⚠️ 以下所有内容总结都来自于 大语言模型的能力,如有错误,仅供参考,谨慎使用
🔴 请注意:千万不要用于严肃的学术场景,只能用于论文阅读前的初筛!
💗 如果您觉得我们的项目对您有帮助 ChatPaperFree ,还请您给我们一些鼓励!⭐️ HuggingFace免费体验
2025-04-26 更新
Are Transformers Able to Reason by Connecting Separated Knowledge in Training Data?
Authors:Yutong Yin, Zhaoran Wang
Humans exhibit remarkable compositional reasoning by integrating knowledge from various sources. For example, if someone learns ( B = f(A) ) from one source and ( C = g(B) ) from another, they can deduce ( C=g(B)=g(f(A)) ) even without encountering ( ABC ) together, showcasing the generalization ability of human intelligence. In this paper, we introduce a synthetic learning task, “FTCT” (Fragmented at Training, Chained at Testing), to validate the potential of Transformers in replicating this skill and interpret its inner mechanism. In the training phase, data consist of separated knowledge fragments from an overall causal graph. During testing, Transformers must infer complete causal graph traces by integrating these fragments. Our findings demonstrate that few-shot Chain-of-Thought prompting enables Transformers to perform compositional reasoning on FTCT by revealing correct combinations of fragments, even if such combinations were absent in the training data. Furthermore, the emergence of compositional reasoning ability is strongly correlated with the model complexity and training-testing data similarity. We propose, both theoretically and empirically, that Transformers learn an underlying generalizable program from training, enabling effective compositional reasoning during testing.
