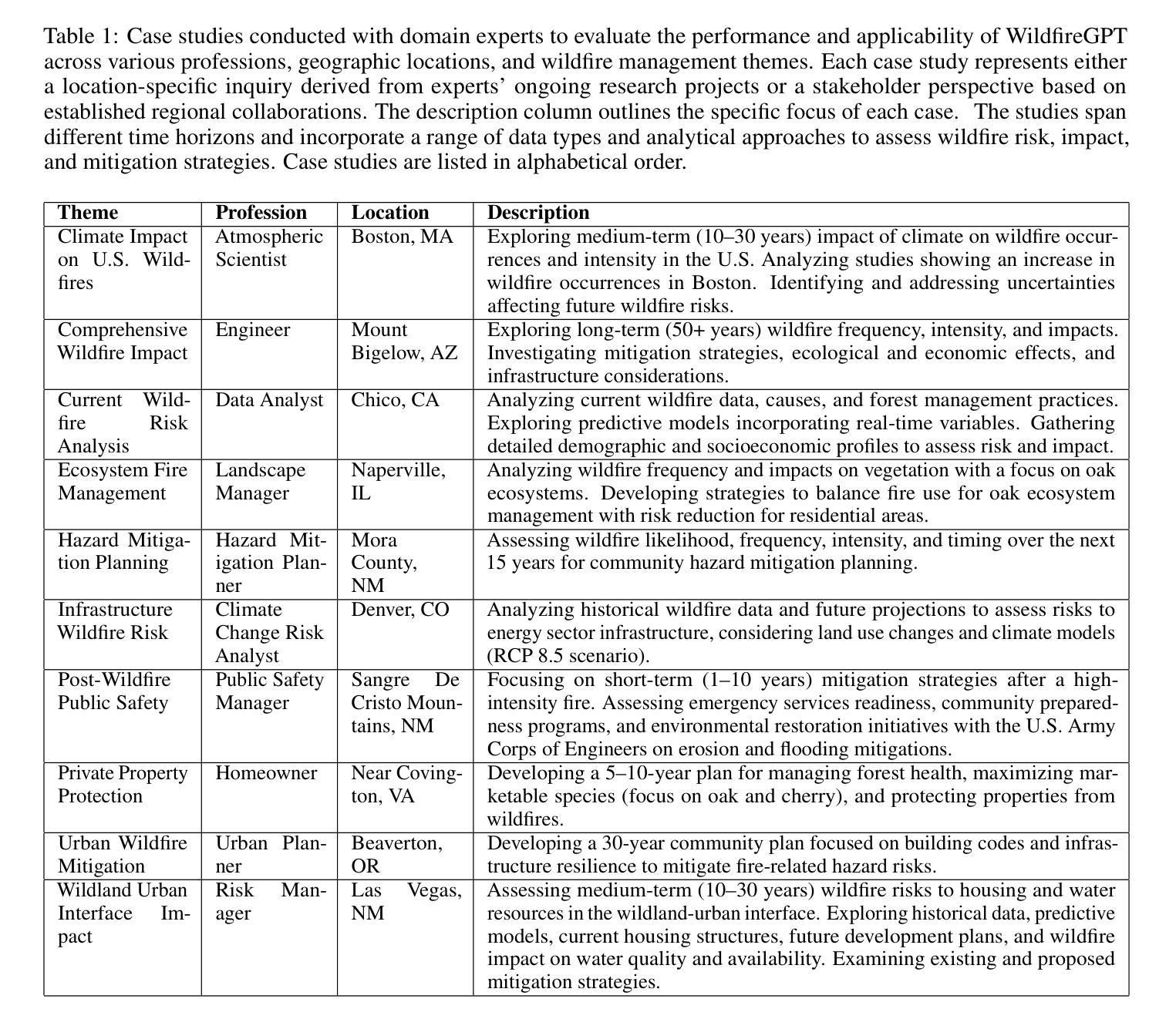
MARFT: Multi-Agent Reinforcement Fine-Tuning
Authors:Junwei Liao, Muning Wen, Jun Wang, Weinan Zhang
LLM-based Multi-Agent Systems have demonstrated remarkable capabilities in addressing complex, agentic tasks requiring multifaceted reasoning and collaboration, from generating high-quality presentation slides to conducting sophisticated scientific research. Meanwhile, RL has been widely recognized for its effectiveness in enhancing agent intelligence, but limited research has investigated the fine-tuning of LaMAS using foundational RL techniques. Moreover, the direct application of MARL methodologies to LaMAS introduces significant challenges, stemming from the unique characteristics and mechanisms inherent to LaMAS. To address these challenges, this article presents a comprehensive study of LLM-based MARL and proposes a novel paradigm termed Multi-Agent Reinforcement Fine-Tuning (MARFT). We introduce a universal algorithmic framework tailored for LaMAS, outlining the conceptual foundations, key distinctions, and practical implementation strategies. We begin by reviewing the evolution from RL to Reinforcement Fine-Tuning, setting the stage for a parallel analysis in the multi-agent domain. In the context of LaMAS, we elucidate critical differences between MARL and MARFT. These differences motivate a transition toward a novel, LaMAS-oriented formulation of RFT. Central to this work is the presentation of a robust and scalable MARFT framework. We detail the core algorithm and provide a complete, open-source implementation to facilitate adoption and further research. The latter sections of the paper explore real-world application perspectives and opening challenges in MARFT. By bridging theoretical underpinnings with practical methodologies, this work aims to serve as a roadmap for researchers seeking to advance MARFT toward resilient and adaptive solutions in agentic systems. Our implementation of the proposed framework is publicly available at: https://github.com/jwliao-ai/MARFT.
