⚠️ 以下所有内容总结都来自于 大语言模型的能力,如有错误,仅供参考,谨慎使用
🔴 请注意:千万不要用于严肃的学术场景,只能用于论文阅读前的初筛!
💗 如果您觉得我们的项目对您有帮助 ChatPaperFree ,还请您给我们一些鼓励!⭐️ HuggingFace免费体验
2025-04-27 更新
ReaL: Efficient RLHF Training of Large Language Models with Parameter Reallocation
Authors:Zhiyu Mei, Wei Fu, Kaiwei Li, Guangju Wang, Huanchen Zhang, Yi Wu
Reinforcement Learning from Human Feedback (RLHF) is a pivotal technique for empowering large language model (LLM) applications. Compared with the supervised training process of LLMs, the RLHF training process is much more sophisticated, requiring a diverse range of computation workloads with intricate dependencies between multiple LLM instances. Therefore, simply adopting the fixed parallelization strategies from supervised training for LLMs can be insufficient for RLHF and result in low training efficiency. To overcome this limitation, we propose a novel technique named parameter ReaLlocation, which dynamically adapts the parallelization strategies for different workloads during training by redistributing LLM parameters across the training cluster. Building upon this idea, we introduce ReaL, a pioneering system for efficient RLHF training. ReaL introduces the concept of an execution plan, which defines a fine-grained resource allocation and parallelization strategy particularly designed for RLHF training. Based on this concept, ReaL employs a tailored search algorithm with a lightweight run-time estimator to automatically discover an efficient execution plan for an instance of RLHF experiment. Subsequently, the runtime engine deploys the selected plan by effectively parallelizing computations and redistributing parameters. We evaluate ReaL on the LLaMA models with up to 70 billion