⚠️ 以下所有内容总结都来自于 大语言模型的能力,如有错误,仅供参考,谨慎使用
🔴 请注意:千万不要用于严肃的学术场景,只能用于论文阅读前的初筛!
💗 如果您觉得我们的项目对您有帮助 ChatPaperFree ,还请您给我们一些鼓励!⭐️ HuggingFace免费体验
2025-04-26 更新
The Sparse Frontier: Sparse Attention Trade-offs in Transformer LLMs
Authors:Piotr Nawrot, Robert Li, Renjie Huang, Sebastian Ruder, Kelly Marchisio, Edoardo M. Ponti
Sparse attention offers a promising strategy to extend long-context capabilities in Transformer LLMs, yet its viability, its efficiency-accuracy trade-offs, and systematic scaling studies remain unexplored. To address this gap, we perform a careful comparison of training-free sparse attention methods at varying model scales, sequence lengths, and sparsity levels on a diverse collection of long-sequence tasks-including novel ones that rely on natural language while remaining controllable and easy to evaluate. Based on our experiments, we report a series of key findings: 1) an isoFLOPS analysis reveals that for very long sequences, larger and highly sparse models are preferable to smaller and dense ones. 2) The level of sparsity attainable while statistically guaranteeing accuracy preservation is higher during decoding than prefilling, and correlates with model size in the former. 3) There is no clear strategy that performs best across tasks and phases, with different units of sparsification or budget adaptivity needed for different scenarios. Even moderate sparsity levels often result in significant performance degradation on at least one task, highlighting that sparse attention is not a universal solution. 4) We introduce and validate novel scaling laws specifically tailored for sparse attention, providing evidence that our findings are likely to hold true beyond our range of experiments. Through these insights, we demonstrate that sparse attention is a key tool to enhance the capabilities of Transformer LLMs for processing longer sequences, but requires careful evaluation of trade-offs for performance-sensitive applications.
稀疏注意力为扩展Transformer大型语言模型(LLM)的长上下文能力提供了有前景的策略,但其可行性、效率与准确度的权衡以及系统扩展研究仍未被探索。为了填补这一空白,我们在一系列长序列任务上,对不同模型规模、序列长度和稀疏水平的训练无关稀疏注意力方法进行了仔细比较。这些任务包括依赖自然语言的新型任务,同时保持可控性和易于评估的特点。基于我们的实验,我们报告了一系列重要发现:
1)isoFLOPS分析表明,对于非常长的序列,更大且高度稀疏的模型优于较小且密集的模型。
2)在解码过程中,在保证准确性保留的统计水平上,可达到的稀疏水平高于预填充阶段,并且与前者的模型规模相关。
3)没有一种策略在所有任务和阶段中表现最佳,不同的稀疏单位或预算适应性策略适用于不同场景。甚至适中的稀疏水平也往往至少在一个任务上导致性能显著下降,这表明稀疏注意力并非万能解决方案。
论文及项目相关链接
摘要
稀疏注意力机制是扩展Transformer大型语言模型(LLM)长文本处理能力的一种有前途的策略,但其可行性、效率与准确度的权衡以及系统性扩展研究仍待探索。为解决这一空白,我们对训练无关的稀疏注意力方法进行了比较,包括在不同模型规模、序列长度和稀疏层次上的多样长序列任务(包括依赖自然语言的新任务)。基于实验,我们报告了一系列关键发现:1)对于极长序列,更大且高度稀疏的模型比小而密集的模型更可取。2)在解码时可达到的保证准确度保留的稀疏水平高于预填充时的水平,并且在前者中与模型大小相关。3)没有一种策略能在所有任务和阶段中表现最佳,不同的稀疏化单位或预算适应性针对不同场景是必要的。即使适度的稀疏水平也往往导致至少一项任务性能显著下降,这表明稀疏注意力并不是万能解决方案。4)我们引入并验证了专门针对稀疏注意力的新扩展定律,证明我们的发现可能在实验范围之外也成立。通过这些见解,我们证明了稀疏注意力是提高Transformer LLM处理长序列能力的重要工具,但对于性能敏感的应用,需要仔细评估权衡。
关键见解
- 对于处理极长序列,考虑模型规模和稀疏度是关键的。较大的稀疏模型表现更佳。
- 解码时的稀疏水平可以更高,并且与模型规模有关。
- 没有一种明确的最佳策略适用于所有任务和阶段,需要针对特定场景调整稀疏化策略。
- 适度的稀疏水平可能会导致某些任务性能显著下降,因此需谨慎使用。
- 稀疏注意力并非万能解决方案,需要结合其他方法使用。
- 针对稀疏注意力的新扩展定律为未来的研究提供了指导。
点此查看论文截图
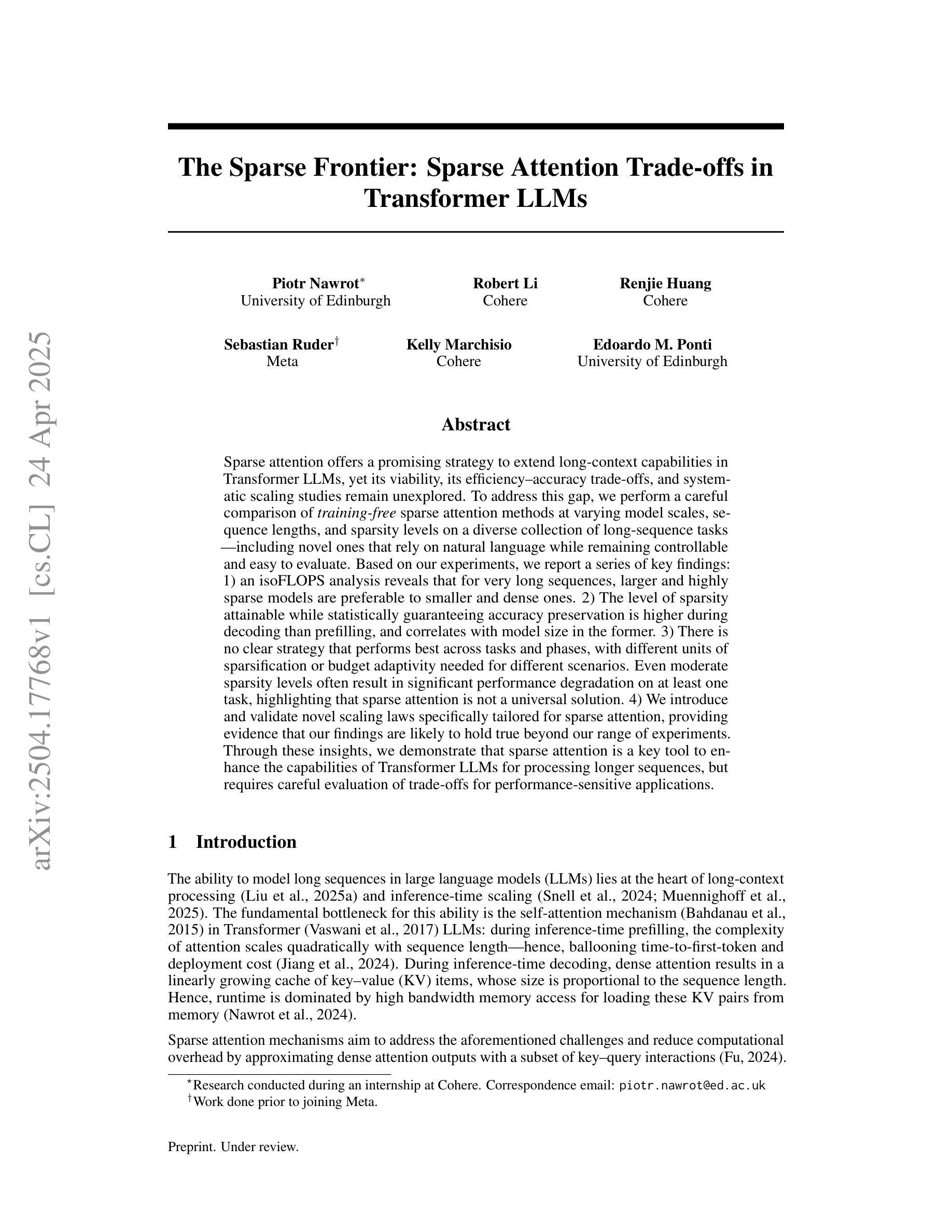
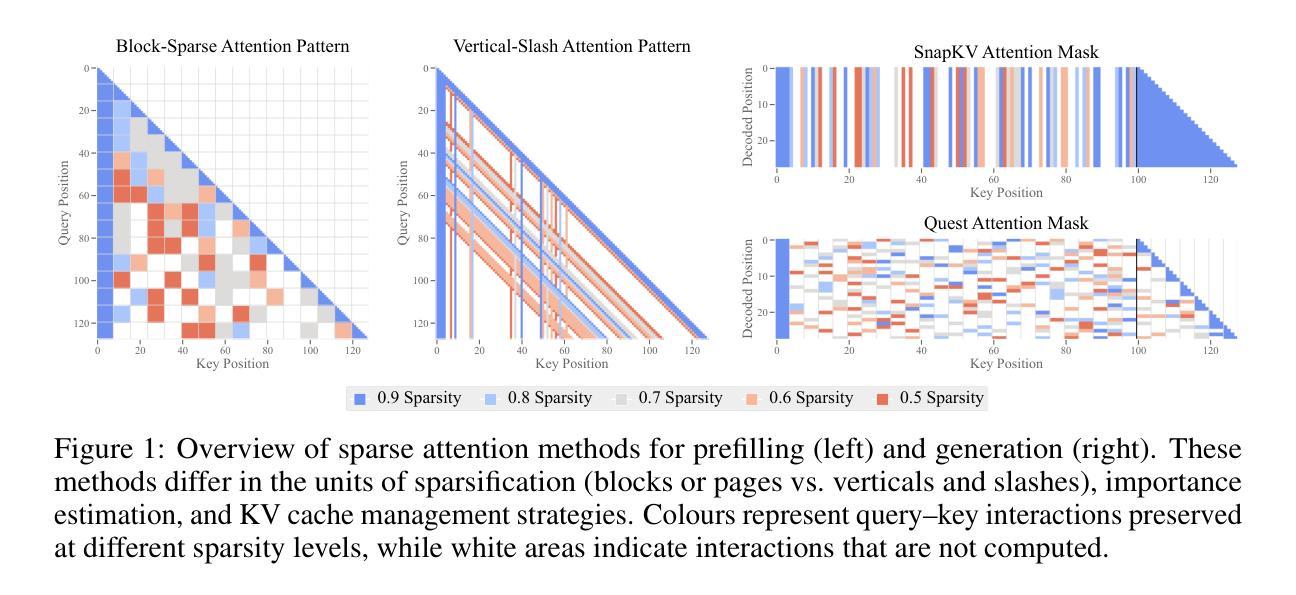
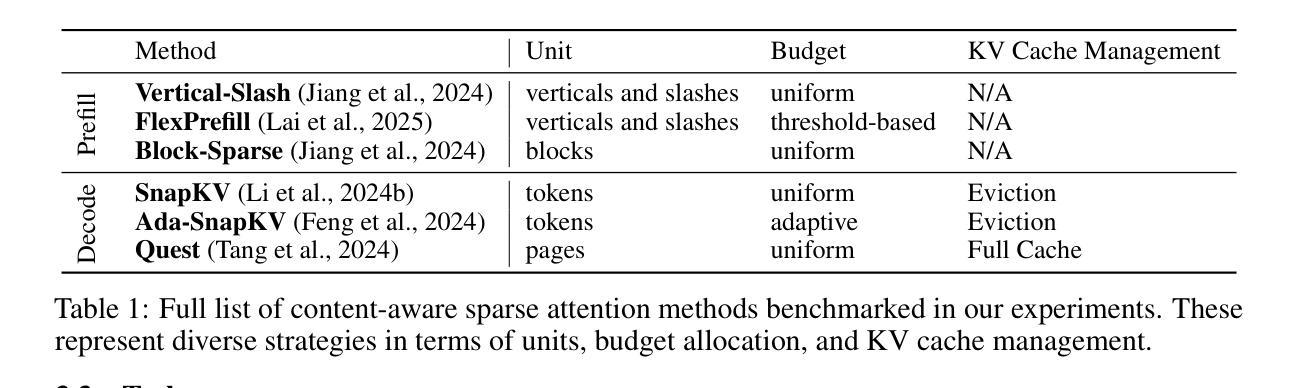
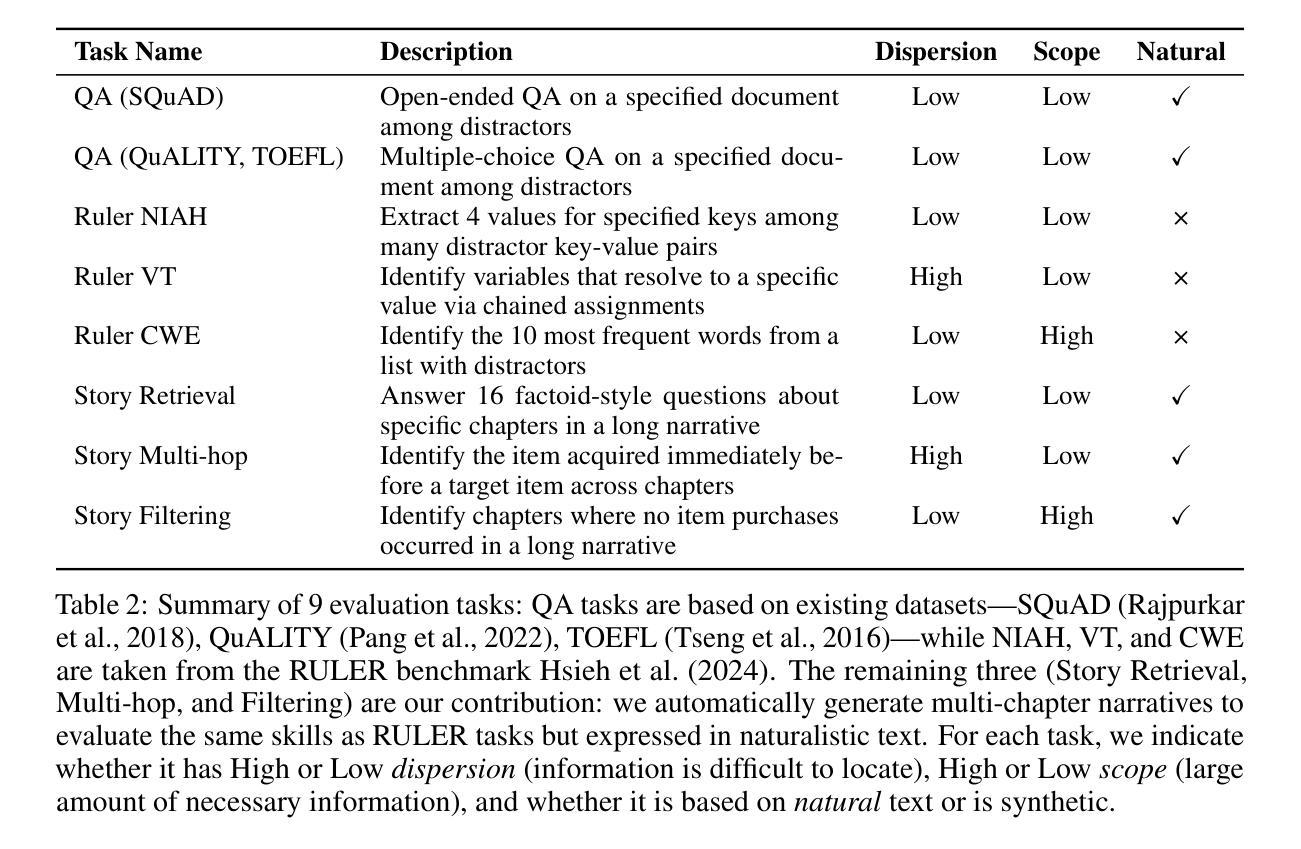
L3: DIMM-PIM Integrated Architecture and Coordination for Scalable Long-Context LLM Inference
Authors:Qingyuan Liu, Liyan Chen, Yanning Yang, Haocheng Wang, Dong Du, Zhigang Mao, Naifeng Jing, Yubin Xia, Haibo Chen
Large Language Models (LLMs) increasingly require processing long text sequences, but GPU memory limitations force difficult trade-offs between memory capacity and bandwidth. While HBM-based acceleration offers high bandwidth, its capacity remains constrained. Offloading data to host-side DIMMs improves capacity but introduces costly data swapping overhead. We identify that the critical memory bottleneck lies in the decoding phase of multi-head attention (MHA) exclusively, which demands substantial capacity for storing KV caches and high bandwidth for attention computation. Our key insight reveals this operation uniquely aligns with modern DIMM-based processing-in-memory (PIM) architectures, which offers scalability of both capacity and bandwidth. Based on this observation and insight, we propose L3, a hardware-software co-designed system integrating DIMM-PIM and GPU devices. L3 introduces three innovations: First, hardware redesigns resolve data layout mismatches and computational element mismatches in DIMM-PIM, enhancing LLM inference utilization. Second, communication optimization enables hiding the data transfer overhead with the computation. Third, an adaptive scheduler coordinates GPU-DIMM-PIM operations to maximize parallelism between devices. Evaluations using real-world traces show L3 achieves up to 6.1$\times$ speedup over state-of-the-art HBM-PIM solutions while significantly improving batch sizes.
大型语言模型(LLMs)越来越需要处理长文本序列,但GPU内存限制使得在内存容量和带宽之间做出艰难的权衡。虽然基于HBM的加速提供了高带宽,但其容量仍然受限。将数据迁移到主机侧DIMMs可以提高容量,但引入了昂贵的数据交换开销。我们确定关键的内存瓶颈仅存在于多头注意力(MHA)的解码阶段,该阶段需要大量存储空间来存储KV缓存和用于注意力计算的高带宽。我们的关键见解是,这一操作与现代DIMMs基于内存处理(PIM)的架构完全契合,该架构在容量和带宽方面提供了可扩展性。基于这一观察力和洞察力,我们提出了L3系统,这是一个结合了DIMM-PIM和GPU设备的软硬件协同设计系统。L3引入了三项创新:首先,硬件重新设计解决了DIMM-PIM中的数据布局不匹配和计算元素不匹配的问题,提高了大型语言模型推理的利用率;其次,通信优化能够隐藏数据传输过程中的计算开销;第三,自适应调度器协调GPU-DIMM-PIM操作,以最大限度地提高设备之间的并行性。使用真实世界数据的评估表明,L3与当前先进的HBM-PIM解决方案相比,速度提高了高达6.1倍,并且显著提高了批处理规模。
论文及项目相关链接
PDF 16 pages, 11 figures
Summary
在大型语言模型(LLM)处理长文本序列时,存在GPU内存限制的问题,这需要在内存容量和带宽之间进行权衡。文章指出关键瓶颈在于多头注意力(MHA)的解码阶段,需要大量内存缓存和高带宽进行注意力计算。文章结合现代DIMM内存中的处理(PIM)架构,提出了一个硬件和软件协同设计的系统L3,该系统结合了DIMM-PIM和GPU设备。L3通过硬件重新设计、通信优化和自适应调度器三个创新点,实现了对LLM推理的高效利用。评估结果表明,与现有的HBM-PIM解决方案相比,L3的速度提高了高达6.1倍,同时显著提高了批次大小。
Key Takeaways
- 大型语言模型(LLM)处理长文本序列时面临GPU内存限制的挑战。
- 关键瓶颈在于多头注意力(MHA)的解码阶段,需要大量内存缓存和高带宽。
- 现代DIMM-PIM架构为解决这个问题提供了可能性,结合了容量和带宽的可扩展性。
- L3系统结合了DIMM-PIM和GPU设备,通过硬件和软件协同设计来解决这个问题。
- L3通过硬件重新设计、通信优化和自适应调度器三个创新点来优化性能。
- L3实现了对LLM推理的高效利用,评估结果表明其速度比现有解决方案更快。
点此查看论文截图
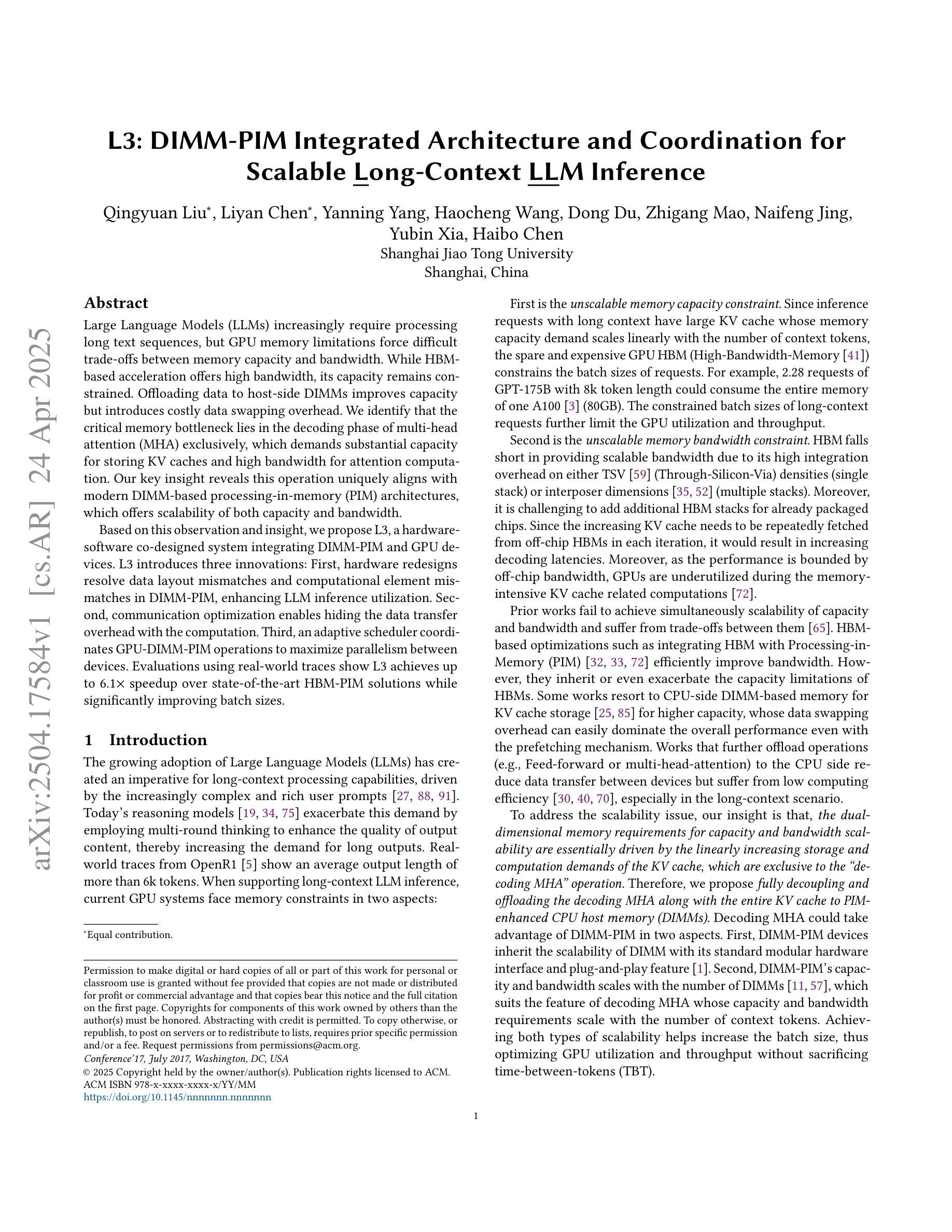
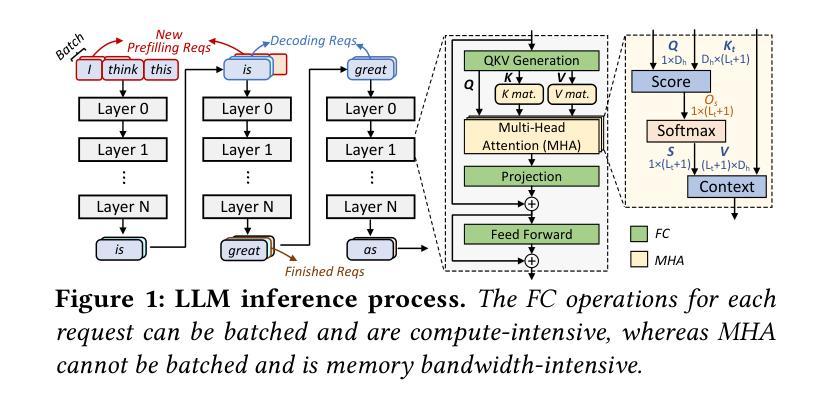
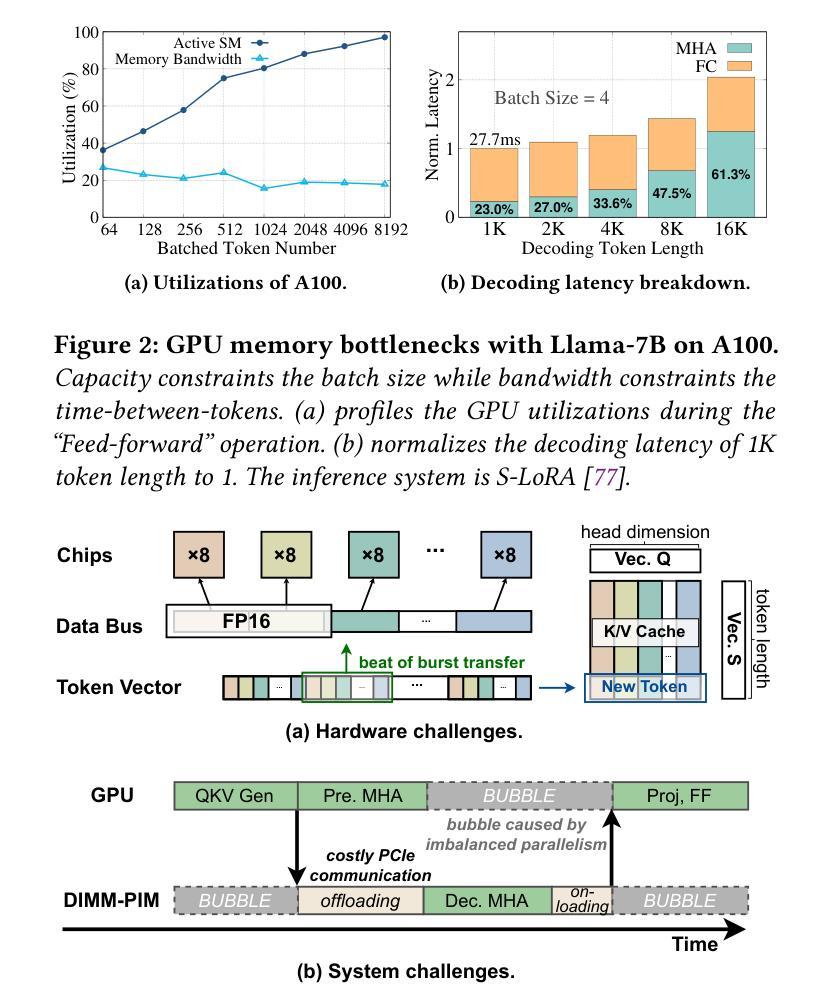
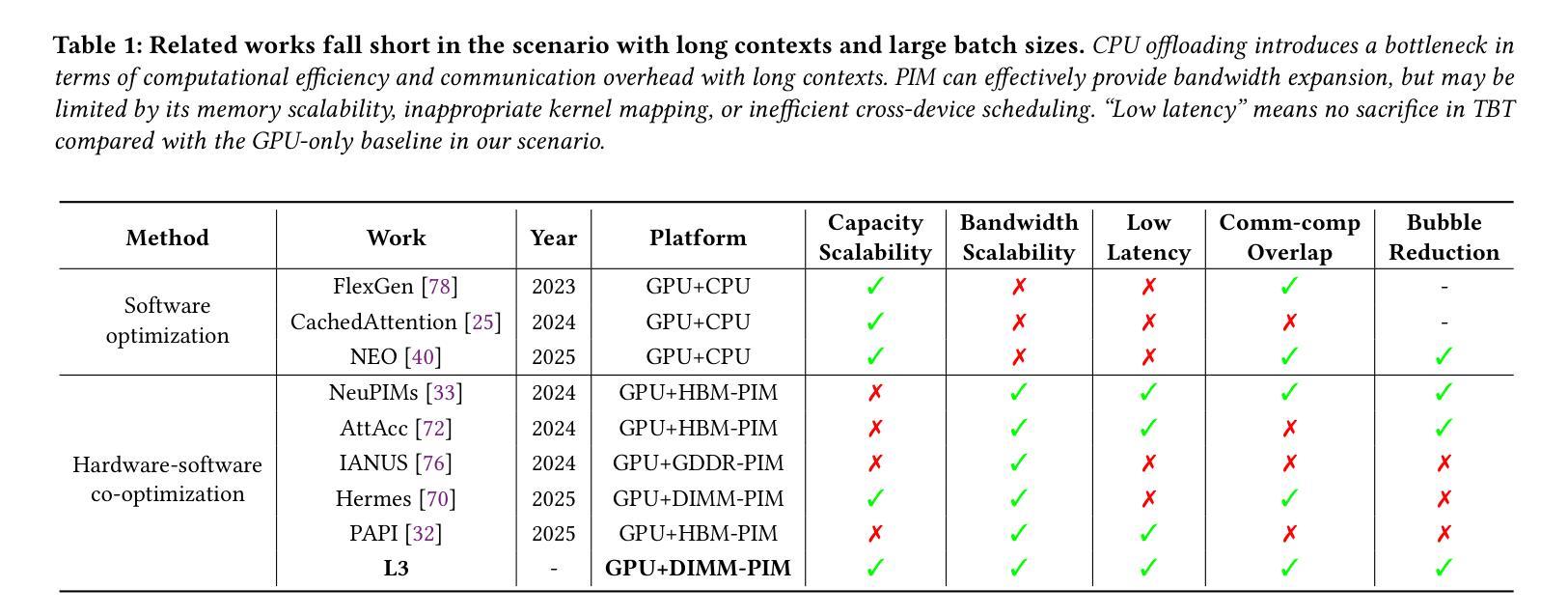
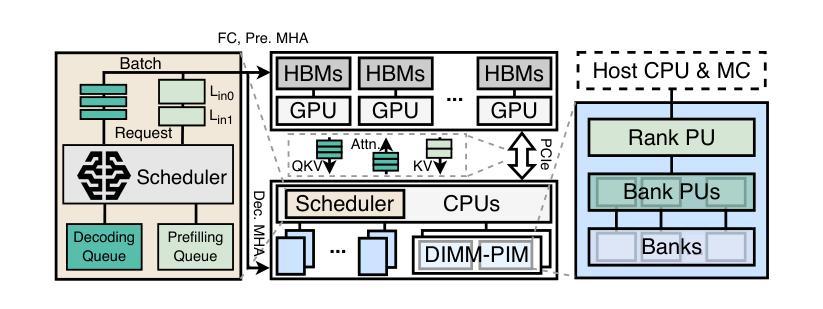
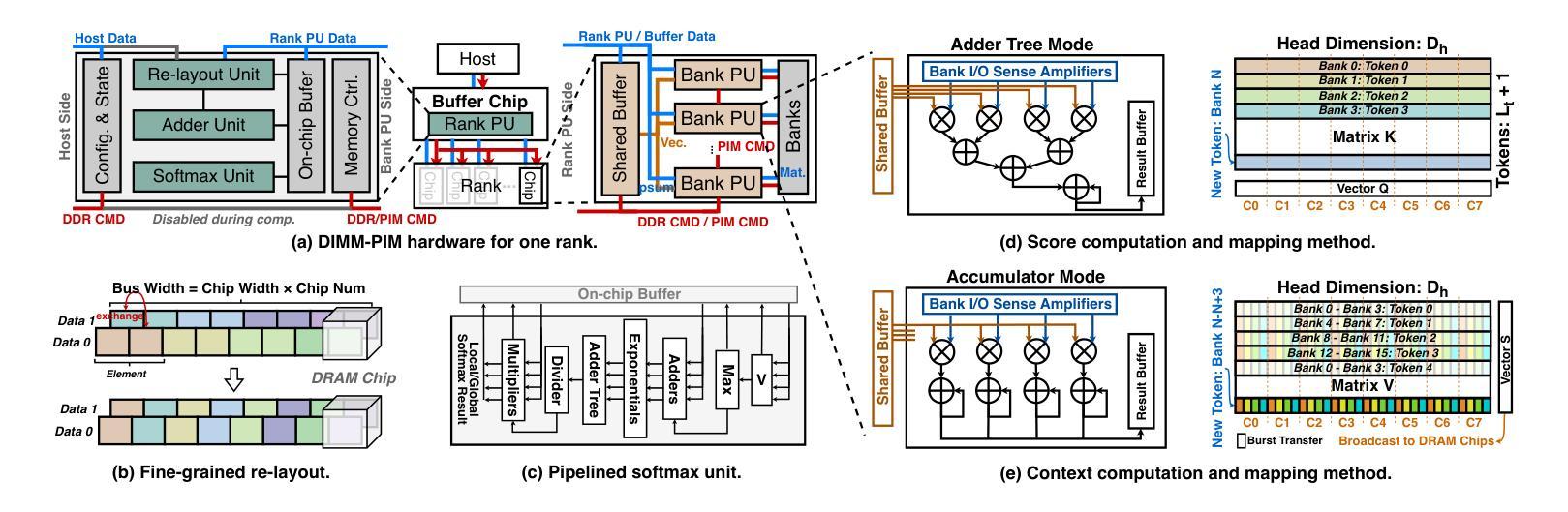
DeepDistill: Enhancing LLM Reasoning Capabilities via Large-Scale Difficulty-Graded Data Training
Authors:Xiaoyu Tian, Sitong Zhao, Haotian Wang, Shuaiting Chen, Yiping Peng, Yunjie Ji, Han Zhao, Xiangang Li
Although large language models (LLMs) have recently achieved remarkable performance on various complex reasoning benchmarks, the academic community still lacks an in-depth understanding of base model training processes and data quality. To address this, we construct a large-scale, difficulty-graded reasoning dataset containing approximately 3.34 million unique queries of varying difficulty levels and about 40 million distilled responses generated by multiple models over several passes. Leveraging pass rate and Coefficient of Variation (CV), we precisely select the most valuable training data to enhance reasoning capability. Notably, we observe a training pattern shift, indicating that reasoning-focused training based on base models requires higher learning rates for effective training. Using this carefully selected data, we significantly improve the reasoning capabilities of the base model, achieving a pass rate of 79.2% on the AIME2024 mathematical reasoning benchmark. This result surpasses most current distilled models and closely approaches state-of-the-art performance. We provide detailed descriptions of our data processing, difficulty assessment, and training methodology, and have publicly released all datasets and methods to promote rapid progress in open-source long-reasoning LLMs. The dataset is available at: https://huggingface.co/datasets/a-m-team/AM-DeepSeek-Distilled-40M
尽管大型语言模型(LLM)最近在各种复杂的推理基准测试中取得了显著的性能,但学术界仍然缺乏对基础模型训练过程和数据质量的深入了解。为了解决这个问题,我们构建了一个大规模、难度分级的推理数据集,包含大约334万个不同难度级别的唯一查询和约由多个模型多次传递生成的4千万个蒸馏响应。我们利用通过率和变异系数(CV)精确选择最有价值的训练数据,以提高推理能力。值得注意的是,我们观察到训练模式的变化,这表明基于基础模型的推理训练需要更高的学习率才能进行有效训练。使用经过精心挑选的数据,我们显著提高了基础模型的推理能力,在AIME2024数学推理基准测试上的通过率达到79.2%。这一结果超越了大多数当前的蒸馏模型,并接近了最先进的性能。我们提供了关于数据处理、难度评估和培训方法的详细描述,并已公开发布所有数据集和方法,以促进开源长推理LLM的快速发展。数据集可在:https://huggingface.co/datasets/a-m-team/AM-DeepSeek-Distilled-40M 获取。
论文及项目相关链接
Summary:
大型语言模型(LLM)在复杂推理基准测试中表现出卓越性能,但仍缺乏对基础模型训练过程和数据质量的深入了解。为此,我们构建了一个大规模、难度分级的推理数据集,包含约334万条唯一查询和约4千万条蒸馏响应。通过利用通过率(pass rate)和变异系数(Coefficient of Variation,CV),我们精确选择了最有价值的训练数据以增强推理能力。此外,观察到训练模式转变,表明基于基础模型的推理训练需要更高的学习率进行有效训练。使用这些数据,我们显著提高了基础模型的推理能力,在AIME2024数学推理基准测试中达到了79.2%的通过率,超越大多数现有蒸馏模型,接近最新技术水平。我们提供了详细的数据处理、难度评估和训练方法描述,并已公开发布所有数据集和方法,以促进开源长推理LLM的快速发展。
Key Takeaways:
- 构建了一个大规模难度分级的推理数据集,包含数百万条查询和响应。
- 利用通过率(pass rate)和变异系数(Coefficient of Variation, CV)选择训练数据以增强推理能力。
- 观察到了训练模式的转变,指出基于基础模型的推理训练需要更高的学习率。
- 通过精心选择数据和调整训练策略,显著提高了基础模型的推理能力。
- 在AIME2024数学推理基准测试中取得了高通过率,表现超越多数现有模型并接近最新技术。
- 提供了详细的数据处理、难度评估及训练方法的描述。
点此查看论文截图
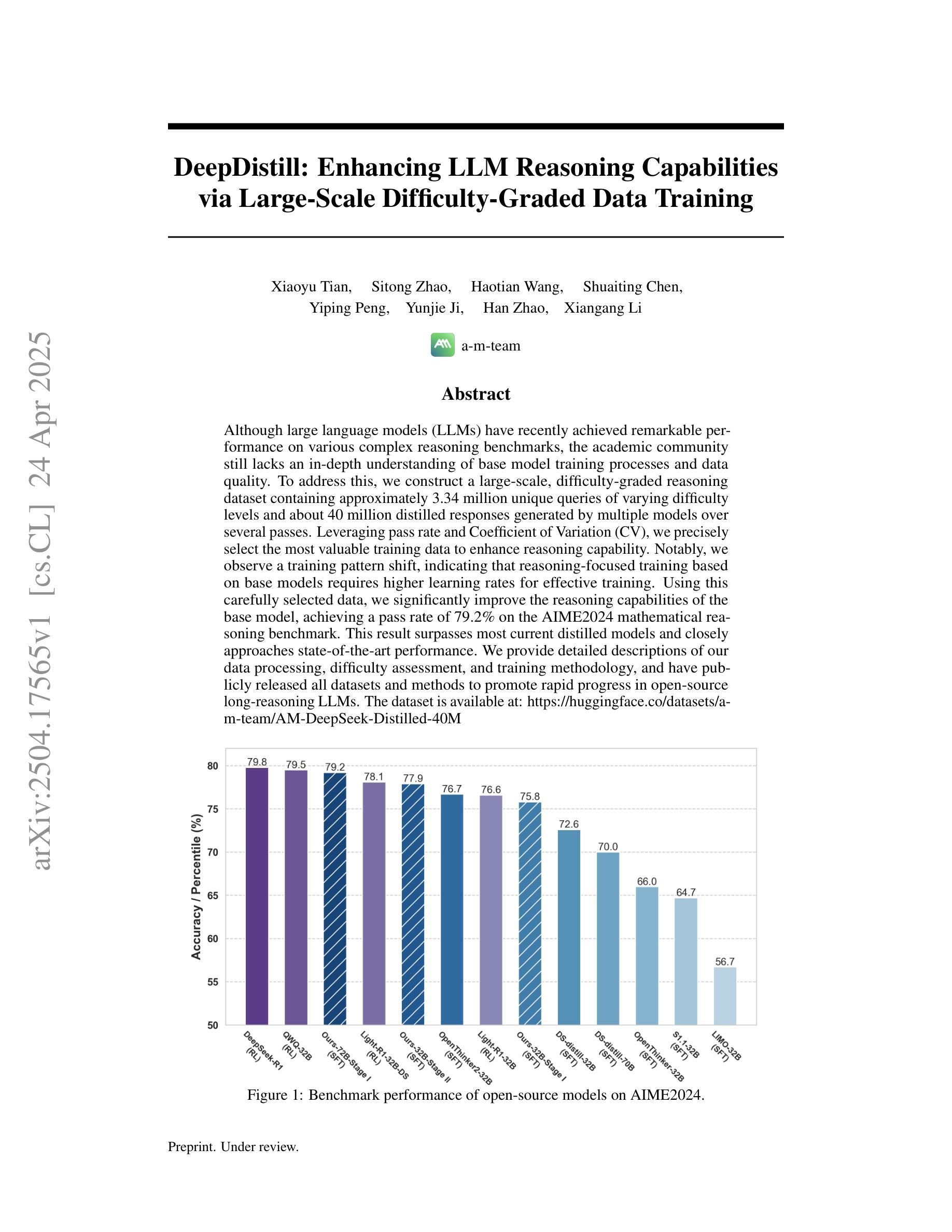
Large Language Model-Driven Concolic Execution for Highly Structured Test Input Generation
Authors:Haoxin Tu, Seongmin Lee, Yuxian Li, Peng Chen, Lingxiao Jiang, Marcel Böhme
How can we perform concolic execution to generate highly structured test inputs for systematically testing parsing programs? Existing concolic execution engines are significantly restricted by (1) input structure-agnostic path constraint selection, leading to the waste of testing effort or missing coverage; (2) limited constraint-solving capability, yielding many syntactically invalid test inputs; (3) reliance on manual acquisition of highly structured seed inputs, resulting in non-continuous testing. This paper proposes Cottontail, a new Large Language Model (LLM)-driven concolic execution engine, to mitigate the above limitations. A more complete program path representation, named Expressive Structural Coverage Tree (ESCT), is first constructed to select structure-aware path constraints. Later, an LLM-driven constraint solver based on a Solve-Complete paradigm is designed to solve the path constraints smartly to get test inputs that are not only satisfiable to the constraints but also valid to the input syntax. Finally, a history-guided seed acquisition is employed to obtain new highly structured test inputs either before testing starts or after testing is saturated. We implemented Cottontail on top of SymCC and evaluated eight extensively tested open-source libraries across four different formats (XML, SQL, JavaScript, and JSON). The experimental result is promising: it shows that Cottontail outperforms state-of-the-art approaches (SymCC and Marco) by 14.15% and 14.31% in terms of line coverage. Besides, Cottontail found 6 previously unknown vulnerabilities (six new CVEs have been assigned). We have reported these issues to developers, and 4 out of them have been fixed so far.