基于LLM的多智能体系统已经在处理复杂的、需要多方面推理和协作的智能任务方面表现出卓越的能力,无论是生成高质量的演示幻灯片还是进行先进的科学研究。与此同时,强化学习已被广泛认可为增强智能体智力的有效方法,但关于使用基础强化学习技术对大型多智能体系统(LaMAS)进行精细调整的研究却有限。此外,将多智能体强化学习(MARL)方法直接应用于LaMAS引入了重大挑战,这些挑战源于LaMAS所固有的独特特征和机制。为了解决这些挑战,本文全面研究了基于LLM的MARL,并提出了一种名为多智能体强化精细调整(MARFT)的新范式。我们针对LaMAS引入了一个通用的算法框架,概述了概念基础、关键区别和实用实施策略。我们首先回顾了从强化学习到强化精细调整(Reinforcement Fine-Tuning)的演变,为在智能体领域进行平行分析奠定了基础。在LaMAS的背景下,我们阐明了MARL和MARFT之间的关键区别。这些区别促使我们朝着面向LaMAS的新型RFT公式发展。本文的核心是提出一个稳健且可扩展的MARFT框架。我们详细介绍了核心算法,并提供了一个完整的开源实现,以促进采用和进一步研究。本文的后面部分探讨了MARFT在现实世界的实际应用前景和面临的挑战。通过弥合理论支持与实用方法之间的鸿沟,这项工作旨在为那些寻求在智能体系统中推进MARFT以产生具有弹性和适应性的解决方案的研究人员提供路线图。我们提出的框架实现公开可用在:https://github.com/jwliao-ai/MARFT。
论文及项目相关链接
PDF 36 pages
Summary
大型语言模型(LLM)为基础的多智能体系统(LaMAS)在解决复杂的、多智能体任务方面表现出卓越的能力,如生成高质量演示幻灯片及开展高级科学研究等。强化学习(RL)在提升智能体智能方面得到广泛认可,但使用基础RL技术微调LaMAS的研究有限。直接应用多智能体强化学习(MARL)方法到LaMAS中引入了巨大挑战。为应对这些挑战,本文全面研究了基于LLM的MARL,并提出了一种新的范式——多智能体强化精细调整(MARFT)。介绍了一种为LaMAS量身定制的通用算法框架,阐述了其概念基础、关键区别和实用实施策略。本文还介绍了从RL到强化精细调整(RFT)的演变,对多智能体领域的并行分析进行设置。在LaMAS的语境下,阐述了MARL和MARFT之间的关键区别,推动了面向LaMAS的新型RFT公式的出现。中心工作是提出一个稳健且可扩展的MARFT框架,并提供了开源实现以促进采用和进一步研究。
Key Takeaways
- LLM-based Multi-Agent Systems展现出在复杂、多智能体任务方面的出色能力。
- RL在提升智能体智能方面有效,但LLM为基础的多智能体系统(LaMAS)的微调研究有限。
- 直接应用MARL方法到LaMAS引入挑战,需新的方法来解决。
- 提出了一种新的范式——多智能体强化精细调整(MARFT)。
- 介绍了为LaMAS量身定制的通用算法框架,包括概念基础、关键区别和实施策略。
- 从RL到强化精细调整(RFT)的演变得到阐述,为研究者提供新的研究方向。
点此查看论文截图
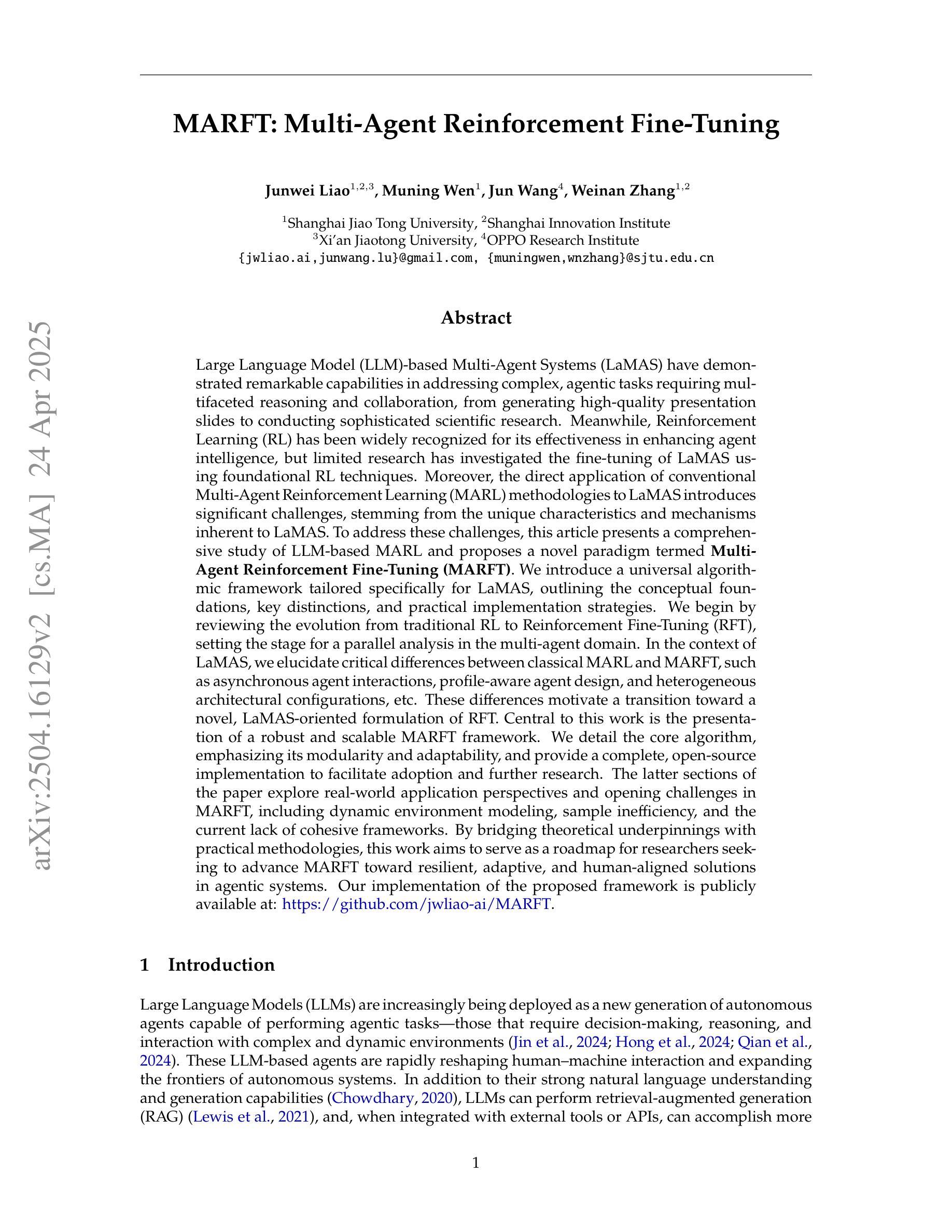
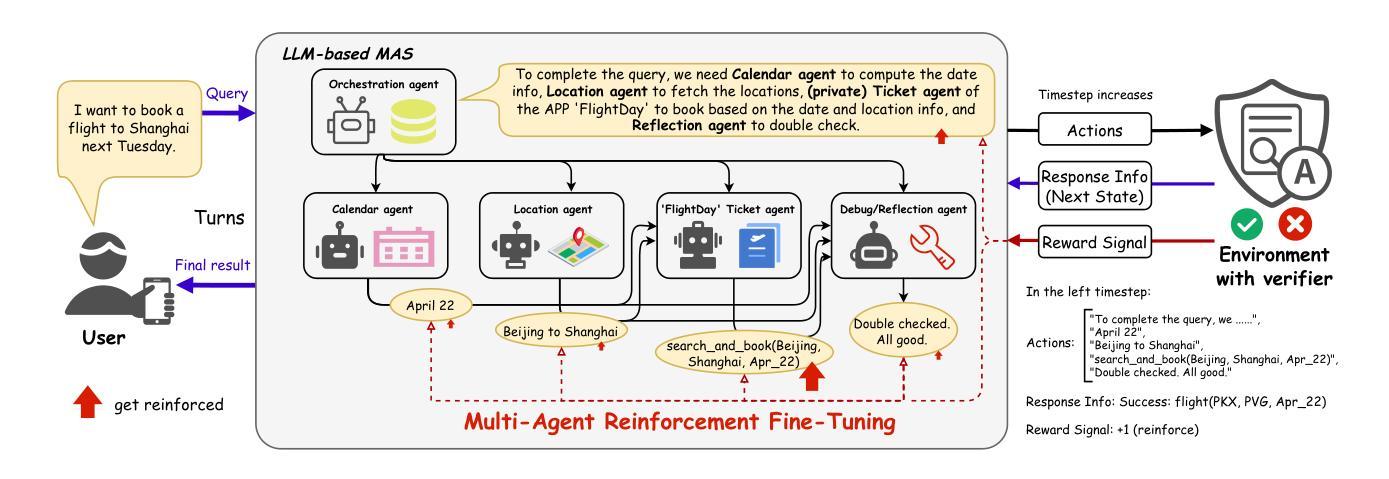
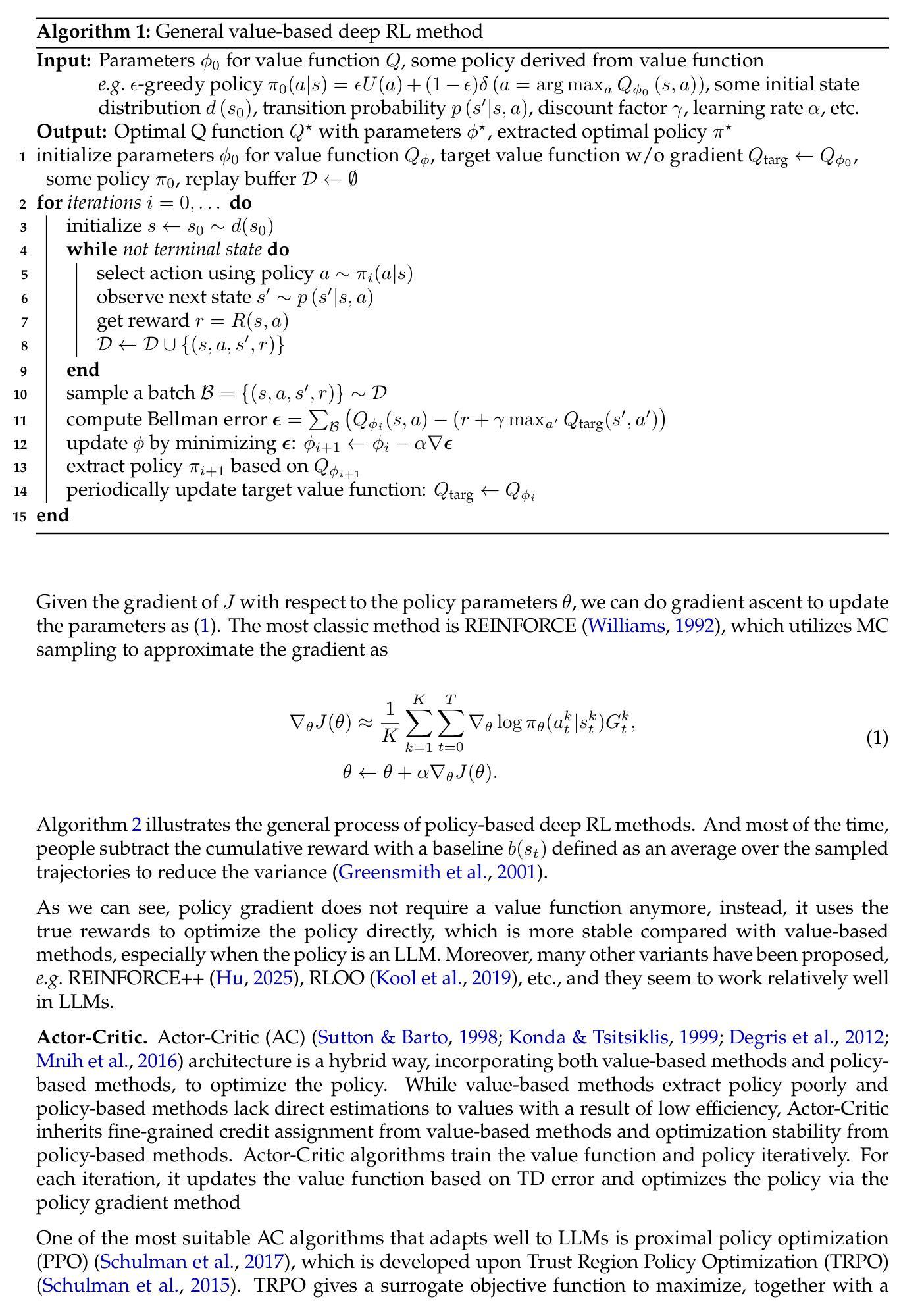
CheatAgent: Attacking LLM-Empowered Recommender Systems via LLM Agent
Authors:Liang-bo Ning, Shijie Wang, Wenqi Fan, Qing Li, Xin Xu, Hao Chen, Feiran Huang