人类能够通过整合来自不同来源的知识展现出惊人的组合推理能力。例如,如果有人从某一来源学习到(B=f(A))并从另一来源学习到(C=g(B)),即使没有同时遇到(ABC),他们也能推断出(C=g(B)=g(f(A))),展示了人类智力的泛化能力。在本文中,我们引入了一项合成学习任务“FTCT”(训练时碎片化,测试时连锁),以验证Transformer复制这项技能的潜力并解释其内在机制。在训练阶段,数据由来自整体因果图的分离知识片段组成。在测试阶段,Transformer必须通过整合这些片段来推断完整的因果图轨迹。我们的研究发现,通过揭示正确的片段组合,即使在训练数据中不存在这样的组合,少数镜头下的思维链提示也能使Transformer在FTCT上表现出组合推理能力。此外,组合推理能力的出现与模型复杂性和训练-测试数据相似性之间存在强烈的相关性。我们从理论和实证上提出,Transformer通过训练学习到了可概括的基本程序,从而在测试期间实现了有效的组合推理。
论文及项目相关链接
PDF Accepted by ICLR 2025
Summary
本文介绍了人类具备将不同来源的知识进行整合进行推理的出色能力。通过引入一种名为“FTCT”(训练时碎片化,测试时串联)的合成学习任务,验证了Transformer模型在复制这种能力方面的潜力,并解读了其内在机制。训练阶段的数据来自整体因果图的分离知识片段,测试阶段,Transformer必须将这些片段整合来推断完整的因果图轨迹。研究发现,通过少量链式思维提示(Chain-of-Thought prompting),Transformer能够在FTCT上执行组合推理,揭示正确的片段组合,即使这些组合在训练数据中不存在。此外,模型的组合推理能力与模型复杂度和训练测试数据相似性密切相关。本文提出,Transformer从训练中学习了一种通用的可概括的程序,在测试时能够进行有效的组合推理。
Key Takeaways
- 人类能够整合来自不同来源的知识进行推理,展示出强大的组合推理能力。
- “FTCT”任务被用来验证Transformer模型在组合推理方面的潜力。
- 在训练阶段,数据由整体因果图的知识片段组成;在测试阶段,Transformer需要整合这些片段来推断完整的因果图轨迹。
- 少量链式思维提示(Chain-of-Thought prompting)有助于Transformer执行组合推理任务。
- 正确的片段组合可以在训练数据中不存在的情况下被揭示。
- 模型复杂度以及训练测试数据的相似性对模型的组合推理能力有很大影响。
点此查看论文截图
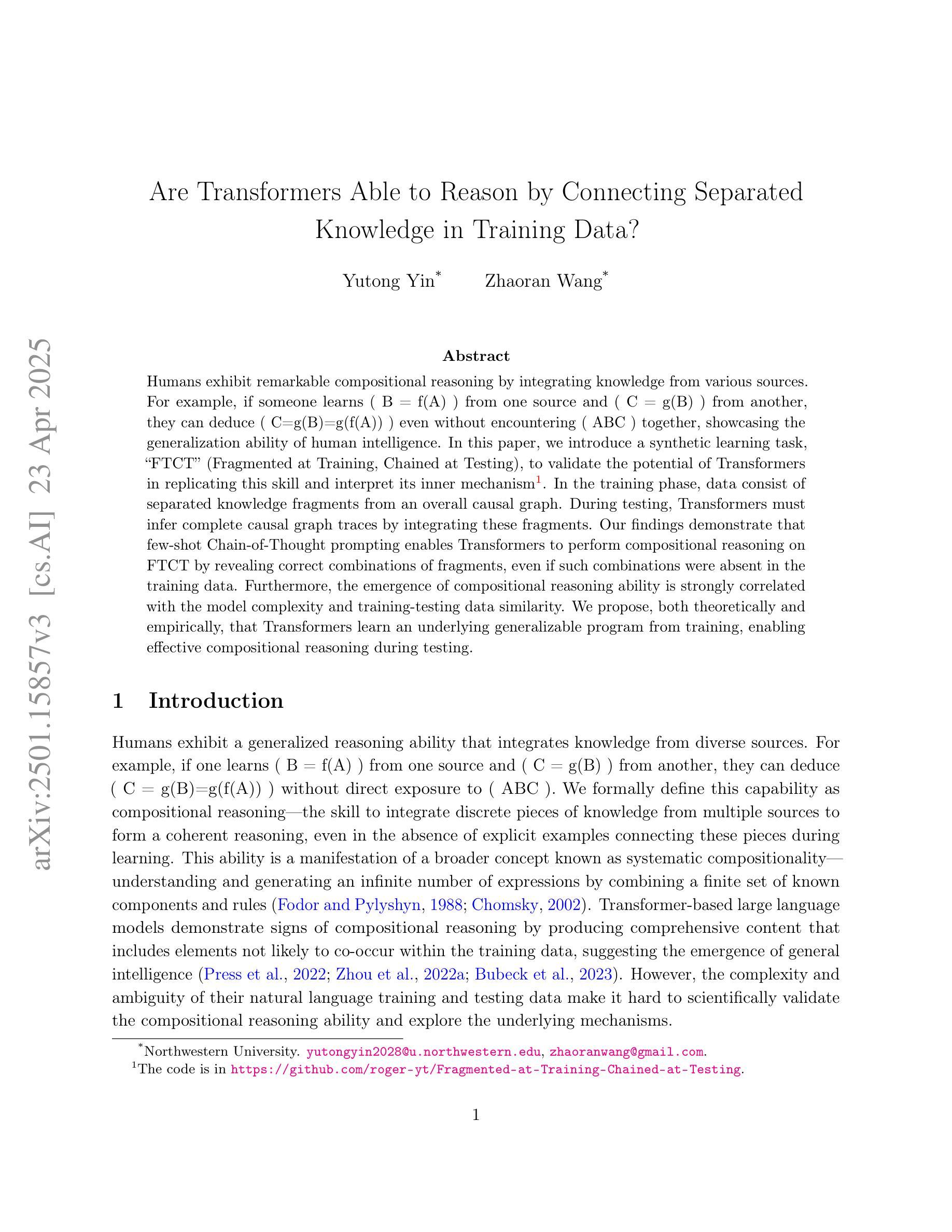
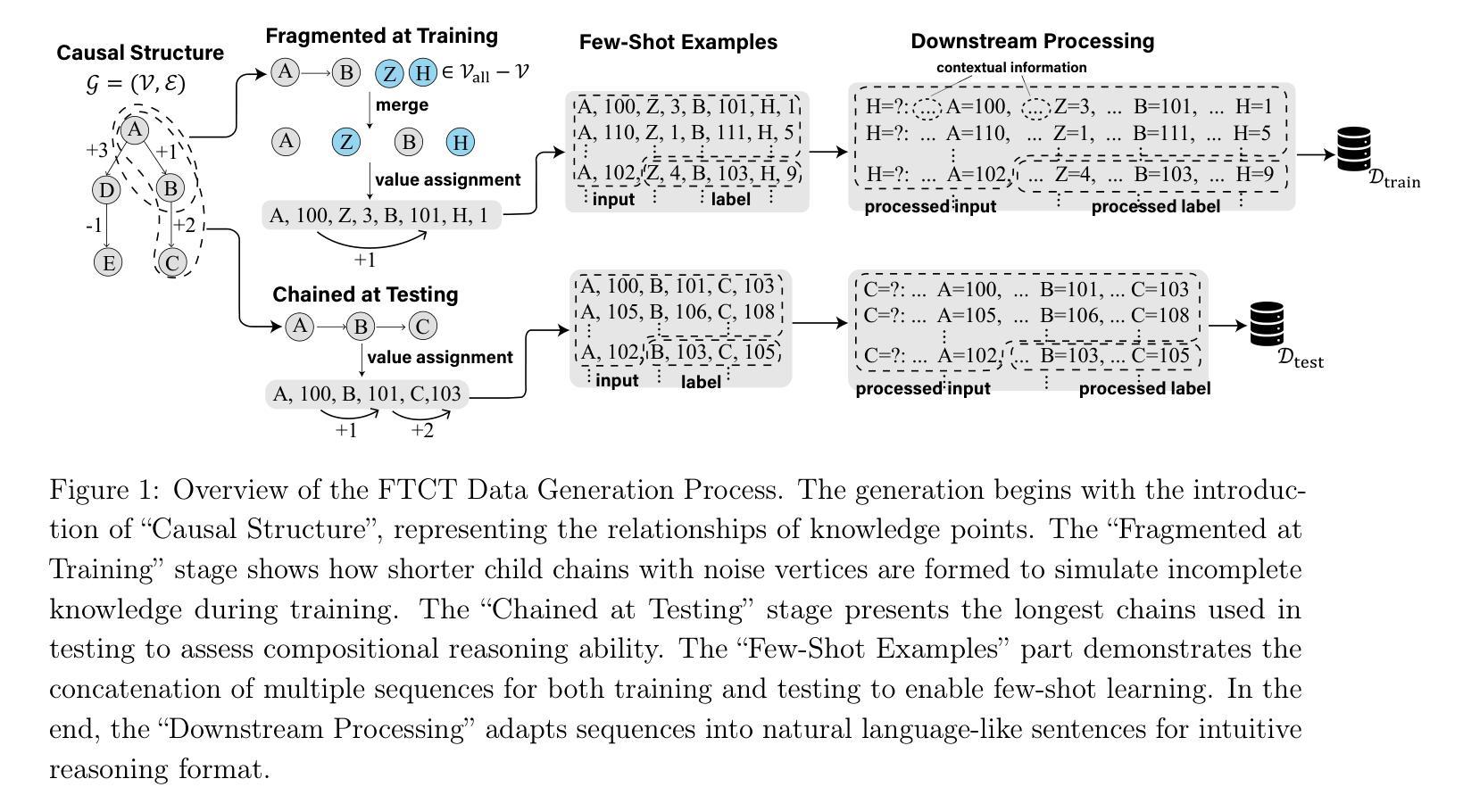
Synthetic Lyrics Detection Across Languages and Genres
Authors:Yanis Labrak, Markus Frohmann, Gabriel Meseguer-Brocal, Elena V. Epure
In recent years, the use of large language models (LLMs) to generate music content, particularly lyrics, has gained in popularity. These advances provide valuable tools for artists and enhance their creative processes, but they also raise concerns about copyright violations, consumer satisfaction, and content spamming. Previous research has explored content detection in various domains. However, no work has focused on the text modality, lyrics, in music. To address this gap, we curated a diverse dataset of real and synthetic lyrics from multiple languages, music genres, and artists. The generation pipeline was validated using both humans and automated methods. We performed a thorough evaluation of existing synthetic text detection approaches on lyrics, a previously unexplored data type. We also investigated methods to adapt the best-performing features to lyrics through unsupervised domain adaptation. Following both music and industrial constraints, we examined how well these approaches generalize across languages, scale with data availability, handle multilingual language content, and perform on novel genres in few-shot settings. Our findings show promising results that could inform policy decisions around AI-generated music and enhance transparency for users.