如何执行符号执行与模拟执行结合的测试方法(Concolic Execution),以生成高度结构化的测试输入,对解析程序进行系统测试?现有的符号执行与模拟执行结合测试引擎受到以下限制:(1)输入结构无关的路径约束选择,导致测试工作浪费或覆盖不足;(2)有限的约束求解能力,产生许多语法上无效的测试输入;(3)高度结构化种子输入的依赖手动获取,导致非连续测试。本文提出了一个新的大型语言模型(LLM)驱动的符号执行与模拟执行结合测试引擎——Cottontail,以缓解上述限制。首先,构建了一个名为表达性结构覆盖树(ESCT)的更完整的程序路径表示,以选择结构感知的路径约束。然后,设计了一种基于求解完整范式的LLM驱动约束求解器,以智能地解决路径约束,从而获得不仅满足约束而且符合输入语法的测试输入。最后,采用历史引导的种子获取方式,在测试开始之前或测试饱和之后获得新的高度结构化的测试输入。我们在SymCC之上实现了Cottontail,并评估了四个不同格式(XML、SQL、JavaScript和JSON)的八个广泛测试的开源库。实验结果具有前景:它表明,在行覆盖率方面,Cottontail比最新方法(SymCC和Marco)高出14.15%和14.31%。此外,Cottontail发现了6个以前未知的漏洞(已分配了六个新的CVE)。我们已经向开发人员报告了这些问题,目前已有四个得到修复。
论文及项目相关链接
PDF 18 pages (including Appendix)
Summary
基于大型语言模型(LLM)的Cottontail执行引擎提出解决concolic执行生成高度结构化测试输入的问题。它解决了现有concolic执行引擎的限制,如路径约束选择的结构无关性、约束求解能力有限以及依赖手动获取高度结构化种子输入的问题。通过构建表达性结构覆盖树(ESCT)进行更完整的程序路径表示,智能地解决路径约束来生成满足约束且符合输入语法的测试输入。实验结果表明,Cottontail在行覆盖率方面优于其他方法,并发现了六个以前未知的漏洞。
Key Takeaways
- Cottontail是一个基于大型语言模型(LLM)的concolic执行引擎,旨在解决现有concolic执行引擎在生成高度结构化测试输入方面的限制。
- 通过构建表达性结构覆盖树(ESCT)来进行更完整的程序路径表示,以选择结构感知的路径约束。
- 采用基于Solve-Complete范式的LLM驱动约束求解器,智能地解决路径约束,生成既满足约束又符合输入语法的测试输入。
- Cottontail实现了历史引导的种子获取,可在测试开始前或测试饱和后获取新的高度结构化测试输入。
点此查看论文截图
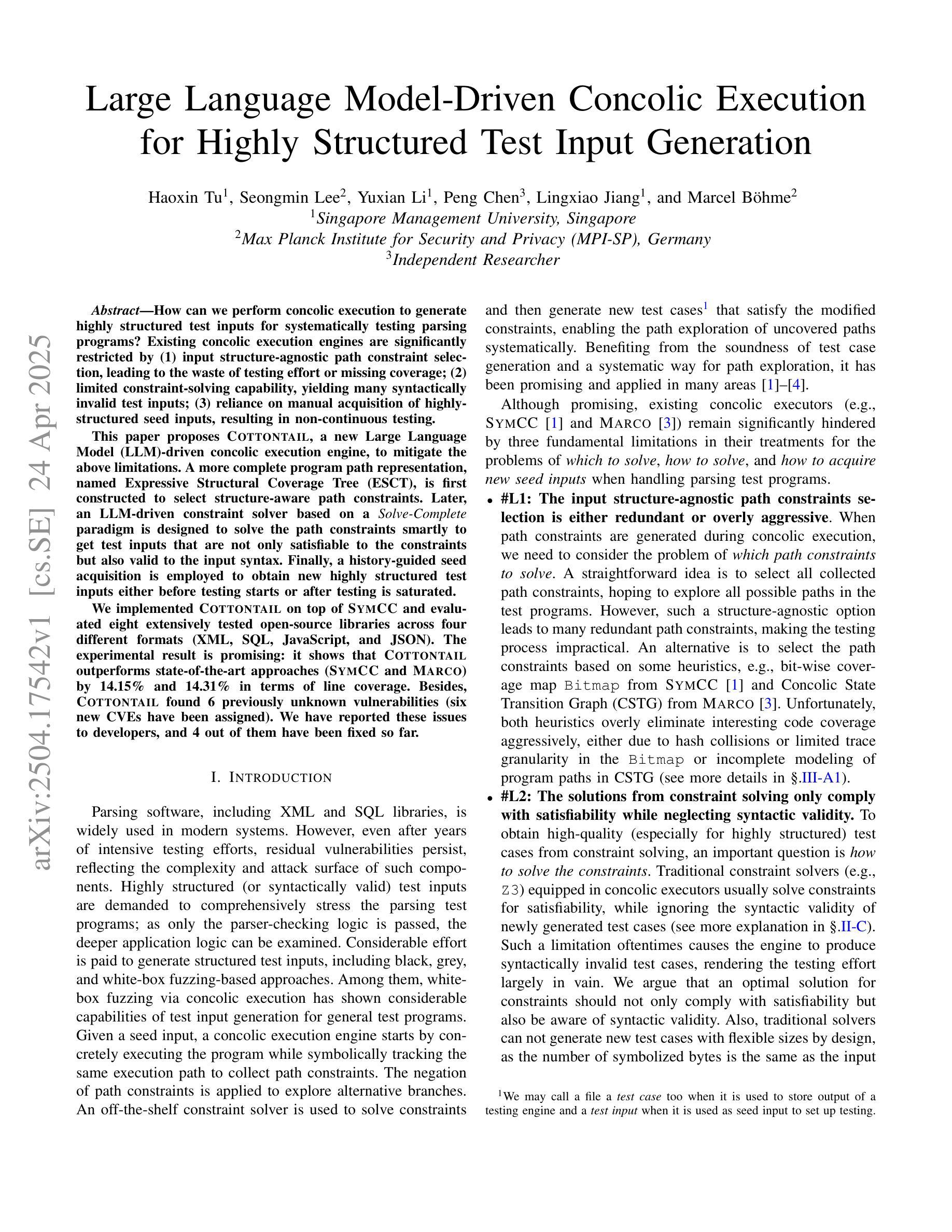
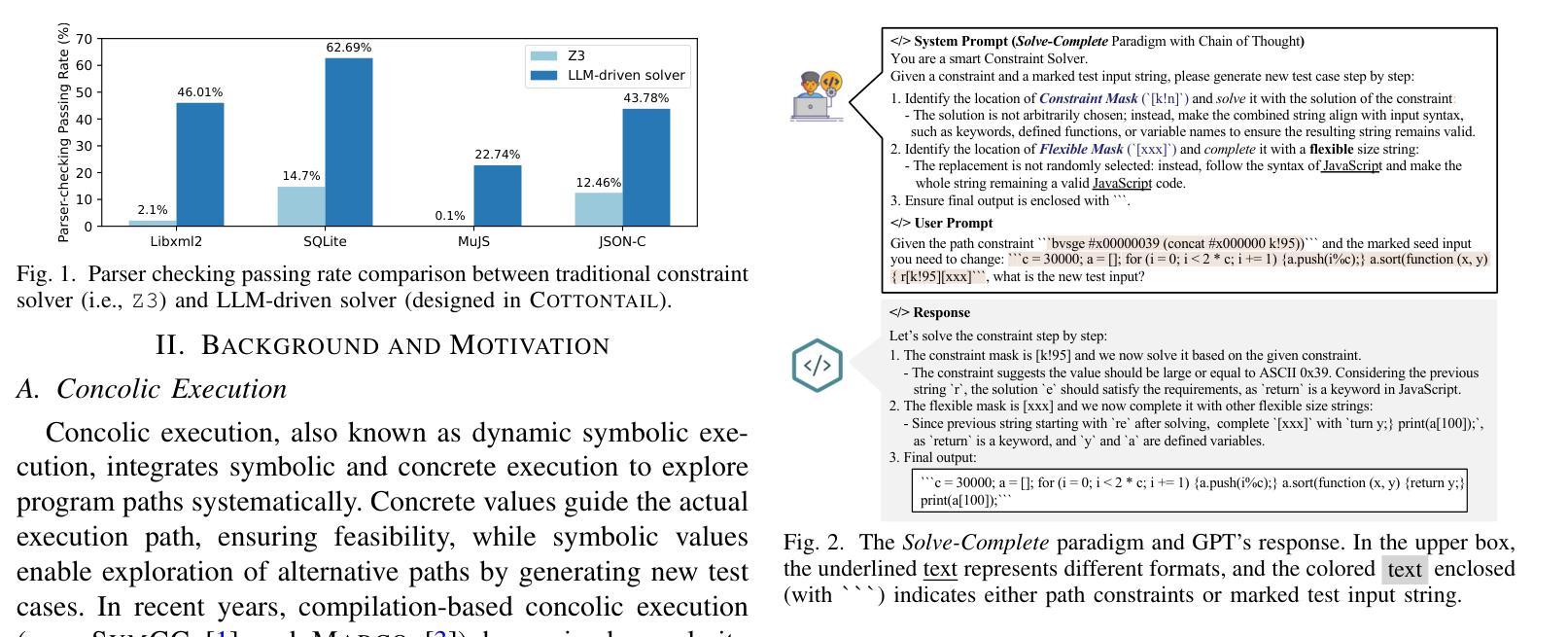
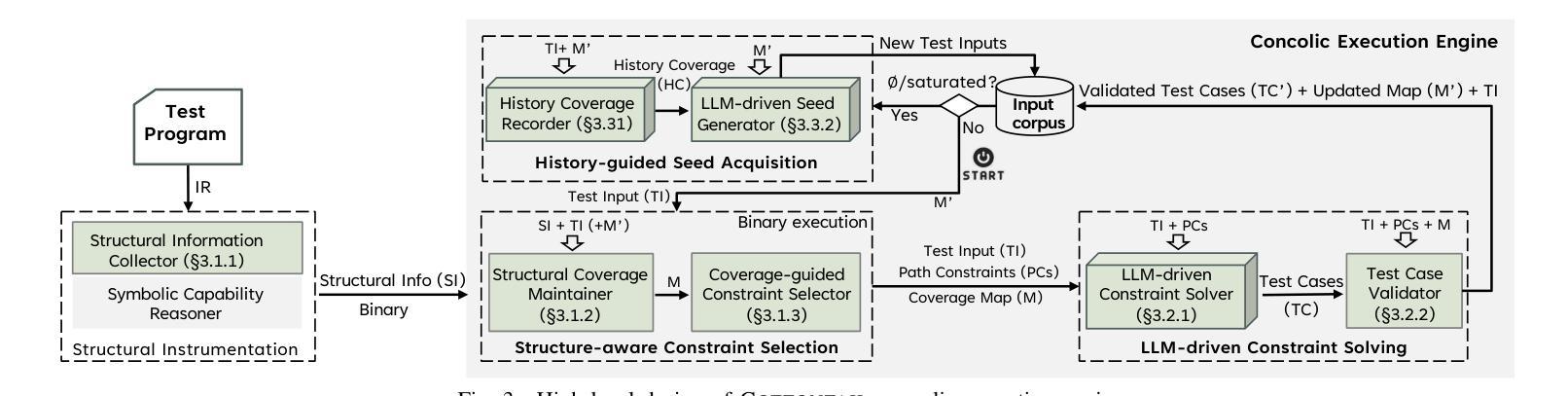
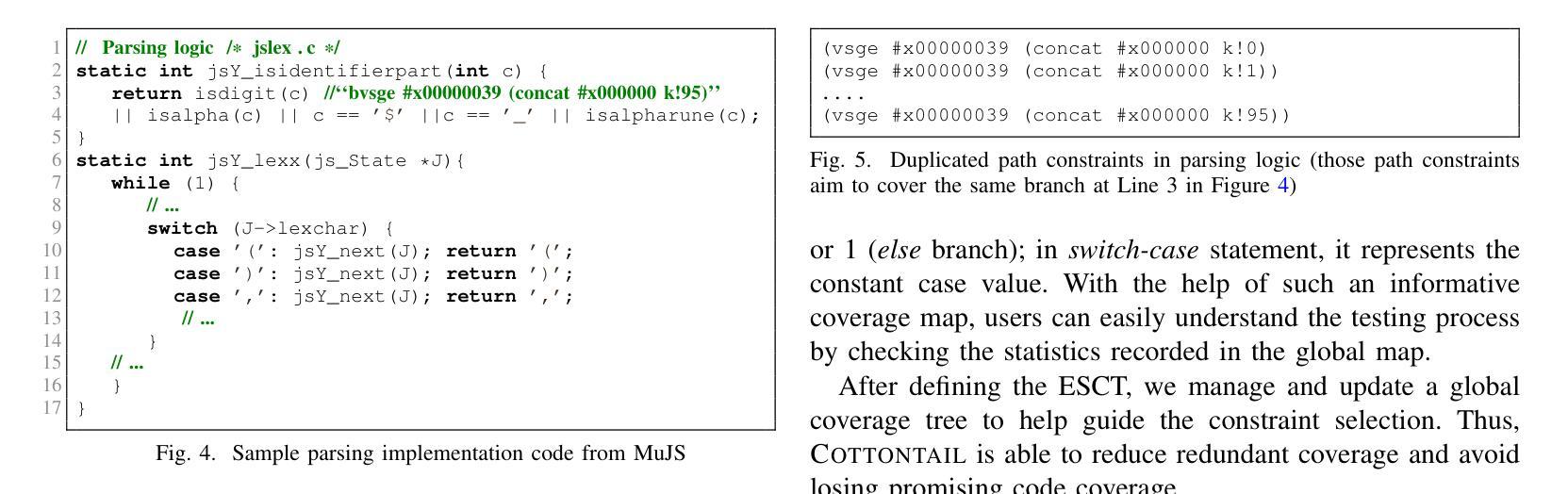
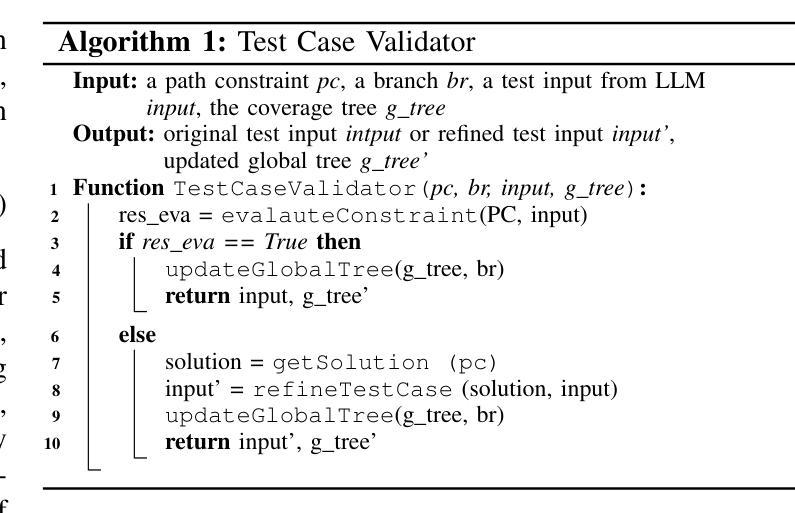
A Novel Graph Transformer Framework for Gene Regulatory Network Inference
Authors:Binon Teji, Swarup Roy
The inference of gene regulatory networks (GRNs) is a foundational stride towards deciphering the fundamentals of complex biological systems. Inferring a possible regulatory link between two genes can be formulated as a link prediction problem. Inference of GRNs via gene coexpression profiling data may not always reflect true biological interactions, as its susceptibility to noise and misrepresenting true biological regulatory relationships. Most GRN inference methods face several challenges in the network reconstruction phase. Therefore, it is important to encode gene expression values, leverege the prior knowledge gained from the available inferred network structures and positional informations of the input network nodes towards inferring a better and more confident GRN network reconstruction. In this paper, we explore the integration of multiple inferred networks to enhance the inference of Gene Regulatory Networks (GRNs). Primarily, we employ autoencoder embeddings to capture gene expression patterns directly from raw data, preserving intricate biological signals. Then, we embed the prior knowledge from GRN structures transforming them into a text-like representation using random walks, which are then encoded with a masked language model, BERT, to generate global embeddings for each gene across all networks. Additionally, we embed the positional encodings of the input gene networks to better identify the position of each unique gene within the graph. These embeddings are integrated into graph transformer-based model, termed GT-GRN, for GRN inference. The GT-GRN model effectively utilizes the topological structure of the ground truth network while incorporating the enriched encoded information. Experimental results demonstrate that GT-GRN significantly outperforms existing GRN inference methods, achieving superior accuracy and highlighting the robustness of our approach.
基因调控网络(GRNs)的推断是解开复杂生物系统基本原理的重要步骤。推断两个基因之间可能的调控关系可以制定为链接预测问题。通过基因共表达谱数据推断GRNs可能并不总是反映真实的生物相互作用,因为其容易受到噪声和误报真实生物调控关系的影响。大多数GRN推断方法在网络重建阶段面临几个挑战。因此,编码基因表达值、利用从现有推断网络结构和输入节点位置信息中获得的先验知识,对于推断更好、更可靠的GRN网络重建非常重要。在本文中,我们探讨了整合多个推断网络以增强基因调控网络(GRNs)的推断。我们主要使用自动编码器嵌入直接从原始数据中捕获基因表达模式,保留复杂的生物信号。然后,我们使用随机游走将GRN结构的先验知识转化为类似文本的表示,然后使用掩码语言模型BERT生成所有网络每个基因的全局嵌入。此外,我们对输入基因网络的位置编码进行嵌入,以更好地识别图中每个唯一基因的位置。这些嵌入被集成到基于图变压器的模型中,用于GRNs推断,名为GT-GRNs。GT-GRNs模型有效地利用真实网络的拓扑结构,同时融入丰富的编码信息。实验结果表明,GT-GRNs显著优于现有的GRN推断方法,具有较高的准确性和稳健性。
论文及项目相关链接
摘要
基因调控网络(GRNs)的推断是解开复杂生物系统基本奥秘的重要步骤。通过基因共表达谱数据推断GRNs可能无法真实反映生物相互作用,因为这种方法容易受到噪声干扰并且可能误报真实的生物调控关系。大多数GRN推断方法在网络重建阶段面临挑战。因此,编码基因表达值、利用现有推断网络结构的先验知识以及输入网络节点的位置信息对于推断更好、更可靠的GRNs网络重建至关重要。本文探索了整合多个推断网络以增强基因调控网络(GRNs)的推断。我们主要使用自动编码器嵌入直接从原始数据中捕获基因表达模式,保留复杂的生物信号。然后,我们使用随机游走将GRNs结构的先验知识转化为文本式表示,并使用掩码语言模型BERT生成所有网络每个基因的全局嵌入。此外,我们对输入基因网络的位置编码进行嵌入,以更好地识别图中每个独特基因的位置。这些嵌入被集成到基于图变压器的模型中,称为GT-GRN,用于GRNs推断。GT-GRN模型有效地利用地面真实网络的拓扑结构,同时融入丰富的编码信息。实验结果表明,GT-GRN显著优于现有的GRN推断方法,具有较高的准确性和稳健性。
关键见解
- 基因调控网络(GRNs)的推断是理解复杂生物系统的基础。
- 现有方法通过基因共表达谱数据推断GRNs可能存在误差,易受噪声干扰并可能误报真实生物调控关系。
- 整合多个推断网络能增强GRNs的推断。
- 使用自动编码器嵌入直接从原始数据中捕获基因表达模式,并保留复杂的生物信号。
- 利用随机游走和BERT模型将先验知识转化为文本表示,并生成每个基因的全局嵌入。
- 整合位置编码以识别图中每个基因的独特位置。
点此查看论文截图
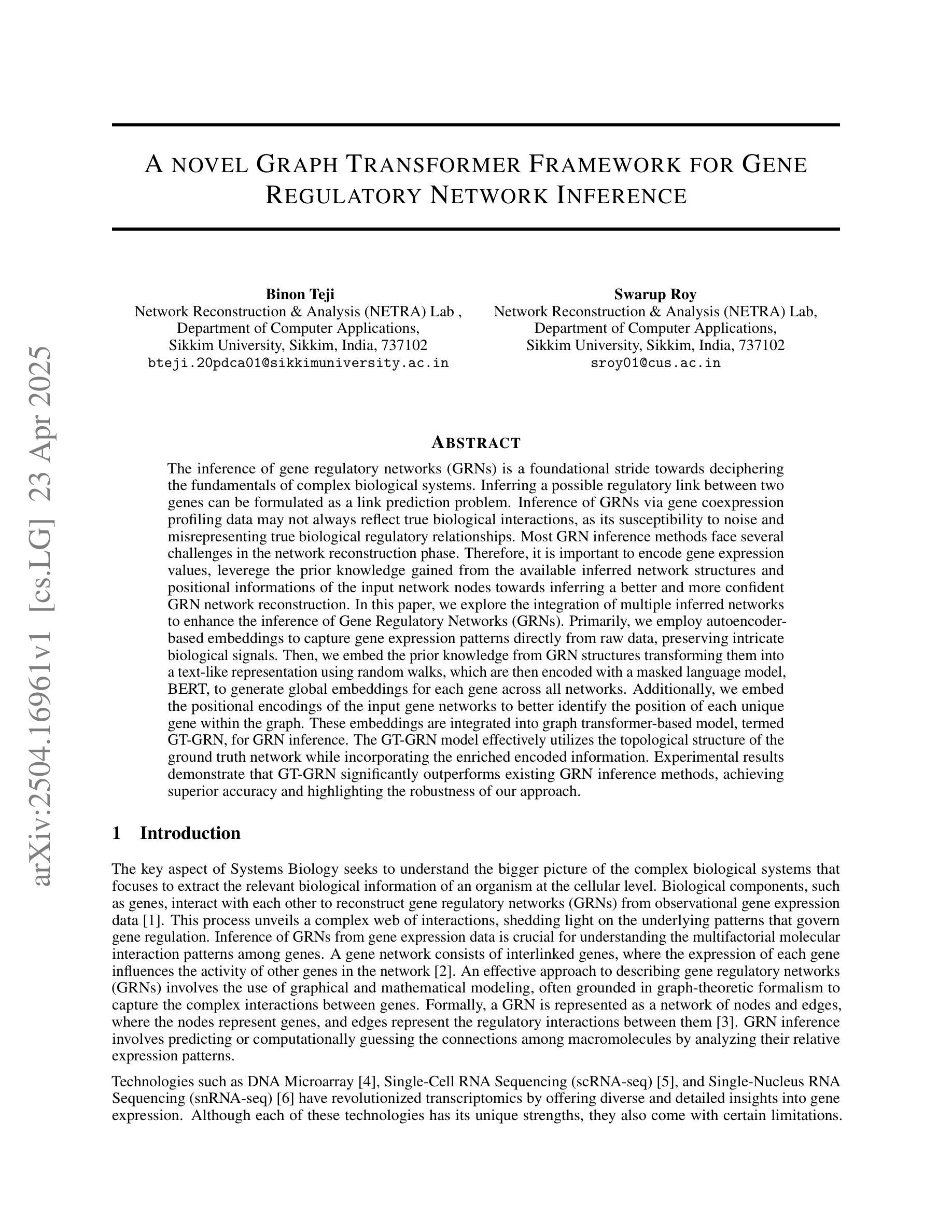
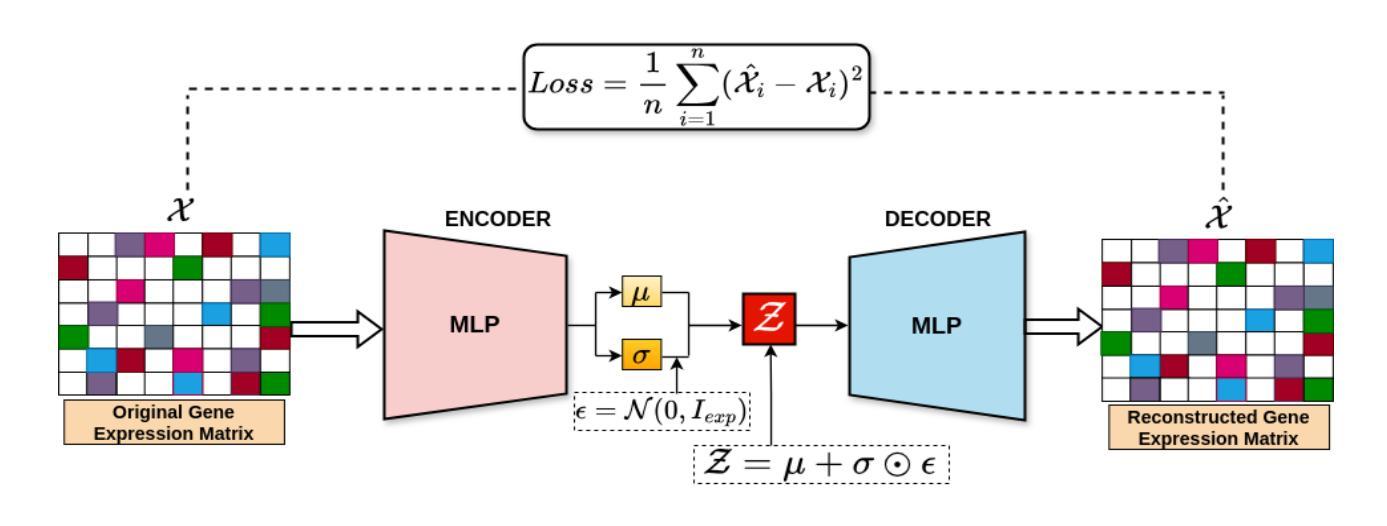
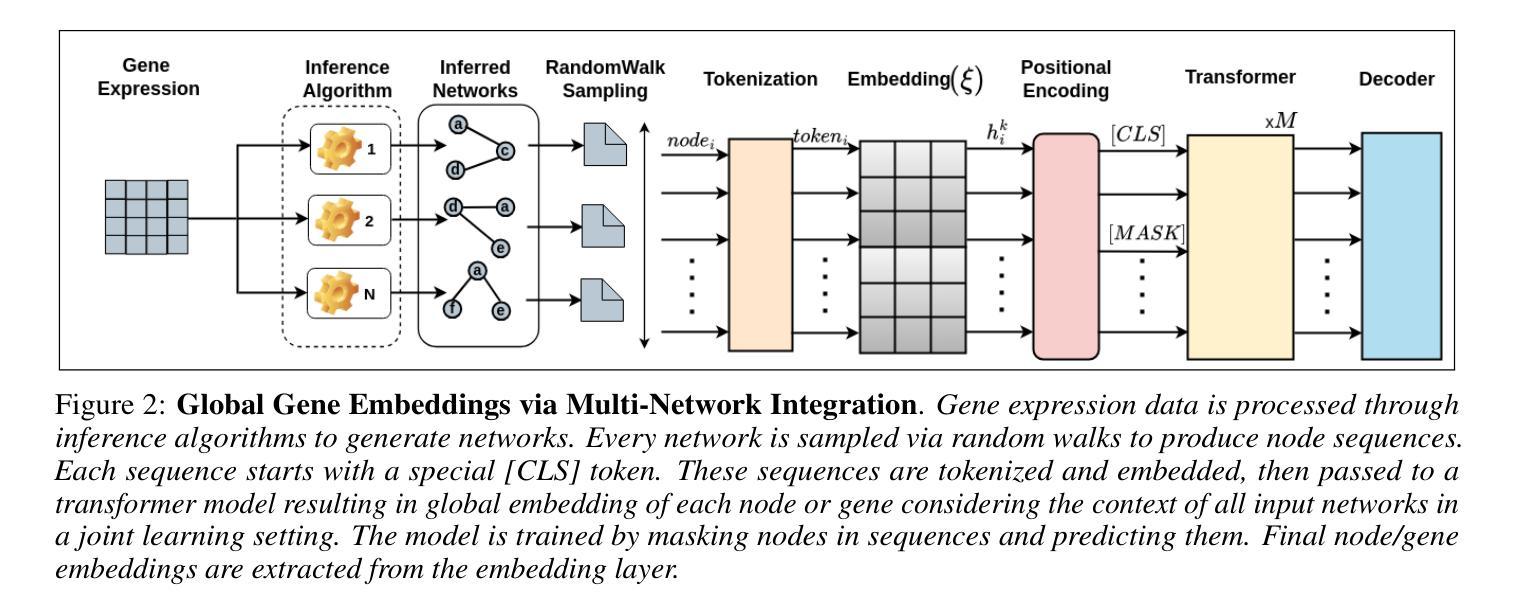
Exploring How LLMs Capture and Represent Domain-Specific Knowledge
Authors:Mirian Hipolito Garcia, Camille Couturier, Daniel Madrigal Diaz, Ankur Mallick, Anastasios Kyrillidis, Robert Sim, Victor Ruhle, Saravan Rajmohan
We study whether Large Language Models (LLMs) inherently capture domain-specific nuances in natural language. Our experiments probe the domain sensitivity of LLMs by examining their ability to distinguish queries from different domains using hidden states generated during the prefill phase. We reveal latent domain-related trajectories that indicate the model’s internal recognition of query domains. We also study the robustness of these domain representations to variations in prompt styles and sources. Our approach leverages these representations for model selection, mapping the LLM that best matches the domain trace of the input query (i.e., the model with the highest performance on similar traces). Our findings show that LLMs can differentiate queries for related domains, and that the fine-tuned model is not always the most accurate. Unlike previous work, our interpretations apply to both closed and open-ended generative tasks
我们研究大型语言模型(LLM)是否天然地捕获自然语言中的特定领域细微差别。我们通过考察预填充阶段生成的隐藏状态来探究LLM对不同领域的查询的区分能力,从而探究其对领域的敏感性。我们揭示了与领域相关的潜在轨迹,表明模型对查询领域的内部识别。我们还研究了这些领域表示对提示风格和来源变化的稳健性。我们的方法利用这些表示为模型选择提供支持,将最佳匹配输入查询领域轨迹的LLM(即在类似轨迹上表现最佳的模型)映射出来。我们的研究结果表明,LLM能够区分相关领域的查询,而且精细调整的模型并不总是最准确的。与以前的工作不同,我们的解释适用于封闭任务和开放式生成任务。
论文及项目相关链接
Summary
大型语言模型(LLM)能否捕捉自然语言中的特定领域细微差别进行了研究。通过实验探究了LLMs在预填充阶段生成的隐藏状态对区分不同领域查询的能力,揭示了模型对查询领域的内部识别轨迹。同时研究了这些领域表征对各种提示风格和来源的稳健性。通过利用这些表征来进行模型选择,找到与输入查询的域轨迹最匹配的LLM(即在类似轨迹上表现最佳的模型)。研究发现LLM能够区分相关领域的查询,并且微调模型并不总是最准确的。与以前的工作不同,我们的解释适用于封闭和开放式的生成任务。
Key Takeaways
- LLMs能够捕捉自然语言中的特定领域细微差别。
- 通过探究LLMs在预填充阶段生成的隐藏状态,揭示了模型对查询领域的内部识别轨迹。
- LLMs能够区分相关领域的查询。
- 模型选择可以通过利用这些领域表征来实现,找到与输入查询的域轨迹最匹配的LLM。
- 即使在类似任务上表现良好的微调模型并不总是最准确的。这一发现对模型选择和任务优化有重要意义。
- 此研究提供的见解适用于不同类型的生成任务,包括封闭式和开放式的任务。这对于在各种任务场景下利用LLM具有指导意义。
点此查看论文截图
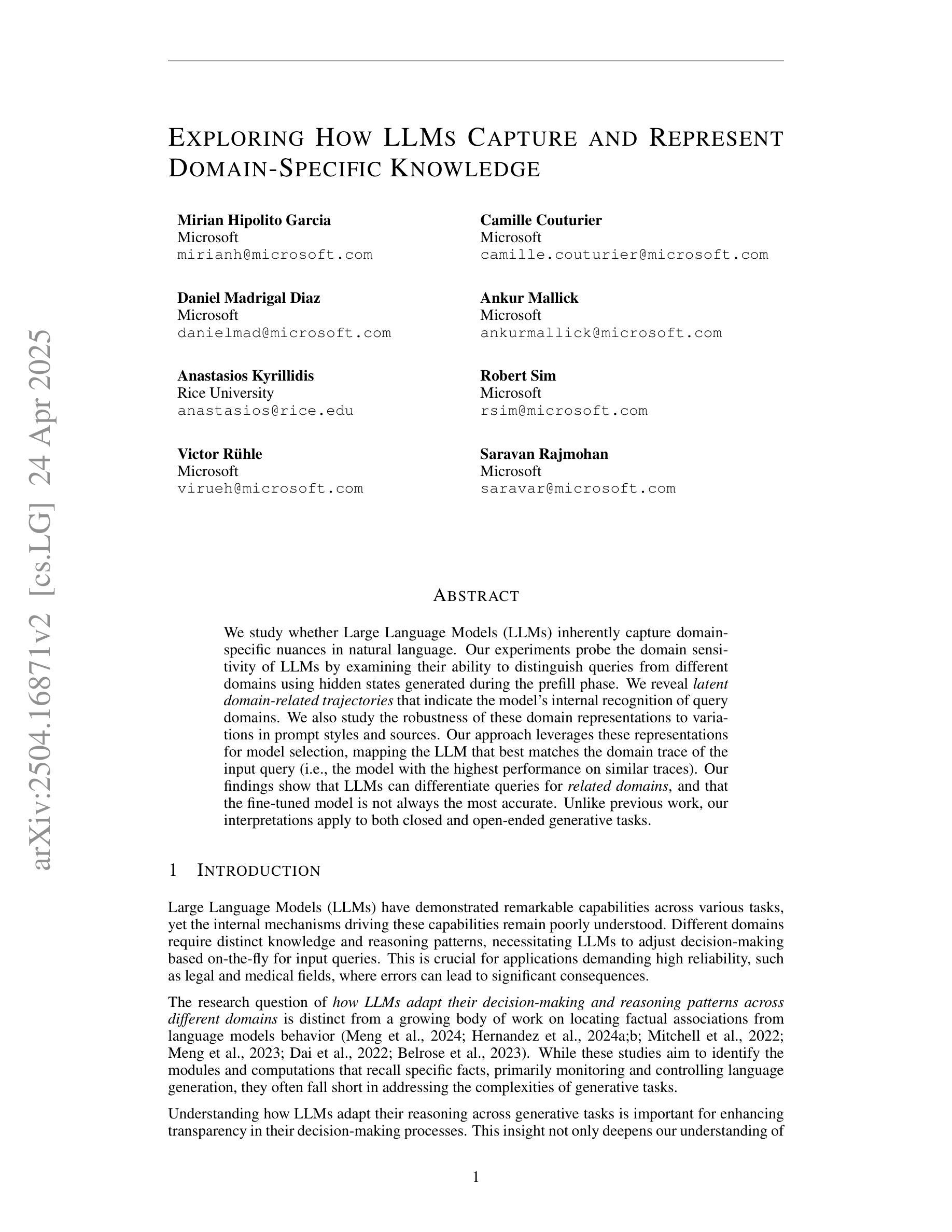
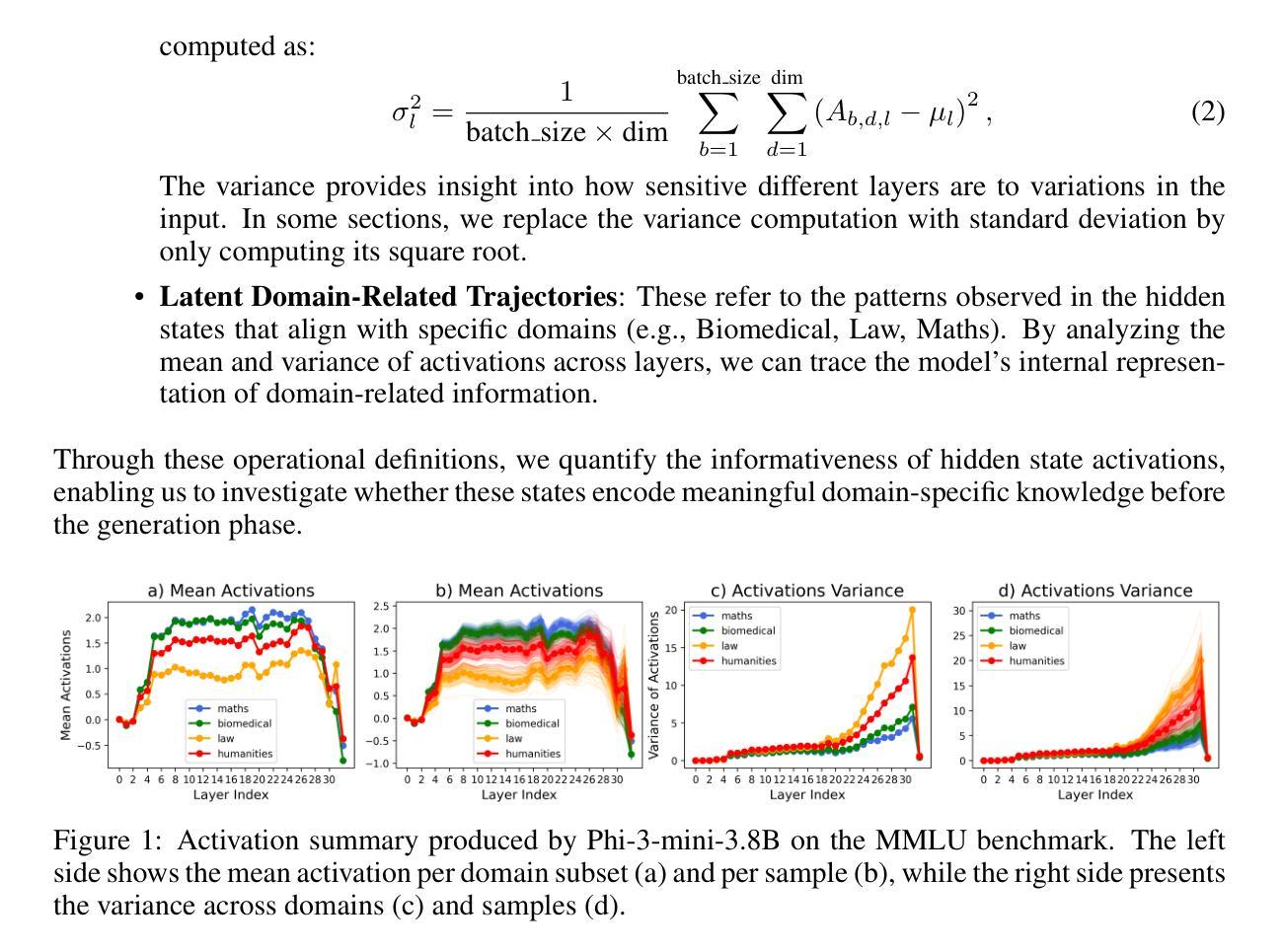
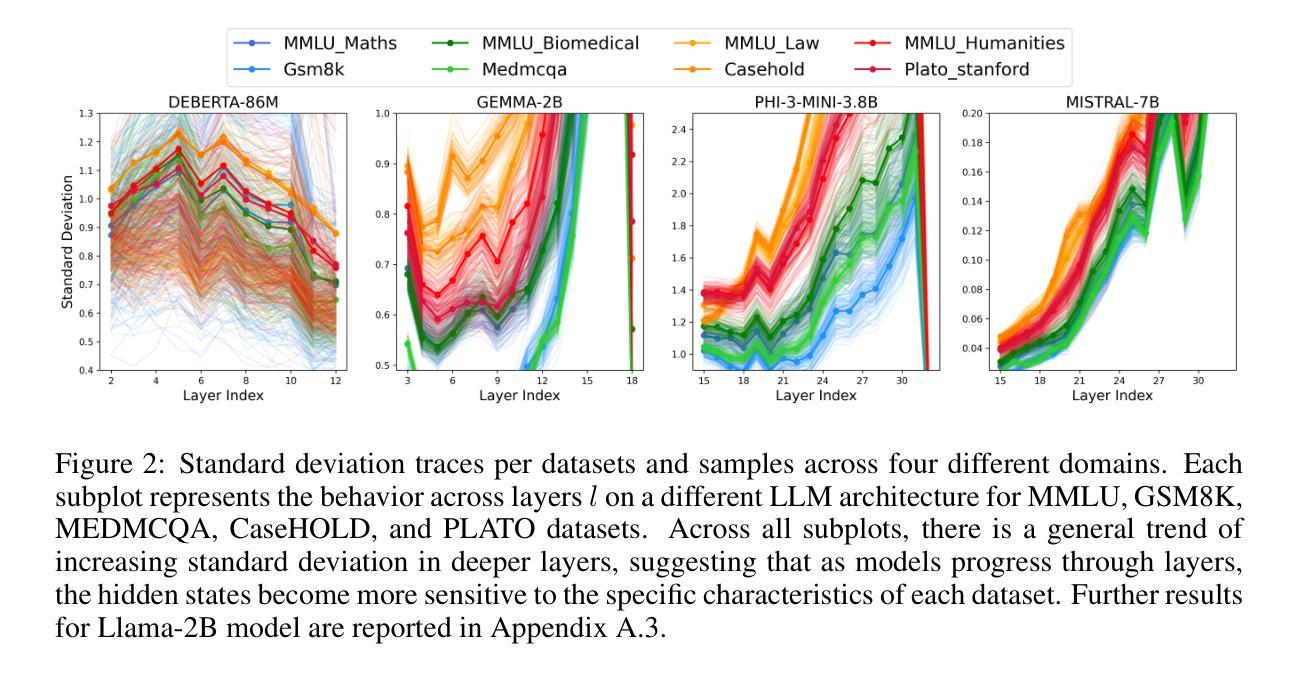
Can Large Language Models Help Multimodal Language Analysis? MMLA: A Comprehensive Benchmark
Authors:Hanlei Zhang, Zhuohang Li, Yeshuang Zhu, Hua Xu, Peiwu Wang, Haige Zhu, Jie Zhou, Jinchao Zhang
Multimodal language analysis is a rapidly evolving field that leverages multiple modalities to enhance the understanding of high-level semantics underlying human conversational utterances. Despite its significance, little research has investigated the capability of multimodal large language models (MLLMs) to comprehend cognitive-level semantics. In this paper, we introduce MMLA, a comprehensive benchmark specifically designed to address this gap. MMLA comprises over 61K multimodal utterances drawn from both staged and real-world scenarios, covering six core dimensions of multimodal semantics: intent, emotion, dialogue act, sentiment, speaking style, and communication behavior. We evaluate eight mainstream branches of LLMs and MLLMs using three methods: zero-shot inference, supervised fine-tuning, and instruction tuning. Extensive experiments reveal that even fine-tuned models achieve only about 60%~70% accuracy, underscoring the limitations of current MLLMs in understanding complex human language. We believe that MMLA will serve as a solid foundation for exploring the potential of large language models in multimodal language analysis and provide valuable resources to advance this field. The datasets and code are open-sourced at https://github.com/thuiar/MMLA.