Recently, Large Language Model (LLM)-empowered recommender systems (RecSys) have brought significant advances in personalized user experience and have attracted considerable attention. Despite the impressive progress, the research question regarding the safety vulnerability of LLM-empowered RecSys still remains largely under-investigated. Given the security and privacy concerns, it is more practical to focus on attacking the black-box RecSys, where attackers can only observe the system’s inputs and outputs. However, traditional attack approaches employing reinforcement learning (RL) agents are not effective for attacking LLM-empowered RecSys due to the limited capabilities in processing complex textual inputs, planning, and reasoning. On the other hand, LLMs provide unprecedented opportunities to serve as attack agents to attack RecSys because of their impressive capability in simulating human-like decision-making processes. Therefore, in this paper, we propose a novel attack framework called CheatAgent by harnessing the human-like capabilities of LLMs, where an LLM-based agent is developed to attack LLM-Empowered RecSys. Specifically, our method first identifies the insertion position for maximum impact with minimal input modification. After that, the LLM agent is designed to generate adversarial perturbations to insert at target positions. To further improve the quality of generated perturbations, we utilize the prompt tuning technique to improve attacking strategies via feedback from the victim RecSys iteratively. Extensive experiments across three real-world datasets demonstrate the effectiveness of our proposed attacking method.
