⚠️ 以下所有内容总结都来自于 大语言模型的能力,如有错误,仅供参考,谨慎使用
🔴 请注意:千万不要用于严肃的学术场景,只能用于论文阅读前的初筛!
💗 如果您觉得我们的项目对您有帮助 ChatPaperFree ,还请您给我们一些鼓励!⭐️ HuggingFace免费体验
2025-04-26 更新
Mamba-Sea: A Mamba-based Framework with Global-to-Local Sequence Augmentation for Generalizable Medical Image Segmentation
Authors:Zihan Cheng, Jintao Guo, Jian Zhang, Lei Qi, Luping Zhou, Yinghuan Shi, Yang Gao
To segment medical images with distribution shifts, domain generalization (DG) has emerged as a promising setting to train models on source domains that can generalize to unseen target domains. Existing DG methods are mainly based on CNN or ViT architectures. Recently, advanced state space models, represented by Mamba, have shown promising results in various supervised medical image segmentation. The success of Mamba is primarily owing to its ability to capture long-range dependencies while keeping linear complexity with input sequence length, making it a promising alternative to CNNs and ViTs. Inspired by the success, in the paper, we explore the potential of the Mamba architecture to address distribution shifts in DG for medical image segmentation. Specifically, we propose a novel Mamba-based framework, Mamba-Sea, incorporating global-to-local sequence augmentation to improve the model’s generalizability under domain shift issues. Our Mamba-Sea introduces a global augmentation mechanism designed to simulate potential variations in appearance across different sites, aiming to suppress the model’s learning of domain-specific information. At the local level, we propose a sequence-wise augmentation along input sequences, which perturbs the style of tokens within random continuous sub-sequences by modeling and resampling style statistics associated with domain shifts. To our best knowledge, Mamba-Sea is the first