多模态语言分析是一个快速发展的领域,它利用多种模态增强对人类会话表述中高级语义的理解。尽管这一领域具有重要意义,但关于多模态大型语言模型(MLLMs)在理解认知级语义方面的能力的研究却很少。在本文中,我们介绍了MMLA,这是一个专门设计用于解决这一差距的综合基准测试。MMLA包含来自舞台和真实世界场景的超过6.1万条多模态表述,涵盖多模态语义的六个核心维度:意图、情感、对话行为、情感倾向、说话风格和沟通行为。我们使用三种方法:零样本推理、监督微调、指令微调,评估了八个主流的大型语言模型和多模态大型语言模型分支。大量实验表明,即使经过精细训练的模型也只能达到约60%~70%的准确率,突显出当前多模态大型语言模型在理解复杂人类语言方面的局限性。我们相信,MMLA将作为探索大型语言模型在多模态语言分析潜力的重要基础,并为推动这一领域的发展提供宝贵的资源。数据集和代码已开源在https://github.com/thuiar/MMLA。
论文及项目相关链接
PDF 23 pages, 5 figures
Summary
本文介绍了多模态语言分析领域的一个综合性基准测试MMLA,旨在评估多模态大型语言模型(MLLMs)对认知级语义的理解能力。MMLA包含超过61K个来自舞台和真实场景的多模态话语,涵盖意图、情感、对话行为等六个核心维度的多模态语义。实验表明,当前MLLMs在理解复杂人类语言方面存在局限性,即使经过精细训练的模型也只能达到约60%~70%的准确率。本文认为MMLA将为探索大型语言模型在多模态语言分析中的潜力提供坚实基础,并为推动该领域发展提供宝贵资源。数据集和代码已开源。
Key Takeaways
- 多模态语言分析是一个利用多种模式增强对人类会话中高层语义理解的新兴领域。
- MMLA基准测试旨在评估多模态大型语言模型(MLLMs)对认知级语义的理解能力。
- MMLA包含来自舞台和真实场景的多模态话语,涵盖多个核心维度的多模态语义。
- 实验表明当前MLLMs在理解复杂人类语言方面存在局限性。
- MMLA为探索大型语言模型在多模态语言分析中的潜力提供了坚实基础。
- 数据集和代码已开源,有助于推动该领域的发展。
点此查看论文截图
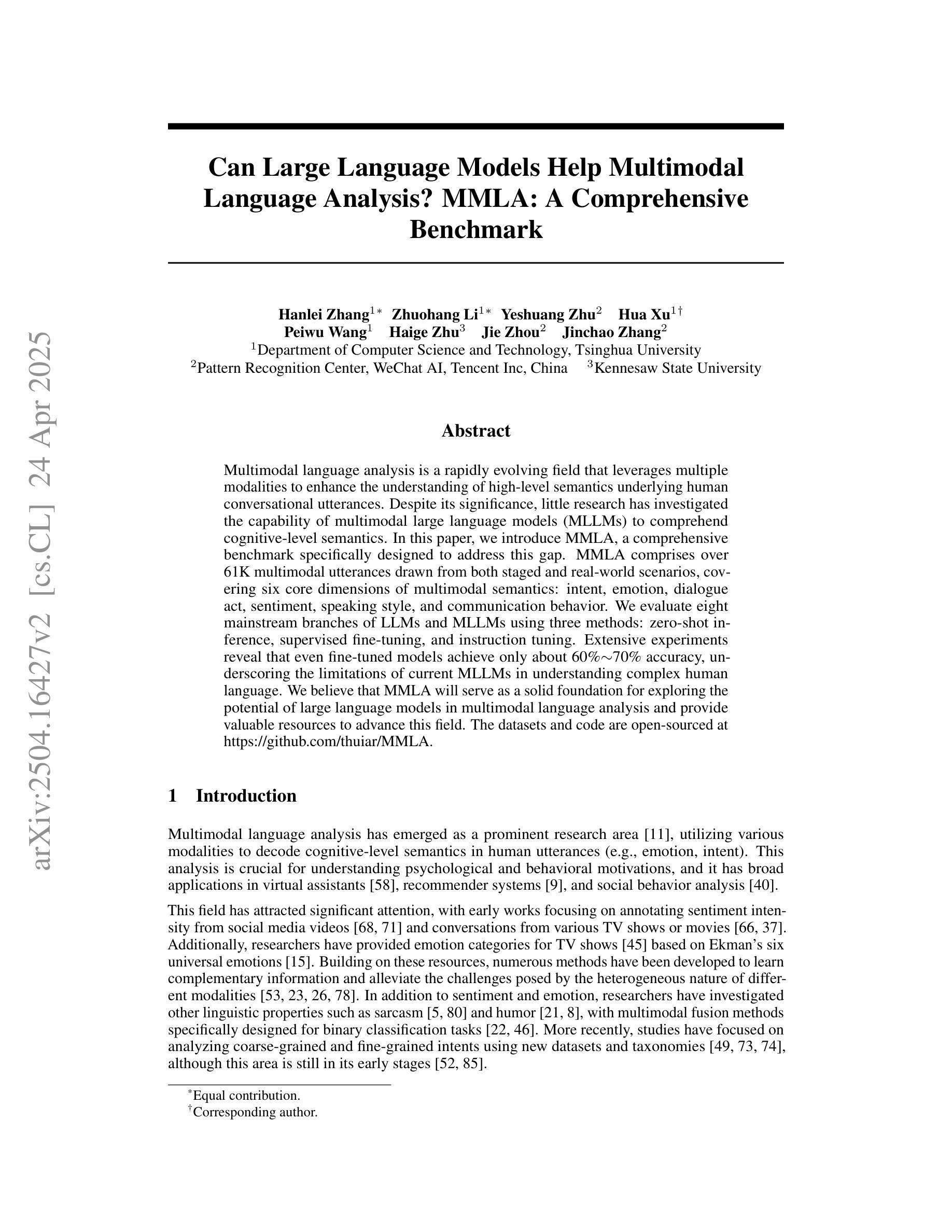
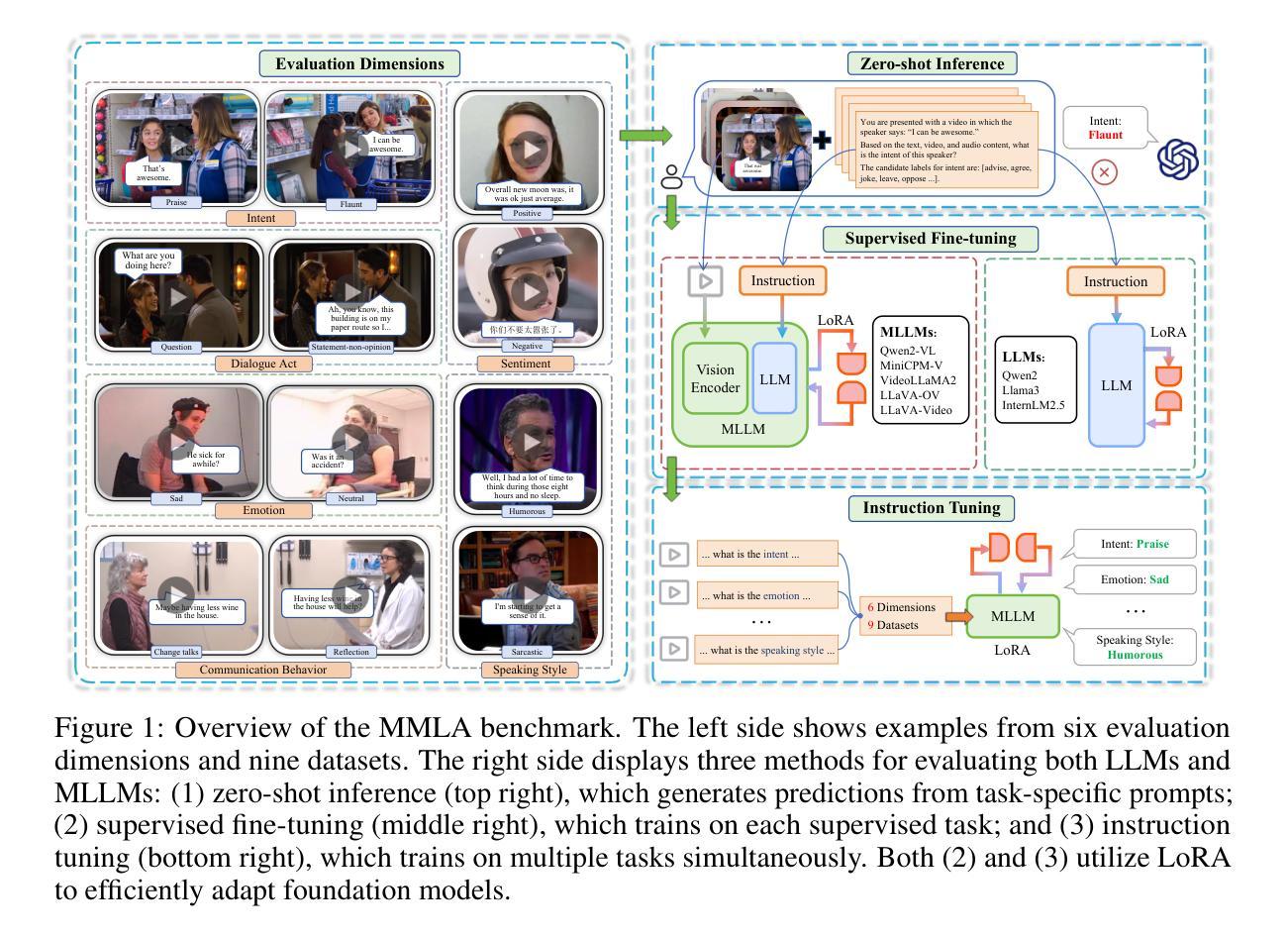
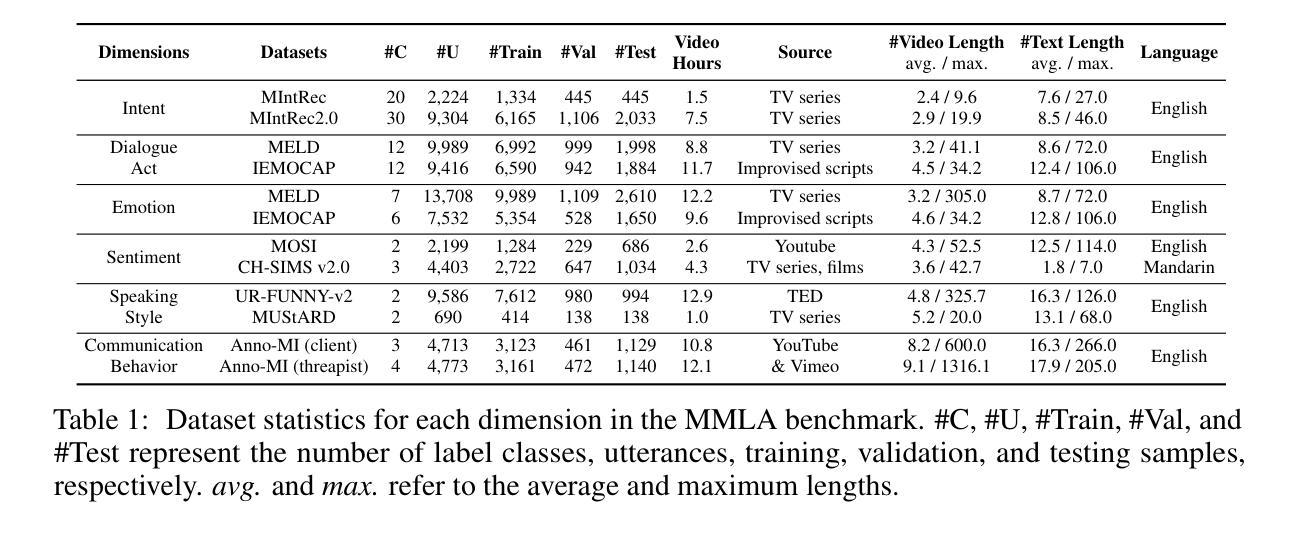
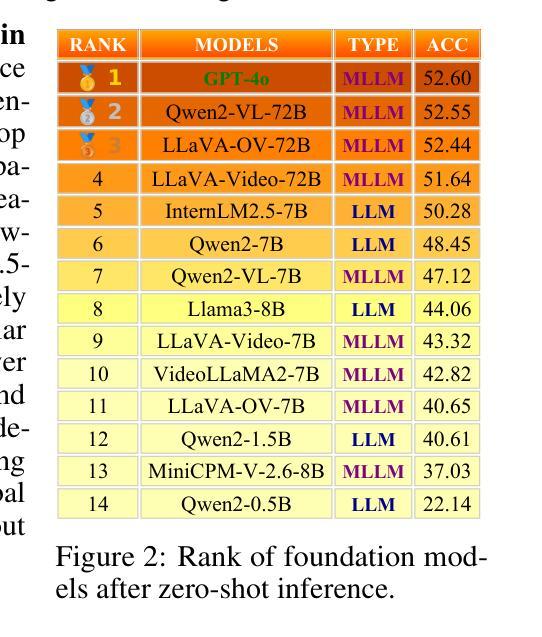
EditLord: Learning Code Transformation Rules for Code Editing
Authors:Weichen Li, Albert Jan, Baishakhi Ray, Chengzhi Mao, Junfeng Yang, Kexin Pei
Code editing is a foundational task in software development, where its effectiveness depends on whether it introduces desired code property changes without changing the original code’s intended functionality. Existing approaches often formulate code editing as an implicit end-to-end task, omitting the fact that code-editing procedures inherently consist of discrete and explicit steps. Thus, they suffer from suboptimal performance and lack of robustness and generalization. We introduce EditLord, a code editing framework that makes the code transformation steps explicit. Our key insight is to employ a language model (LM) as an inductive learner to extract code editing rules from the training code pairs as concise meta-rule sets. Such rule sets will be manifested for each training sample to augment them for finetuning or assist in prompting- and iterative-based code editing. EditLordoutperforms the state-of-the-art by an average of 22.7% in editing performance and 58.1% in robustness while achieving 20.2% higher functional correctness across critical software engineering and security applications, LM models, and editing modes.
代码编辑是软件开发中的基础任务,其有效性取决于是否在不改变原始代码预期功能的情况下引入了所需的代码属性更改。现有方法通常将代码编辑制定为隐式的端到端任务,忽略了代码编辑过程本质上包含离散和明确步骤这一事实。因此,它们面临性能不佳、缺乏稳健性和泛化能力的问题。我们引入了EditLord,这是一个使代码转换步骤明确的代码编辑框架。我们的关键见解是,采用语言模型(LM)作为归纳学习者,从训练代码对中提取简洁的元规则集作为代码编辑规则。针对每个训练样本显示此类规则集,以增强它们进行微调或辅助基于提示和迭代的代码编辑。EditLord在编辑性能上平均比现有技术高出22.7%,在稳健性上高出58.1%,同时在关键软件工程和安全应用程序、语言模型以及编辑模式方面实现了20.2%更高的功能正确性。
论文及项目相关链接
摘要
代码编辑是软件开发中的基础任务,其有效性取决于是否在改变原始代码意图功能的同时引入所需的代码属性更改。现有方法通常将代码编辑制定为隐式的端到端任务,忽略了代码编辑过程本质上包含离散和明确的步骤。因此,它们存在性能不佳、缺乏稳健性和泛化能力的问题。我们引入了EditLord代码编辑框架,使代码转换步骤明确。我们的关键见解是,采用语言模型(LM)作为归纳学习者,从训练代码对中提取代码编辑规则,形成简洁的元规则集。这些规则集将为每个训练样本提供辅助微调或辅助提示和迭代式代码编辑。EditLord在编辑性能、稳健性和功能正确性方面均优于现有技术,在关键软件工程和安全应用程序、语言模型和编辑模式方面平均提高了22.7%、58.1%和20.2%。
要点摘要
- 代码编辑是软件开发中的核心任务,要求在不改变原始代码意图功能的前提下引入所需的代码属性更改。
- 现有方法将代码编辑视为隐式的端到端任务,忽略了其固有的离散和明确步骤,导致性能不佳和缺乏稳健性。
- EditLord框架旨在使代码转换步骤明确,通过采用语言模型提取代码编辑规则来提高性能。
- 语言模型作为归纳学习者,从训练代码对中提取简洁的元规则集,为每个训练样本提供辅助。
- EditLord通过明确的编辑步骤和元规则集的应用,显著提高了编辑性能、稳健性和功能正确性。
- 在关键软件工程和安全应用程序方面,EditLord相较于现有技术平均提升了22.7%的编辑性能、58.1%的稳健性和20.2%的功能正确性。
点此查看论文截图
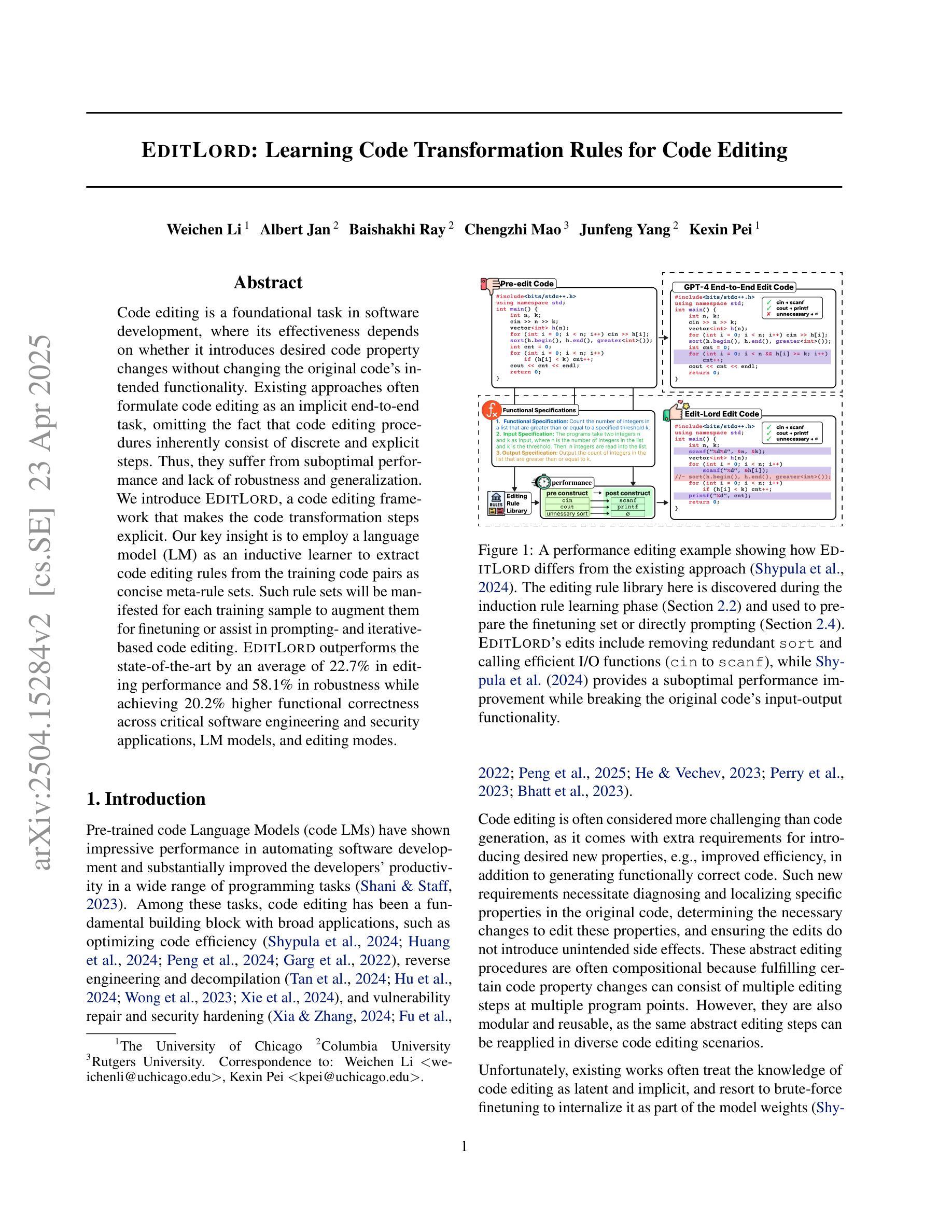
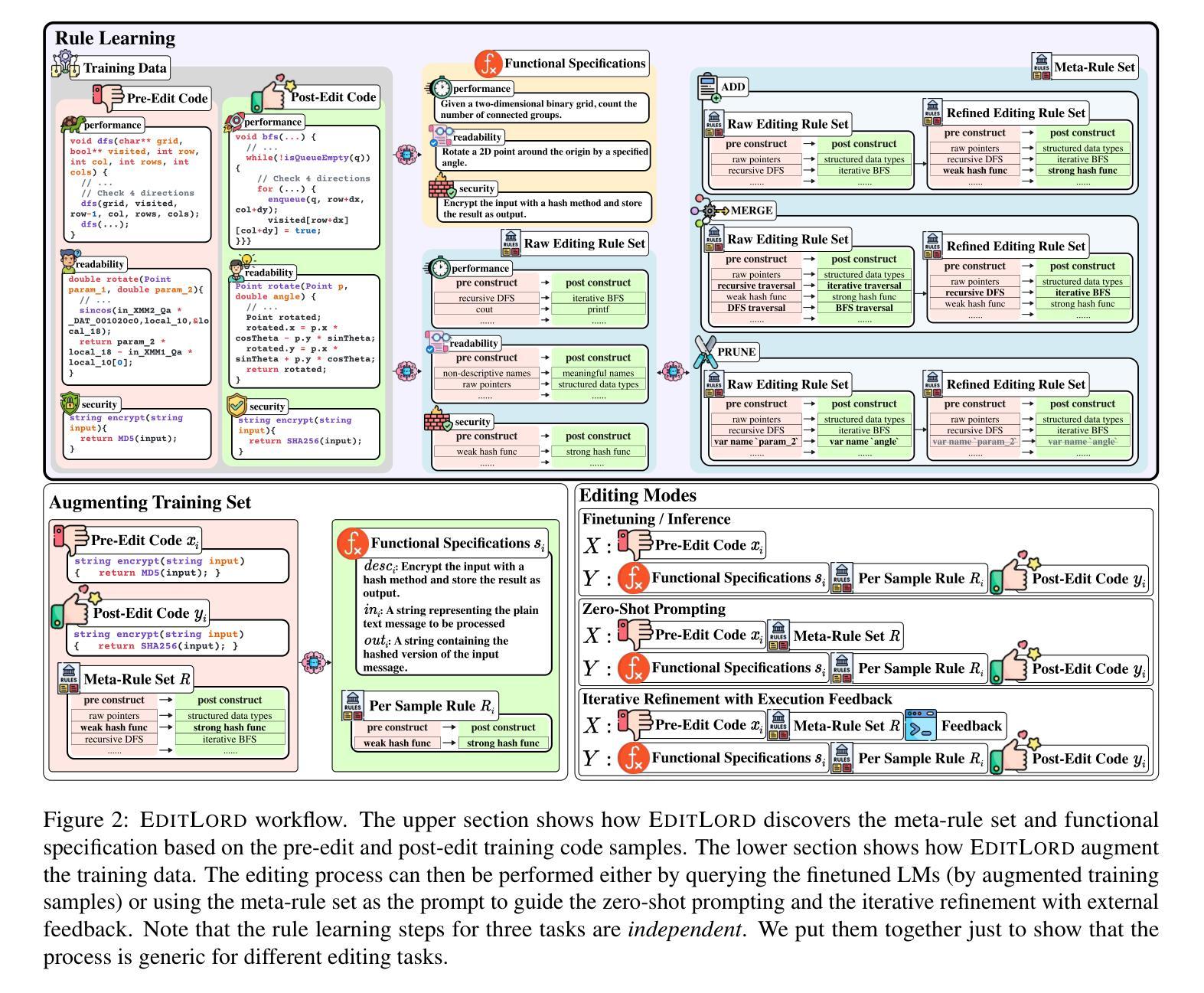
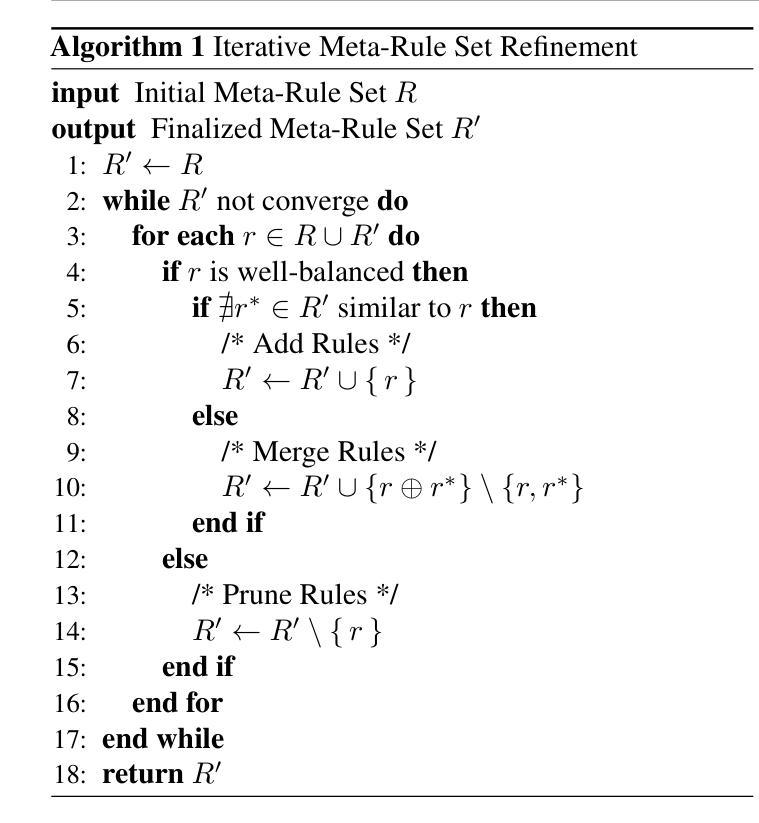
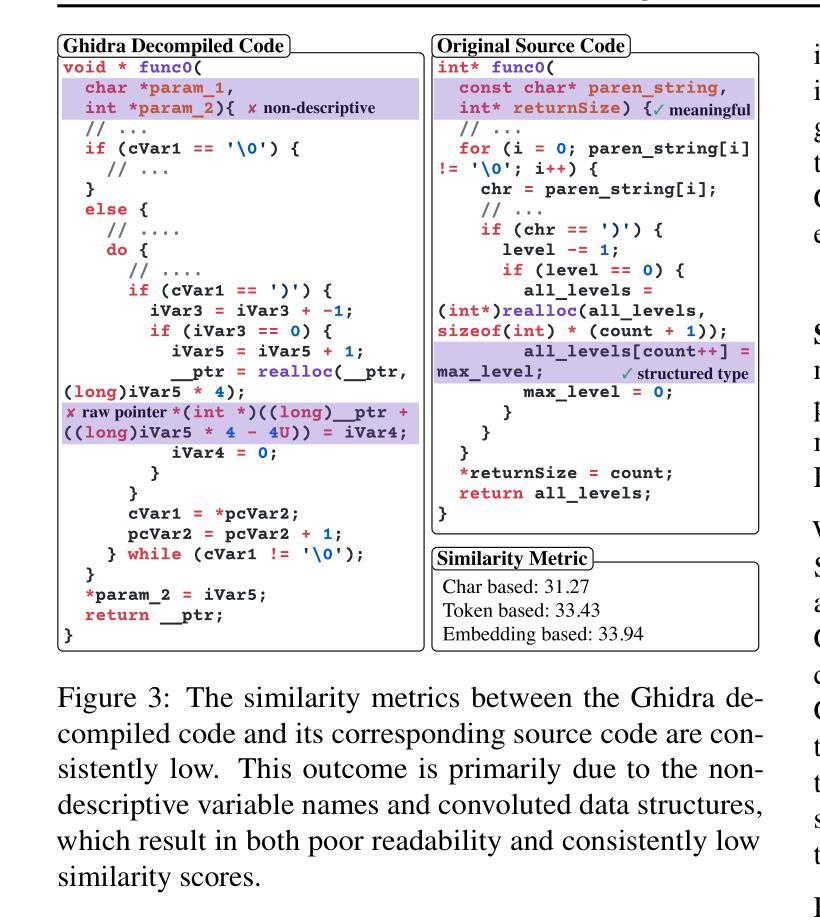
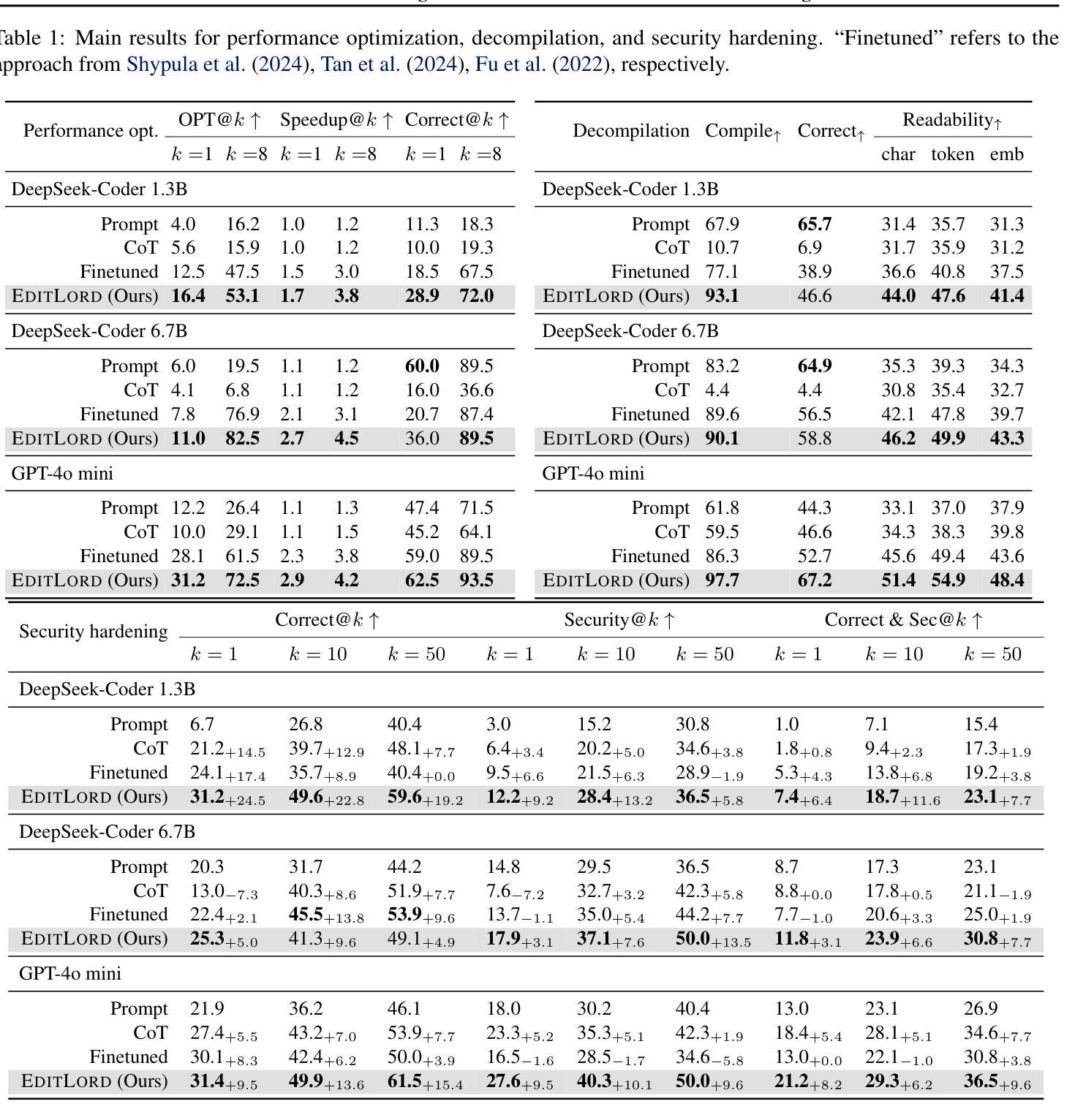
Transferable text data distillation by trajectory matching
Authors:Rong Yao, Hailin Hu, Yifei Fu, Hanting Chen, Wenyi Fang, Fanyi Du, Kai Han, Yunhe Wang
In the realm of large language model (LLM), as the size of large models increases, it also brings higher training costs. There is a urgent need to minimize the data size in LLM training. Compared with data selection method, the data distillation method aims to synthesize a small number of data samples to achieve the training effect of the full data set and has better flexibility. Despite its successes in computer vision, the discreteness of text data has hitherto stymied its exploration in natural language processing (NLP). In this work, we proposed a method that involves learning pseudo prompt data based on trajectory matching and finding its nearest neighbor ID to achieve cross-architecture transfer. During the distillation process, we introduce a regularization loss to improve the robustness of our distilled data. To our best knowledge, this is the first data distillation work suitable for text generation tasks such as instruction tuning. Evaluations on two benchmarks, including ARC-Easy and MMLU instruction tuning datasets, established the superiority of our distillation approach over the SOTA data selection method LESS. Furthermore, our method demonstrates a good transferability over LLM structures (i.e., OPT to Llama).