最近,大型语言模型(LLM)赋能的推荐系统(RecSys)在个性化用户体验方面取得了显著进展,并引起了广泛关注。尽管取得了令人印象深刻的进展,但关于LLM赋能的RecSys的安全漏洞的研究问题仍然很大程度上未被探究。考虑到安全和隐私的担忧,更实际的是关注攻击黑盒RecSys,攻击者只能观察系统的输入和输出。然而,传统的采用强化学习(RL)代理的攻击方法由于处理复杂文本输入、规划和推理的能力有限,因此攻击LLM赋能的RecSys并不有效。另一方面,LLM在模拟人类决策过程方面表现出强大的能力,因此为攻击RecSys的代理提供了前所未有的机会。因此,本文提出了一个名为CheatAgent的新型攻击框架,该框架利用LLM的人类化能力,开发了一个基于LLM的代理来攻击LLM赋能的RecSys。具体来说,我们的方法首先确定插入位置,以最小的输入修改实现最大影响。之后,LLM代理被设计成在目标位置插入对抗性扰动。为了进一步提高生成扰动的质量,我们利用提示调整技术,通过来自受害者RecSys的反馈来改进攻击策略。在三个真实数据集上的广泛实验证明了我们提出的攻击方法的有效性。
论文及项目相关链接
PDF Accepted by KDD 2024;
总结
LLM赋能的推荐系统虽然在个性化用户体验上取得了显著进展,但其安全漏洞的研究仍待深入。本文提出一种名为CheatAgent的新型攻击框架,利用LLM的人化能力攻击LLM赋能的推荐系统。通过识别插入位置并生成对抗性扰动,实验证明该方法的有效性。
关键见解
- 大型语言模型(LLM)赋能的推荐系统(RecSys)在个性化用户体验方面有显著进步,但安全漏洞研究仍待深入。
- 针对LLM赋能的RecSys的安全攻击更实际地集中在黑盒RecSys上,攻击者只能观察系统的输入和输出。
- 传统采用强化学习(RL)代理的攻击方法因处理复杂文本输入、规划和推理能力有限,对LLM赋能的RecSys效果不佳。
- LLM因其模拟人类决策过程的卓越能力,为攻击RecSys提供了前所未有的机会。