近年来,使用大型语言模型(LLM)生成音乐内容,特别是歌词,越来越受欢迎。这些进步为艺术家提供了有价值的工具,增强了他们的创作过程,但同时也引发了关于版权侵犯、消费者满意度和内容泛滥的担忧。之前的研究已经在各个领域探索了内容检测。然而,没有研究关注音乐中的文本模式——歌词。为了弥补这一空白,我们从多种语言、音乐流派和艺术家中精心制作了一个真实和合成歌词的多样化数据集。生成管道使用人工和自动化方法进行了验证。我们对现有合成文本检测方法在歌词上的表现进行了全面评估,这是一种以前未被探索过的数据类型。我们还研究了通过无监督域适应调整最佳性能特征的方法。遵循音乐和工业约束,我们研究了这些方法在多语言环境中的泛化能力、随着数据可用性而扩展的能力、处理多种语言内容的能力以及在少量样本设置中表现新流派的能力。我们的研究结果令人鼓舞,可以为人工智能生成音乐的政策决策提供信息,提高用户的透明度。
论文及项目相关链接
PDF Published in the TrustNLP Workshop at NAACL 2025
Summary
大型语言模型在音乐内容生成,特别是歌词生成方面的应用日益普及,为艺术家提供了有价值的工具,同时也引发了版权侵犯、消费者满意度和内容垃圾信息等问题。先前的研究已探讨了不同领域的内容检测,但尚无针对音乐中的文本模式——歌词的研究。为解决这一空白,我们收集了来自多种语言、音乐流派和艺术家的真实和合成歌词数据集,并使用人工和自动化方法对生成管道进行了验证。我们对现有的合成文本检测方法在歌词上的表现进行了全面评估,并探索了通过无监督域适应调整最佳性能特征的方法。我们的研究结果有望为人工智能生成的音乐的政策决策提供依据,提高用户透明度。
Key Takeaways