在大型语言模型(LLM)领域,随着大型模型规模的增加,其训练成本也随之提高。因此,迫切需要对大型语言模型训练所需的数据量进行缩减。相较于数据选择方法,数据蒸馏方法旨在合成少量数据样本以实现全数据集的培训效果,并且具有更好的灵活性。尽管其在计算机视觉领域已经取得了成功,但文本数据的离散性迄今为止阻碍了其在自然语言处理(NLP)中的探索。
论文及项目相关链接
Summary
大型语言模型(LLM)的训练成本随着模型规模的增大而增加,迫切需要减小训练数据的大小。相较于数据选择方法,数据蒸馏方法可以合成少量数据样本以实现全数据集的培训效果,具有更好的灵活性。尽管在计算机视觉领域取得了成功,文本数据的离散性阻碍了其在自然语言处理(NLP)领域的应用探索。本研究提出了一种基于轨迹匹配学习伪提示数据的方法,通过寻找最近邻ID实现跨架构迁移。在蒸馏过程中,引入正则化损失以提高蒸馏数据的稳健性。据我们所知,这是首个适用于指令调整等文本生成任务的数据蒸馏工作。在ARC-Easy和MMLU指令调整数据集上的评估表明,我们的蒸馏方法优于现有最佳的数据选择方法LESS,并且在LLM结构之间具有良好的可迁移性(例如,从OPT到Llama)。
Key Takeaways
- 大型语言模型(LLM)的训练成本高昂,存在对减小训练数据大小的需求。
- 数据蒸馏是一种可以合成少量数据样本以实现全数据集培训效果的方法,具有灵活性。
- 尽管数据蒸馏在计算机视觉领域有所成就,但在自然语言处理(NLP)中的应用仍面临文本数据离散性的挑战。
- 本研究通过轨迹匹配学习伪提示数据的方法,实现跨架构迁移。
- 在蒸馏过程中引入正则化损失以提高数据的稳健性。
- 该研究提出的蒸馏方法优于现有的数据选择方法,如在ARC-Easy和MMLU指令调整数据集上的表现。
点此查看论文截图
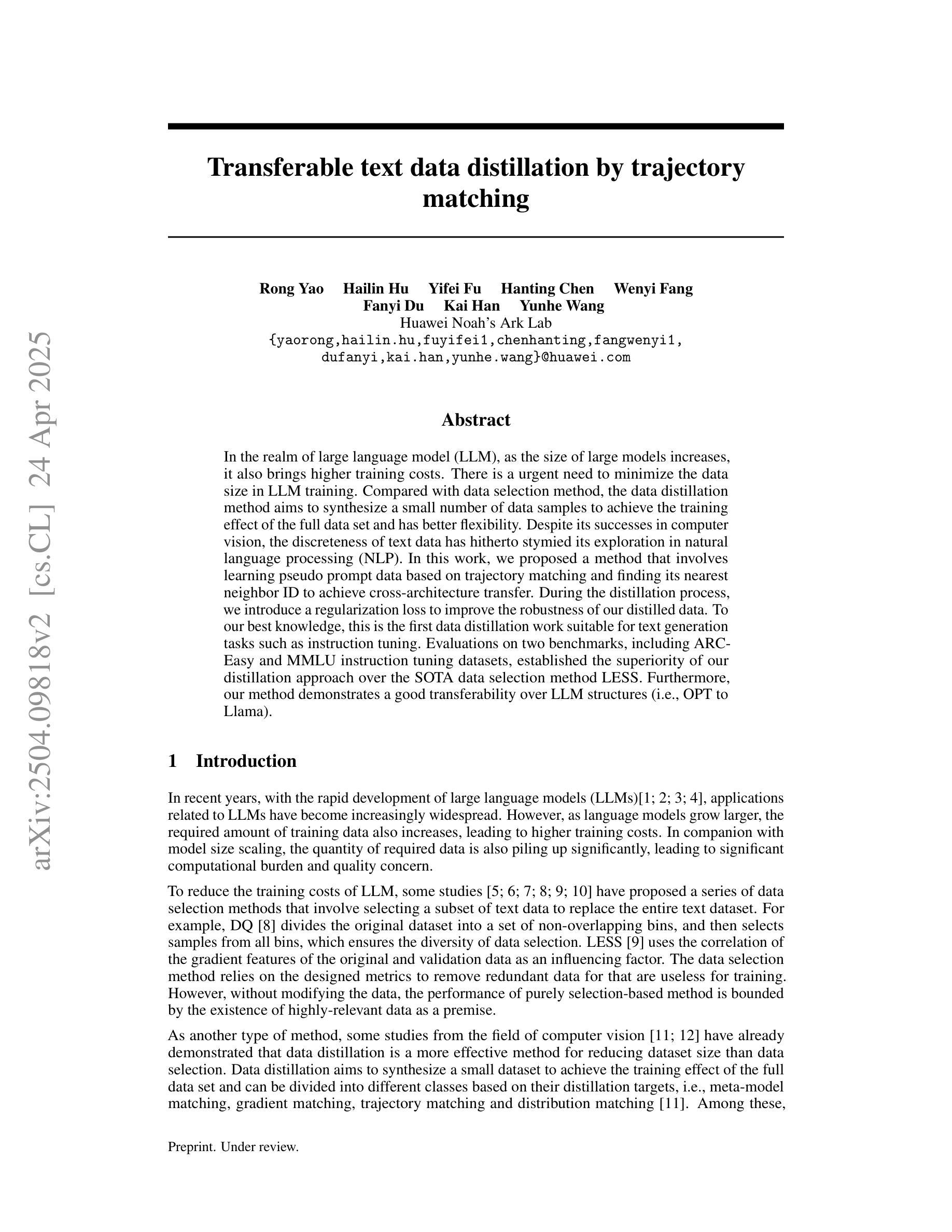
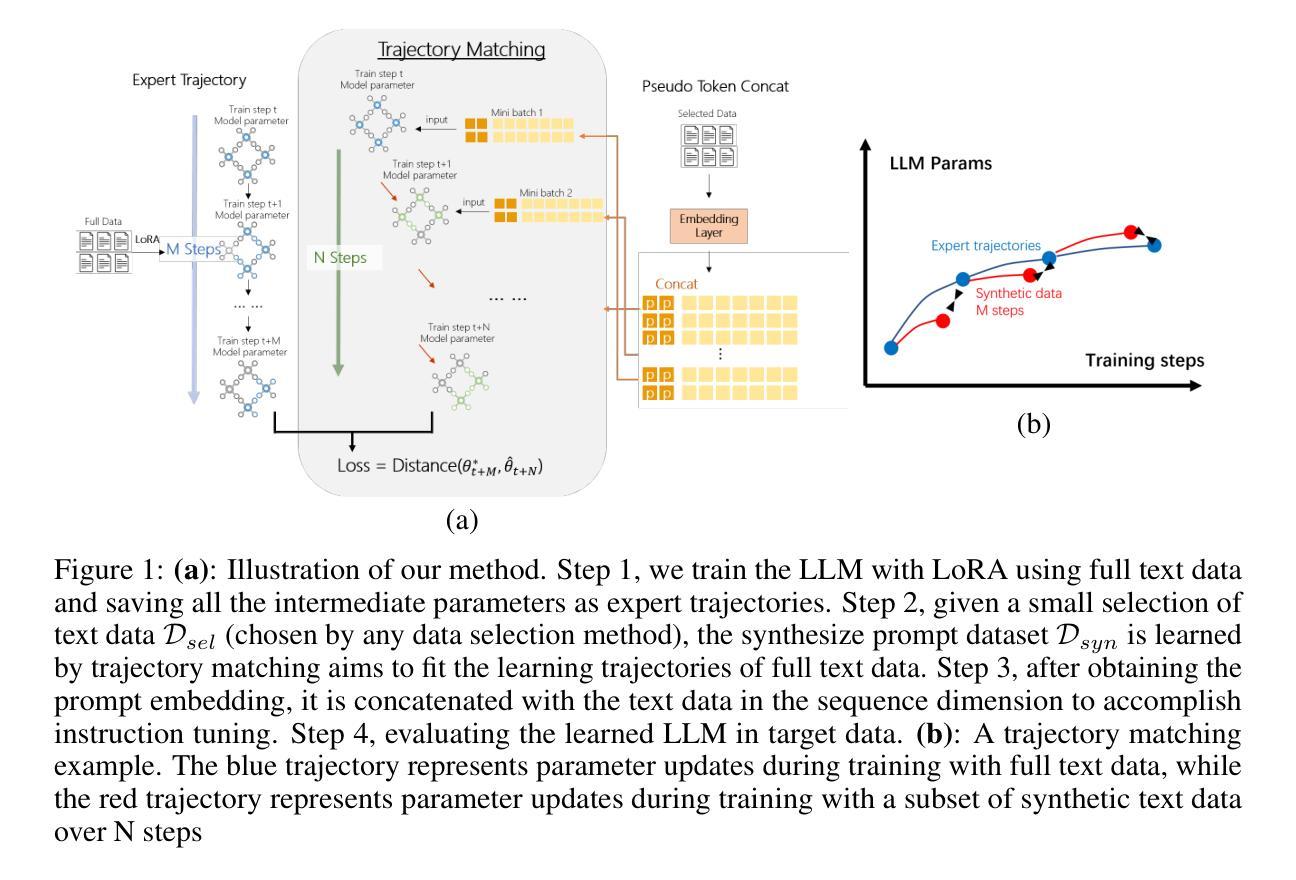
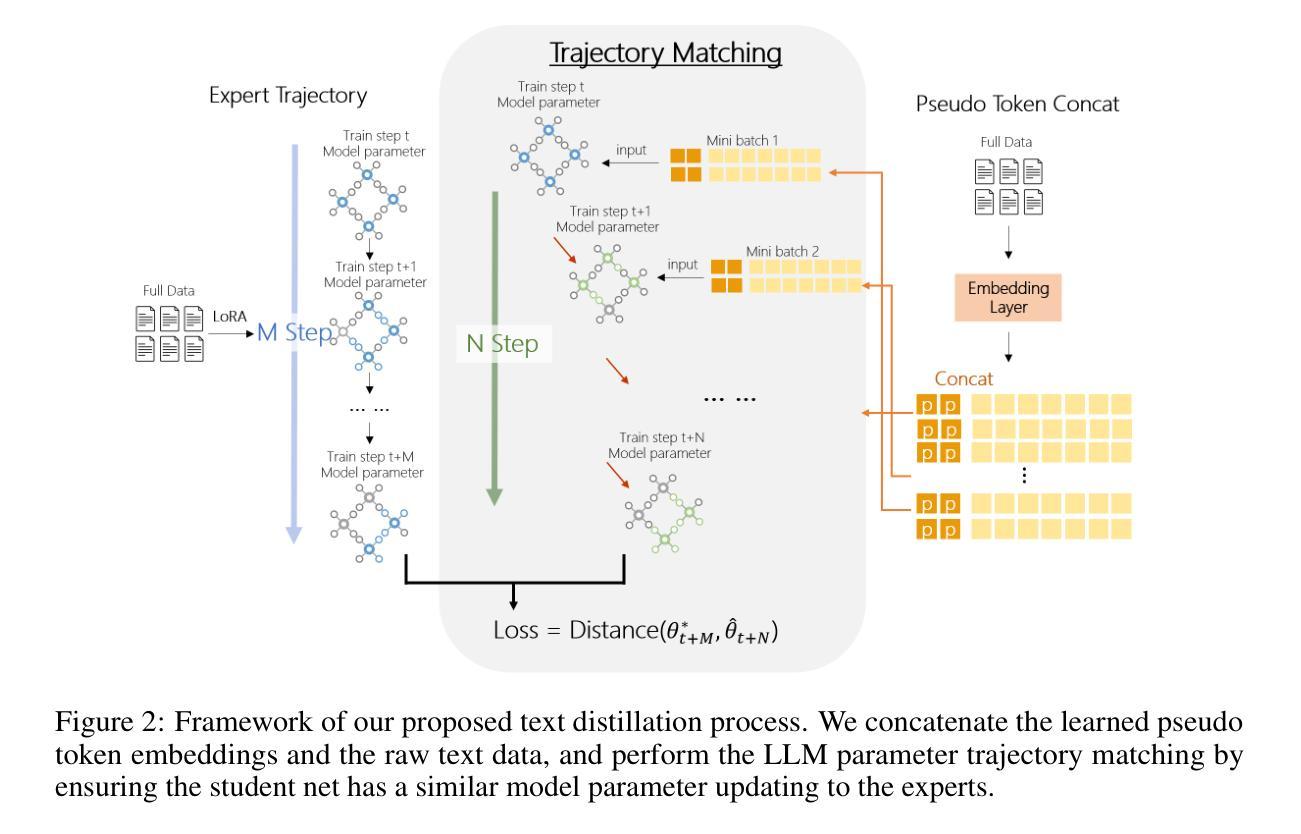
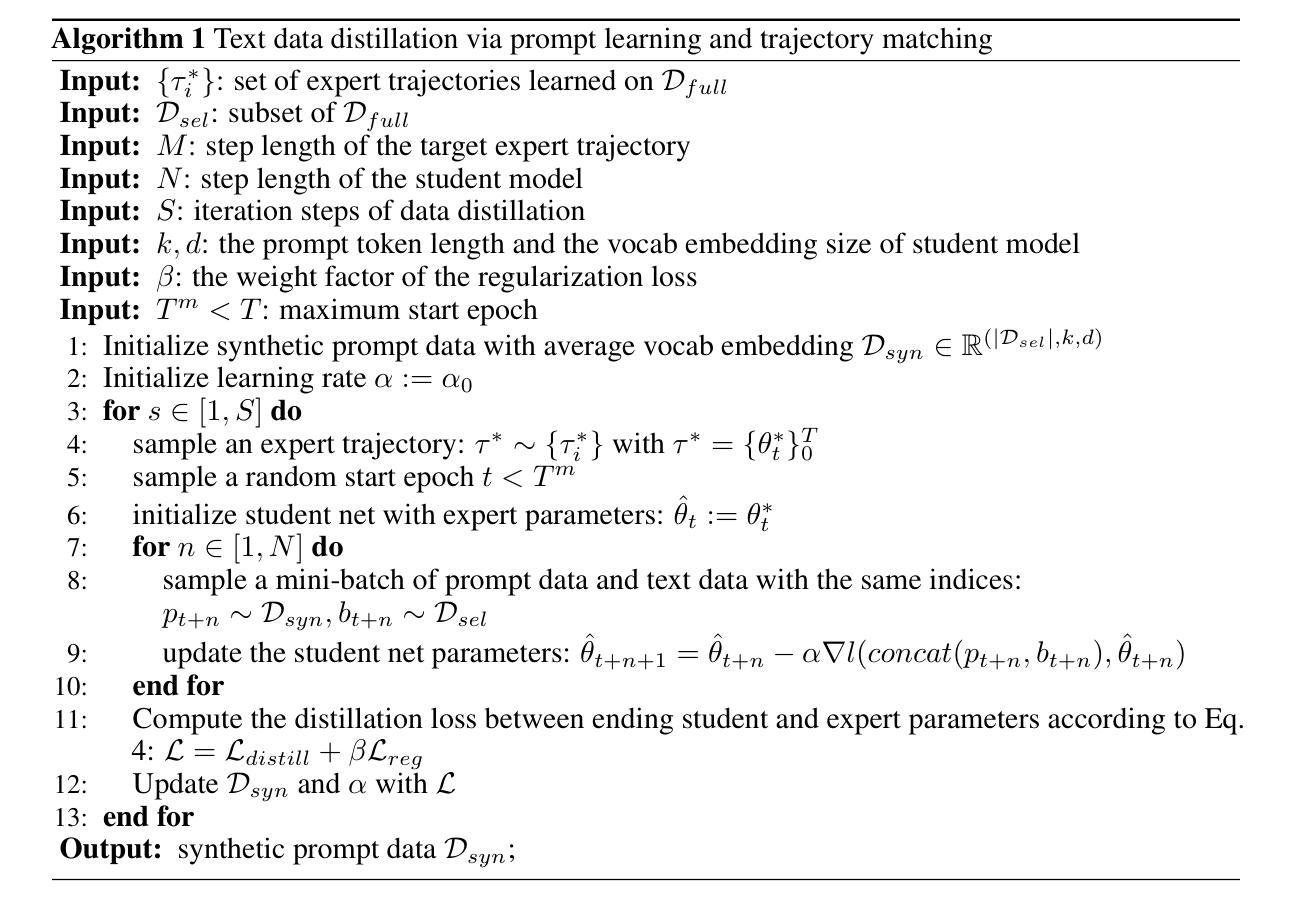
AudioX: Diffusion Transformer for Anything-to-Audio Generation
Authors:Zeyue Tian, Yizhu Jin, Zhaoyang Liu, Ruibin Yuan, Xu Tan, Qifeng Chen, Wei Xue, Yike Guo
Audio and music generation have emerged as crucial tasks in many applications, yet existing approaches face significant limitations: they operate in isolation without unified capabilities across modalities, suffer from scarce high-quality, multi-modal training data, and struggle to effectively integrate diverse inputs. In this work, we propose AudioX, a unified Diffusion Transformer model for Anything-to-Audio and Music Generation. Unlike previous domain-specific models, AudioX can generate both general audio and music with high quality, while offering flexible natural language control and seamless processing of various modalities including text, video, image, music, and audio. Its key innovation is a multi-modal masked training strategy that masks inputs across modalities and forces the model to learn from masked inputs, yielding robust and unified cross-modal representations. To address data scarcity, we curate two comprehensive datasets: vggsound-caps with 190K audio captions based on the VGGSound dataset, and V2M-caps with 6 million music captions derived from the V2M dataset. Extensive experiments demonstrate that AudioX not only matches or outperforms state-of-the-art specialized models, but also offers remarkable versatility in handling diverse input modalities and generation tasks within a unified architecture. The code and datasets will be available at https://zeyuet.github.io/AudioX/
音频和音乐生成在许多应用中已成为至关重要的任务,然而现有方法面临重大局限:它们在孤立环境中运行,无法跨模态统一能力,缺乏高质量的多模态训练数据,并且难以有效整合各种输入。在这项工作中,我们提出了AudioX,这是一个统一的扩散转换器模型,用于任何内容转音频和音乐生成。不同于以前的特定领域模型,AudioX可以高质量地生成通用音频和音乐,同时提供灵活的自然语言控制,无缝处理各种模态,包括文本、视频、图像、音乐和音频。其关键创新之处在于多模态掩膜训练策略,该策略会屏蔽跨模态的输入,并迫使模型从被屏蔽的输入中学习,从而产生稳健和统一的跨模态表示。为了解决数据稀缺问题,我们筛选了两个综合数据集:基于VGGSound数据集的19万条音频字幕的vggsound-caps,以及从V2M数据集中派生的600万条音乐字幕的V2M-caps。大量实验表明,AudioX不仅与最先进的专用模型相匹配或表现更佳,而且在处理各种输入模态和生成任务方面表现出卓越的通用性。代码和数据集将在[https://zeyuet.github.io/AudioX/]上提供。
论文及项目相关链接
PDF The code and datasets will be available at https://zeyuet.github.io/AudioX/
Summary
本工作提出了AudioX,一个统一的扩散Transformer模型,用于任何内容生成音频和音乐。该模型可高质量生成通用音频和音乐,支持灵活的自然语言控制,并能无缝处理多种模态,包括文本、视频、图像、音乐和音频。其核心创新在于多模态掩膜训练策略,通过跨模态输入掩膜,迫使模型从掩膜输入中学习,产生稳健统一的跨模态表示。为解决数据稀缺问题,我们创建了两个大型数据集:基于VGGSound数据集的19万音频字幕的vggsound-caps和从V2M数据集中派生的6百万音乐字幕的V2M-caps。实验表明,AudioX不仅与最先进的特定模型相匹配或表现更佳,而且在一个统一架构中处理各种输入模态和生成任务时表现出卓越的多功能性。
Key Takeaways
- AudioX是一个统一的扩散Transformer模型,用于任何内容生成音频和音乐。
- 可生成高质量通用音频和音乐,并支持灵活的自然语言控制。
- 无缝处理多种模态,包括文本、视频、图像、音乐和音频。
- 核心创新在于多模态掩膜训练策略,产生稳健统一的跨模态表示。
- 解决数据稀缺问题,创建了两个大型数据集:vggsound-caps和V2M-caps。
- 实验表明AudioX性能优越,与最先进的特定模型相匹配或表现更佳。
点此查看论文截图
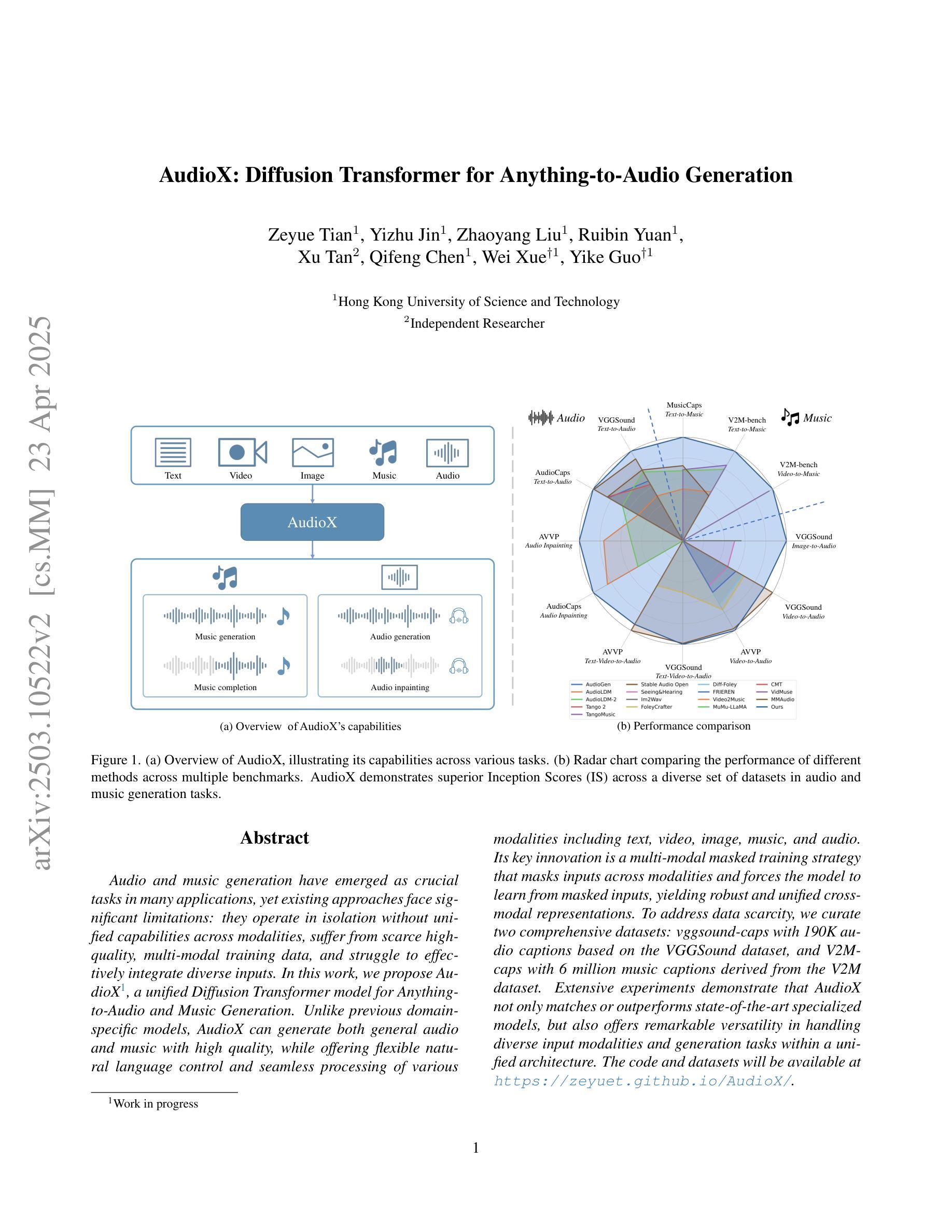
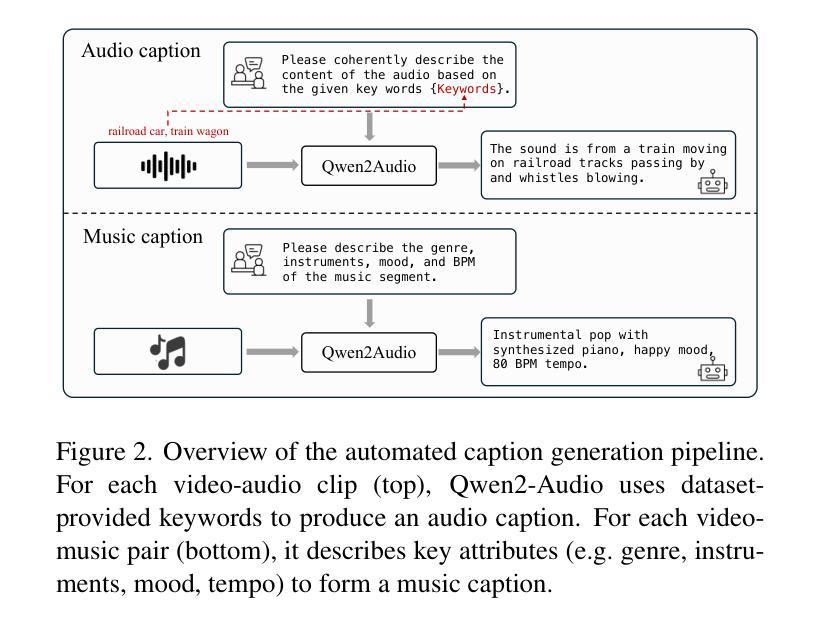
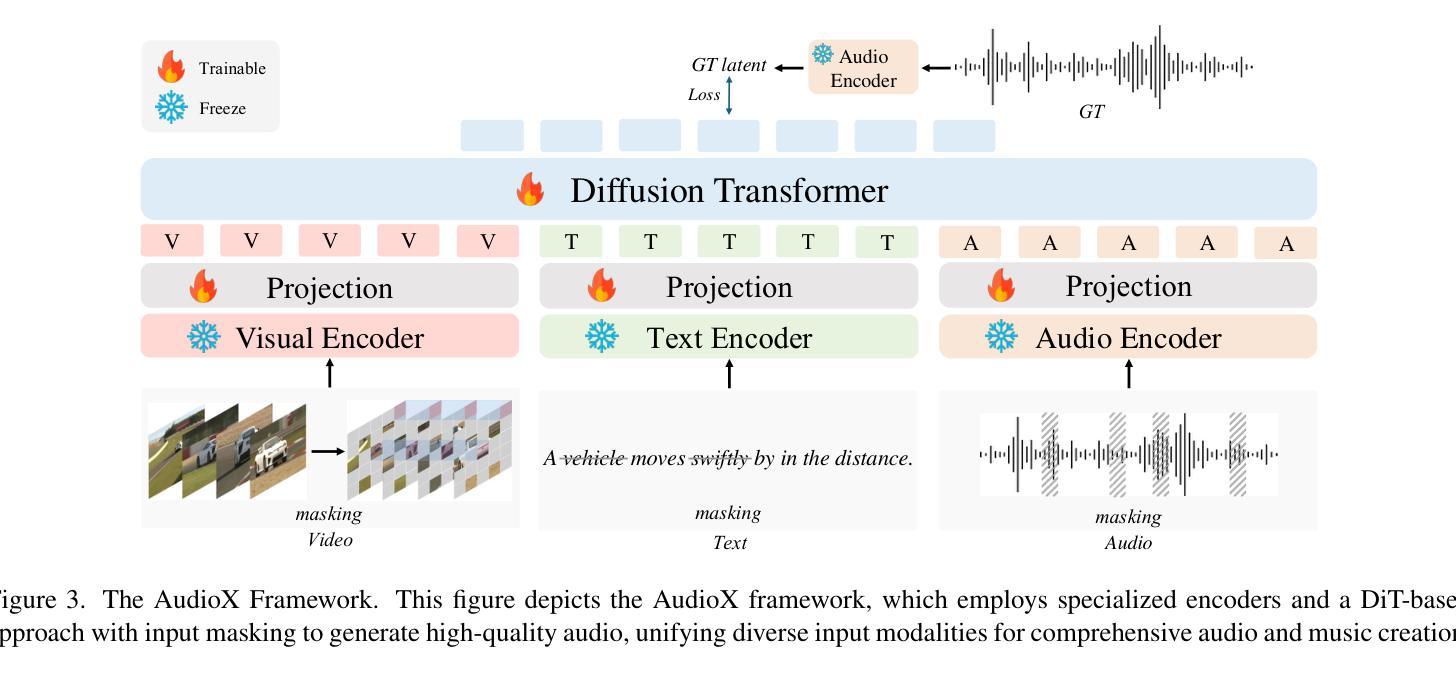
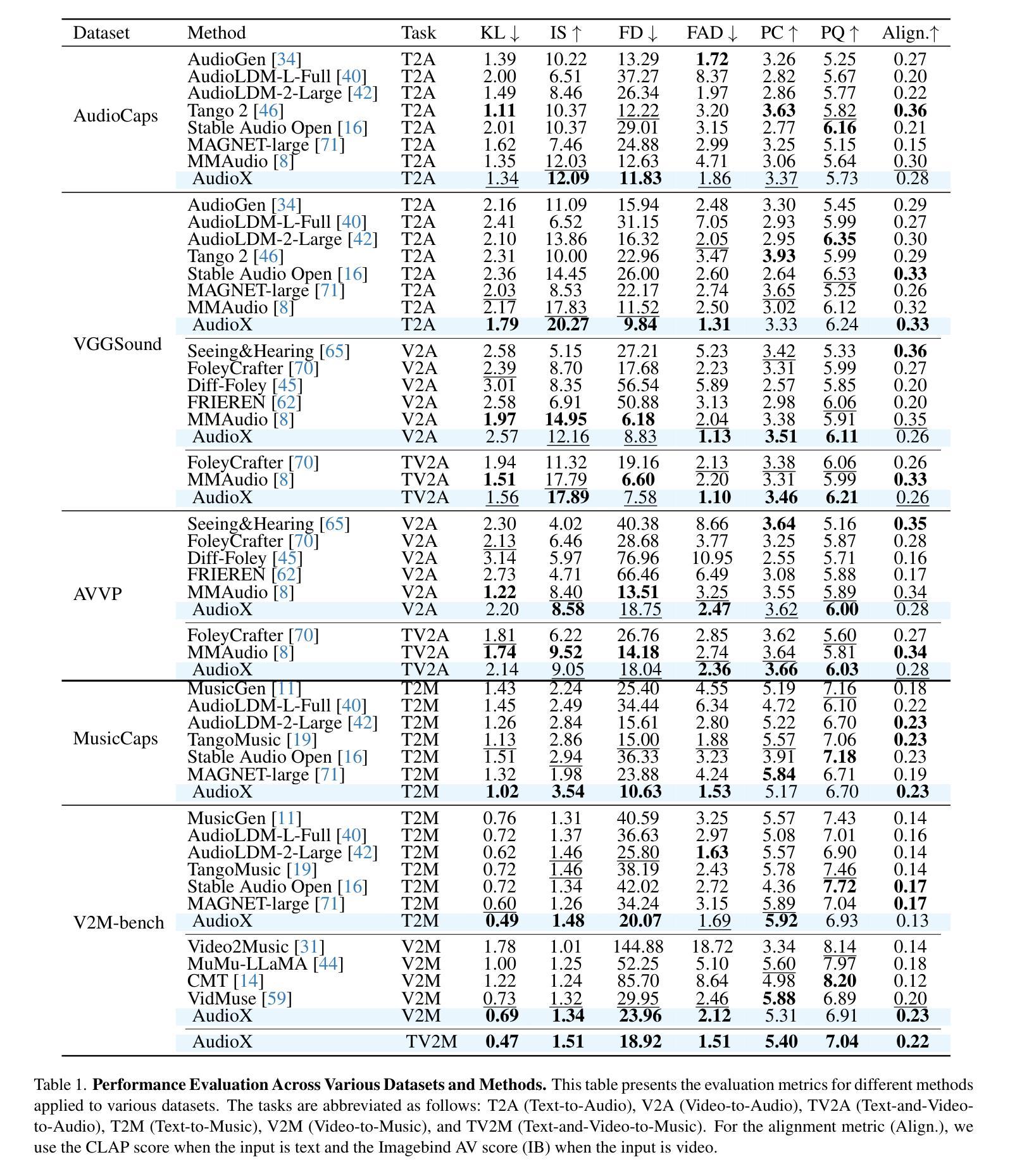
HierarQ: Task-Aware Hierarchical Q-Former for Enhanced Video Understanding
Authors:Shehreen Azad, Vibhav Vineet, Yogesh Singh Rawat
Despite advancements in multimodal large language models (MLLMs), current approaches struggle in medium-to-long video understanding due to frame and context length limitations. As a result, these models often depend on frame sampling, which risks missing key information over time and lacks task-specific relevance. To address these challenges, we introduce HierarQ, a task-aware hierarchical Q-Former based framework that sequentially processes frames to bypass the need for frame sampling, while avoiding LLM’s context length limitations. We introduce a lightweight two-stream language-guided feature modulator to incorporate task awareness in video understanding, with the entity stream capturing frame-level object information within a short context and the scene stream identifying their broader interactions over longer period of time. Each stream is supported by dedicated memory banks which enables our proposed Hierachical Querying transformer (HierarQ) to effectively capture short and long-term context. Extensive evaluations on 10 video benchmarks across video understanding, question answering, and captioning tasks demonstrate HierarQ’s state-of-the-art performance across most datasets, proving its robustness and efficiency for comprehensive video analysis.
尽管多模态大型语言模型(MLLM)取得了进展,但当前的方法在中长视频理解方面仍面临挑战,因为存在帧和上下文长度的限制。因此,这些模型通常依赖于帧采样,这有可能在长时间内遗漏关键信息并缺乏特定任务的关联性。为了解决这些挑战,我们引入了 HierarQ,这是一个基于任务感知的分层 Q-Former 框架,它能够按顺序处理帧,从而绕过帧采样的需要,同时避免 LLM 的上下文长度限制。我们引入了一个轻量级的双流语言引导特征调制器,以在视频理解中融入任务感知能力,其中实体流在短语境内捕获帧级对象信息,场景流则识别长时间内的更广泛的交互。每个流都受到专用内存库的支持,这使得我们提出的分层查询转换器(HierarQ)可以有效地捕获短期和长期的上下文。在视频理解、问答和字幕任务等十个视频基准测试上的广泛评估表明,HierarQ 在大多数数据集上的性能都处于最新水平,证明了其在综合视频分析中的稳健性和效率。
论文及项目相关链接
PDF Accepted in CVPR 2025
Summary
当前的多模态大型语言模型在处理中等至长视频时面临挑战,存在帧和上下文长度限制。因此,这些模型通常依赖于帧采样,这可能会错过关键信息并缺乏特定任务的关联性。为解决这些问题,我们提出了HierarQ框架,它通过任务感知的层次型Q-Former进行帧的序列处理,绕过帧采样的需要,避免LLM的上下文长度限制。我们引入了一个轻量级的双流语言引导特征调制器,将任务感知融入视频理解中,实体流捕捉短上下文内的帧级对象信息,场景流识别长时间内的更广泛的交互。每个流都受到专用内存库的支持,这使得我们提出的层次查询转换器(HierarQ)能够有效地捕捉短期和长期的上下文。在跨越视频理解、问答和字幕任务的十个视频基准测试上的评估表明,HierarQ在大多数数据集上表现出卓越的性能,证明了其在全面的视频分析中的稳健性和效率。
Key Takeaways
- 当前的多模态大型语言模型在处理中等至长视频时存在挑战,主要因为帧和上下文长度的限制。
- 现有模型常依赖帧采样,这可能错过关键信息并缺乏特定任务的关联性。
- HierarQ框架通过任务感知的层次型Q-Former进行帧的序列处理,绕过帧采样的限制。
- HierarQ引入了语言引导的特征调制器,包含实体流和场景流以分别捕捉帧级和场景级的信息。
- 实体流和场景流都受到专用内存库的支持,以有效地捕捉短期和长期的上下文。
- HierarQ在多个视频理解、问答和字幕任务的数据集上表现出卓越性能。
点此查看论文截图
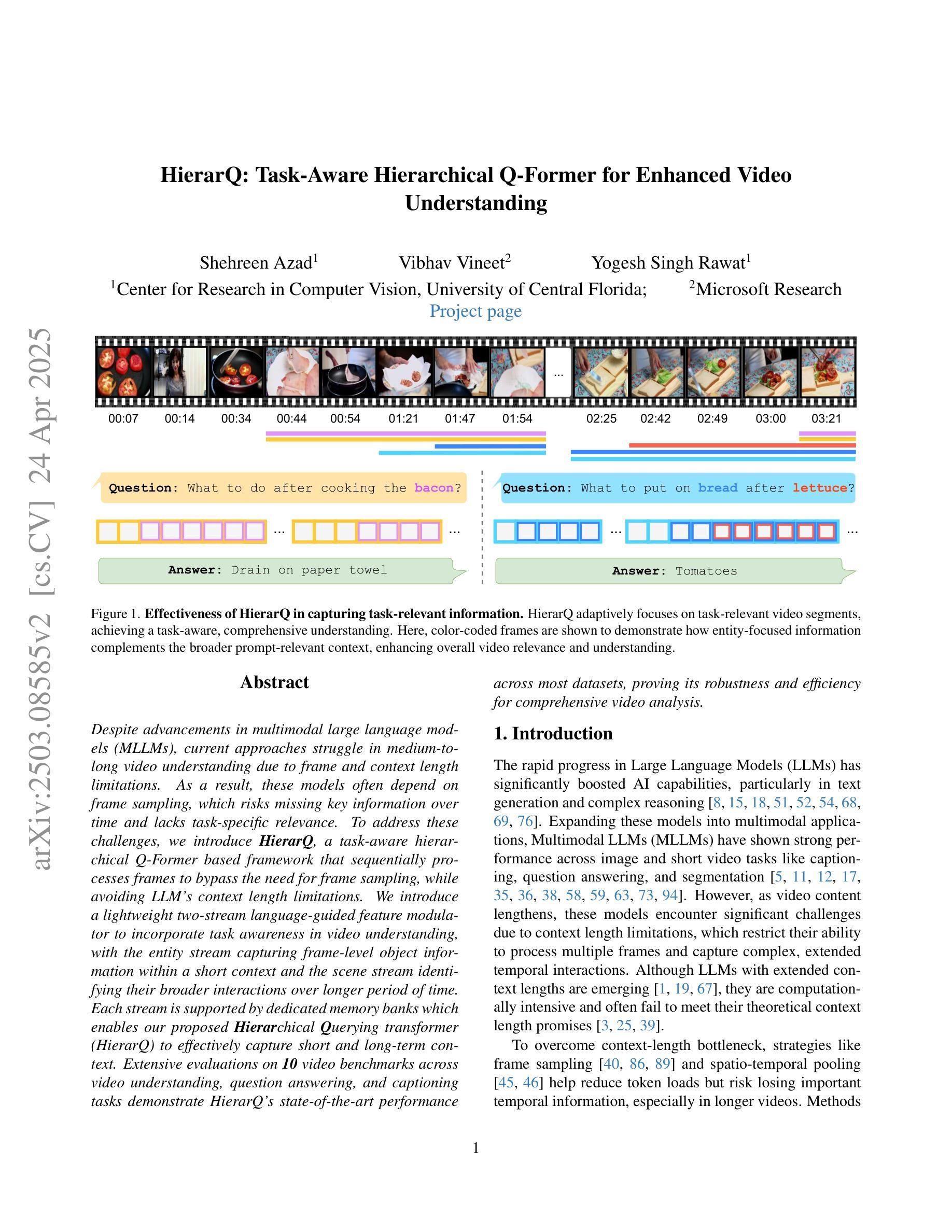
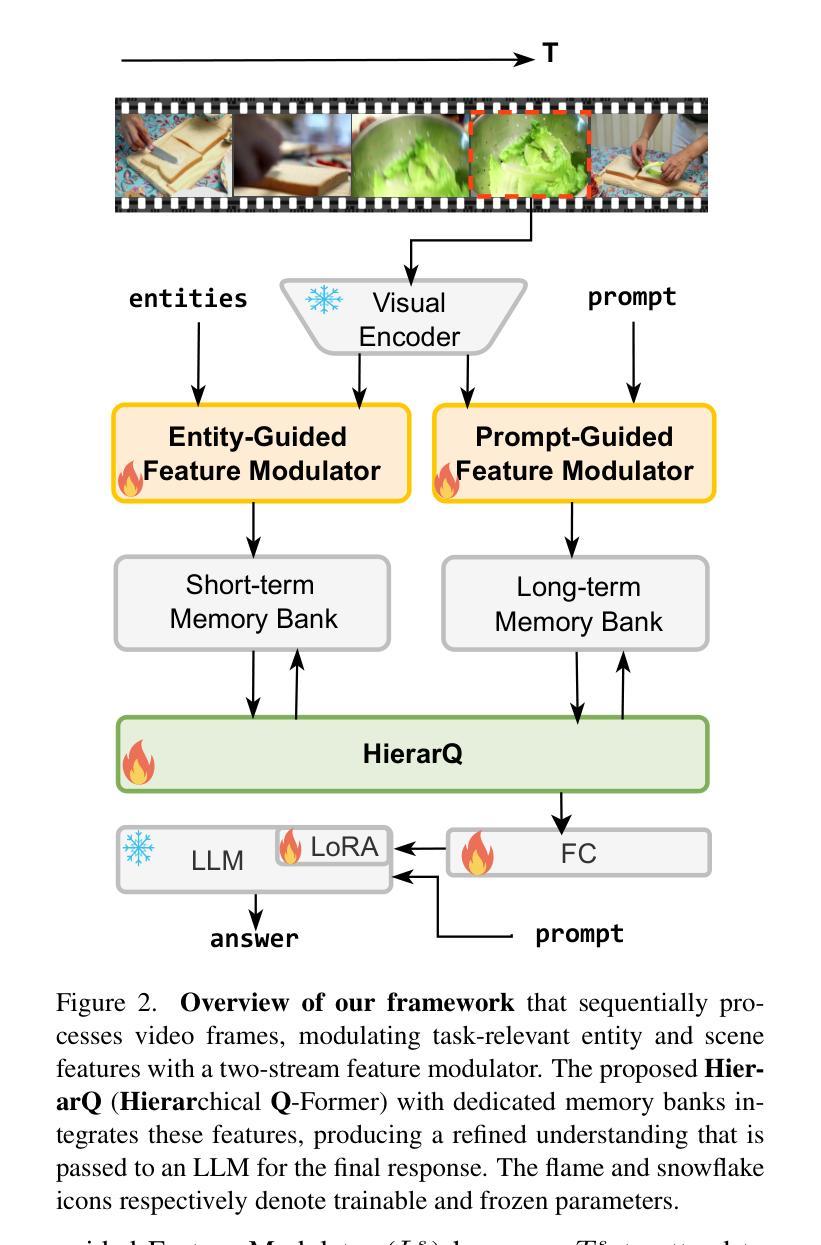
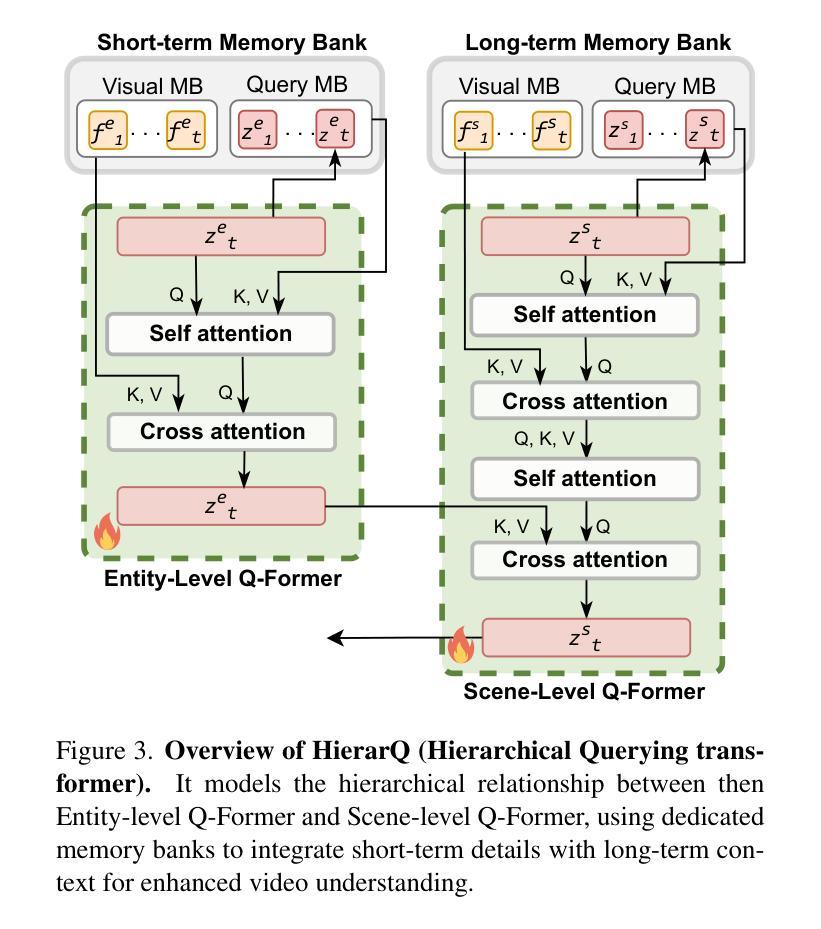
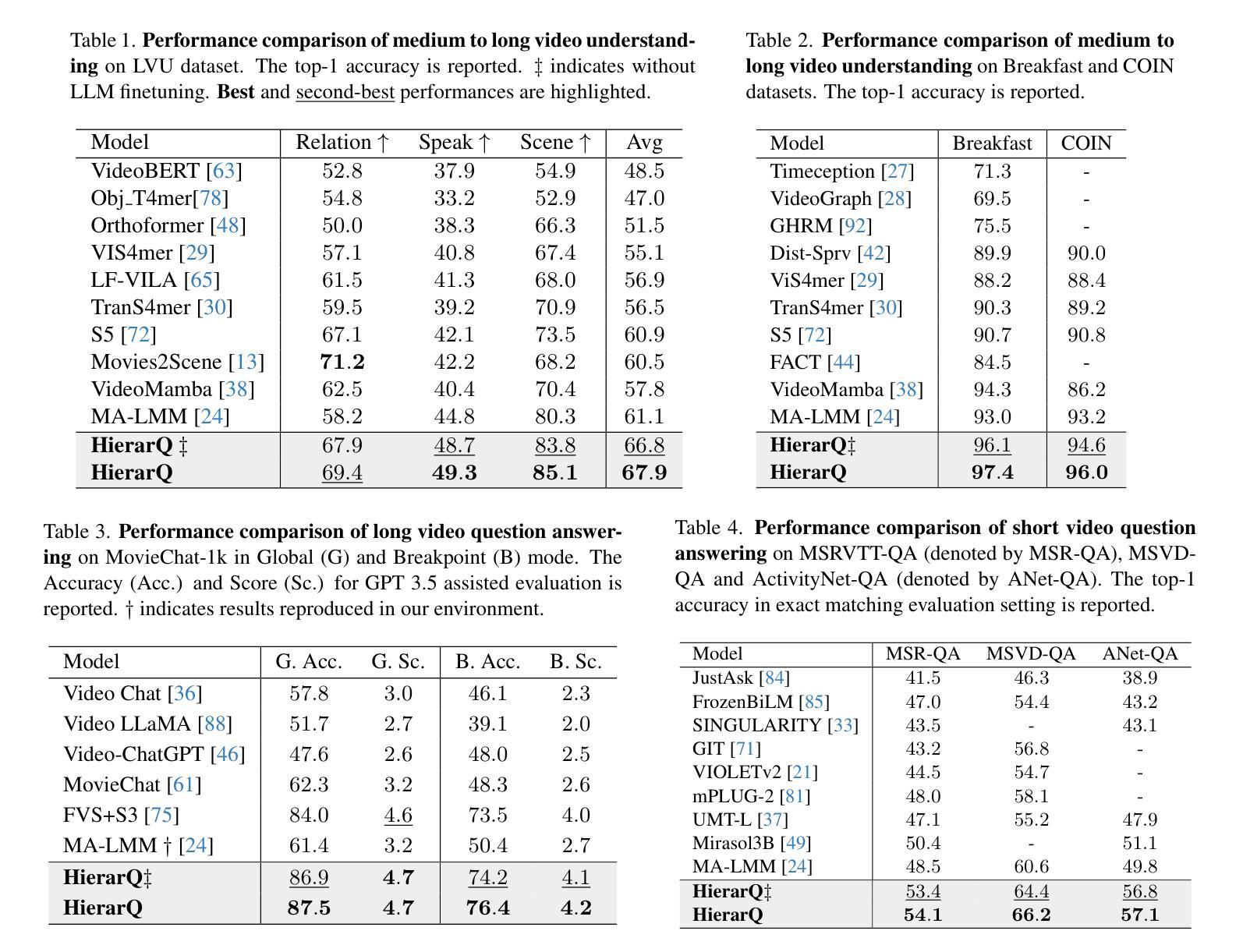
CallNavi, A Challenge and Empirical Study on LLM Function Calling and Routing
Authors:Yewei Song, Xunzhu Tang, Cedric Lothritz, Saad Ezzini, Jacques Klein, Tegawendé F. Bissyandé, Andrey Boytsov, Ulrick Ble, Anne Goujon
API-driven chatbot systems are increasingly integral to software engineering applications, yet their effectiveness hinges on accurately generating and executing API calls. This is particularly challenging in scenarios requiring multi-step interactions with complex parameterization and nested API dependencies. Addressing these challenges, this work contributes to the evaluation and assessment of AI-based software development through three key advancements: (1) the introduction of a novel dataset specifically designed for benchmarking API function selection, parameter generation, and nested API execution; (2) an empirical evaluation of state-of-the-art language models, analyzing their performance across varying task complexities in API function generation and parameter accuracy; and (3) a hybrid approach to API routing, combining general-purpose large language models for API selection with fine-tuned models and prompt engineering for parameter generation. These innovations significantly improve API execution in chatbot systems, offering practical methodologies for enhancing software design, testing, and operational workflows in real-world software engineering contexts.