- 本文提出的新型攻击框架CheatAgent,利用LLM的人化能力来攻击LLM赋能的RecSys,先识别插入位置再生成对抗性扰动。
- 通过利用提示调整技术,CheatAgent能够通过来自受害者RecSys的反馈来改进攻击策略。
点此查看论文截图
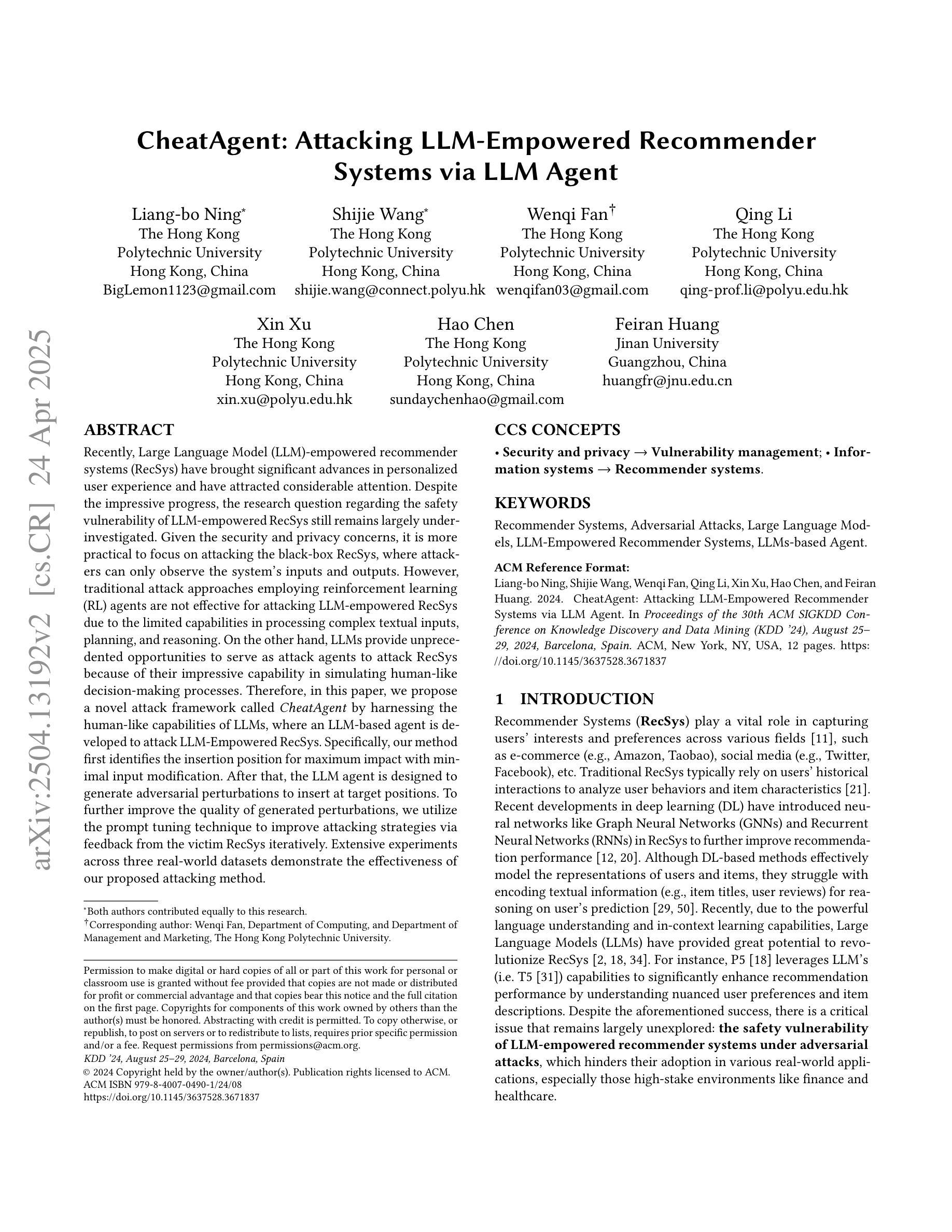
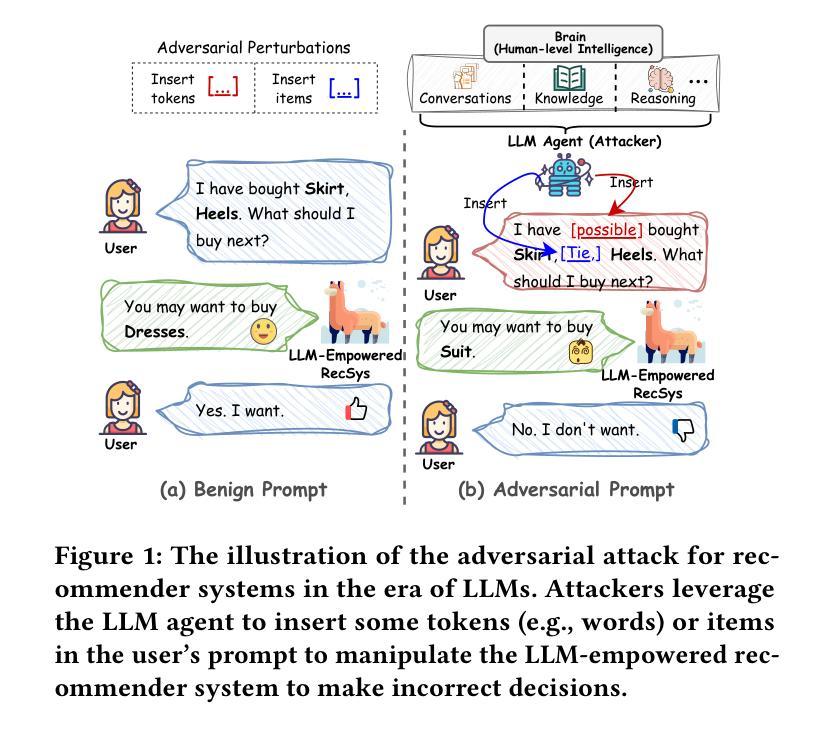
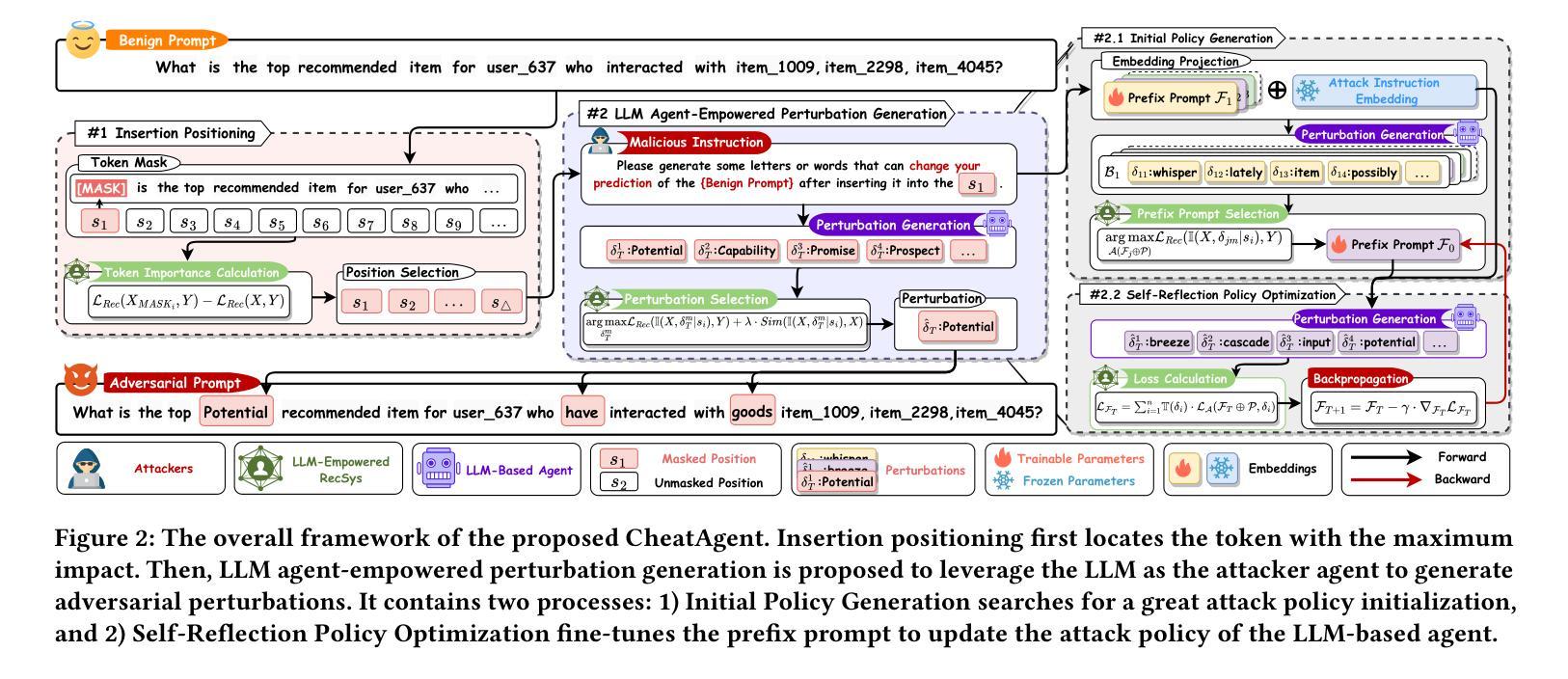
SWE-PolyBench: A multi-language benchmark for repository level evaluation of coding agents
Authors:Muhammad Shihab Rashid, Christian Bock, Yuan Zhuang, Alexander Buchholz, Tim Esler, Simon Valentin, Luca Franceschi, Martin Wistuba, Prabhu Teja Sivaprasad, Woo Jung Kim, Anoop Deoras, Giovanni Zappella, Laurent Callot
Coding agents powered by large language models have shown impressive capabilities in software engineering tasks, but evaluating their performance across diverse programming languages and real-world scenarios remains challenging. We introduce SWE-PolyBench, a new multi-language benchmark for