work to explore the generalization of Mamba for medical image segmentation, providing an advanced and promising Mamba-based architecture with strong robustness to domain shifts. Remarkably, our proposed method is the first to surpass a Dice coefficient of 90% on the Prostate dataset, which exceeds previous SOTA of 88.61%. The code is available at https://github.com/orange-czh/Mamba-Sea.
针对存在分布偏移的医疗图像分割问题,领域泛化(DG)已成为一种在源领域训练模型并推广至未见目标领域的颇具前景的设置。现有的DG方法主要基于CNN或ViT架构。最近,以Mamba为代表的高级状态空间模型在各种有监督医疗图像分割中取得了令人鼓舞的结果。Mamba的成功主要归功于其捕捉长距离依赖性的能力,同时保持线性复杂度与输入序列长度,使其成为CNN和ViT的有力替代方案。受成功的启发,本文探索了Mamba架构在解决DG中的分布偏移问题以进行医疗图像分割的潜力。具体来说,我们提出了一种基于Mamba的新型框架Mamba-Sea,它结合了全局到局部的序列增强,以提高模型在领域迁移问题下的泛化能力。我们的Mamba-Sea引入了一种全局增强机制,旨在模拟不同站点外观的潜在变化,旨在抑制模型对领域特定信息的学习。在局部层面,我们提出了沿输入序列的序列增强,通过建模和重新采样与领域迁移相关的风格统计,扰动随机连续子序列内标记的风格。据我们所知,Mamba-Sea是探索医疗图像分割中Mamba泛化的第一项工作,提供了一种先进且对领域迁移具有强大稳健性的Mamba架构。值得注意的是,我们提出的方法在前列腺数据集上的迪克系数首次超过90%,超过了之前的最佳纪录88.61%。代码可在[https://github.com/orange-czh/Mamba-Sea找到。]