API驱动的聊天机器人系统越来越成为软件工程技术应用的重要组成部分,但其有效性取决于API调用的准确生成和执行。在需要多步交互、复杂参数化和嵌套API依赖的场景下,这尤其具有挑战性。针对这些挑战,本研究通过三个关键进展为基于人工智能的软件开发评估做出了贡献:(1)引入专门用于基准测试API函数选择、参数生成和嵌套API执行的新型数据集;(2)对最先进语言模型的实证评估,分析其在不同任务复杂性和API函数生成的参数准确性方面的性能;(3)一种API路由的混合方法,结合用于API选择的通用大型语言模型与针对参数生成的经过微调模型和提示工程。这些创新显著提高了聊天机器人系统中的API执行能力,为增强现实世界软件工程技术环境中的软件设计、测试和操作流程提供了实用方法。
论文及项目相关链接
Summary
在软件工程应用中,API驱动的聊天机器人系统越来越重要,但其有效性取决于准确生成和执行API调用。这项工作通过三个关键进展对AI在软件开发中的应用进行了评估:引入专门用于基准测试API功能选择、参数生成和嵌套API执行的新数据集;对先进语言模型的实证评估;以及API路由的混合方法。这些创新大大提高了API在聊天机器人系统中的执行能力,为增强软件设计、测试和操作流程提供了实用方法。
Key Takeaways
- API驱动的聊天机器人系统在软件工程中越来越重要。
- 准确生成和执行API调用是确保系统有效性的关键。
- 工作贡献一:引入专门用于API功能选择、参数生成和嵌套API执行基准测试的新数据集。
- 工作贡献二:对先进语言模型的性能进行了实证评估,分析其在不同任务复杂度和参数准确性方面的表现。
- 提出了一种混合API路由方法,结合通用大型语言模型进行API选择和精细调整模型以及提示工程进行参数生成。
- 这些创新大大提高了聊天机器人系统中API的执行能力。
点此查看论文截图
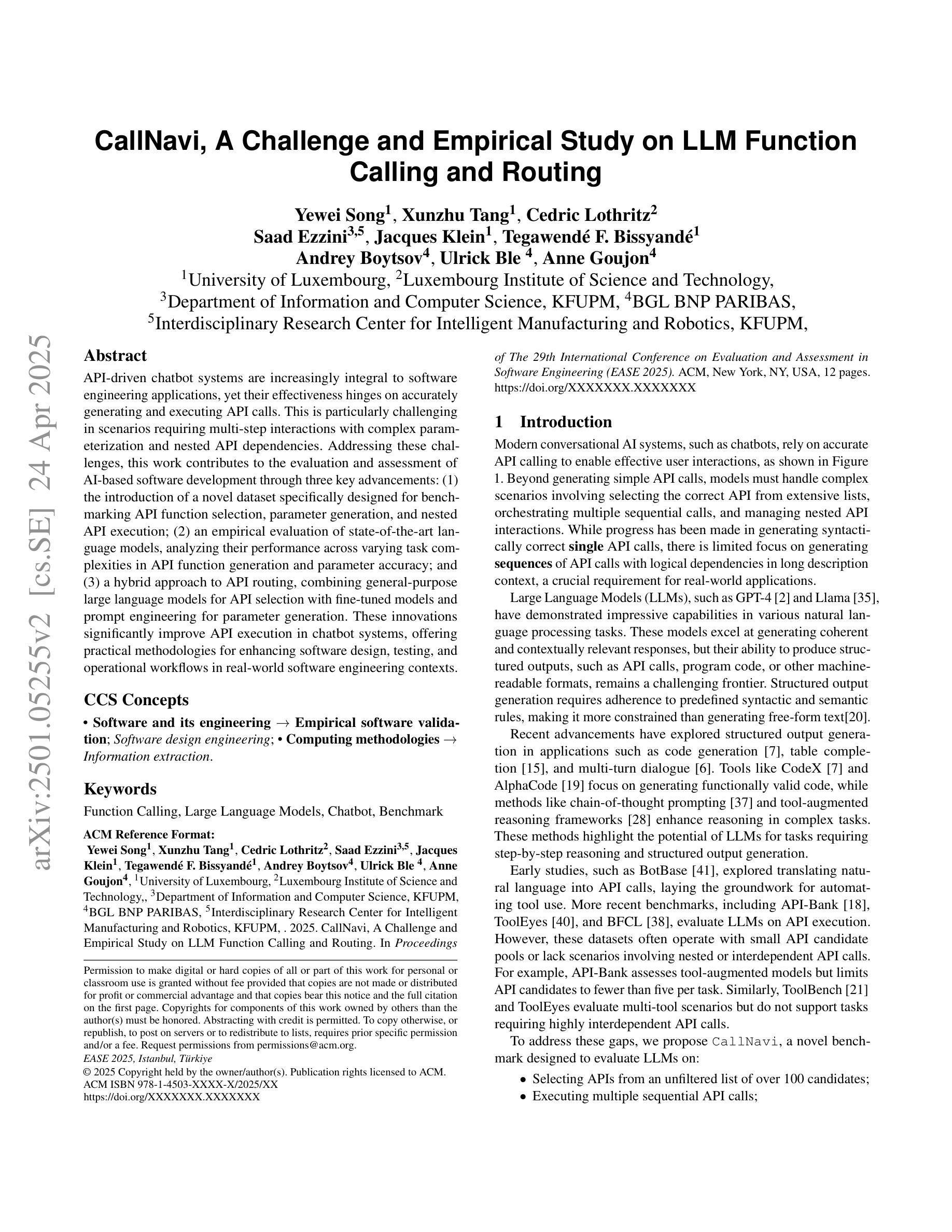
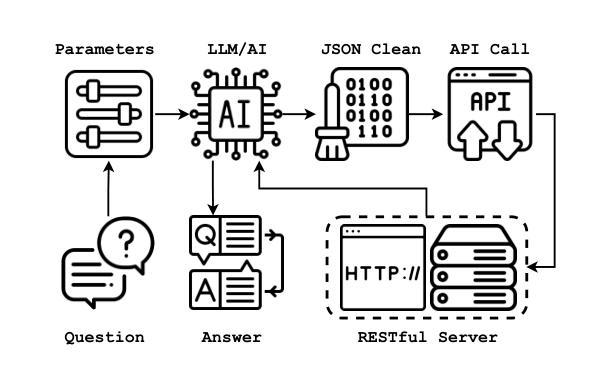
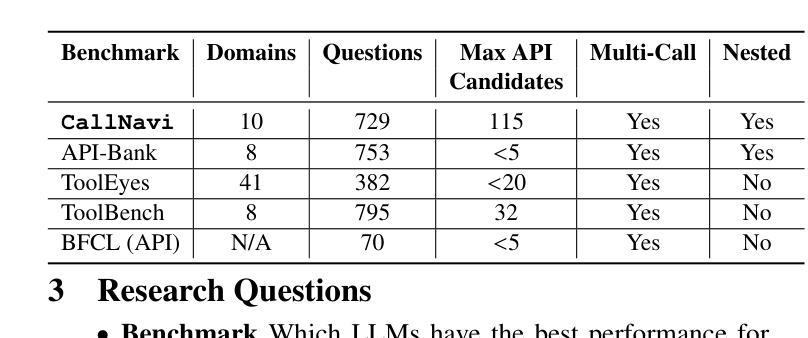
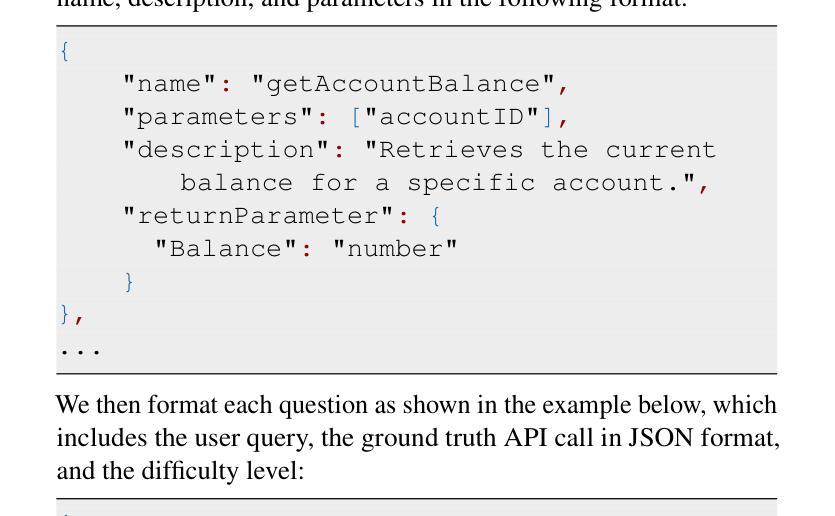
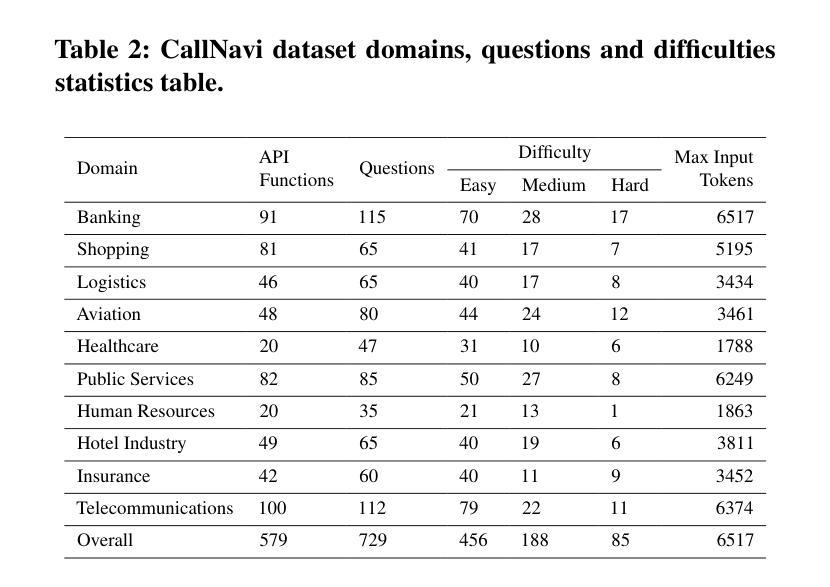
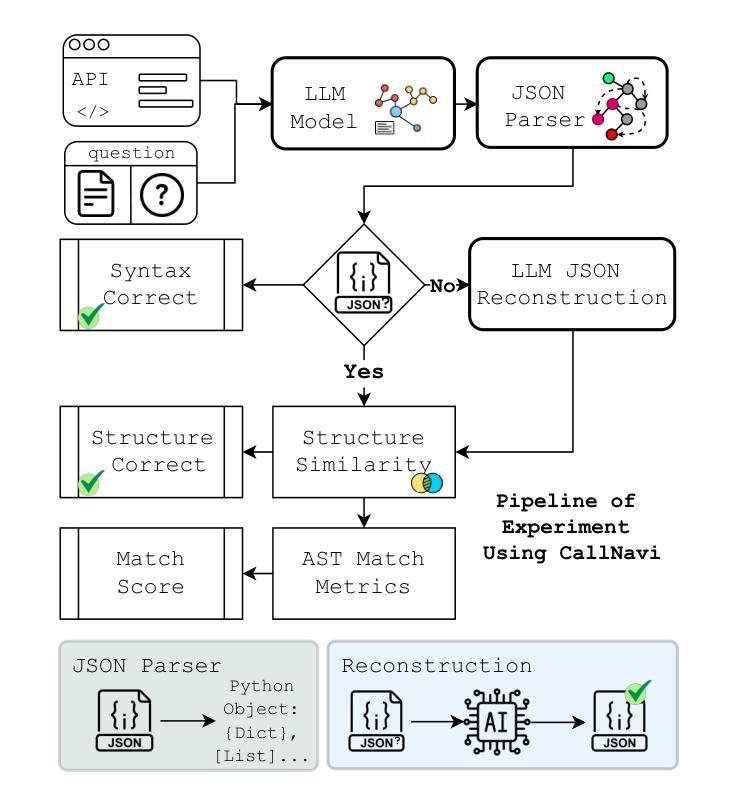
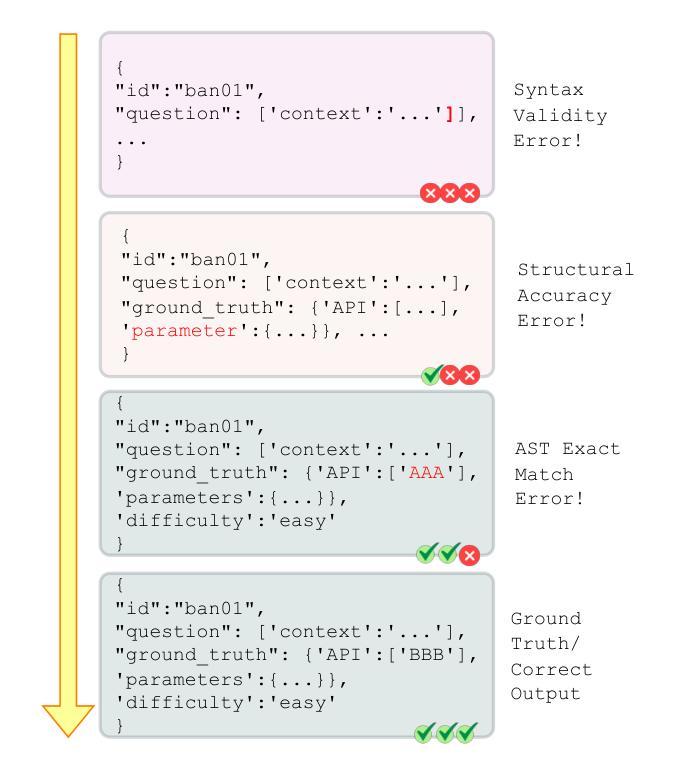
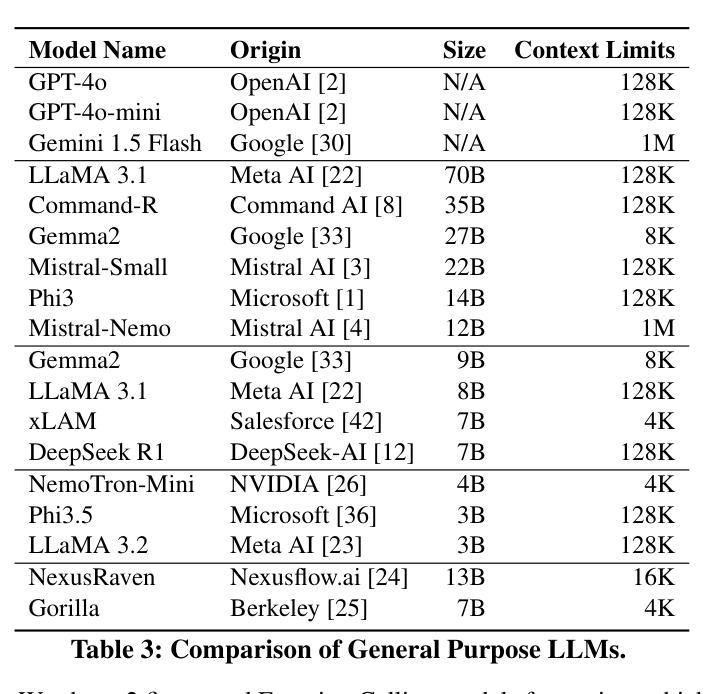
3D-LLaVA: Towards Generalist 3D LMMs with Omni Superpoint Transformer
Authors:Jiajun Deng, Tianyu He, Li Jiang, Tianyu Wang, Feras Dayoub, Ian Reid
Current 3D Large Multimodal Models (3D LMMs) have shown tremendous potential in 3D-vision-based dialogue and reasoning. However, how to further enhance 3D LMMs to achieve fine-grained scene understanding and facilitate flexible human-agent interaction remains a challenging problem. In this work, we introduce 3D-LLaVA, a simple yet highly powerful 3D LMM designed to act as an intelligent assistant in comprehending, reasoning, and interacting with the 3D world. Unlike existing top-performing methods that rely on complicated pipelines-such as offline multi-view feature extraction or additional task-specific heads-3D-LLaVA adopts a minimalist design with integrated architecture and only takes point clouds as input. At the core of 3D-LLaVA is a new Omni Superpoint Transformer (OST), which integrates three functionalities: (1) a visual feature selector that converts and selects visual tokens, (2) a visual prompt encoder that embeds interactive visual prompts into the visual token space, and (3) a referring mask decoder that produces 3D masks based on text description. This versatile OST is empowered by the hybrid pretraining to obtain perception priors and leveraged as the visual connector that bridges the 3D data to the LLM. After performing unified instruction tuning, our 3D-LLaVA reports impressive results on various benchmarks.
当前,3D大型多模态模型(3D LMMs)在基于3D视觉的对话和推理方面显示出巨大的潜力。然而,如何进一步增强3D LMMs以实现精细场景理解和促进灵活的人机交互仍然是一个具有挑战性的问题。在这项工作中,我们引入了名为“三维LLaVA”的简单而强大的三维模型。它旨在充当智能助理,用于理解、推理和与三维世界交互。不同于现有高性能的方法,这些方法依赖于复杂的管道,如离线多视图特征提取或额外的特定任务头,三维LLaVA采用极简设计,具有集成架构,仅采用点云作为输入。三维LLaVA的核心是一个全新的Omni Superpoint Transformer(OST),它集成了三种功能:(1)视觉特征选择器,用于转换和选择视觉令牌;(2)视觉提示编码器将交互式视觉提示嵌入视觉令牌空间;(3)参照掩码解码器根据文本描述生成三维掩码。这种通用OST通过混合预训练获得感知先验知识,并被用作连接三维数据和LLM的视觉连接器。经过统一的指令微调后,我们的三维LLaVA在各种基准测试中取得了令人印象深刻的结果。
论文及项目相关链接
PDF Accepted by CVPR 2025
Summary
本文介绍了针对三维世界理解、推理和交互的智能助手——名为“LLaVA”的大型三维多模态模型(Large Multimodal Model)。它采用极简设计,仅使用点云作为输入,通过混合预训练强化感知先验。其核心为全新的Omni Superpoint Transformer(OST),它融合了视觉特征选择、交互式视觉提示嵌入和基于文本描述生成三维掩码的功能。该模型在多个基准测试中表现出卓越性能。
Key Takeaways
- 当前大型三维多模态模型(3D LMMs)在基于三维视觉的对话和推理方面展现出巨大潜力。
- 提出了一种名为“LLaVA”的新型模型,旨在实现场景精细化理解及灵活的人机交互。
- LLaVA模型设计极简,以点云为输入,通过集成架构进行信息处理。
- 模型核心为Omni Superpoint Transformer(OST),融合了视觉特征选择、视觉提示嵌入和基于文本描述生成三维掩码功能。
- LLaVA采用混合预训练,通过感知先验增强模型的性能。
- 模型实现了统一的指令调整优化性能表现。
点此查看论文截图
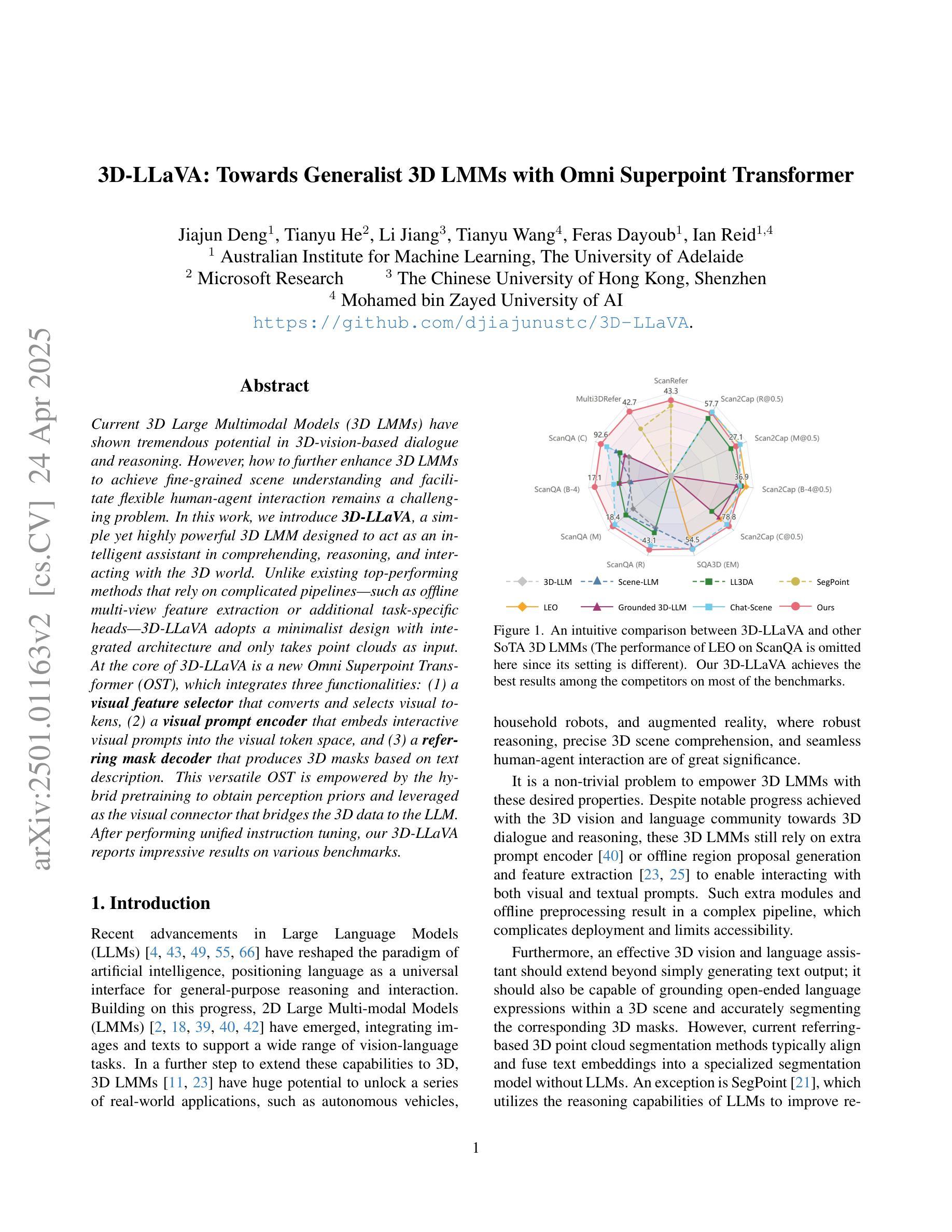
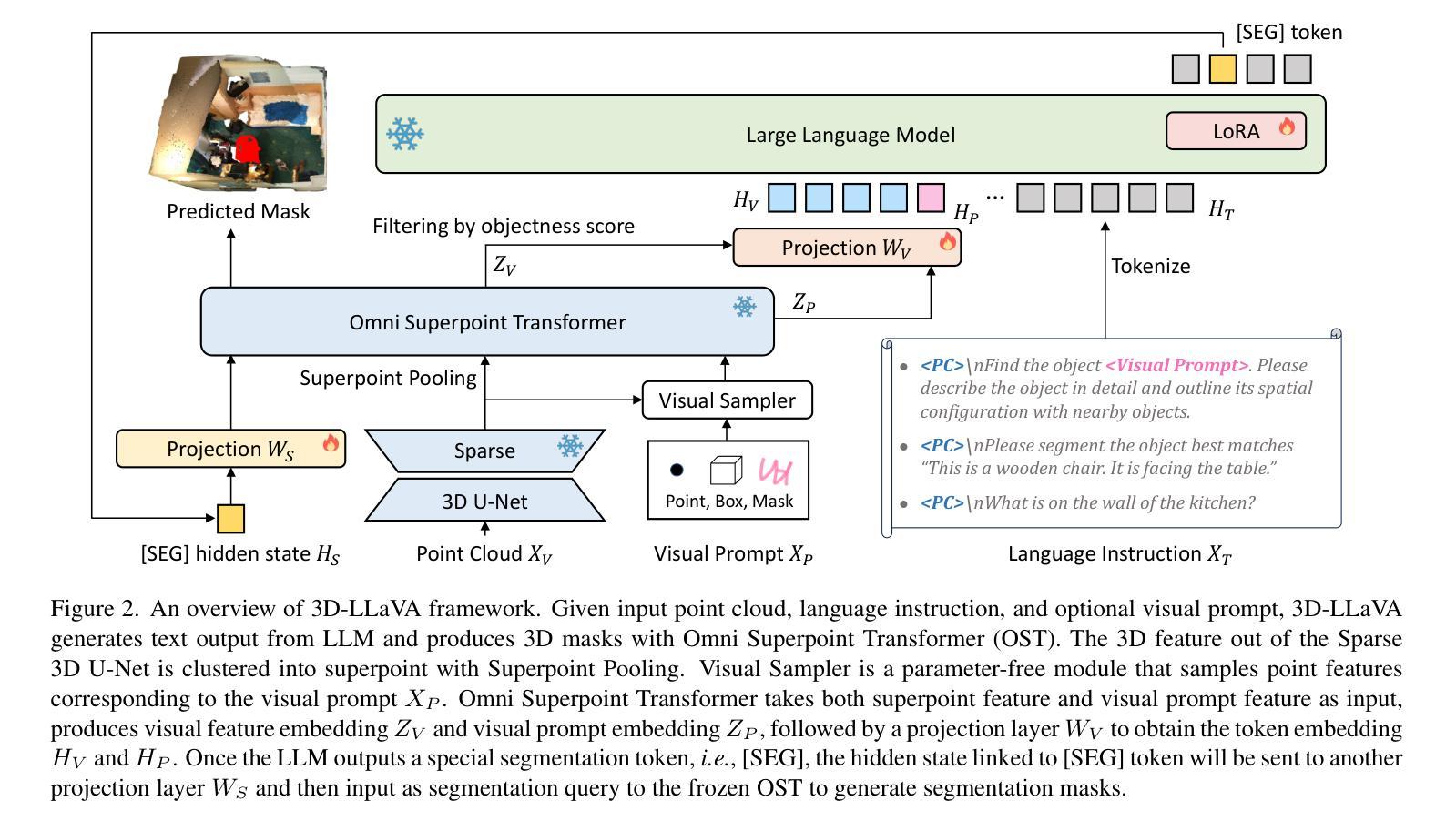
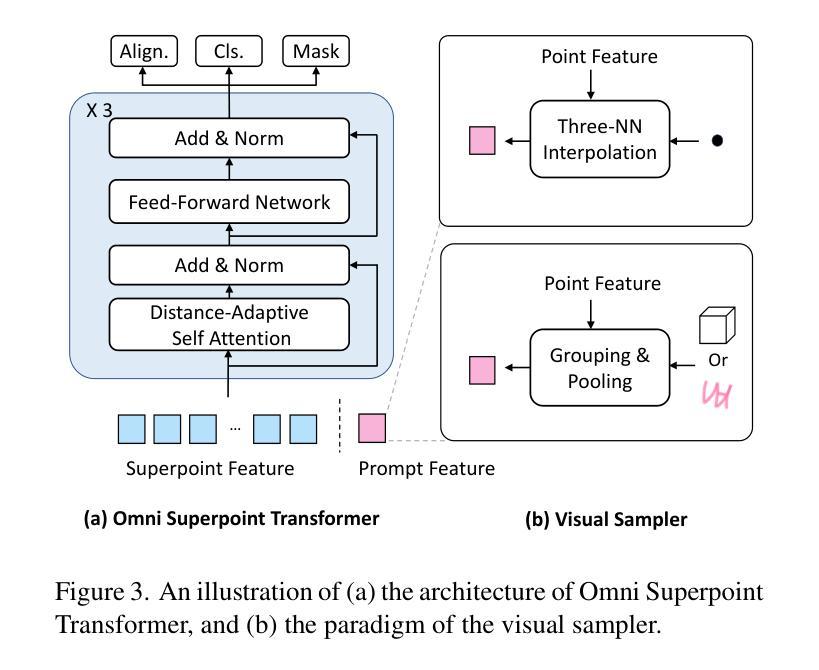
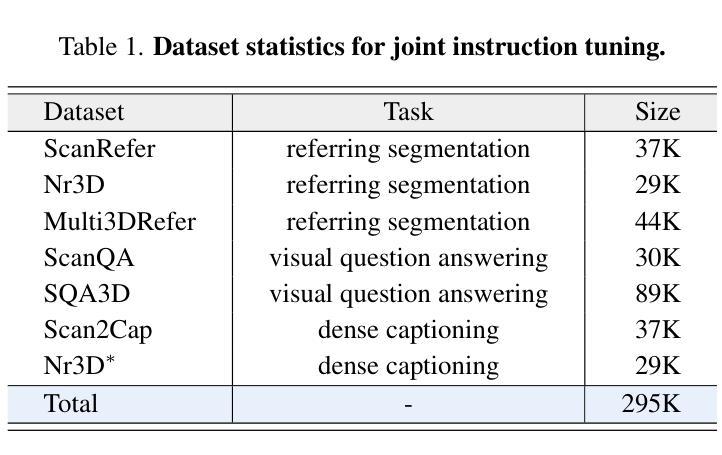
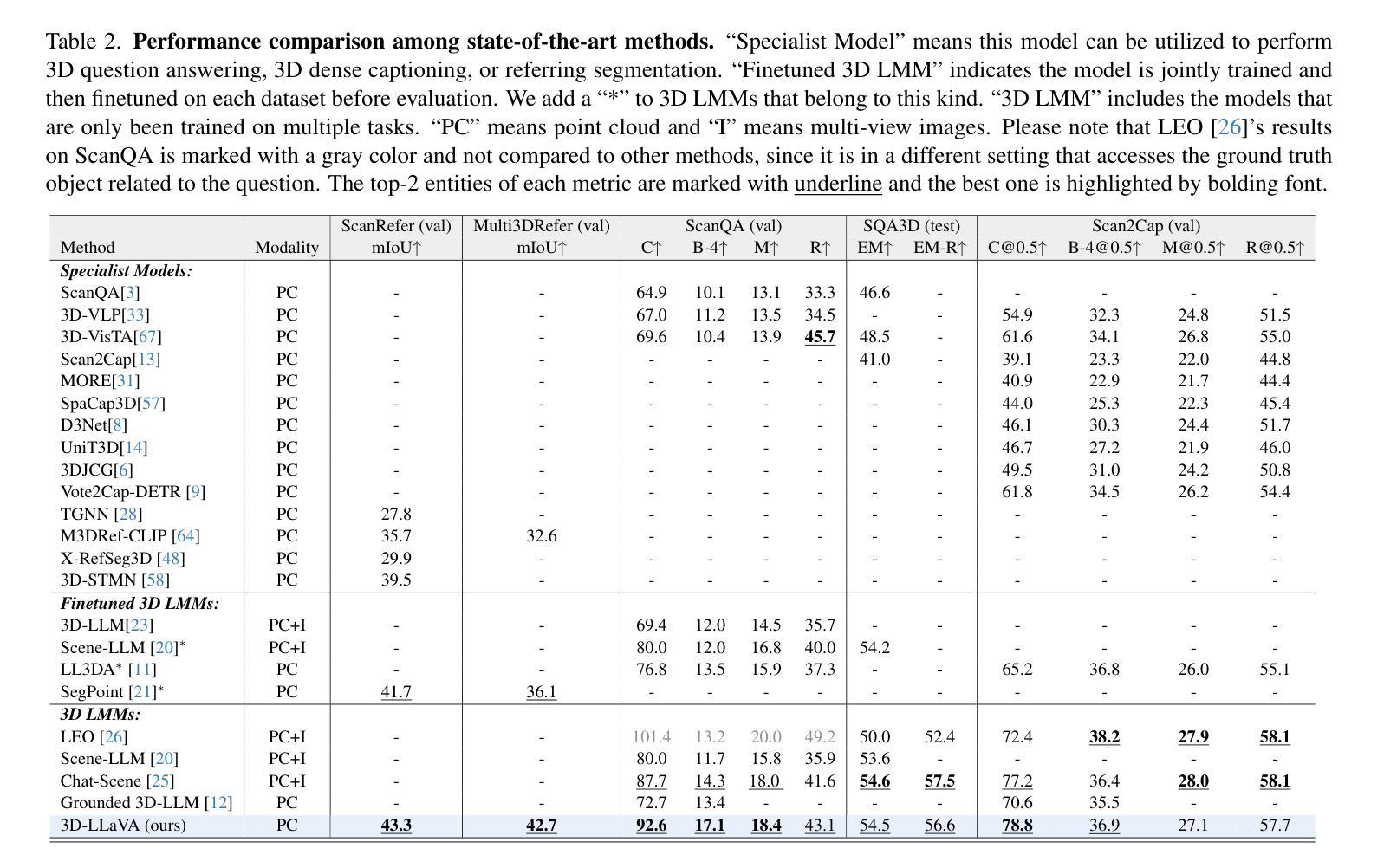
QUART-Online: Latency-Free Large Multimodal Language Model for Quadruped Robot Learning
Authors:Xinyang Tong, Pengxiang Ding, Yiguo Fan, Donglin Wang, Wenjie Zhang, Can Cui, Mingyang Sun, Han Zhao, Hongyin Zhang, Yonghao Dang, Siteng Huang, Shangke Lyu
This paper addresses the inherent inference latency challenges associated with deploying multimodal large language models (MLLM) in quadruped vision-language-action (QUAR-VLA) tasks. Our investigation reveals that conventional parameter reduction techniques ultimately impair the performance of the language foundation model during the action instruction tuning phase, making them unsuitable for this purpose. We introduce a novel latency-free quadruped MLLM model, dubbed QUART-Online, designed to enhance inference efficiency without degrading the performance of the language foundation model. By incorporating Action Chunk Discretization (ACD), we compress the original action representation space, mapping continuous action values onto a smaller set of discrete representative vectors while preserving critical information. Subsequently, we fine-tune the MLLM to integrate vision, language, and compressed actions into a unified semantic space. Experimental results demonstrate that QUART-Online operates in tandem with the existing MLLM system, achieving real-time inference in sync with the underlying controller frequency, significantly boosting the success rate across various tasks by 65%. Our project page is https://quart-online.github.io.