repository-level, execution-based evaluation of coding agents. SWE-PolyBench contains 2110 instances from 21 repositories and includes tasks in Java (165), JavaScript (1017), TypeScript (729) and Python (199), covering bug fixes, feature additions, and code refactoring. We provide a task and repository-stratified subsample (SWE-PolyBench500) and release an evaluation harness allowing for fully automated evaluation. To enable a more comprehensive comparison of coding agents, this work also presents a novel set of metrics rooted in syntax tree analysis. We evaluate leading open source coding agents on SWE-PolyBench, revealing their strengths and limitations across languages, task types, and complexity classes. Our experiments show that current agents exhibit uneven performances across languages and struggle with complex problems while showing higher performance on simpler tasks. SWE-PolyBench aims to drive progress in developing more versatile and robust AI coding assistants for real-world software engineering. Our datasets and code are available at: https://github.com/amazon-science/SWE-PolyBench
由大型语言模型驱动的编码代理在软件工程任务中表现出了令人印象深刻的能力,但在多种编程语言和现实场景中对它们的性能进行评估仍然具有挑战性。我们引入了SWE-PolyBench,这是一个新的多语言基准测试,用于对编码代理进行存储库级别的基于执行的评价。SWE-PolyBench包含来自21个存储库的2110个实例,涵盖了Java(165个)、JavaScript(1017个)、TypeScript(729个)和Python(199个)的任务,包括错误修复、功能添加和代码重构。我们提供了一个任务和存储库分层子样本(SWE-PolyBench500),并发布了一个评估工具,可以实现全自动评估。为了能够对编码代理进行更全面的比较,这项工作还提出了基于语法树分析的新指标集。我们在SWE-PolyBench上评估了领先的开源编码代理,揭示了它们在语言、任务类型和复杂类方面的优势和局限性。我们的实验表明,当前代理在不同语言之间的性能表现不均衡,对复杂问题感到困扰,而在简单任务上表现较好。SWE-PolyBench的目标是推动开发更通用和更稳健的AI编码助手,用于现实世界的软件工程。我们的数据集和代码可在:https://github.com/amazon-science/SWE-PolyBench上找到。
论文及项目相关链接
PDF 20 pages, 6 figures, corrected author name spelling
Summary