论文及项目相关链接
PDF Accepted by IEEE TMI 2025. The code is available at https://github.com/orange-czh/Mamba-Sea
Summary
针对医学图像分割中的分布偏移问题,领域泛化(DG)成为训练能在未见目标领域泛化的模型的一种有前途的设置。受到Mamba等先进状态空间模型在监督医学图像分割中的成功的启发,本文探索了Mamba架构在解决医学图像分割中的领域泛化分布偏移问题的潜力。提出了基于Mamba的新框架Mamba-Sea,通过全球到局部的序列增强来提高模型在领域偏移问题下的泛化能力。Mamba-Sea首次探索了Mamba在医学图像分割中的泛化能力,提供了一种强大且对领域偏移具有鲁棒性的Mamba架构。
Key Takeaways
- 领域泛化是解决医学图像分割中分布偏移问题的有效方法。
- Mamba架构具有捕捉长距离依赖关系的能力,同时保持线性计算复杂度,使其成为CNN和ViT的有前途的替代方案。
- Mamba-Sea框架结合了全局到局部的序列增强,旨在提高模型在领域偏移问题下的泛化能力。
- Mamba-Sea首次将Mamba架构应用于医学图像分割的领域泛化,表现出强大的性能。
- Mamba-Sea通过在全局增强机制中模拟不同站点外观的潜在变化,抑制模型对领域特定信息的学习。
- 在局部层面,Mamba-Sea通过建模和重采样与领域偏移相关的风格统计,对输入序列进行序列增强。
点此查看论文截图
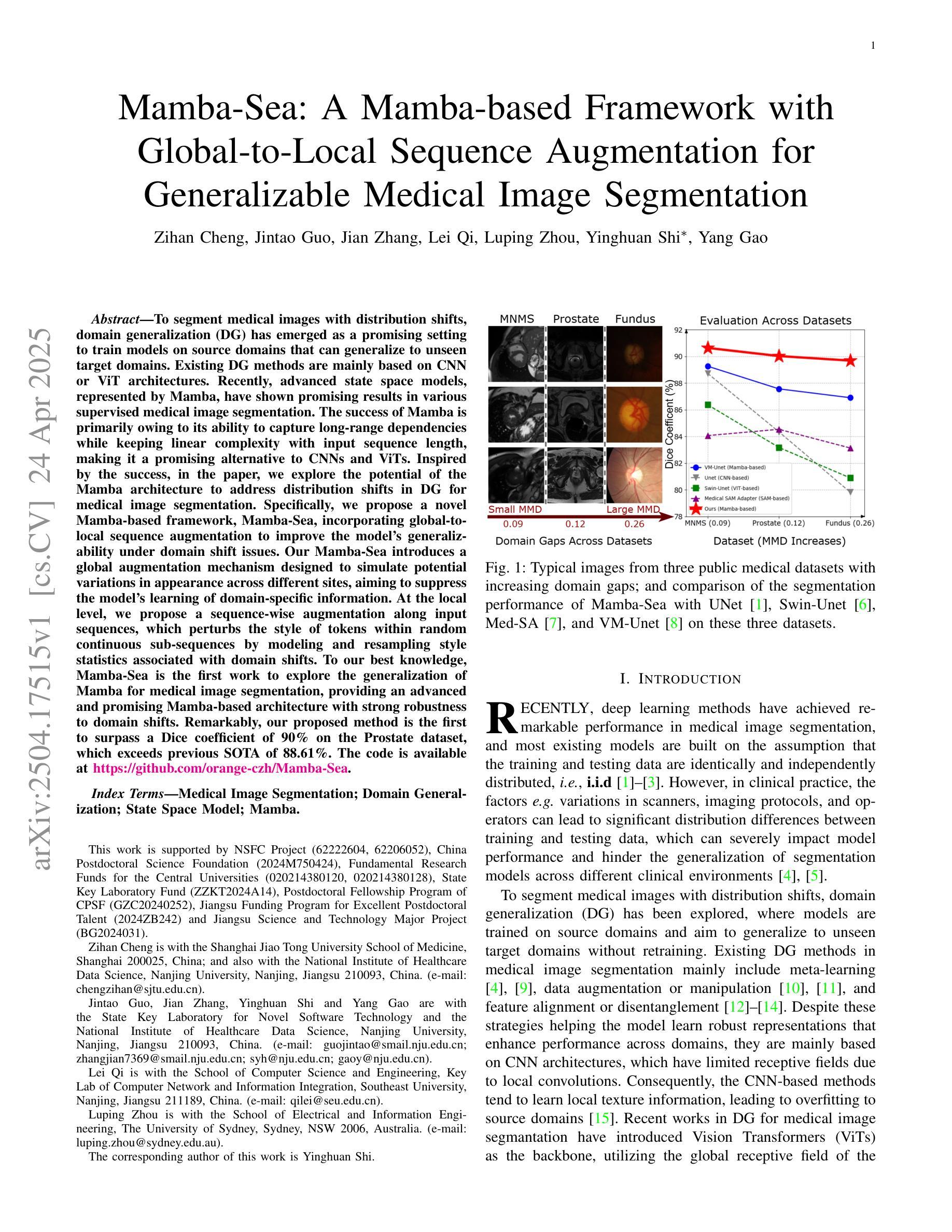
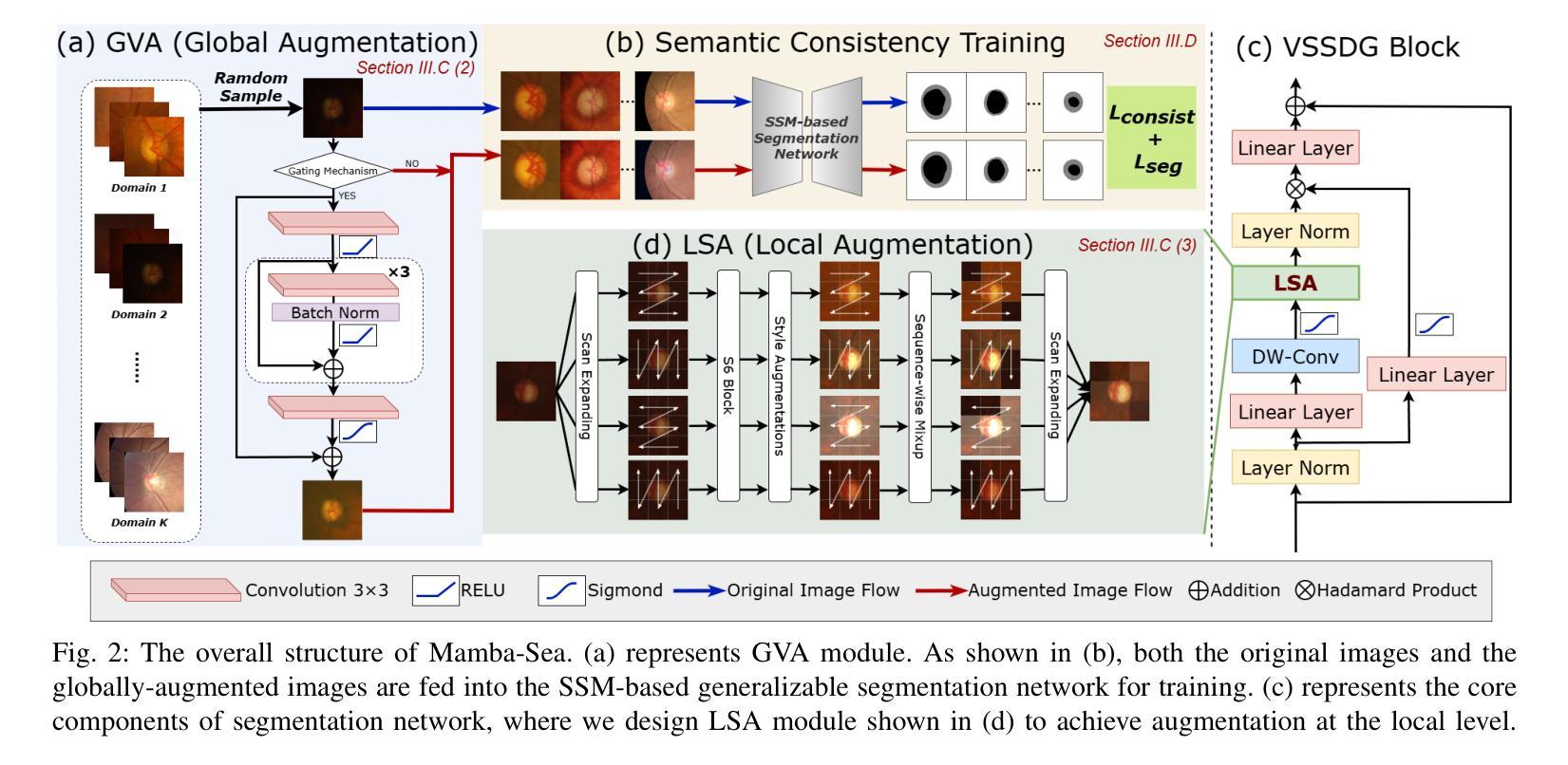
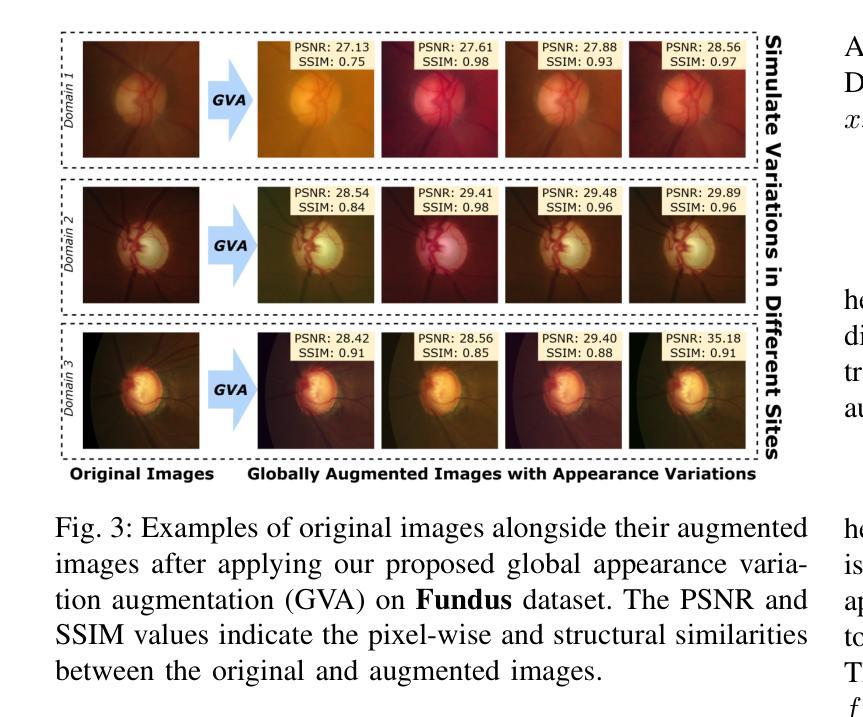
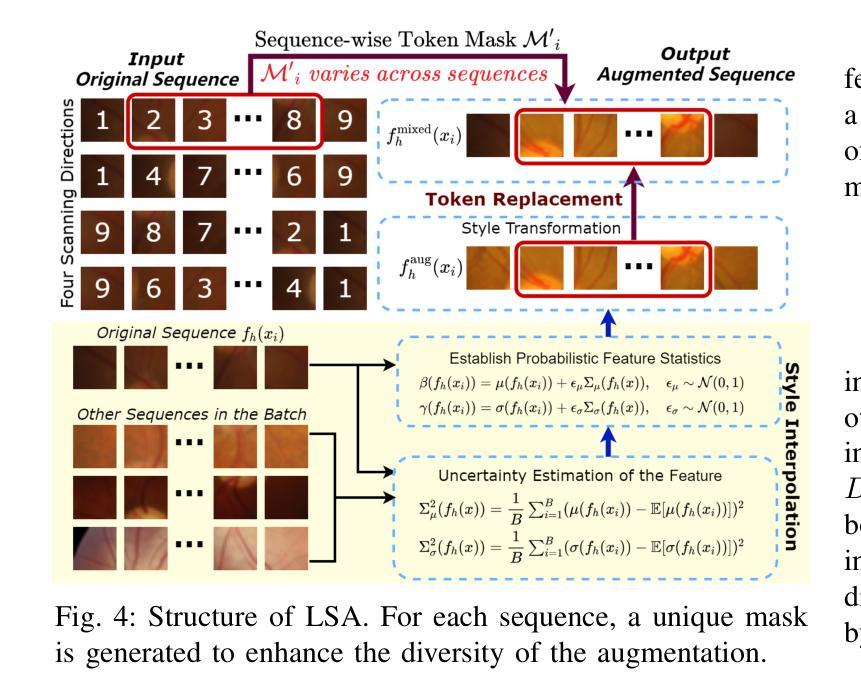