本文旨在解决在四足视觉语言动作(QUAR-VLA)任务中部署多模态大型语言模型(MLLM)所面临的固有推理延迟挑战。我们的调查发现,传统的参数减少技术最终会损害语言基础模型在动作指令调整阶段的性能,使其不适合此目的。我们引入了一种新型的无延迟四足MLLM模型,名为QUART-Online,旨在提高推理效率,同时不降低语言基础模型的性能。通过引入动作片段离散化(ACD),我们压缩了原始动作表示空间,将连续动作值映射到一组较小的离散代表向量上,同时保留关键信息。随后,我们对MLLM进行微调,以将视觉、语言和压缩动作集成到一个统一的语义空间中。实验结果表明,QUART-Online与现有MLLM系统协同工作,实现与底层控制器频率同步的实时推理,在各种任务中的成功率提高了65%。我们的项目页面是https://quart-online.github.io。
论文及项目相关链接
PDF Accepted to ICRA 2025; Github page: https://quart-online.github.io
Summary
本文探讨了部署多模态大型语言模型(MLLM)在四足视觉-语言-动作(QUAR-VLA)任务时面临的固有推理延迟挑战。研究发现,传统的参数减少技术最终会损害语言基础模型在动作指令调整阶段的性能,使其不适合此用途。为此,文章提出了一种新型的无延迟四足MLLM模型,名为QUART-Online,旨在提高推理效率,同时不降低语言基础模型的性能。通过引入动作块离散化(ACD)技术,该模型压缩了原始动作表示空间,将连续动作值映射到一组较小的离散代表向量上,同时保留了关键信息。随后,对MLLM进行微调,以将视觉、语言和压缩动作集成到统一的语义空间中。实验结果表明,QUART-Online与现有MLLM系统协同工作,实现了与底层控制器频率同步的实时推理,成功提高了各项任务的成功率达65%。
Key Takeaways
- 本文探讨了多模态大型语言模型(MLLM)在四足视觉-语言-动作(QUAR-VLA)任务中的推理延迟问题。
- 传统的参数减少技术会损害语言基础模型在动作指令调整阶段的性能。
- 提出了新型的无延迟四足MLLM模型——QUART-Online,旨在提高推理效率且不影响语言基础模型的性能。
- QUART-Online通过引入动作块离散化(ACD)技术,压缩了动作表示空间。
- QUART-Online实现了与现有MLLM系统的协同工作,实现了实时推理。
- QUART-Online显著提高了各项任务的成功率,达到65%。
- 项目的在线资源可通过https://quart-online.github.io访问。
点此查看论文截图
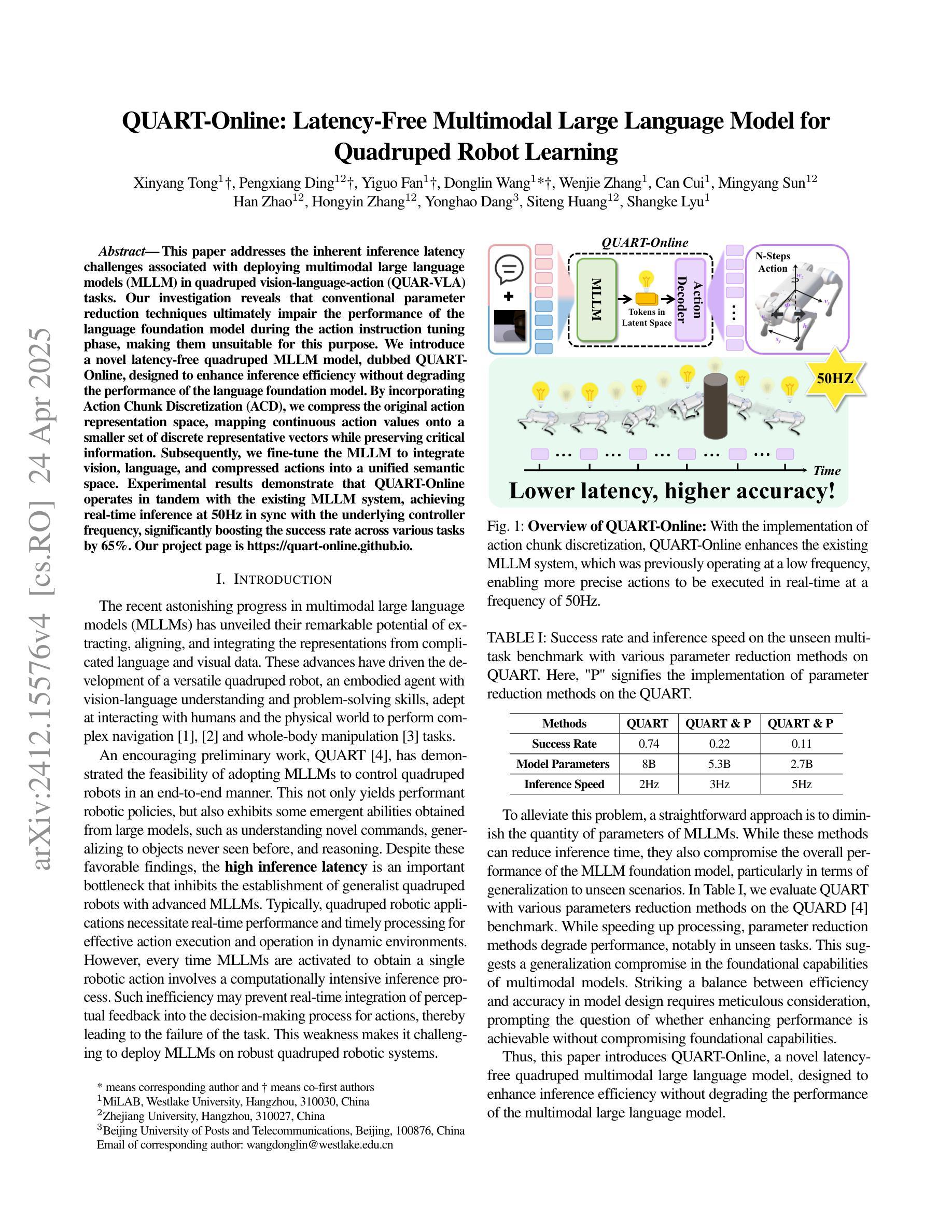
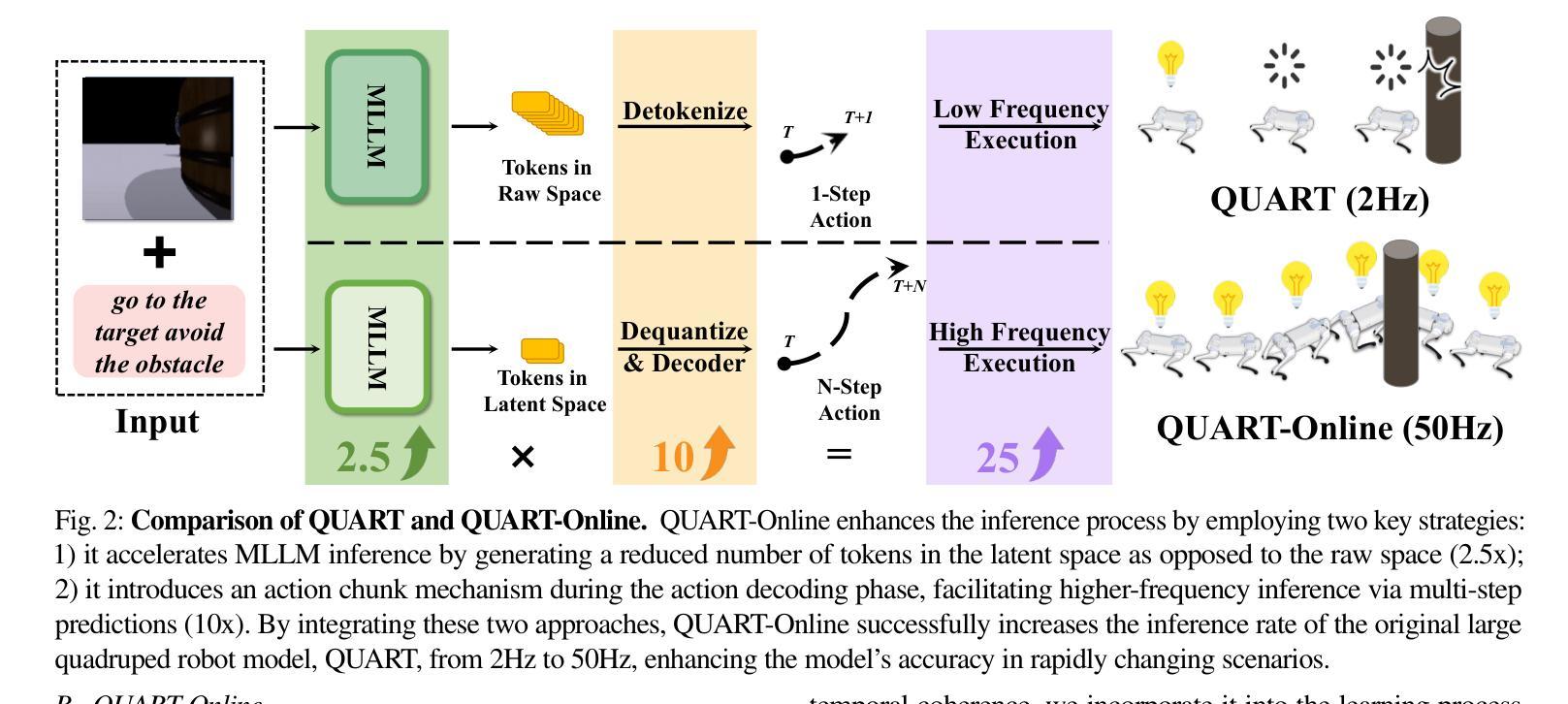
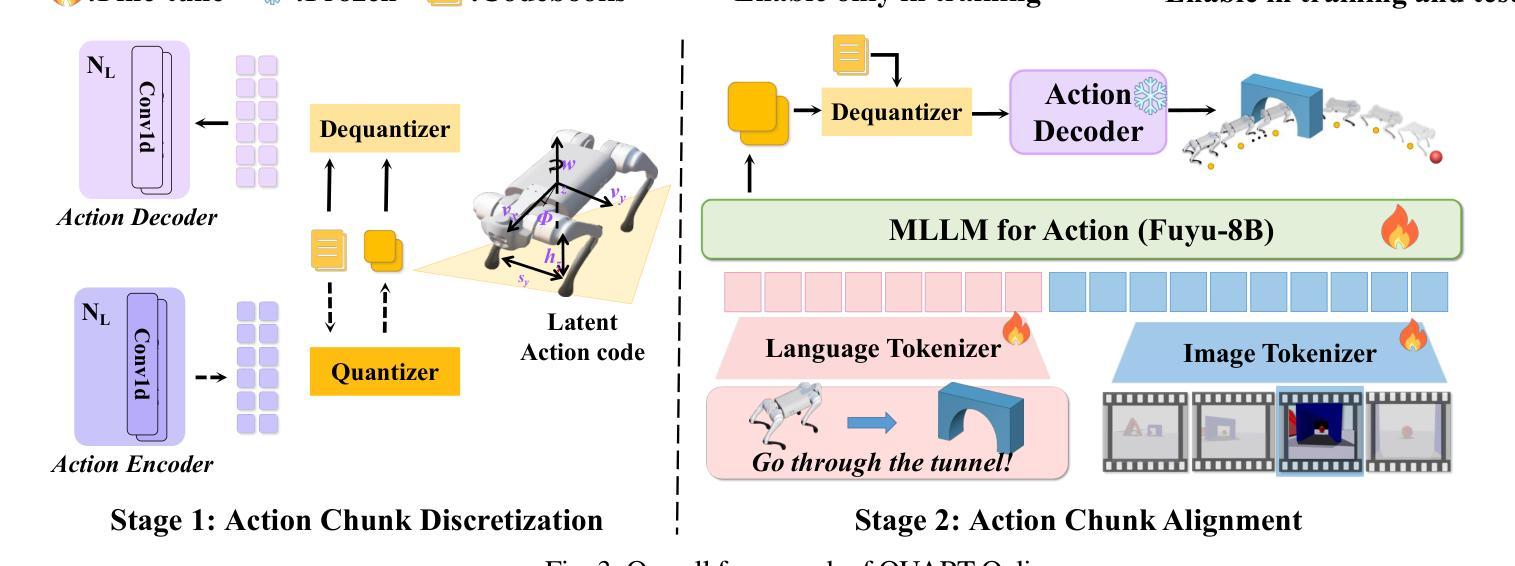
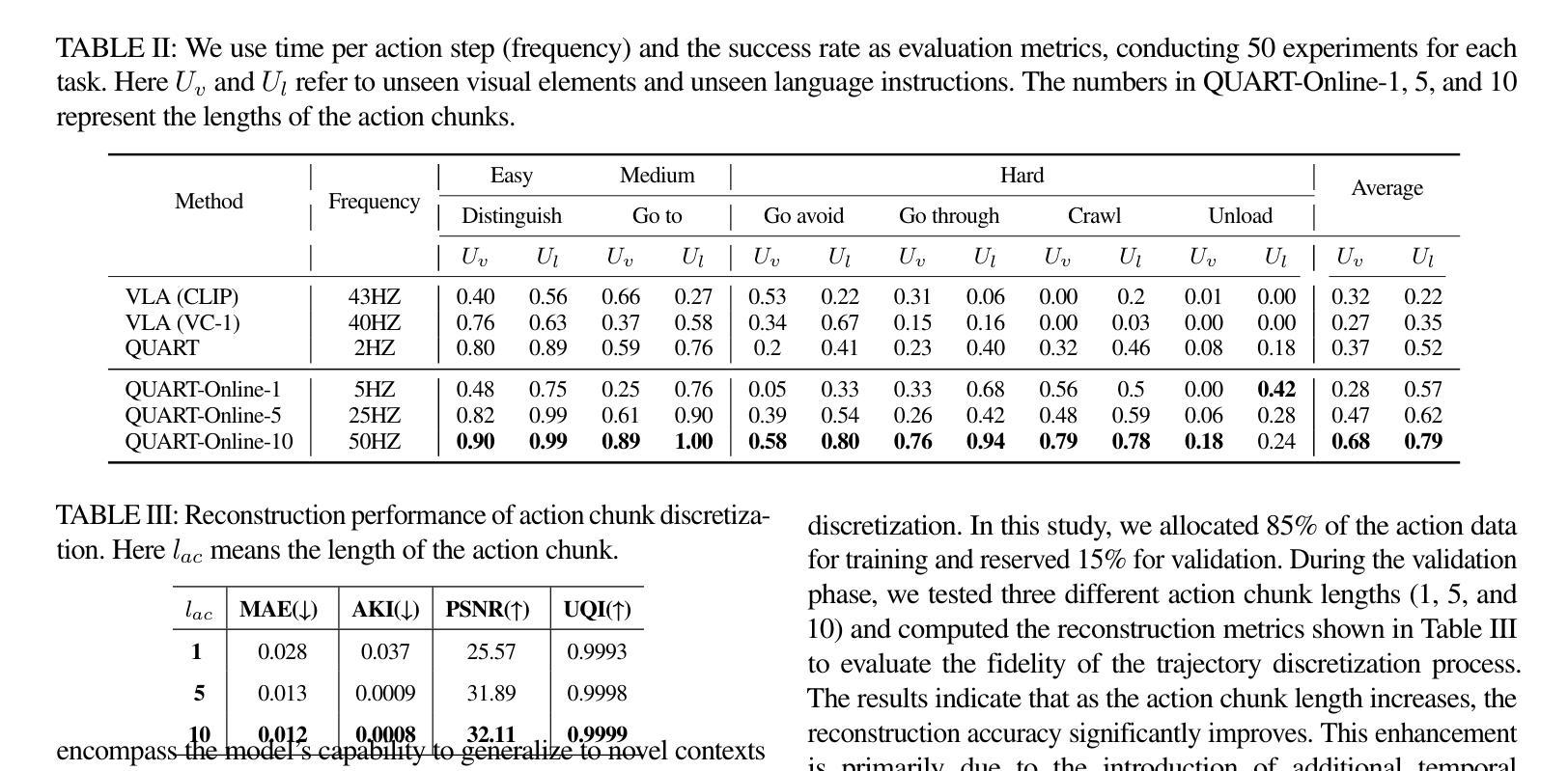
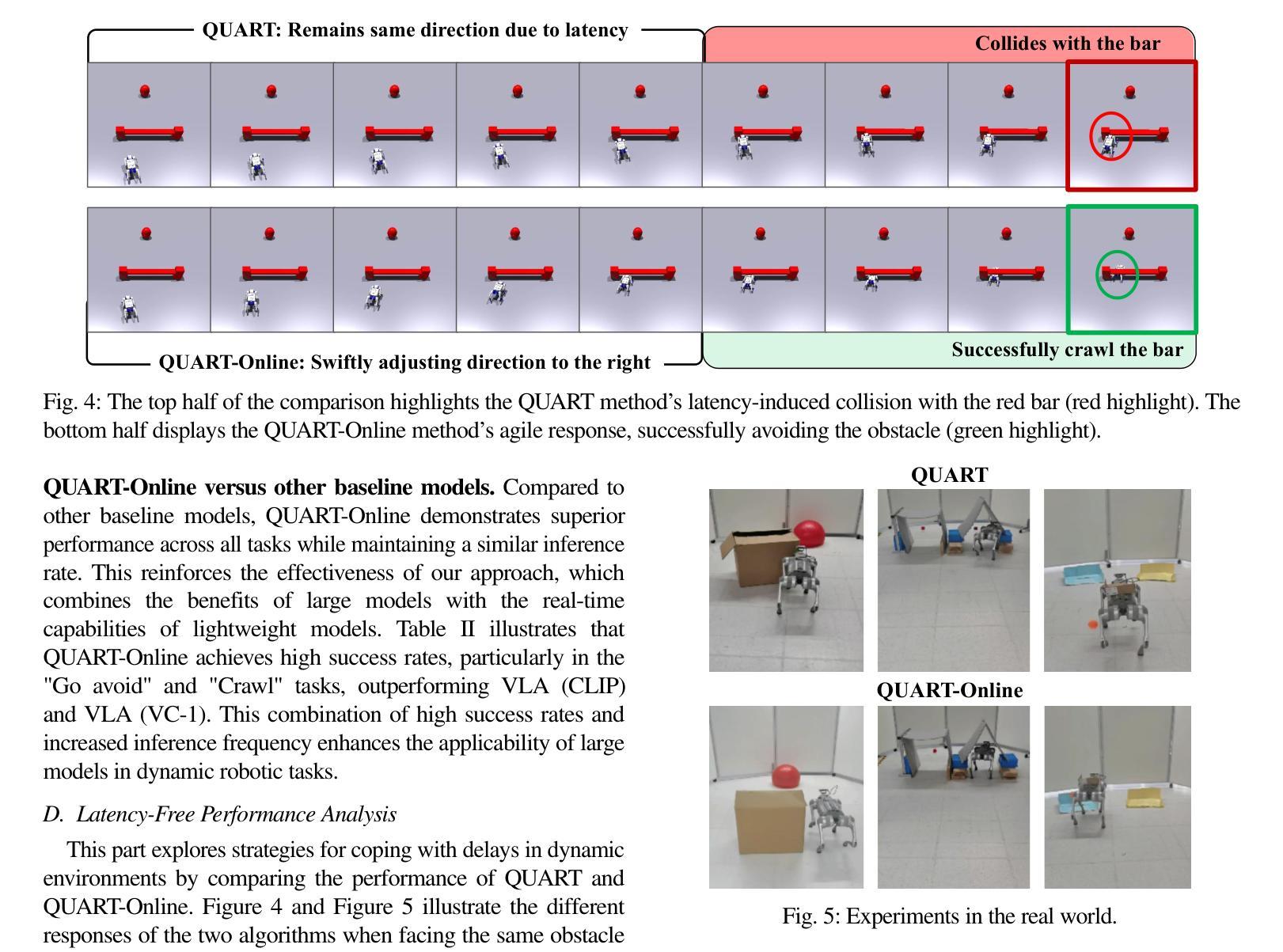
MedMax: Mixed-Modal Instruction Tuning for Training Biomedical Assistants
Authors:Hritik Bansal, Daniel Israel, Siyan Zhao, Shufan Li, Tung Nguyen, Aditya Grover
Recent advancements in mixed-modal generative have opened new avenues for developing unified biomedical assistants capable of analyzing biomedical images, answering complex questions about them, and generating multimodal patient reports. However, existing datasets face challenges such as small sizes, limited coverage of biomedical tasks and domains, and a reliance on narrow sources. To address these gaps, we present MedMax, a large-scale multimodal biomedical instruction-tuning dataset for mixed-modal foundation models. With 1.47 million instances, MedMax encompasses a diverse range of tasks, including interleaved image-text generation, biomedical image captioning and generation, visual chat, and report understanding. These tasks span knowledge across diverse biomedical domains, including radiology and histopathology, grounded in medical papers and YouTube videos. Subsequently, we fine-tune a mixed-modal foundation model on the MedMax dataset, achieving significant performance improvements: a 26% gain over the Chameleon model and an 18.3% improvement over GPT-4o across 12 downstream biomedical visual question-answering tasks. Finally, we introduce a unified evaluation suite for biomedical tasks to guide the development of mixed-modal biomedical AI assistants. The data, model, and code is available at https://mint-medmax.github.io/.
近期混合模态生成技术的进展为开发能够分析生物医学图像、回答关于它们的复杂问题并生成多模态患者报告的统一生物医学助理开辟了新途径。然而,现有数据集面临规模较小、生物医学任务和领域覆盖有限以及依赖狭窄来源等挑战。为了解决这些差距,我们推出了MedMax,这是一个用于混合模态基础模型的大型多模态生物医学指令调整数据集。MedMax包含147万个实例,涵盖各种任务,包括交替图像文本生成、生物医学图像描述和生成、视觉聊天和报告理解。这些任务涵盖了放射学和病理生理学等多样化的生物医学领域知识,基于医学论文和YouTube视频。随后,我们在MedMax数据集上对混合模态基础模型进行了微调,取得了显著的性能提升:在12个下游生物医学视觉问答任务上,相对于变色龙模型提高了26%,相对于GPT-4o提高了18.3%。最后,我们为生物医学任务引入了一套统一的评估套件,以指导混合模态生物医学人工智能助理的开发。数据、模型和代码可在https://mint-medmax.github.io/获取。
论文及项目相关链接
PDF 29 pages
Summary
近期混合模态生成技术的进展为开发能够分析生物医学图像、回答相关问题并生成多模态患者报告的统一生物医学助理提供了新的途径。针对现有数据集面临的挑战,如规模小、生物医学任务和领域覆盖有限以及对狭窄来源的依赖,我们推出了MedMax,这是一个大规模的多模态生物医学指令调整数据集,用于混合模态基础模型。MedMax包含147万个实例,涵盖多种任务,如交错图像文本生成、生物医学图像描述和生成、视觉聊天和报告理解等,跨越不同的生物医学领域,如放射学和病理学。在MedMax数据集上微调混合模态基础模型,实现了显著的性能提升,在12个下游生物医学视觉问答任务上分别较Chameleon模型和GPT-4o提高了26%和18.3%。最后,我们为生物医学任务引入了一个统一的评估套件,以指导混合模态生物医学人工智能助理的开发。
Key Takeaways
- 混合模态生成技术的最新进展为生物医学助理的开发提供了新的机会。
- MedMax是一个大规模的多模态生物医学指令调整数据集,用于混合模态基础模型。
- MedMax包含多样的任务,如图像文本生成、生物医学图像描述和生成、视觉聊天和报告理解等。
- MedMax数据集涵盖了广泛的生物医学领域,包括放射学和病理学。
- 在MedMax上微调的混合模态基础模型在多个生物医学视觉问答任务上实现了显著的性能提升。
- 引入了一个统一的评估套件,以指导生物医学任务的混合模态人工智能助理的开发。
点此查看论文截图
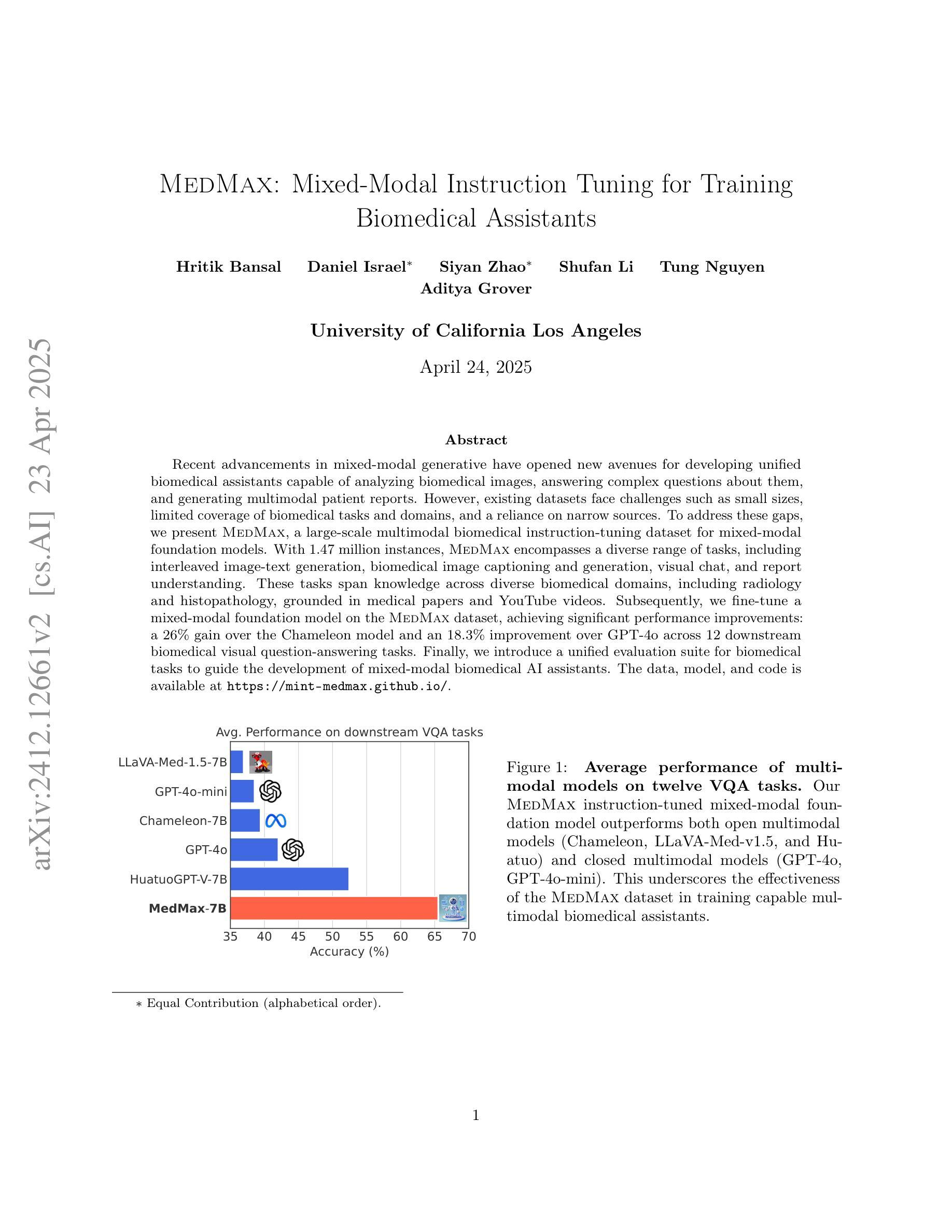
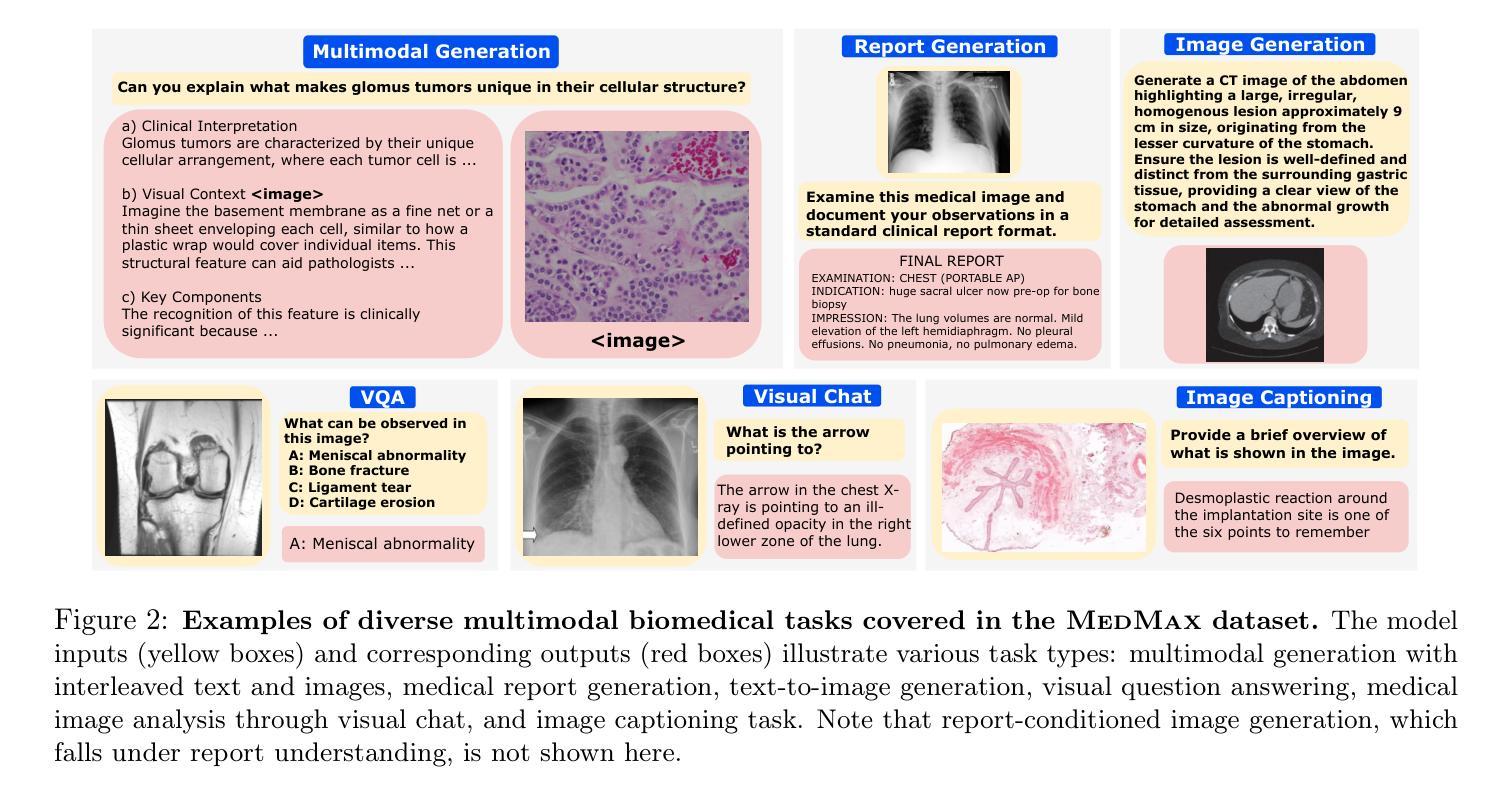
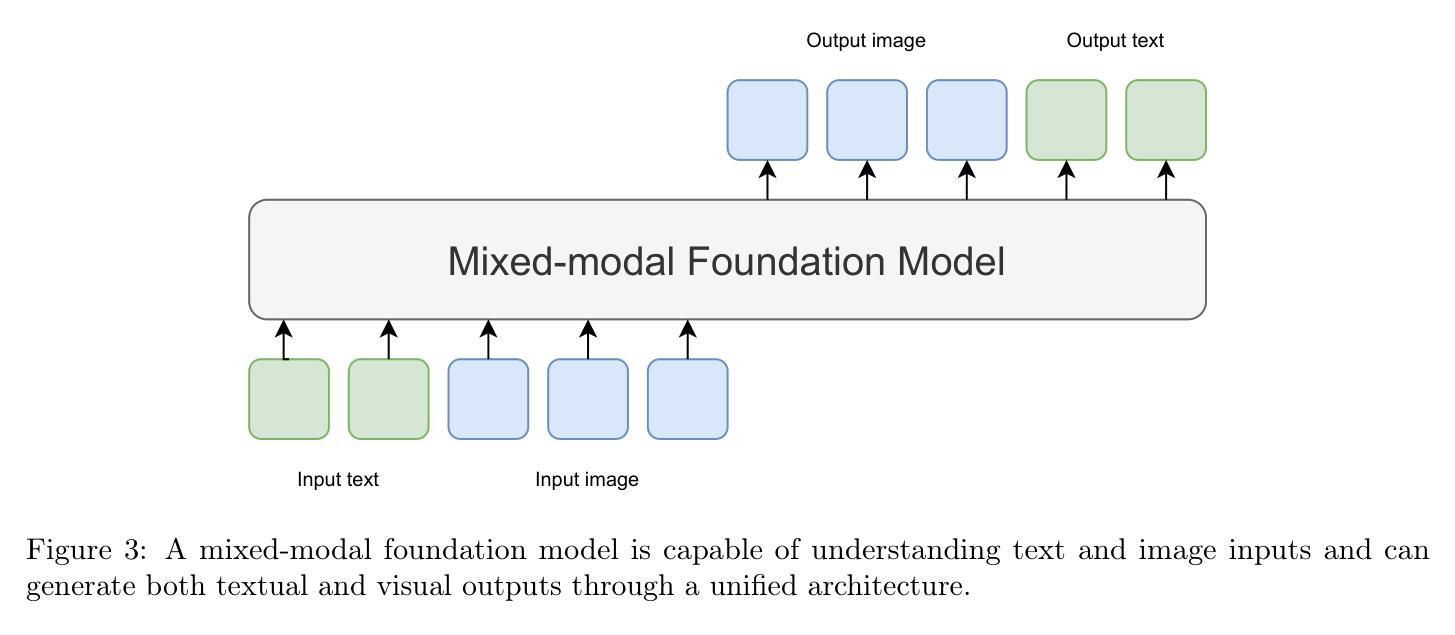
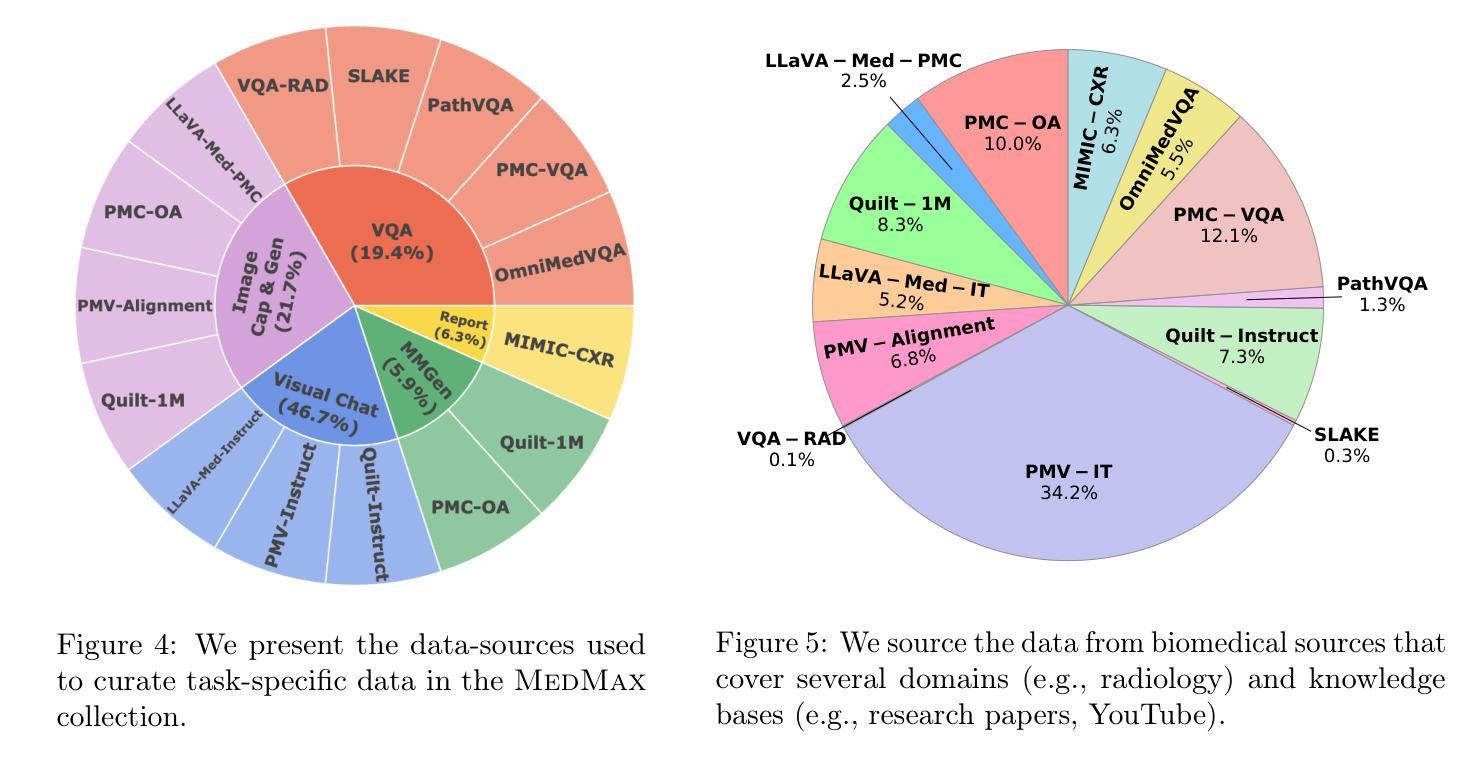
Fine-Grained and Multi-Dimensional Metrics for Document-Level Machine Translation
Authors:Yirong Sun, Dawei Zhu, Yanjun Chen, Erjia Xiao, Xinghao Chen, Xiaoyu Shen
Large language models (LLMs) have excelled in various NLP tasks, including machine translation (MT), yet most studies focus on sentence-level translation. This work investigates the inherent capability of instruction-tuned LLMs for document-level translation (docMT). Unlike prior approaches that require specialized techniques, we evaluate LLMs by directly prompting them to translate entire documents in a single pass. Our results show that this method improves translation quality compared to translating sentences separately, even without document-level fine-tuning. However, this advantage is not reflected in BLEU scores, which often favor sentence-based translations. We propose using the LLM-as-a-judge paradigm for evaluation, where GPT-4 is used to assess document coherence, accuracy, and fluency in a more nuanced way than n-gram-based metrics. Overall, our work demonstrates that instruction-tuned LLMs can effectively leverage document context for translation. However, we caution against using BLEU scores for evaluating docMT, as they often provide misleading outcomes, failing to capture the quality of document-level translation. Code and the outputs from GPT4-as-a-judge are available at https://github.com/EIT-NLP/BLEUless_DocMT
大型语言模型(LLM)在各种自然语言处理任务中表现出色,包括机器翻译(MT)。然而,大多数研究都集中在句子级别的翻译上。本研究旨在探究指令优化后的LLM在文档级别翻译(docMT)方面的固有能力。不同于需要专门技术的先前方法,我们通过直接提示LLM一次性翻译整个文档来评估其性能。结果表明,这种方法在提高翻译质量方面优于分别翻译句子,即使在未经文档级别微调的情况下也是如此。然而,这一优势并没有体现在BLEU分数上,BLEU分数通常更倾向于基于句子的翻译。我们提出了使用LLM作为评判者的评估范式,利用GPT-4以更为细致的方式评估文档的连贯性、准确性和流畅性,而非基于n元语法的指标。总体而言,我们的工作表明,指令优化后的LLM可以有效地利用文档上下文进行翻译。但是,我们警告说,不应使用BLEU分数来评估docMT,因为它们在评估文档级别翻译的质量时常常提供误导性的结果。有关GPT4作为评判者的代码和输出可在https://github.com/EIT-NLP/BLEUless_DocMT找到。
论文及项目相关链接
PDF Accepted at NAACL 2025 Student Research Workshop
Summary:大型语言模型(LLM)在包括机器翻译(MT)在内的各种自然语言处理任务中表现出色。本研究关注指令训练LLM在文档级别翻译(docMT)上的内在能力。通过直接提示LLM一次性翻译整个文档,发现该方法相较于单独翻译句子能提高翻译质量,且无需对文档级别进行微调。然而,这一优势并未体现在BLEU分数上,因此提出使用LLM作为法官模式进行评估,利用GPT-4评估文档连贯性、准确性和流畅性。研究结果表明,指令训练LLM能有效利用文档上下文进行翻译。但警告称,对于docMT的评估,BLEU分数常给出误导性结果,无法准确反映文档级别翻译的质量。
Key Takeaways:
- LLM在文档级别翻译(docMT)上具有内在能力。
- 通过直接提示LLM翻译整个文档,可提高翻译质量。
- 相较于句子级翻译,LLM在文档级翻译中无需额外微调。
- BLEU分数无法全面反映文档级别翻译的质量。
- 提出使用LLM作为法官模式,利用GPT-4评估文档翻译的连贯性、准确性和流畅性。
- LLM能有效利用文档上下文进行翻译。
点此查看论文截图