大型语言模型驱动的编码代理在软件工程任务中展现出令人印象深刻的能力,但跨多种编程语言和现实场景的性能评估仍然具有挑战性。为此,我们推出了SWE-PolyBench,这是一个新的多语言基准测试,用于对编码代理进行仓库级的执行评估。SWE-PolyBench包含来自21个仓库的2110个实例,涵盖Java、JavaScript、TypeScript和Python中的任务,包括错误修复、功能添加和代码重构。我们还提供了一个任务和仓库分层子样本(SWE-PolyBench500),并发布了一个评估工具,可以进行全自动评估。为了更全面地比较编码代理,本文还提出了基于语法树分析的新指标集。我们在SWE-PolyBench上评估了领先的开源编码代理,揭示了它们在语言、任务类型和复杂类方面的优势和局限性。实验表明,当前代理在不同语言之间的性能不均,对复杂问题表现出挣扎,而在简单任务上表现较好。SWE-PolyBench旨在推动开发更通用和稳健的AI编程助手,用于现实世界的软件工程。
Key Takeaways
- 大型语言模型驱动的编码代理在软件工程任务中表现出强大的能力。
- 跨多种编程语言和现实场景的性能评估是当前的挑战。
- 推出了新的多语言基准测试SWE-PolyBench,用于评估编码代理。
- SWE-PolyBench包含多种语言和任务类型的实例,包括错误修复、功能添加和代码重构。
- 提供了SWE-PolyBench500子样本和全自动评估工具。
- 新指标集基于语法树分析,用于更全面的编码代理比较。
点此查看论文截图
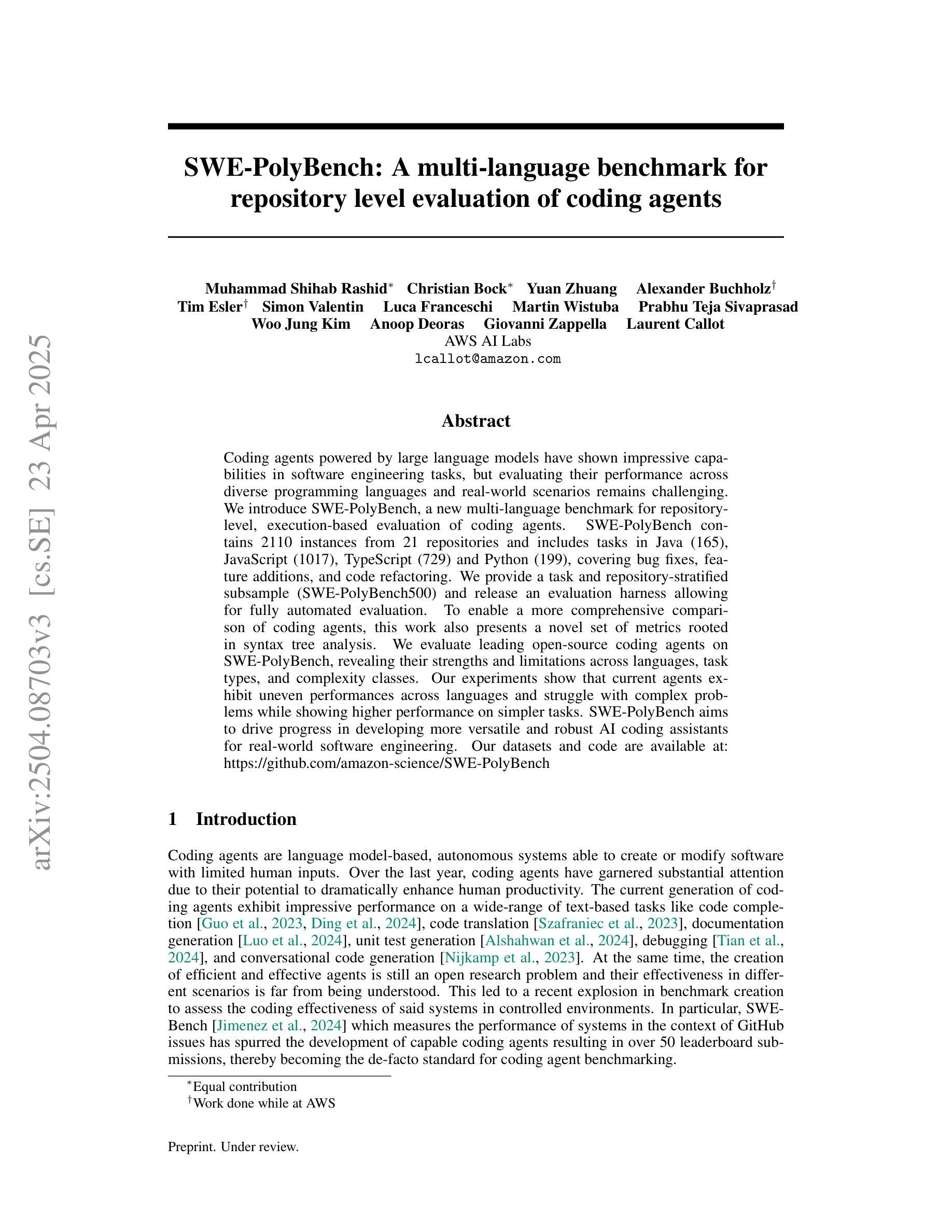
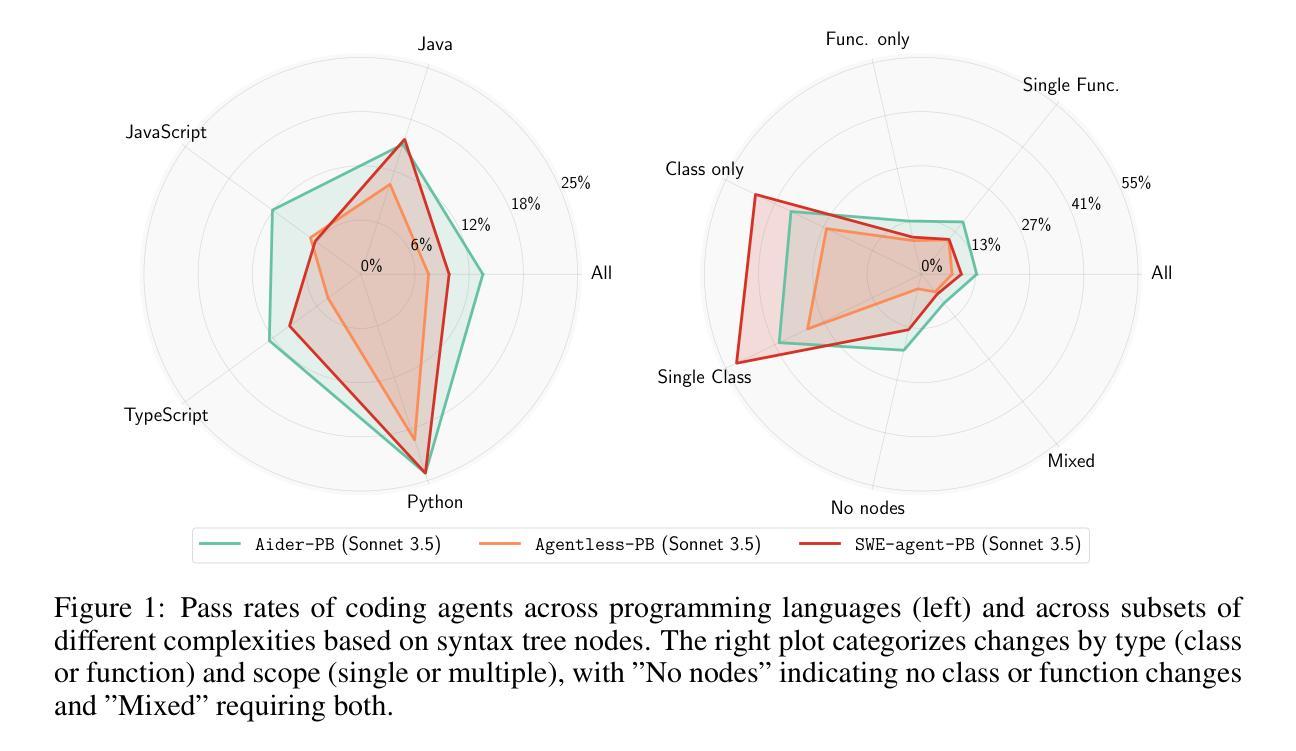
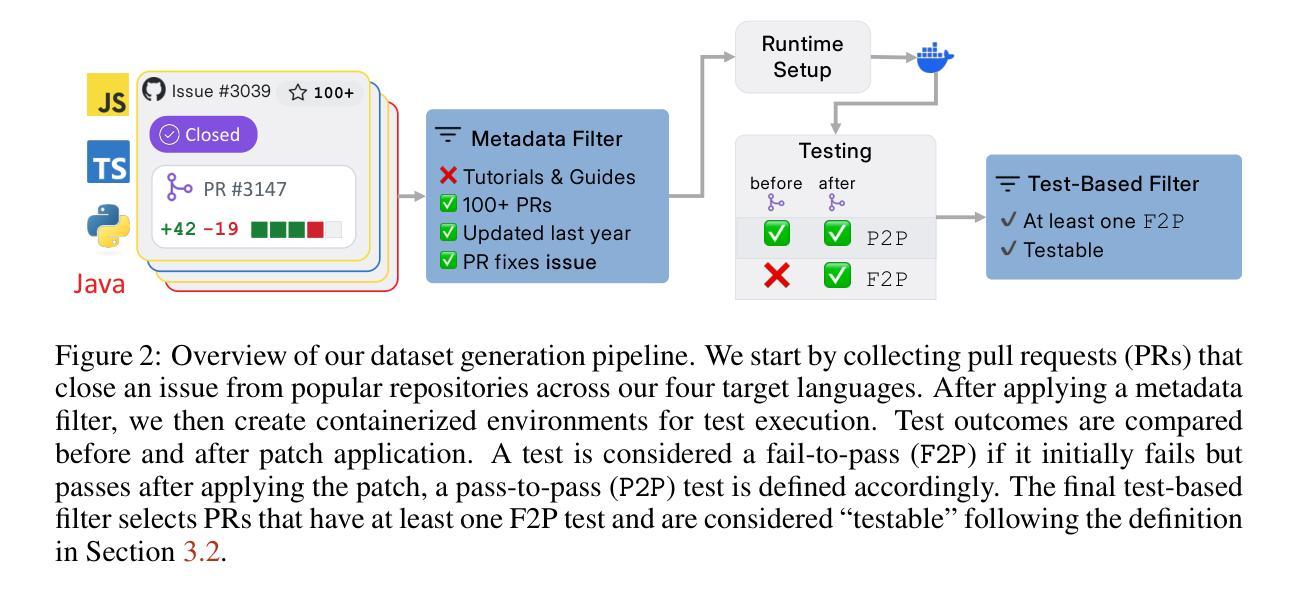
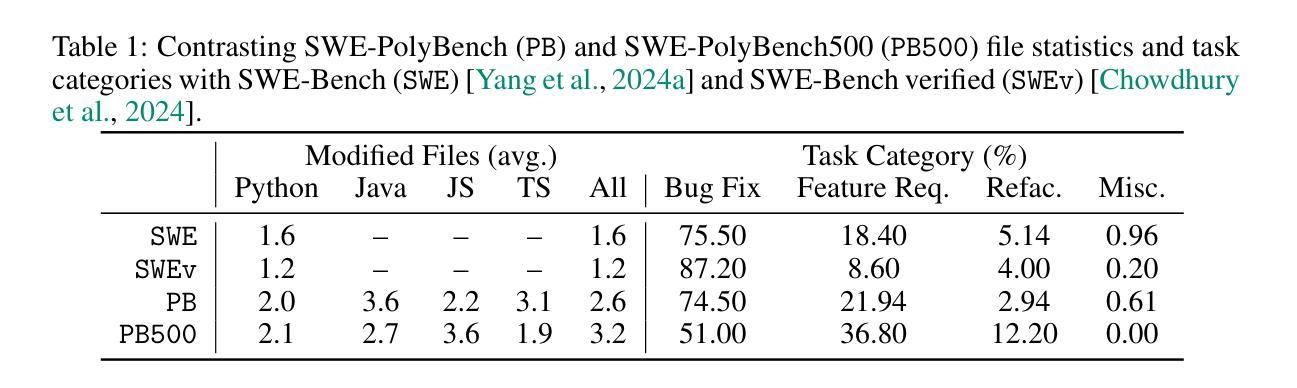
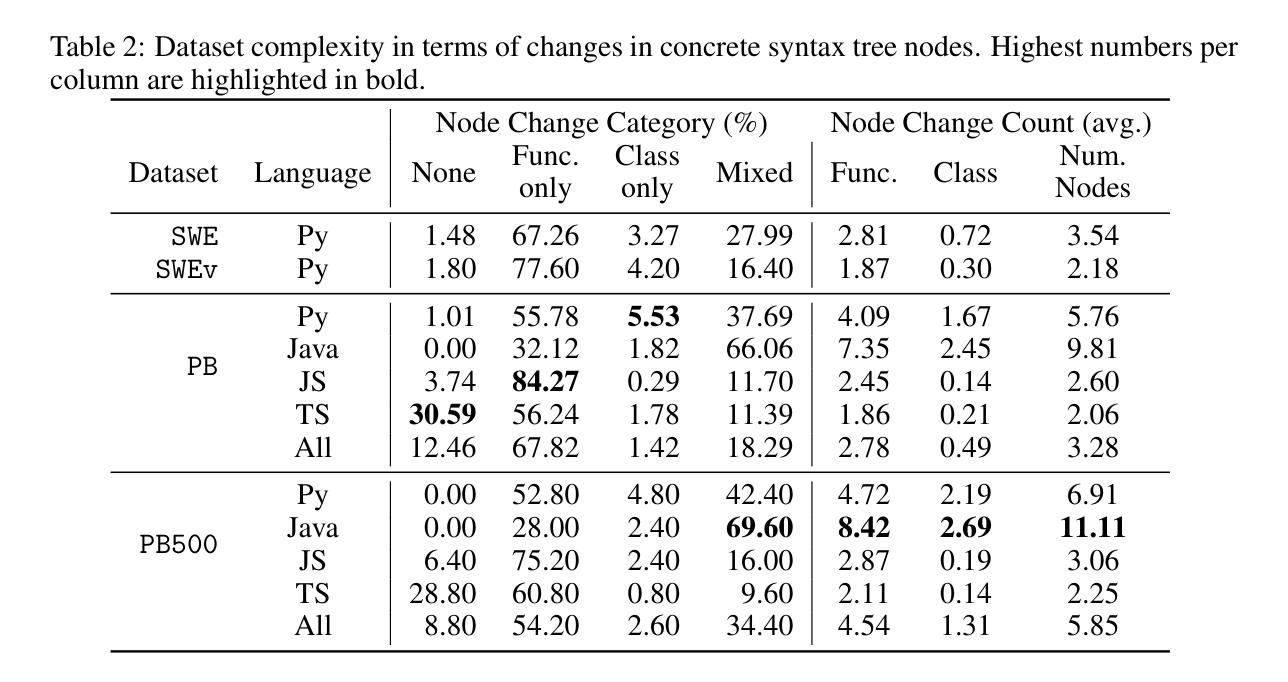