- 大型语言模型在音乐内容生成中的应用逐渐普及,特别是在歌词生成方面。
- 这些应用为艺术家提供了有价值的工具,但也引发了版权侵犯、消费者满意度和内容垃圾信息等问题。
- 目前尚未有针对音乐中的文本模式——歌词的研究。
- 为了解决这一空白,收集了真实和合成歌词数据集,包括多种语言、音乐流派和艺术家。
- 生成管道的验证采用了人工和自动化方法。
- 对现有的合成文本检测方法在歌词上的表现进行了全面评估。
点此查看论文截图
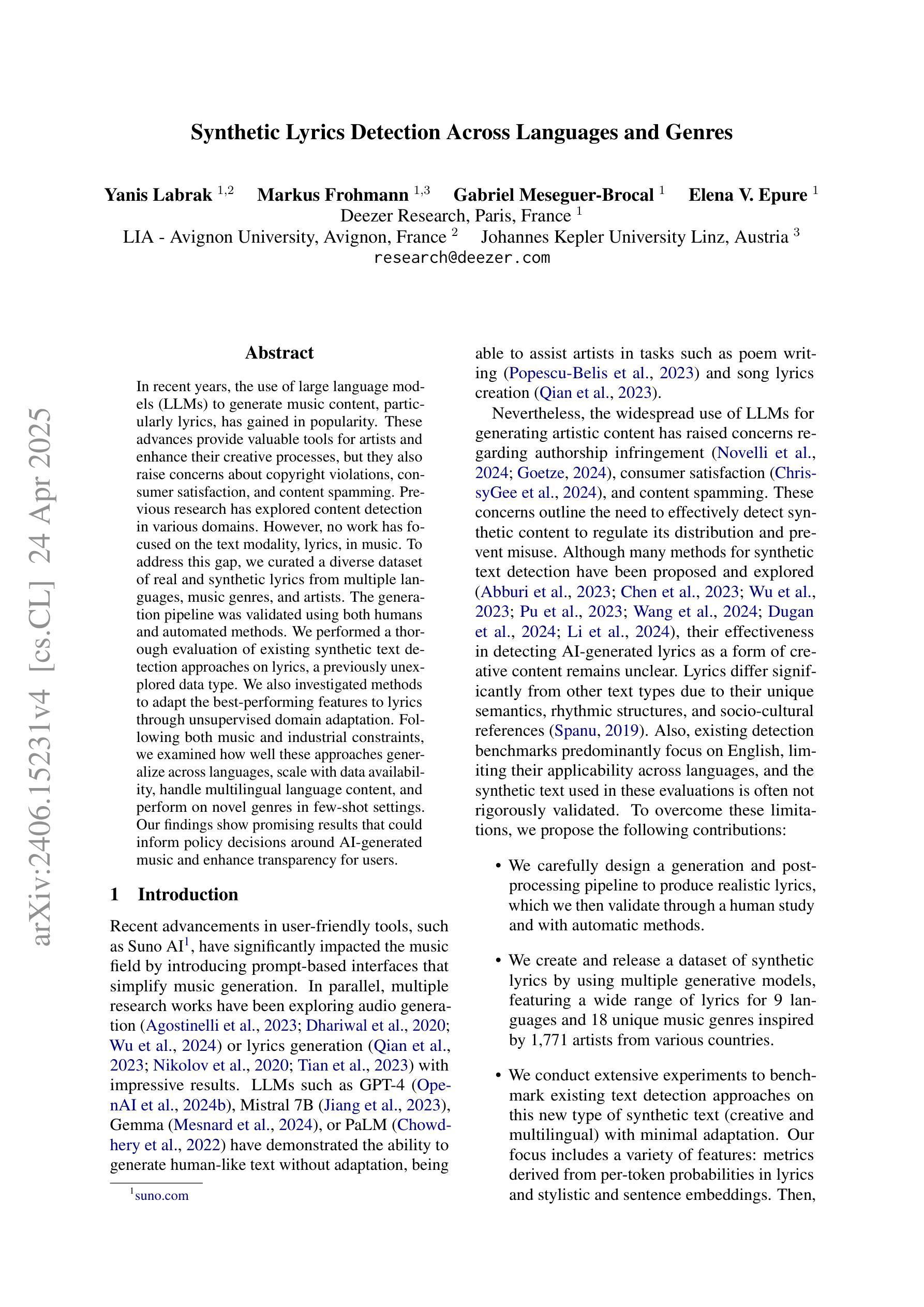
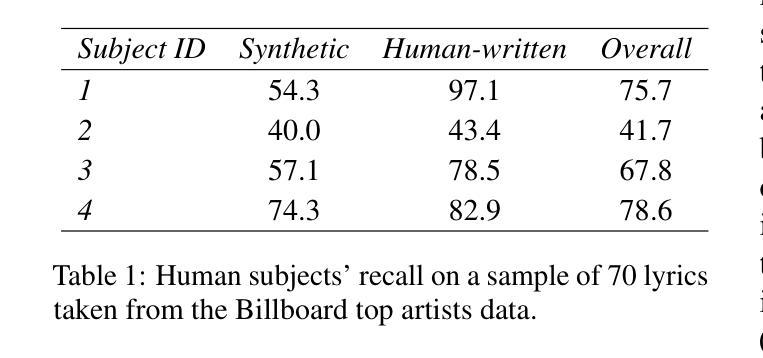
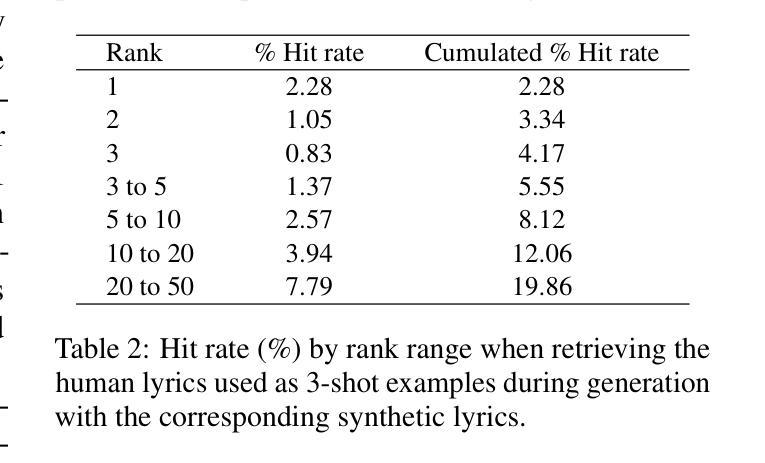
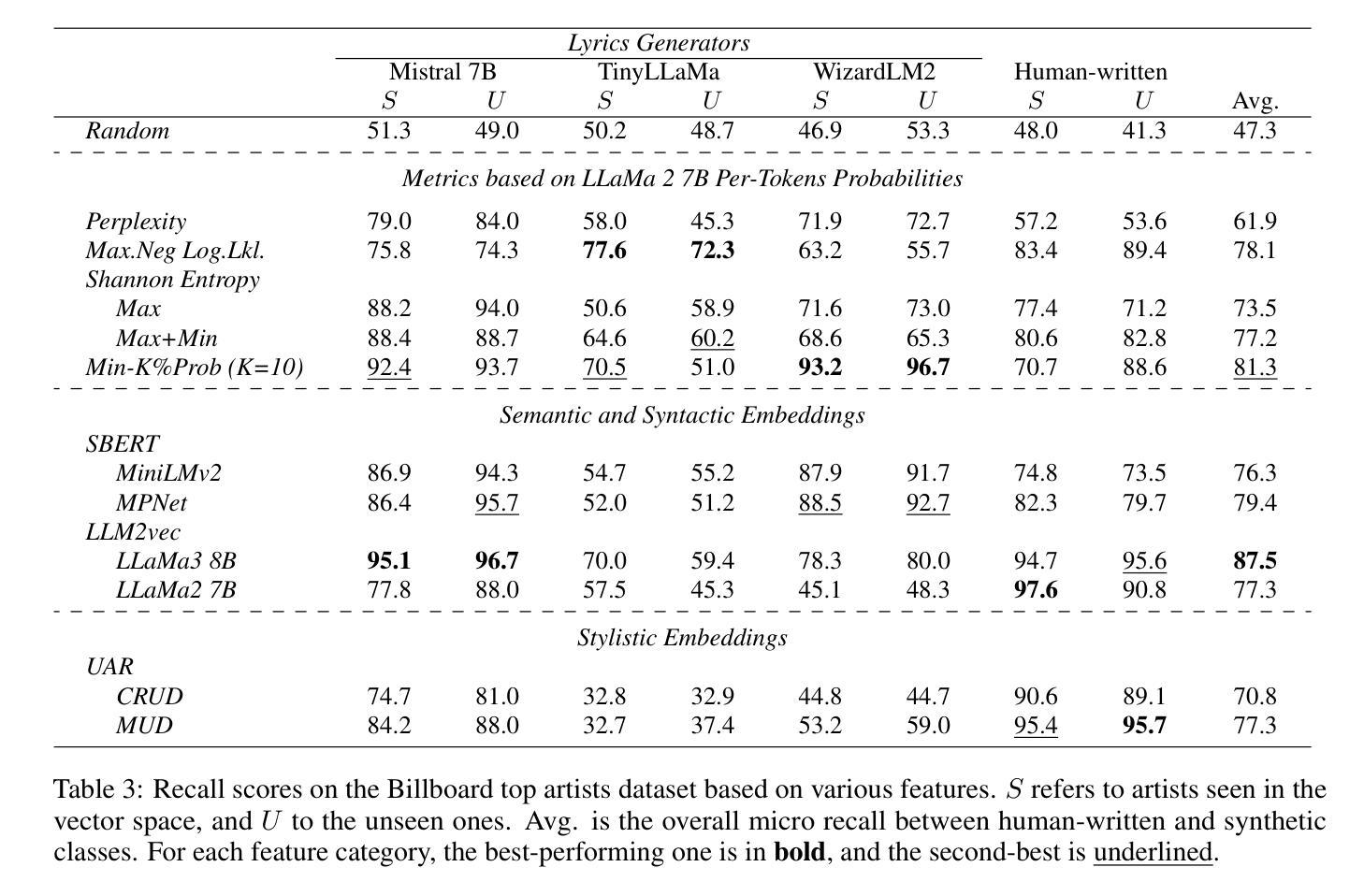