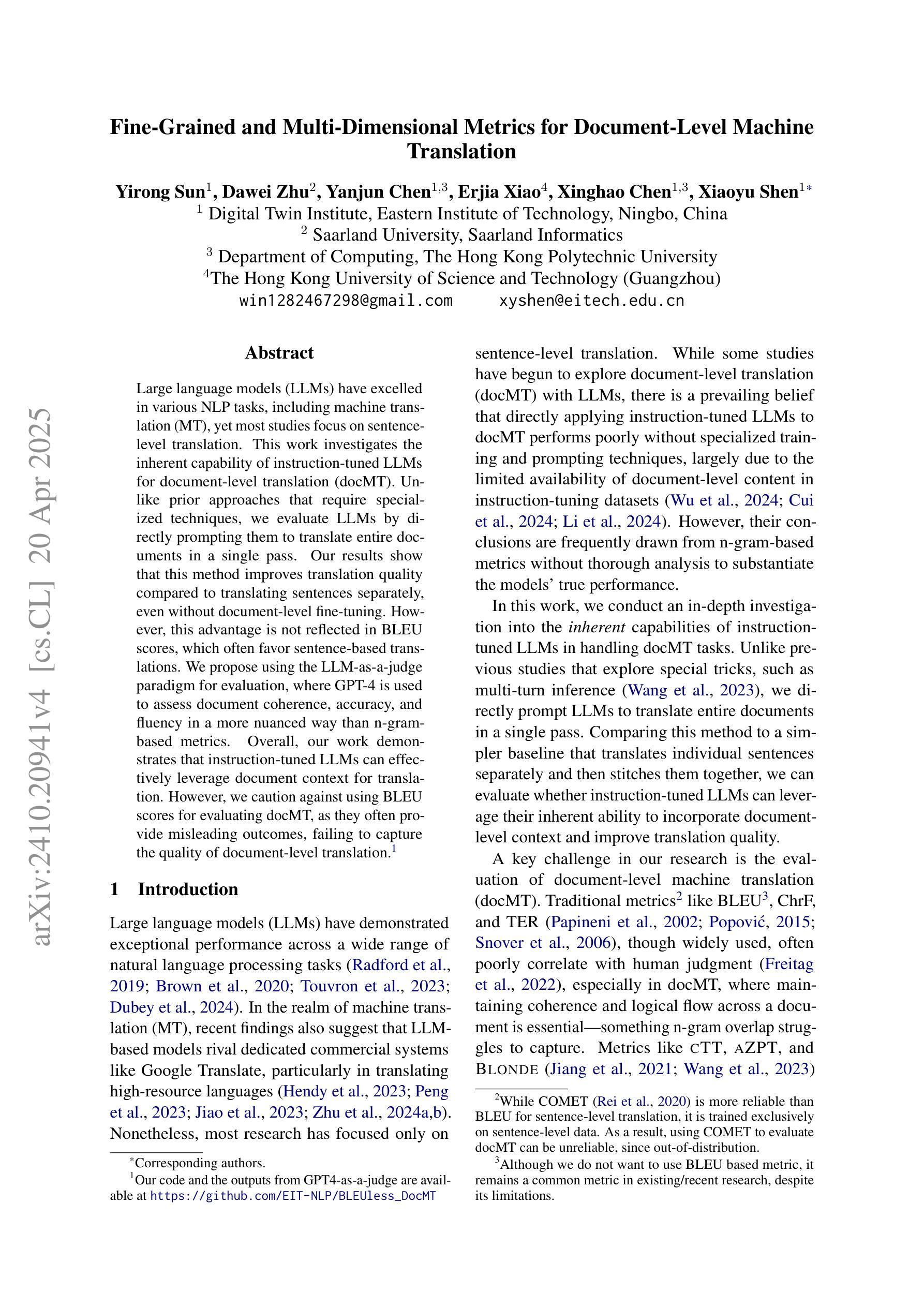
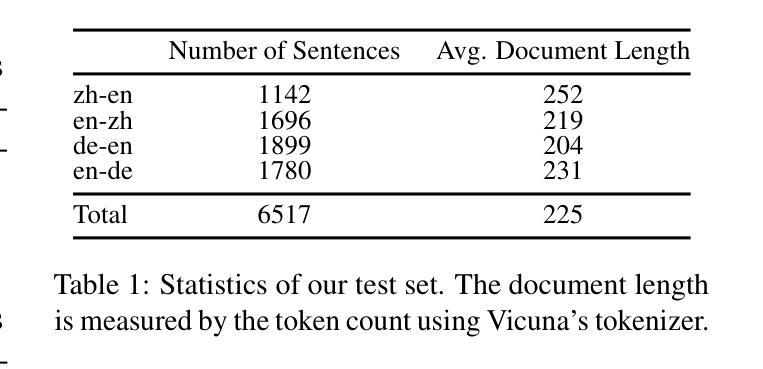
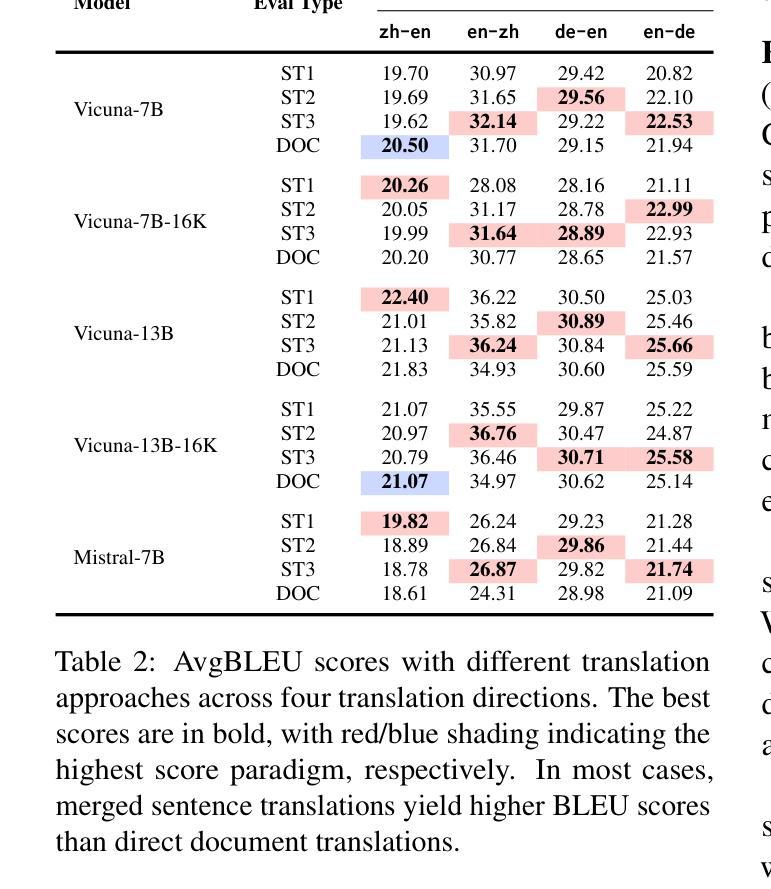
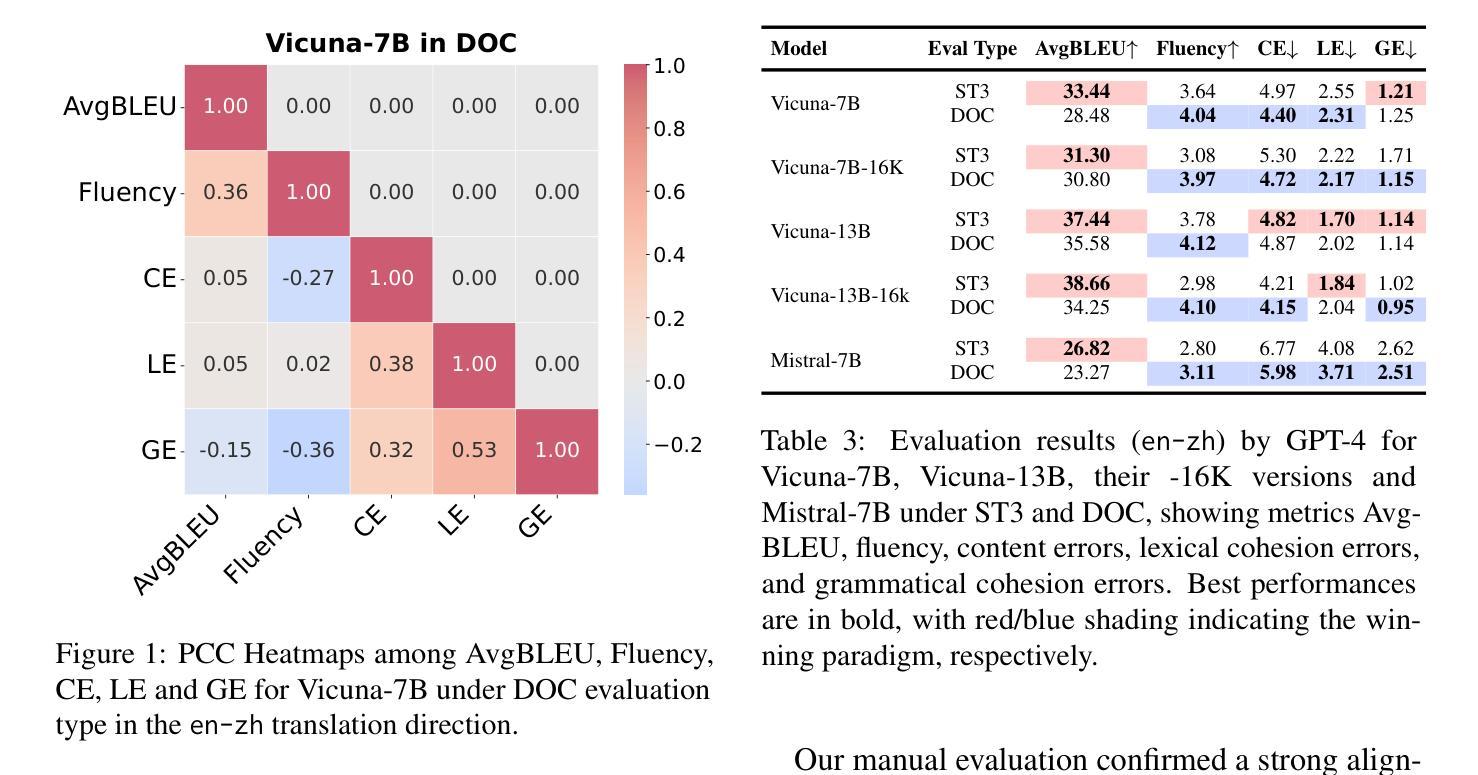
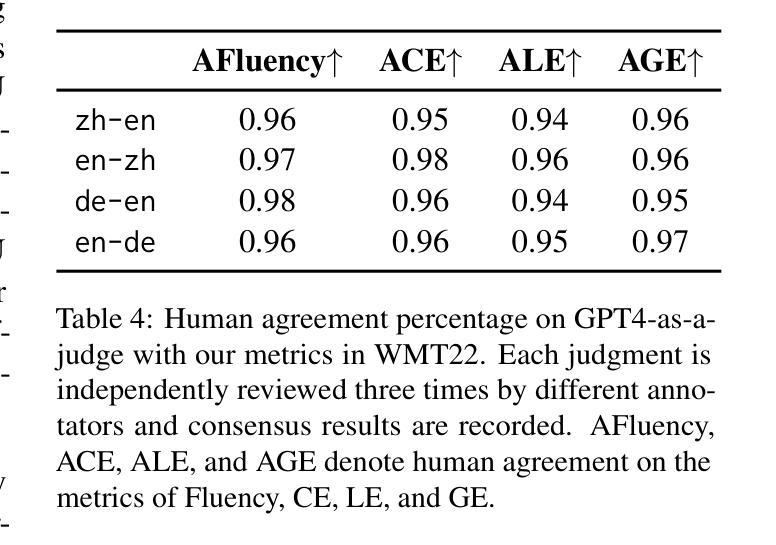
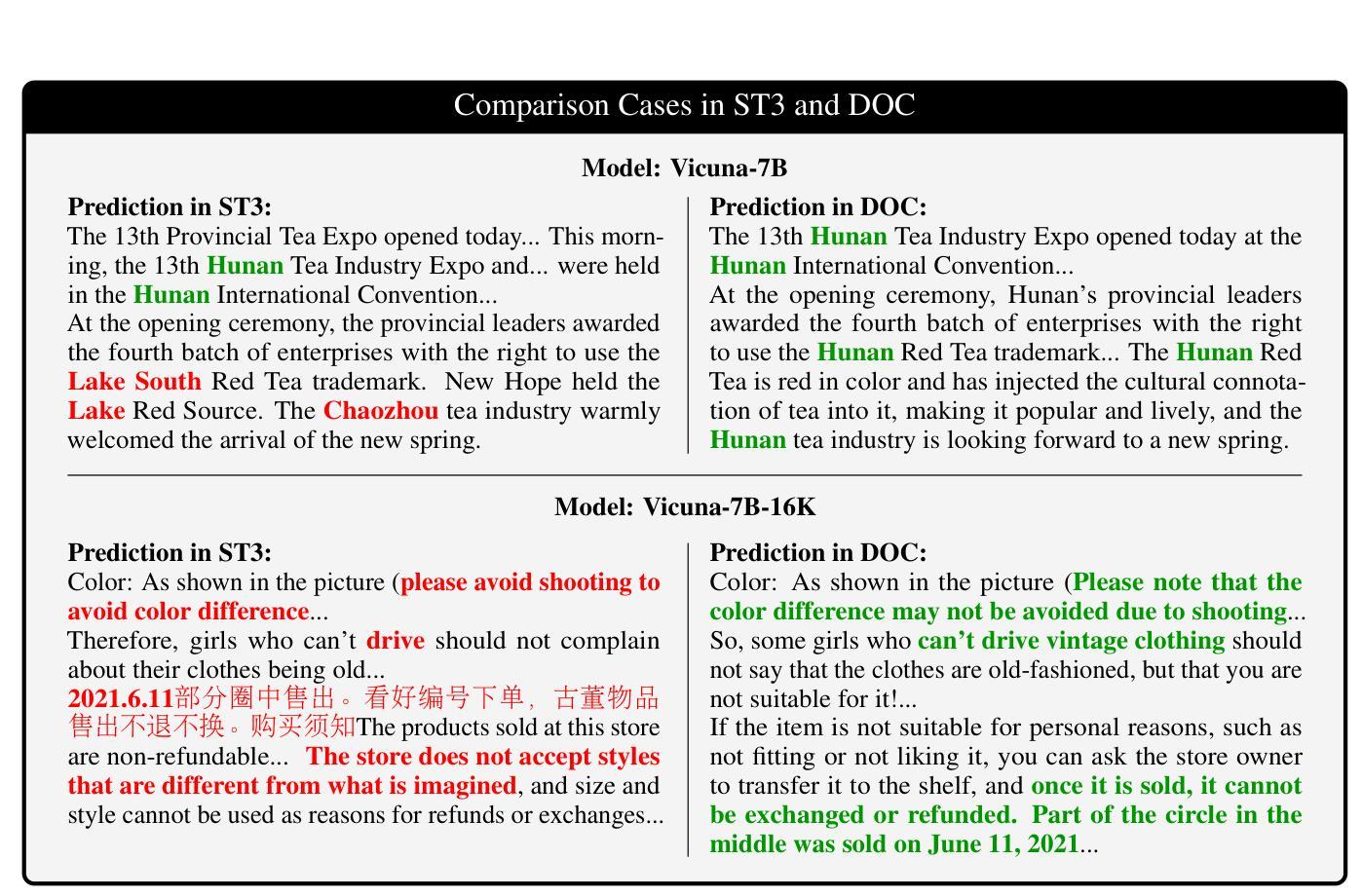
Context-Parametric Inversion: Why Instruction Finetuning Can Worsen Context Reliance
Authors:Sachin Goyal, Christina Baek, J. Zico Kolter, Aditi Raghunathan
A standard practice when using large language models is for users to supplement their instruction with an input context containing new information for the model to process. However, models struggle to reliably follow the input context, especially when it conflicts with their parametric knowledge from pretraining. In-principle, one would expect models to adapt to the user context better after instruction finetuning, particularly when handling knowledge conflicts. However, we observe a surprising failure mode: during instruction tuning, the context reliance under knowledge conflicts initially increases as expected, but then gradually decreases as instruction finetuning progresses. This happens while the performance on standard benchmarks keeps on increasing far after this drop. We call this phenomenon context-parametric inversion and observe it across multiple general purpose instruction tuning datasets such as TULU, Alpaca and Ultrachat, across different model families like Llama, Mistral, and Pythia. We perform various controlled studies and theoretical analysis to show that context-parametric inversion occurs due to examples in the instruction finetuning data where the input context provides information that aligns with model’s parametric knowledge. Our analysis suggests some natural mitigation strategies with limited but insightful gains, and serves as a useful starting point in addressing this deficiency in instruction finetuning.
在使用大型语言模型时,用户的标准做法是通过包含新信息的输入上下文来补充指令,以供模型处理。然而,模型在可靠地遵循输入上下文方面存在困难,尤其当它与预训练中的参数知识发生冲突时。原则上,人们会期望模型在指令微调后能更好地适应用户上下文,特别是在处理知识冲突时。然而,我们观察到了一种令人惊讶的失败模式:在指令调整过程中,知识冲突下的上下文依赖最初如预期增加,但随着指令微调的进行,它逐渐减少。这种现象发生在标准基准测试的性能持续提高之后。我们将这种现象称为“上下文参数反转”,并在多个通用指令微调数据集(如TULU、Alpaca和Ultrachat)以及不同模型家族(如Llama、Mistral和Pythia)中观察到它。我们进行了各种对照研究和理论分析,以表明上下文参数反转是由于指令微调数据中的示例导致的,其中输入上下文提供了与模型参数知识相符的信息。我们的分析提出了一些自然缓解策略,虽然收效有限但颇有见识,并为解决指令微调中的这一缺陷提供了有用的起点。
论文及项目相关链接
PDF Published at ICLR 2025 (Oral)
Summary
大型语言模型在使用时,用户通常会补充输入上下文以提供新信息供模型处理。然而,当上下文与模型的预训练参数知识发生冲突时,模型在可靠地遵循上下文方面会遇到困难。原则上,人们期望模型在指令微调后能更好地适应用户上下文,特别是在处理知识冲突时。但观察到一个意外情况:在指令调整过程中,虽然初始阶段上下文依赖程度如预期的那样增加,但随着指令微调的进行逐渐减少。这种情况发生在性能在标准基准测试上持续增加之后。我们将这种现象称为“上下文参数反转”,并在多个通用指令调整数据集(如TULU、Alpaca和Ultrachat)以及不同模型家族(如Llama、Mistral和Pythia)中观察到它。我们进行各种受控研究和理论分析,显示上下文参数反转是由于指令微调数据中的示例导致的,其中输入上下文提供了与模型参数知识相符的信息。我们的分析提出了一些自然缓解策略,虽然效果有限但颇具启发性,并为解决指令微调中的这一缺陷提供了一个有用的起点。
Key Takeaways
- 大型语言模型在处理与用户指令相关的上下文时可能遇到困难,尤其是在知识冲突的情况下。
- 在指令微调过程中,模型对上下文的依赖程度呈现先增后减的趋势。
- 这种趋势变化发生在模型在标准基准测试上的性能持续提高之后。
- 上下文参数反转现象在多个通用指令调整数据集和不同模型家族中均被观察到。
- 上下文参数反转是由于指令微调数据中的示例导致的,其中输入上下文与模型的参数知识相符。
- 我们的分析提出了自然缓解策略,虽然效果有限但具有启发性。
点此查看论文截图
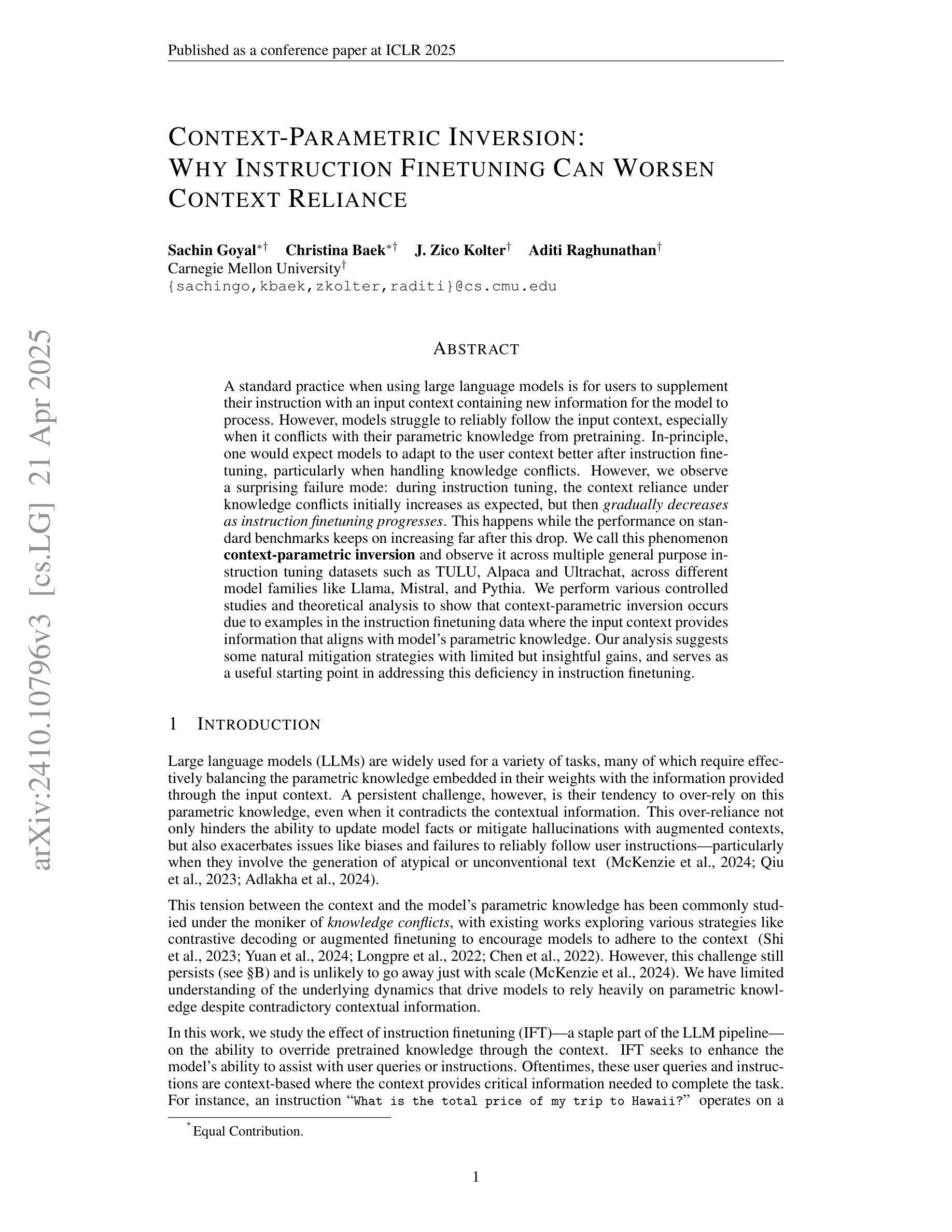
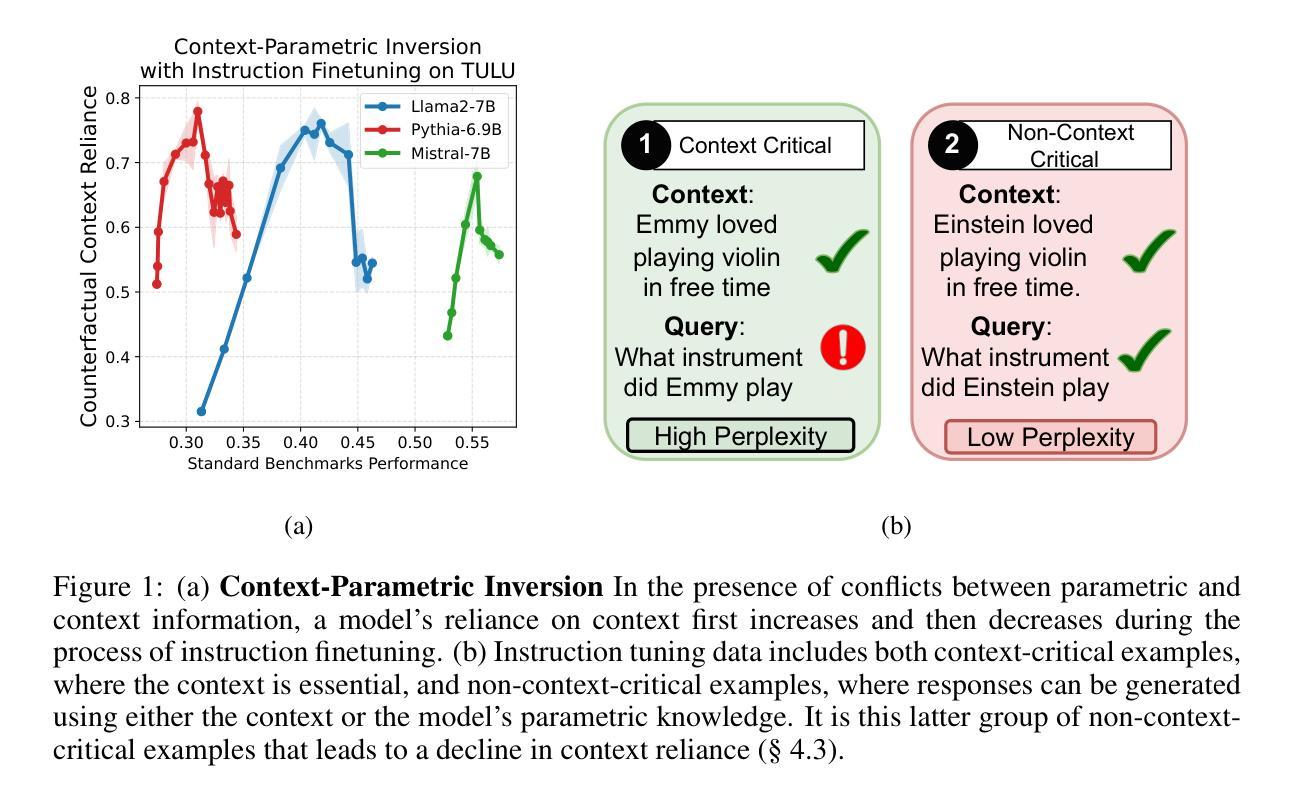
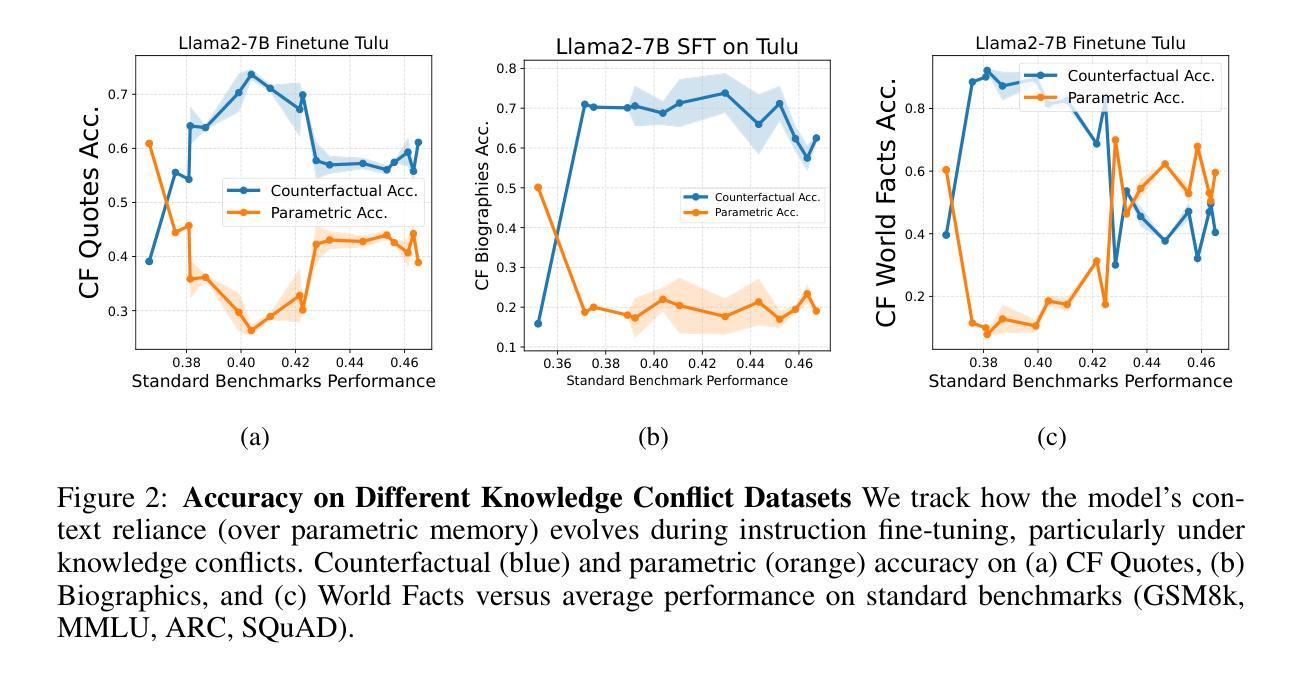
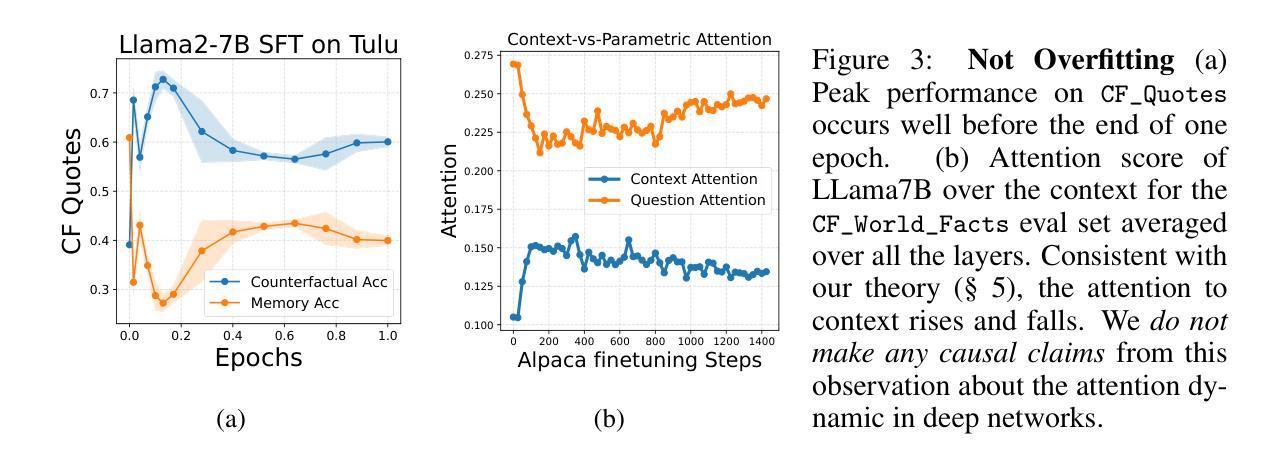
Selective Attention Improves Transformer
Authors:Yaniv Leviathan, Matan Kalman, Yossi Matias
Unneeded elements in the attention’s context degrade performance. We introduce Selective Attention, a simple parameter-free change to the standard attention mechanism which reduces attention to unneeded elements. Selective attention consistently improves language modeling and downstream task performance in a variety of model sizes and context lengths. For example, transformers trained with the language modeling objective on C4 with selective attention perform language modeling equivalently to standard transformers with ~2X more heads and parameters in their attention modules. Selective attention also allows decreasing the size of the attention’s context buffer, leading to meaningful reductions in the memory and compute requirements during inference. For example, transformers trained on C4 with context sizes of 512, 1,024, and 2,048 need 16X, 25X, and 47X less memory for their attention module, respectively, when equipped with selective attention, as those without selective attention, with the same validation perplexity.
注意力上下文中的多余元素会降低性能。我们引入了选择性注意力机制,这是对标准注意力机制的简单无参数改进,可以减少对不需要元素的关注。选择性注意力在各种模型大小和上下文长度中都能提高语言建模和下游任务性能。例如,在C4上使用语言建模目标训练的变压器,通过选择性注意力机制,其语言建模能力与标准变压器相当,但注意力模块中的头数和参数增加了约两倍。选择性注意力还允许减小注意力上下文缓冲区的大小,从而减少了推理过程中的内存和计算需求。例如,在C4上训练的上下文大小为512、1024和2048的变压器,在使用选择性注意力后,其注意力模块的所需内存分别减少了无需选择性注意力的模型的16倍、25倍和47倍,同时保持了相同的验证困惑度。
论文及项目相关链接
PDF ICLR 2025
Summary:选择性注意力机制能有效减少不必要的元素关注度,从而提升语言模型和下游任务的性能表现。相较于传统注意力机制,选择性注意力可在减少参数的同时,提高模型性能。此外,选择性注意力还能降低注意力上下文缓冲区的规模,从而减少推理过程中的内存和计算需求。
Key Takeaways:
- 选择性注意力机制有助于减少不必要的元素关注度。
- 选择性注意力机制可以提高语言模型和下游任务的性能表现。
- 选择性注意力机制在减少参数的同时提升模型性能。
- 选择性注意力机制可降低注意力上下文缓冲区规模。
- 选择性注意力有助于减少推理过程中的内存需求。
- 选择性注意力有助于减少推理过程中的计算需求。
点此查看论文截图
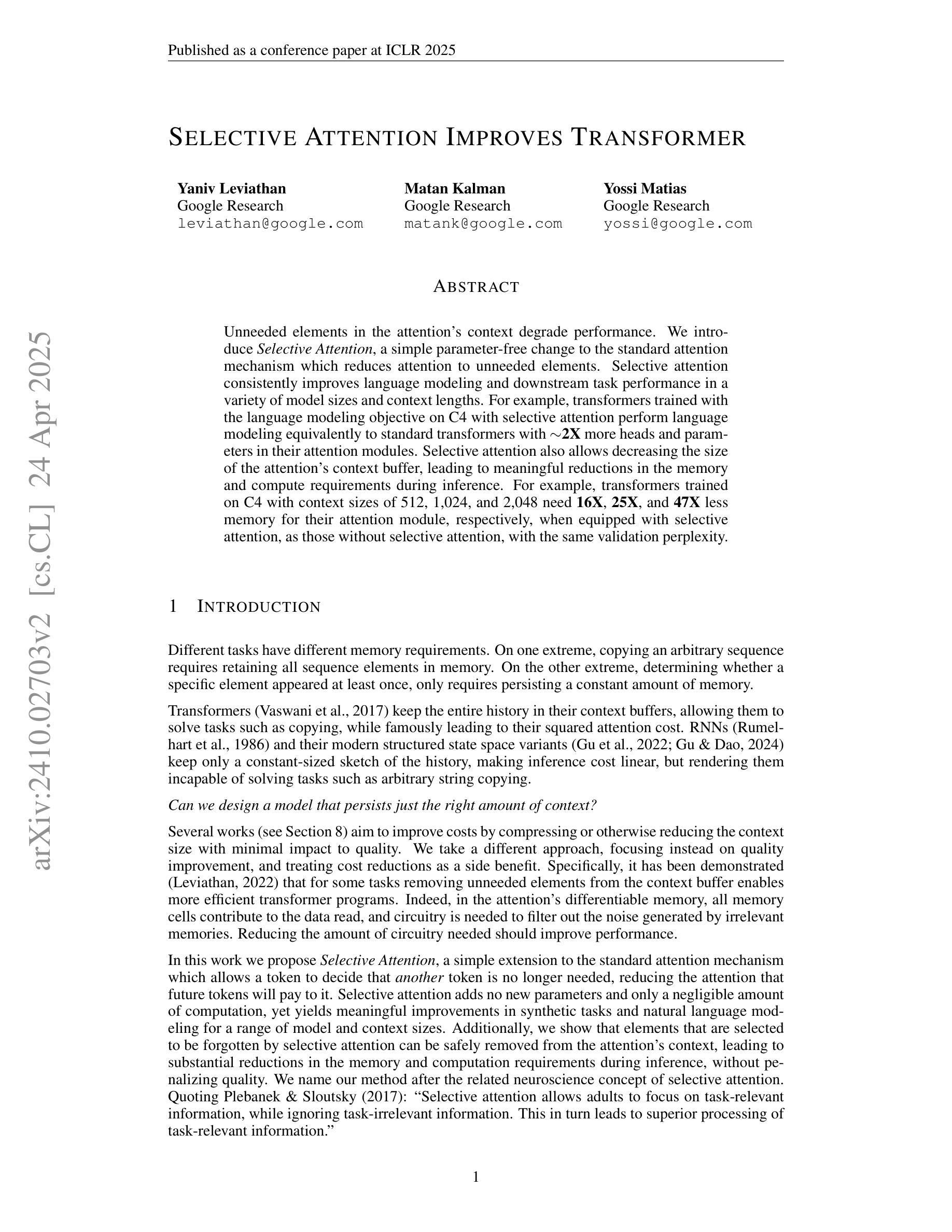
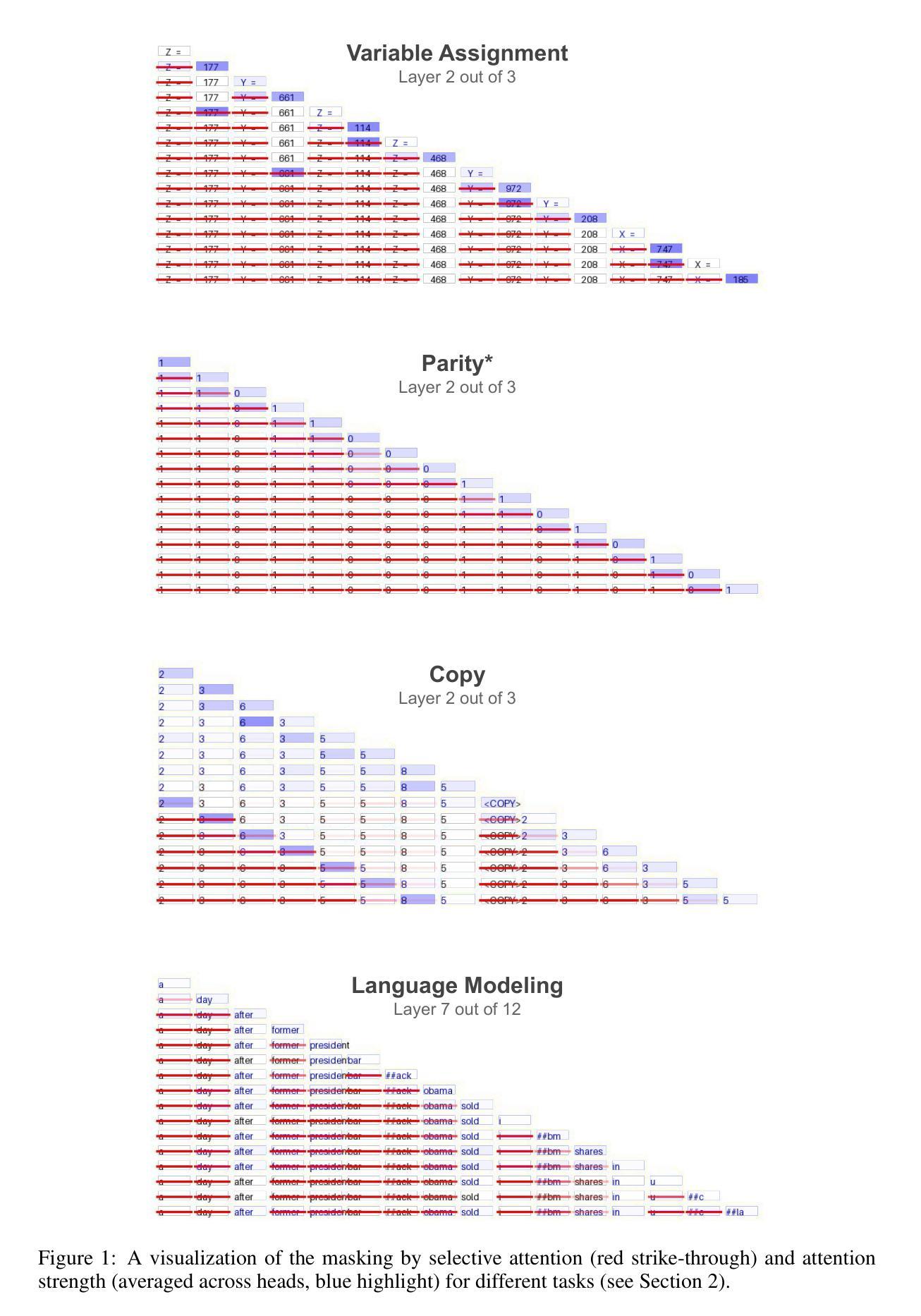
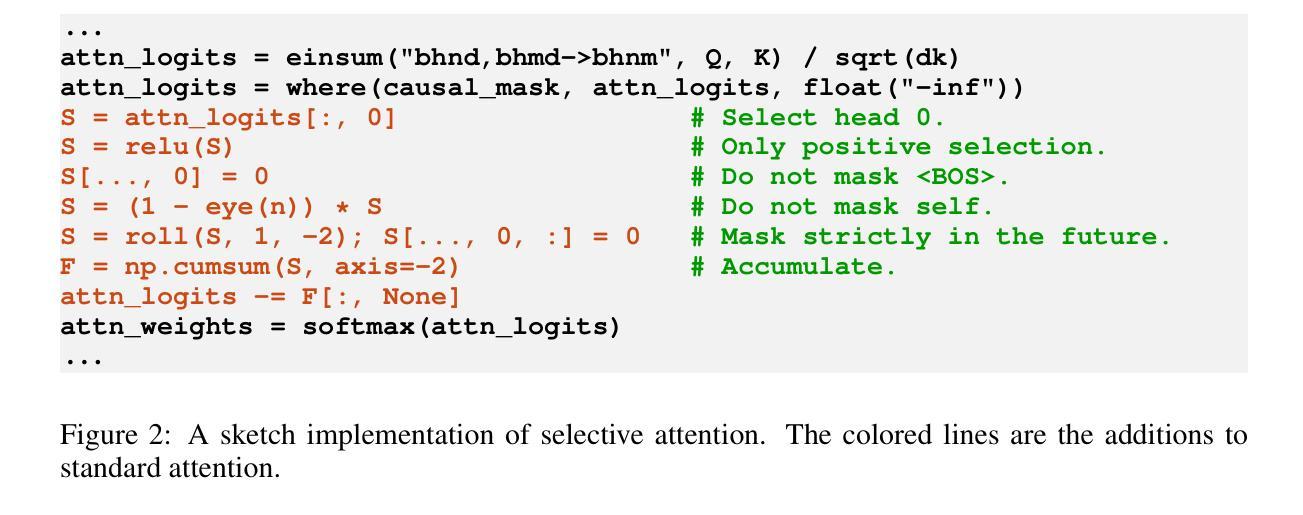
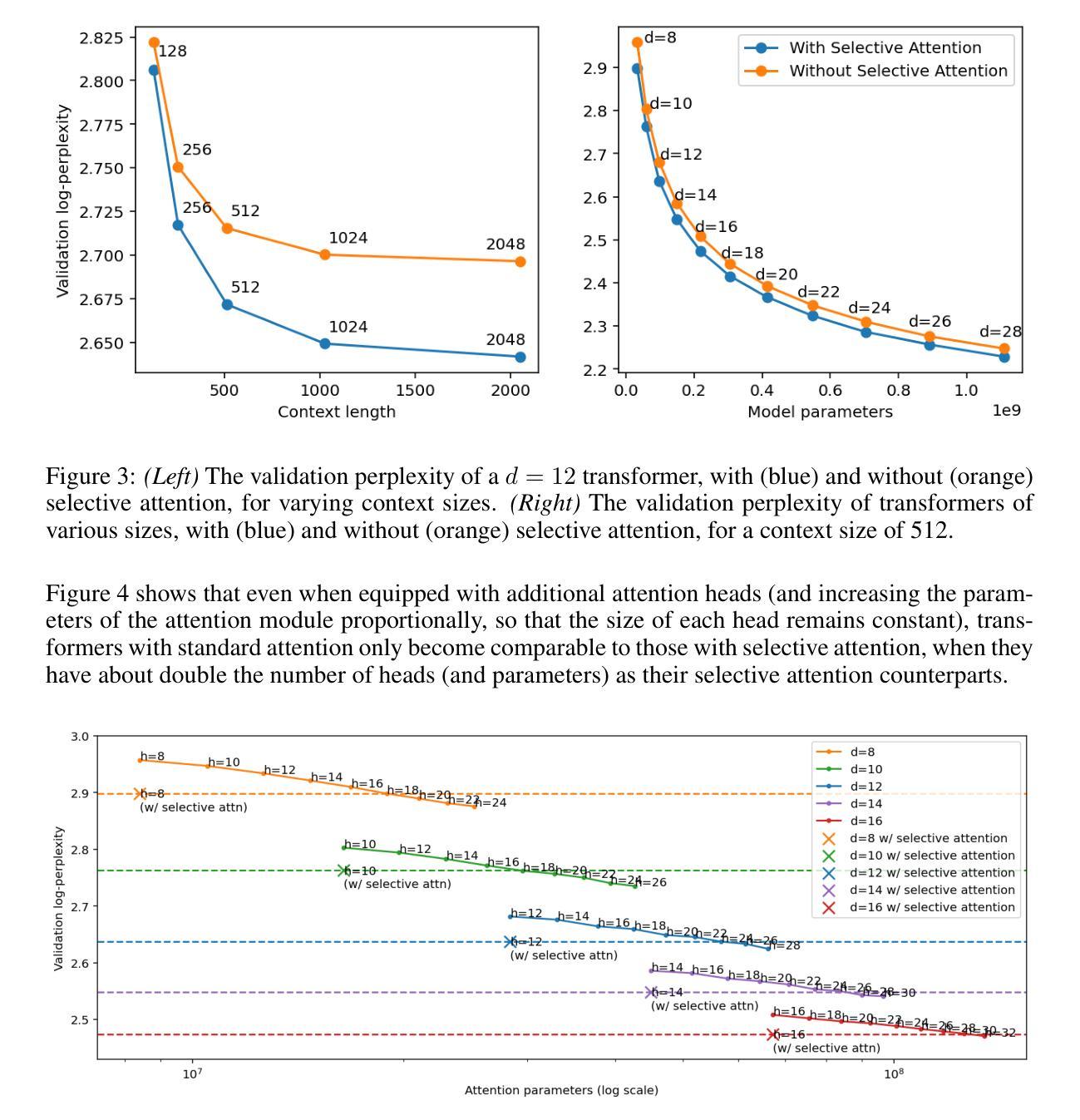
Measuring and Enhancing Trustworthiness of LLMs in RAG through Grounded Attributions and Learning to Refuse
Authors:Maojia Song, Shang Hong Sim, Rishabh Bhardwaj, Hai Leong Chieu, Navonil Majumder, Soujanya Poria
LLMs are an integral component of retrieval-augmented generation (RAG) systems. While many studies focus on evaluating the overall quality of end-to-end RAG systems, there is a gap in understanding the appropriateness of LLMs for the RAG task. To address this, we introduce Trust-Score, a holistic metric that evaluates the trustworthiness of LLMs within the RAG framework. Our results show that various prompting methods, such as in-context learning, fail to effectively adapt LLMs to the RAG task as measured by Trust-Score. Consequently, we propose Trust-Align, a method to align LLMs for improved Trust-Score performance. 26 out of 27 models aligned using Trust-Align substantially outperform competitive baselines on ASQA, QAMPARI, and ELI5. Specifically, in LLaMA-3-8b, Trust-Align outperforms FRONT on ASQA (up 12.56), QAMPARI (up 36.04), and ELI5 (up 17.69). Trust-Align also significantly enhances models’ ability to correctly refuse and provide quality citations. We also demonstrate the effectiveness of Trust-Align across different open-weight models, including the LLaMA series (1b to 8b), Qwen-2.5 series (0.5b to 7b), and Phi3.5 (3.8b). We release our code at https://github.com/declare-lab/trust-align.
LLM是检索增强生成(RAG)系统的核心组成部分。尽管许多研究专注于评估端到端RAG系统的整体质量,但对于LLM在RAG任务中的适用性仍存在理解上的空白。为了解决这一问题,我们引入了Trust-Score,这是一个全面评估LLM在RAG框架内可信度的指标。我们的结果表明,像上下文学习这样的各种提示方法,无法有效地使LLM适应由Trust-Score衡量的RAG任务。因此,我们提出了Trust-Align方法,以对LLM进行对齐,以提高Trust-Score的性能。在ASQA、QAMPARI和ELI5上,使用Trust-Align对齐的26个模型中的大多数都显著优于竞争基线。具体来说,在LLaMA-3-8b中,Trust-Align在ASQA(提高12.56)、QAMPARI(提高36.04)和ELI5(提高17.69)上的表现均优于FRONT。Trust-Align还显著提高了模型正确拒绝和提供高质量引用的能力。我们还证明了Trust-Align在不同开放权重模型中的有效性,包括LLaMA系列(1b到8b)、Qwen-2.5系列(0.5b到7b)和Phi3.5(3.8b)。我们已将代码发布在https://github.com/declare-lab/trust-align。
论文及项目相关链接
PDF Published at ICLR 2025 (Oral)
Summary
LLMs在检索增强生成(RAG)系统中扮演重要角色。针对LLMs在RAG任务中的适用性,引入Trust-Score这一全面评估指标。研究结果显示,上下文学习等提示方法无法有效适应RAG任务,而Trust-Align方法则可提高LLMs的Trust-Score表现。采用Trust-Align对齐的26个模型中,大部分在ASQA、QAMPARI和ELI5等多个基准测试中表现优越。Trust-Align可显著提高模型拒绝不当引用和提供高质量引用的能力。该方法适用于多种规模不同的开源模型。
Key Takeaways
- LLMs是RAG系统的重要组成部分。
- 现有研究存在对LLMs在RAG任务中适用性的理解差距。
- Trust-Score被引入作为评估LLMs在RAG框架中可信度的全面指标。
- 提示方法(如上下文学习)未能有效适应RAG任务,表现不佳。
- Trust-Align方法能提高LLMs的Trust-Score表现。
- 采用Trust-Align对齐的模型在多个基准测试中表现优越。
点此查看论文截图
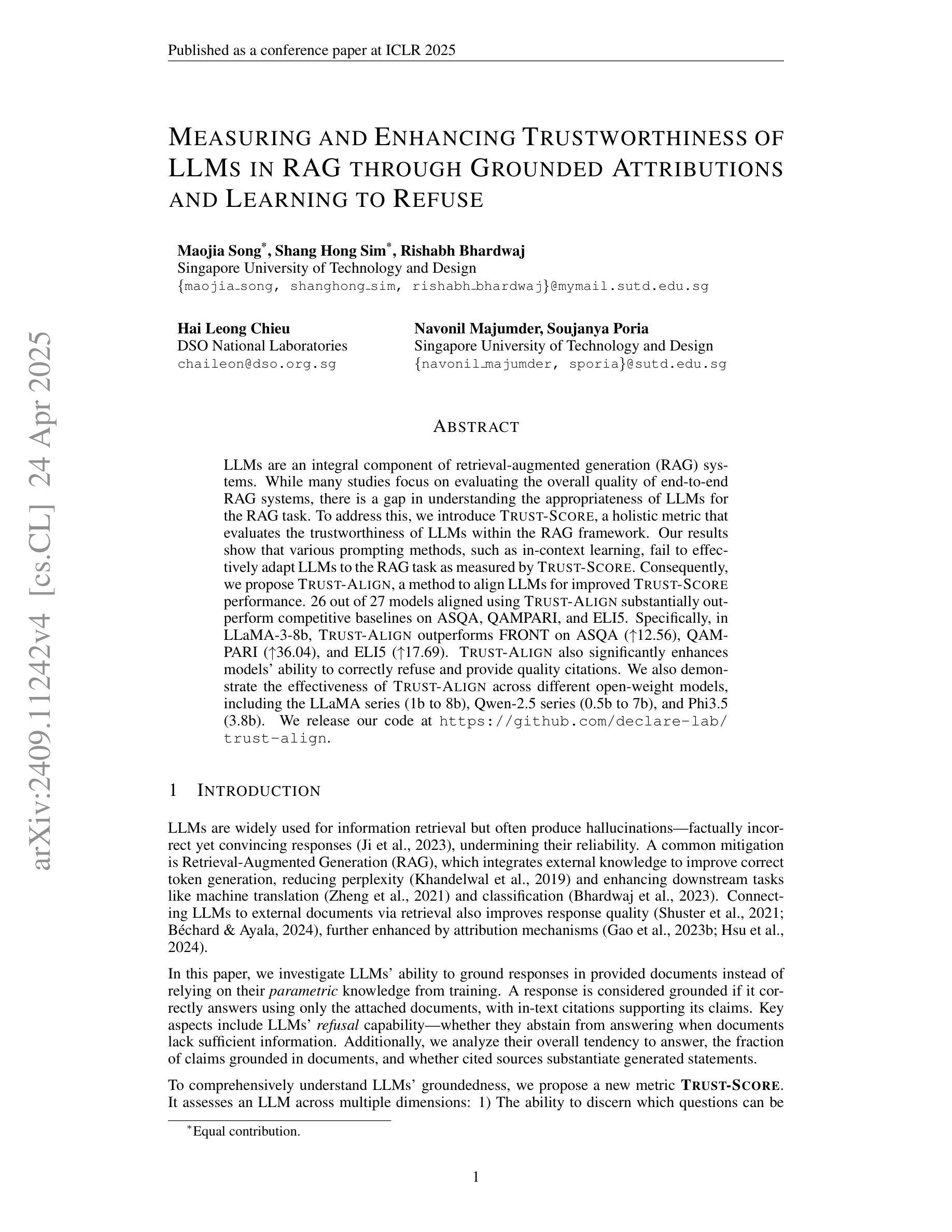
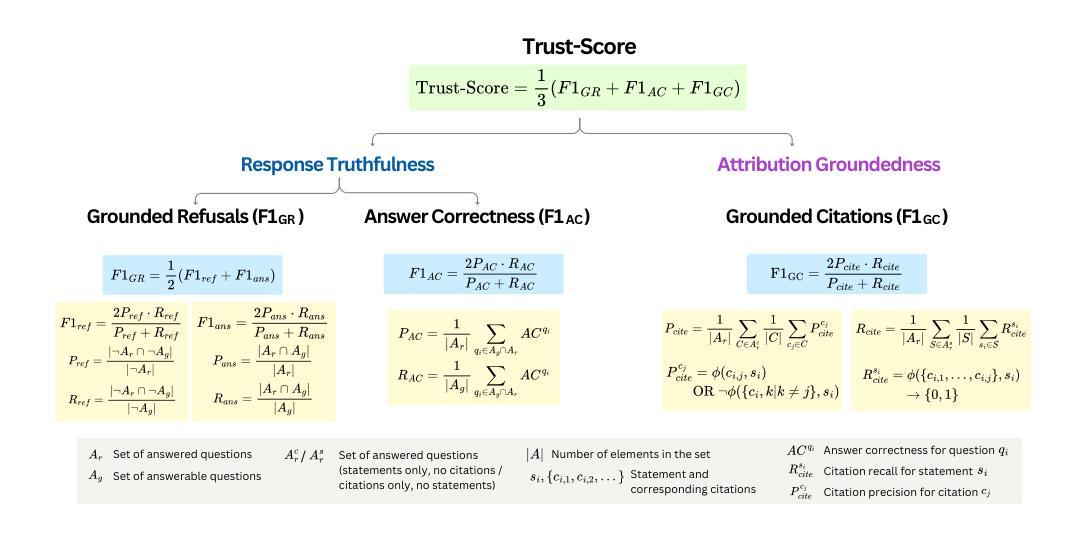
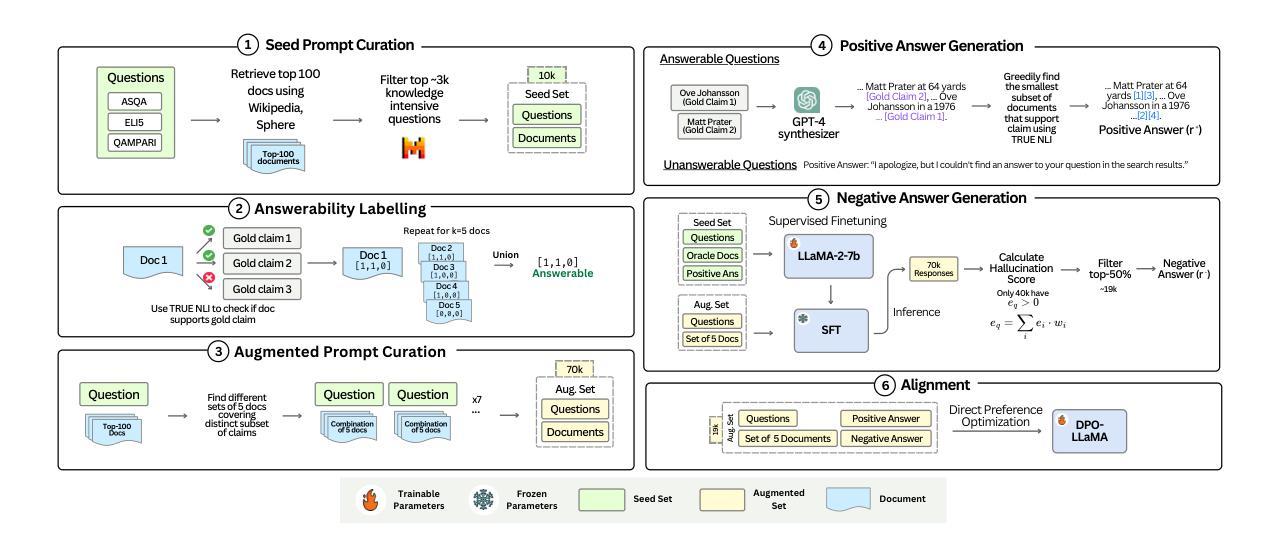
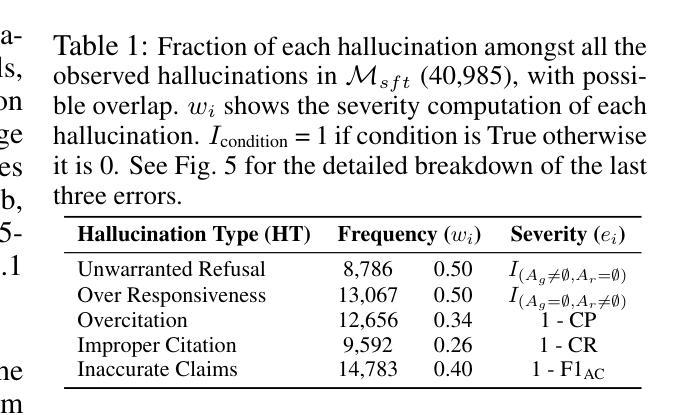
Large Language Model for Verilog Generation with Code-Structure-Guided Reinforcement Learning
Authors:Ning Wang, Bingkun Yao, Jie Zhou, Xi Wang, Zhe Jiang, Nan Guan
Recent advancements in large language models (LLMs) have sparked significant interest in the automatic generation of Register Transfer Level (RTL) designs, particularly using Verilog. Current research on this topic primarily focuses on pre-training and instruction tuning, but the effectiveness of these methods is constrained by the limited availability of training data, as public Verilog code is far less abundant than software code. In particular, these methods struggle to effectively capture Verilog parallel code structures, which fundamentally differ from the imperative, sequential control flow typical in most software programming languages. This paper introduces VeriSeek, an LLM enhanced by reinforcement learning using a limited amount of high-quality training data to achieve high Verilog code generation performance. Our reinforcement learning approach employs code structure information as feedback signals to refine the pre-trained model, enabling it to effectively learn important patterns from Verilog code with parallel structures. Experiments show that VeriSeek outperforms state-of-the-art methods across multiple benchmarks.
最近大型语言模型(LLM)的进展引发了人们对自动生成寄存器传输级别(RTL)设计的浓厚兴趣,特别是使用Verilog设计。当前关于这一主题的研究主要集中在预训练和指令调整上,但这些方法的有效性受到训练数据有限性的制约,因为公共Verilog代码比软件代码要少得多。尤其这些方法在捕获Verilog并行代码结构时遇到困难,这些结构与大多数软件编程语言中的命令式、顺序控制流根本不同。本文介绍了VeriSeek,这是一个通过强化学习增强的大型语言模型,使用有限的高质量训练数据实现高性能的Verilog代码生成。我们的强化学习方法采用代码结构信息作为反馈信号来优化预训练模型,使其能够有效地从具有并行结构的Verilog代码中学习重要模式。实验表明,VeriSeek在多个基准测试上优于最新技术的方法。
论文及项目相关链接
Summary
近期大型语言模型(LLM)在自动生成寄存器传输级(RTL)设计,特别是使用Verilog语言方面取得显著进展。研究主要集中于预训练和指令调整,但受限于公共Verilog代码较软件代码稀缺,这些方法的效果有限。本文提出VeriSeek,通过强化学习增强LLM,利用有限的高质量训练数据实现高性能的Verilog代码生成。实验表明,VeriSeek在多个基准测试中表现优于现有方法。
Key Takeaways
- 大型语言模型(LLM)在自动生成寄存器传输级(RTL)设计方面取得进展。
- 研究集中在预训练和指令调整上,但受限于Verilog代码的稀缺性。
- Verilog并行代码结构与大多数软件编程语言中的命令式、顺序控制流存在根本差异。
- 提出的VeriSeek采用强化学习增强LLM,有效利用有限的高质量训练数据。
- VeriSeek通过利用代码结构信息作为反馈信号来优化预训练模型。
- VeriSeek能有效学习Verilog代码中的重要模式,尤其是并行结构。
点此查看论文截图
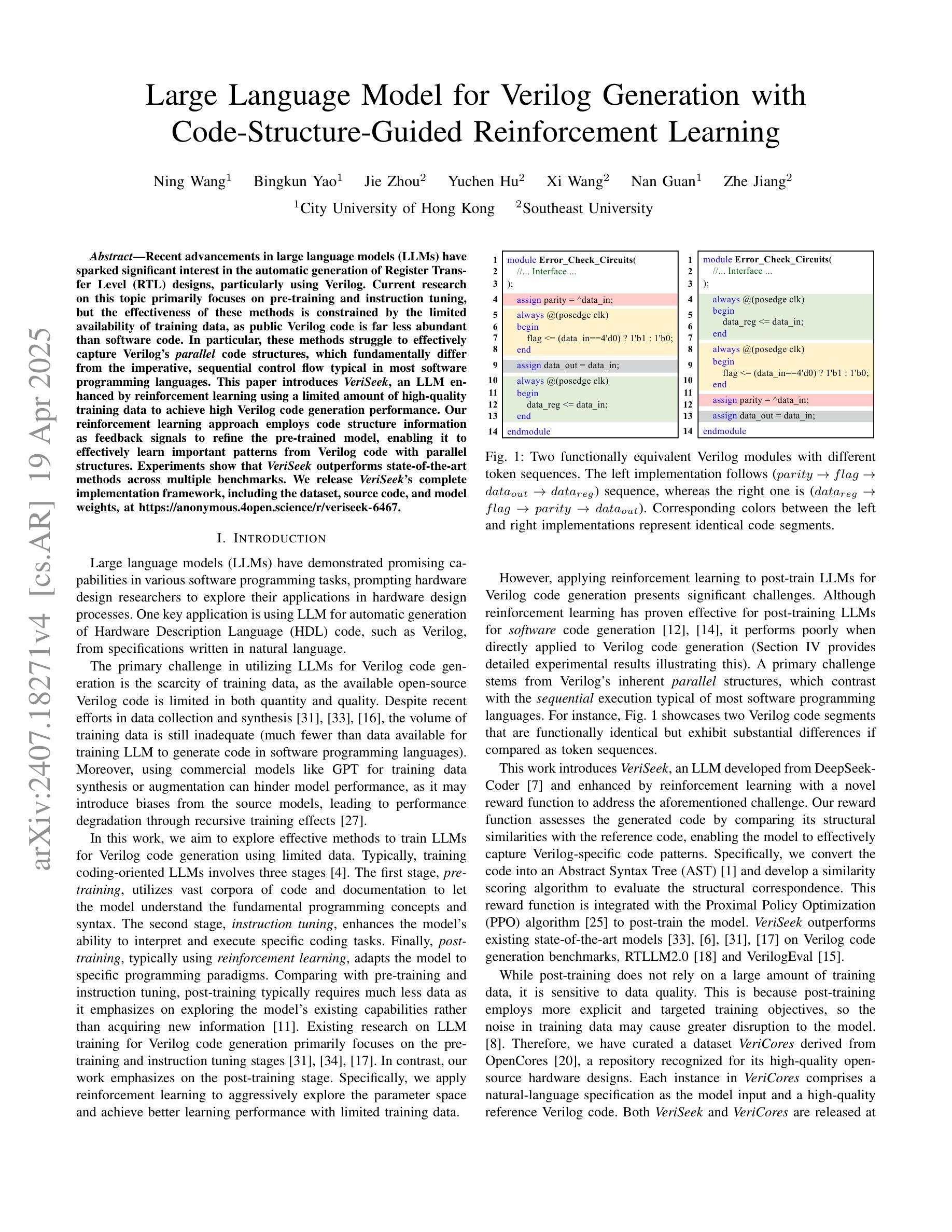
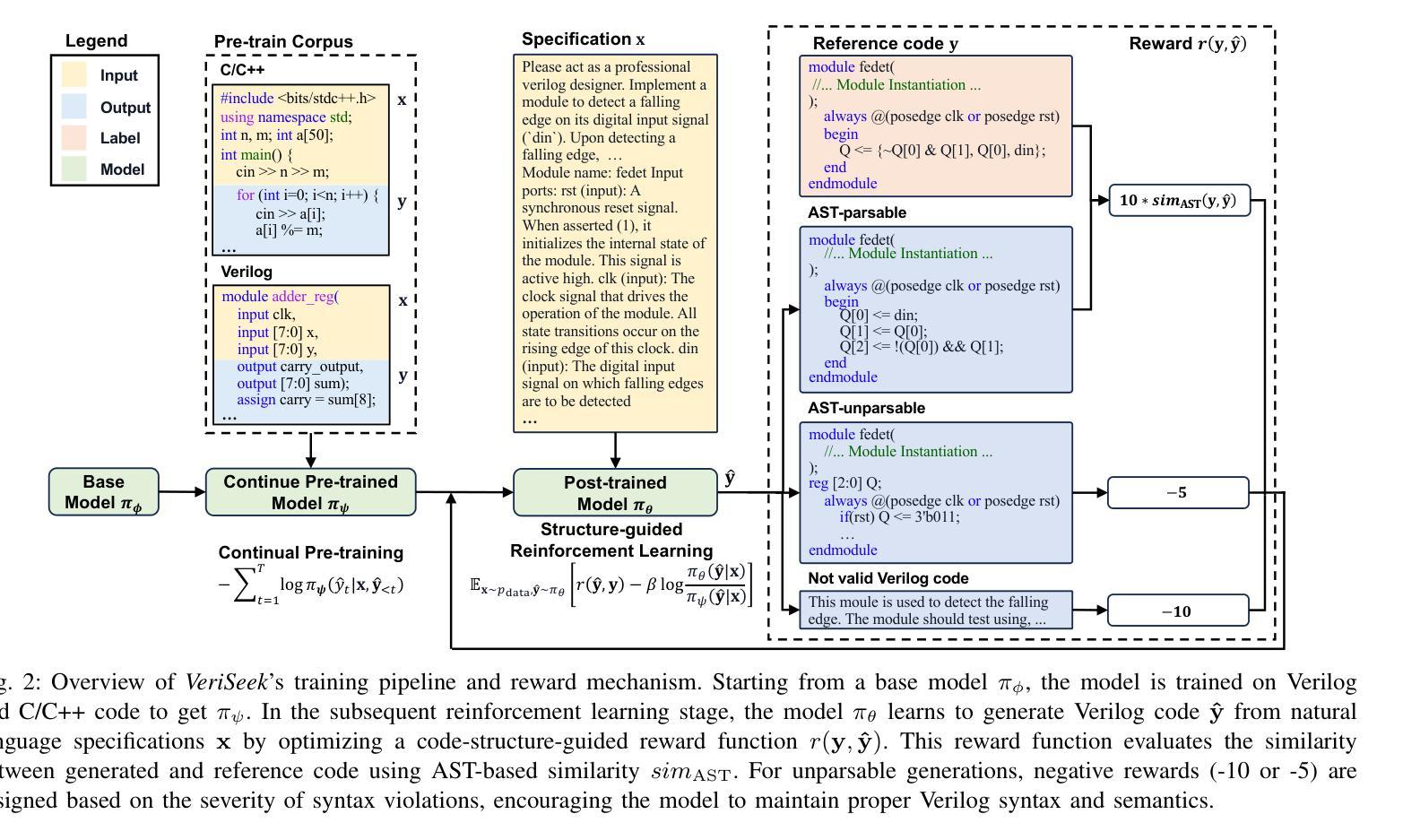
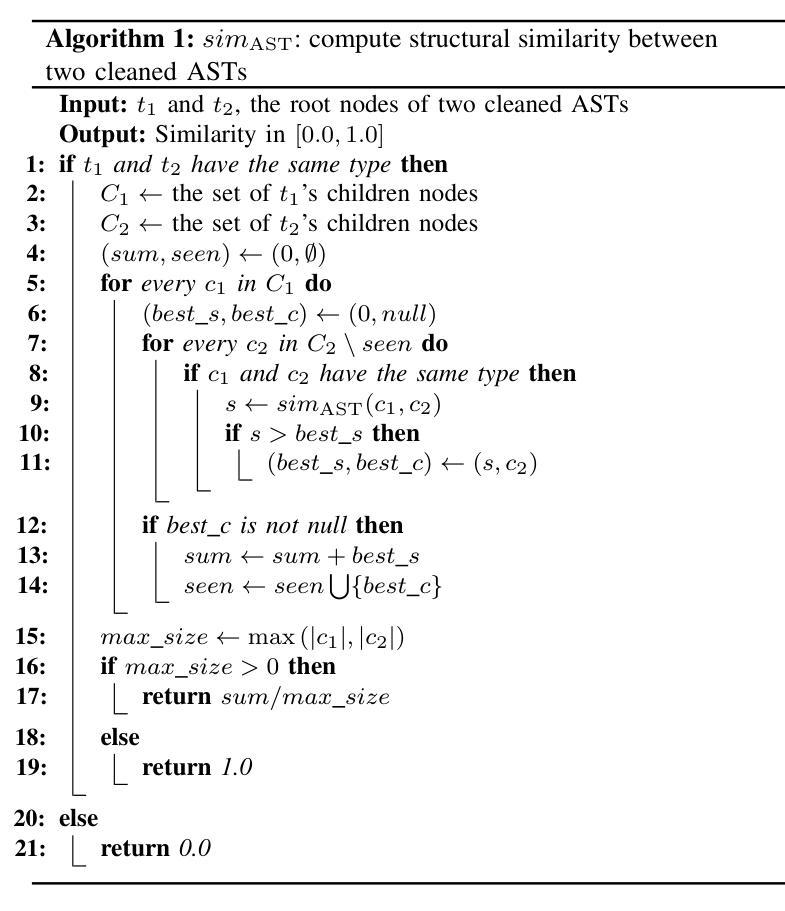
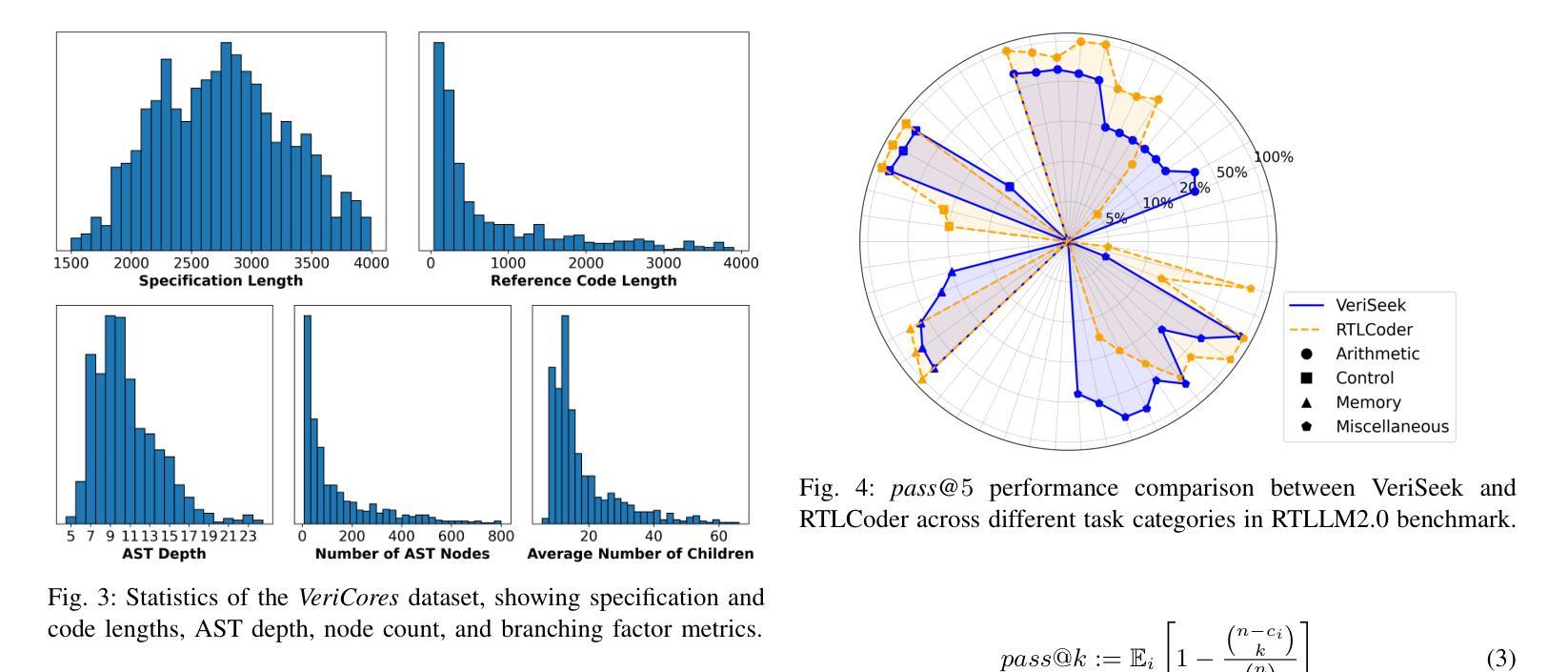
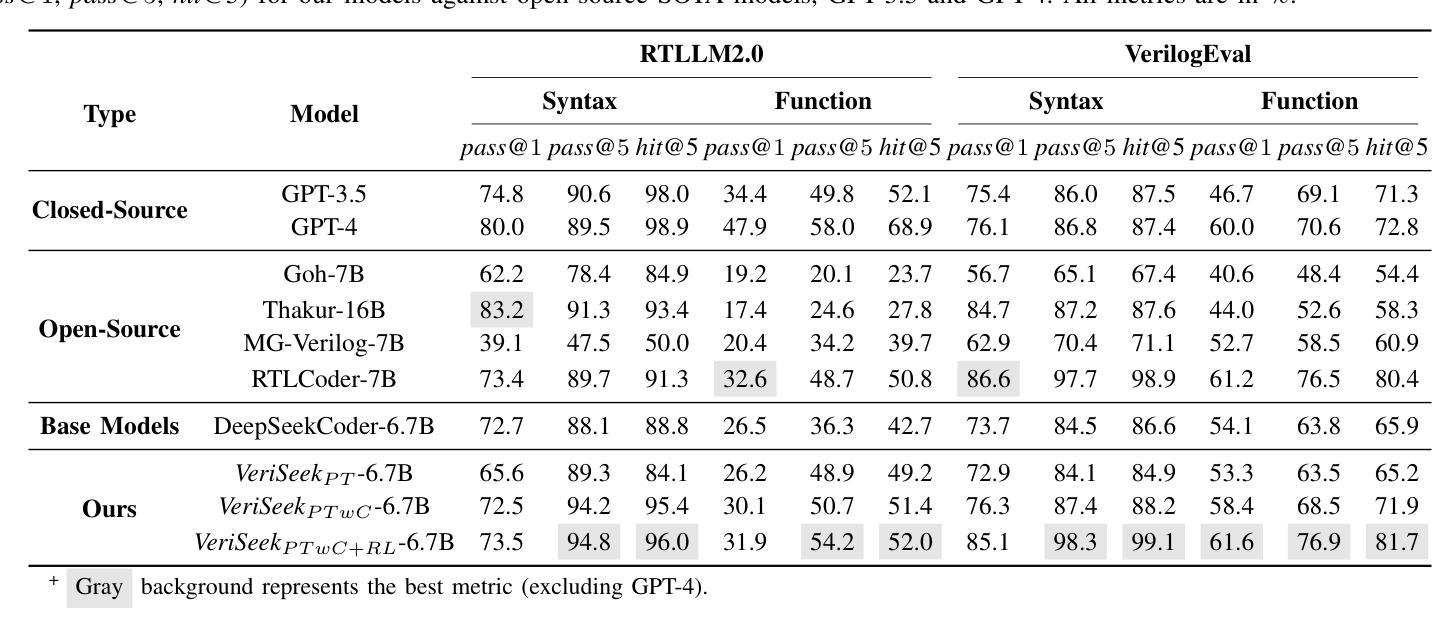
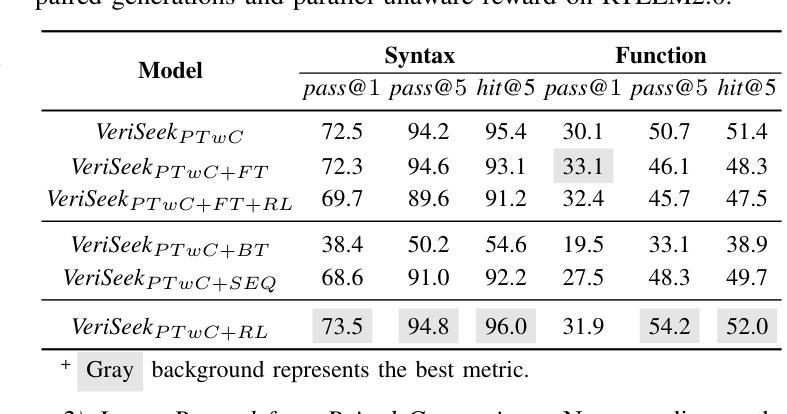
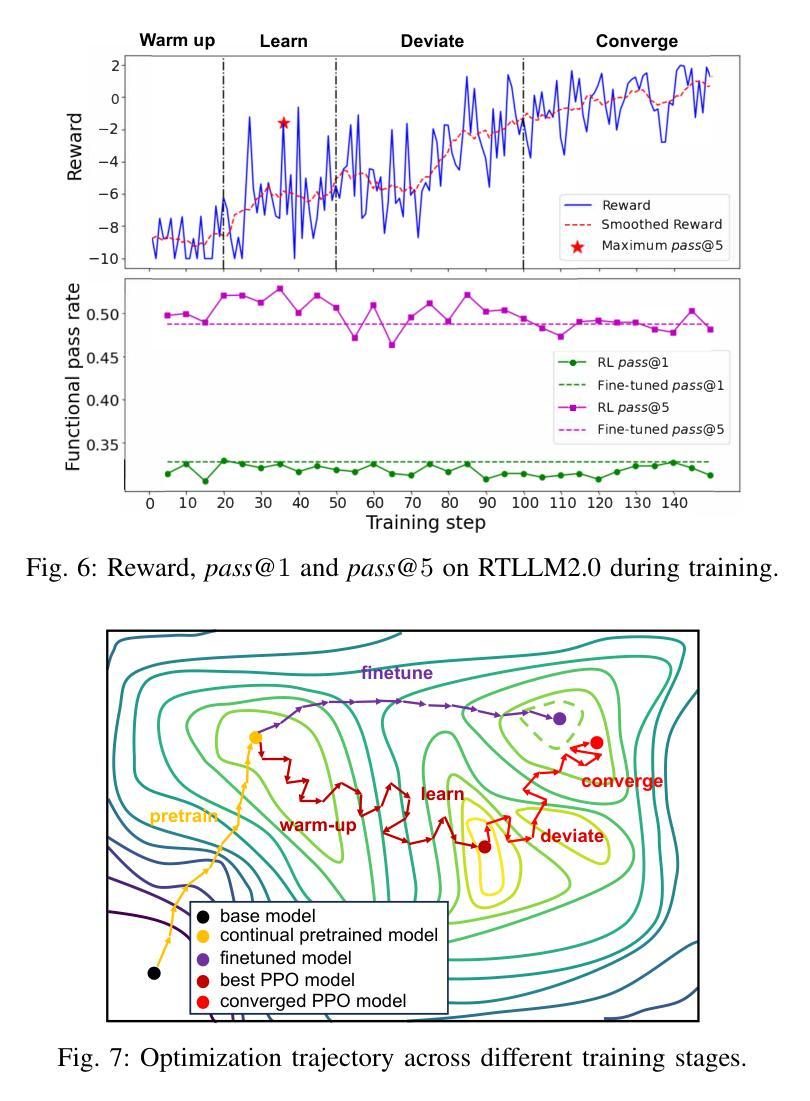
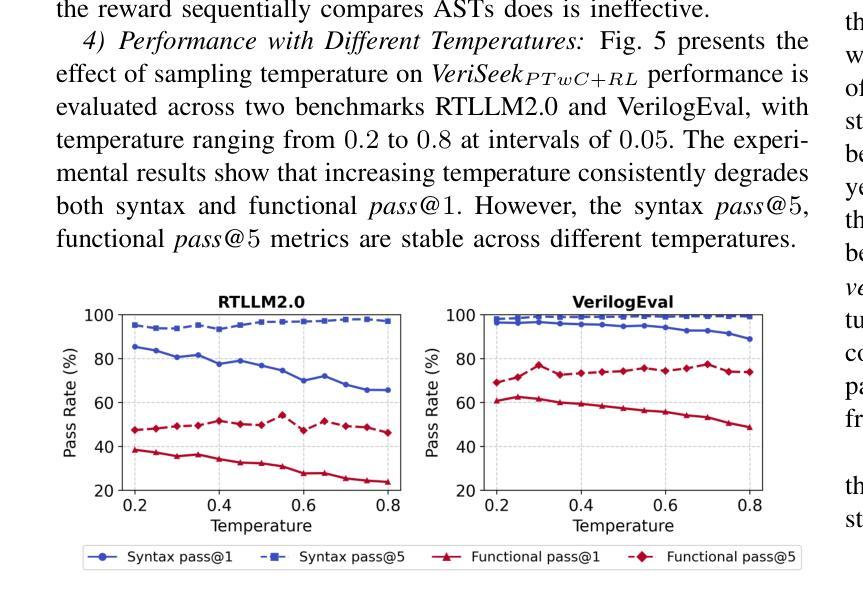
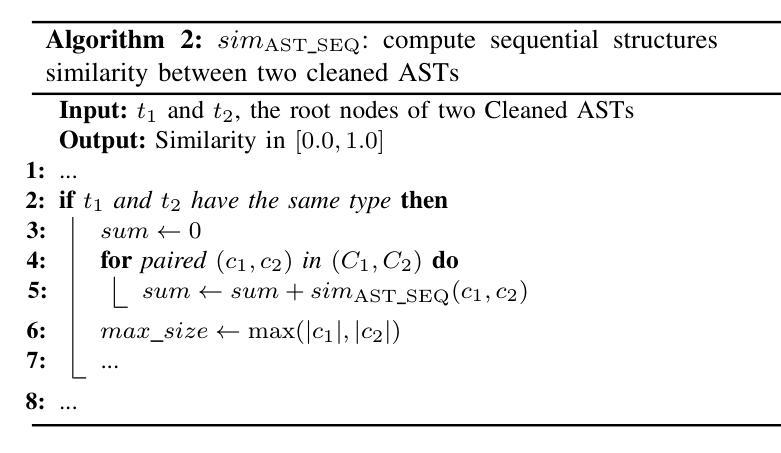