parameters and 128 GPUs. The experimental results demonstrate that ReaL achieves speedups of up to $3.58\times$ compared to baseline methods. Furthermore, the execution plans generated by ReaL exhibit an average of $81%$ performance improvement over heuristic approaches based on Megatron-LM in the long-context scenario. The source code of ReaL is publicly available at https://github.com/openpsi-project/ReaLHF .
强化学习从人类反馈(RLHF)是赋能大型语言模型(LLM)应用的关键技术。与LLM的监督训练过程相比,RLHF训练过程更为复杂,需要多种计算工作负载,并且在多个LLM实例之间存在复杂的相关性。因此,仅仅采用固定并行化策略进行LLM的监督训练对于RLHF来说是不足的,并可能导致训练效率低下。为了克服这一局限性,我们提出了一种名为参数重新分配的新型技术,它通过重新分配LLM参数来动态适应训练过程中的不同工作负载。基于这个想法,我们引入了ReaL系统,这是一个高效的RLHF训练系统。ReaL引入了执行计划的概念,该执行计划定义了针对RLHF训练特别设计的精细资源分配和并行化策略。基于此概念,ReaL采用定制的搜索算法和轻量级运行时估计器,以自动发现RLHF实验实例的有效执行计划。随后,运行时引擎通过有效地并行计算和重新分配参数来部署所选计划。我们在高达70亿参数和128个GPU的LLaMA模型上评估了ReaL的性能。实验结果表明,与基准方法相比,ReaL实现了高达3.58倍的速度提升。此外,ReaL生成的执行计划在长上下文场景中相对于基于Megatron-LM的启发式方法平均提高了8en%的性能。ReaL的源代码可在https://github.com/openpsi-project/ReaLHF公开获取。
论文及项目相关链接
PDF 11 pages (20 pages with references and the appendix), 17 figures. Accepted by MLSys 25
Summary
强化学习从人类反馈(RLHF)是赋能大型语言模型(LLM)应用的关键技术。相较于LLM的监督训练过程,RLHF的训练过程更为复杂,需要多样的计算工作量并且在多个LLM实例之间存在精细的依赖关系。为此,我们提出了参数重新分配(parameter ReaLlocation)这一新技术,它能够根据工作负载的不同动态调整并行化策略来提高训练效率。基于此思想,我们引入了ReaL系统,该系统通过执行计划实现精细粒度的资源分配和并行化策略,特别是为RLHF训练设计。ReaL能够自动发现高效的执行计划,并在运行时引擎中部署所选计划,有效提高计算并行性和参数重新分配。实验结果表明,ReaL相较于基础方法最高实现了$3.58\times$的加速效果。其源代码已公开在GitHub上。
Key Takeaways
- 强化学习从人类反馈(RLHF)在赋能大型语言模型(LLM)应用中扮演重要角色。
- RLHF训练过程相较于监督训练更为复杂,需要多样化的计算工作量以及精细的依赖管理。
- 参数重新分配技术能够动态调整并行化策略以适应不同的训练工作负载。
- ReaL系统通过执行计划实现精细粒度的资源分配和专为RLHF设计的并行化策略。
- ReaL能够自动发现高效的执行计划以提高计算并行性和参数分配效率。
- 实验结果表明,ReaL相较于基础方法有明显的加速效果。
点此查看论文截图
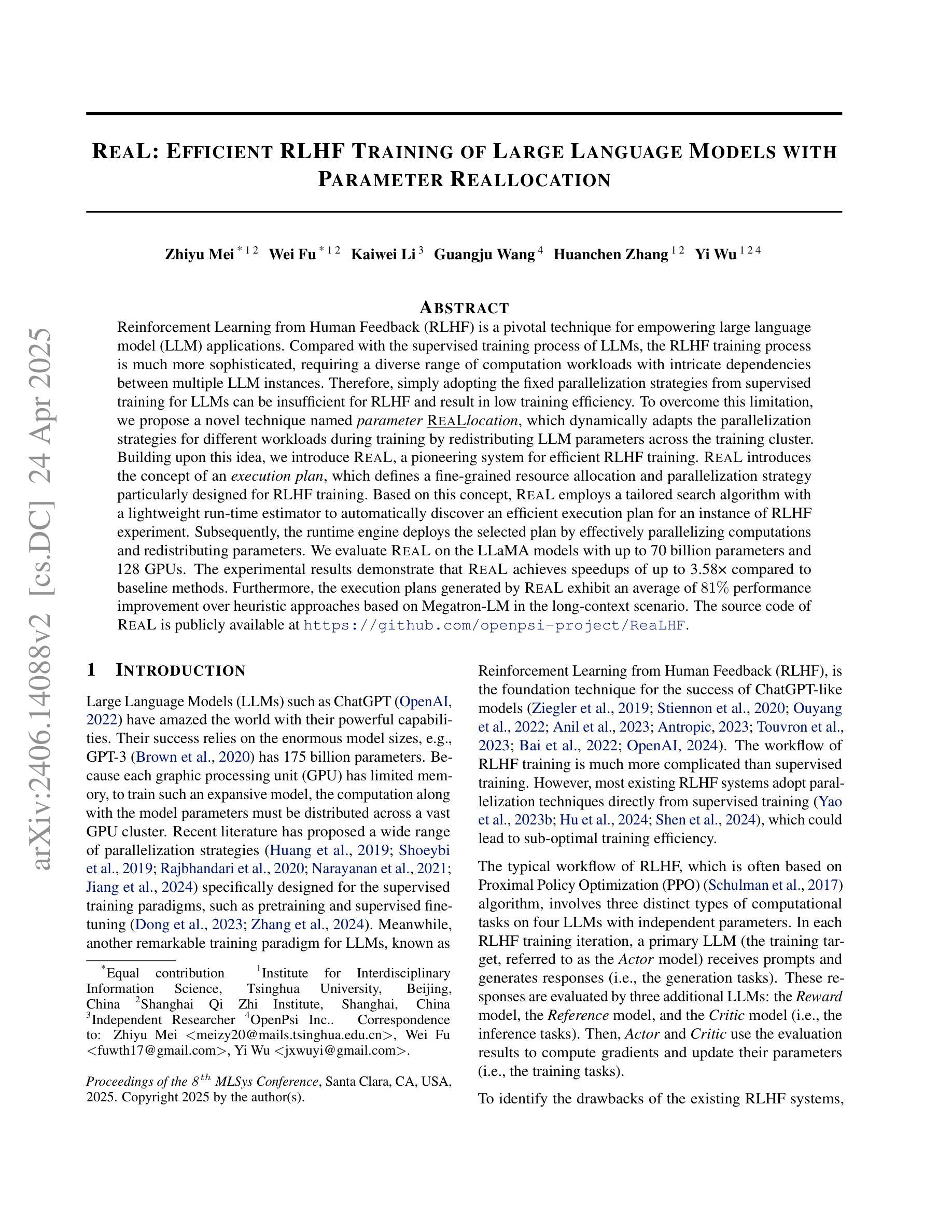
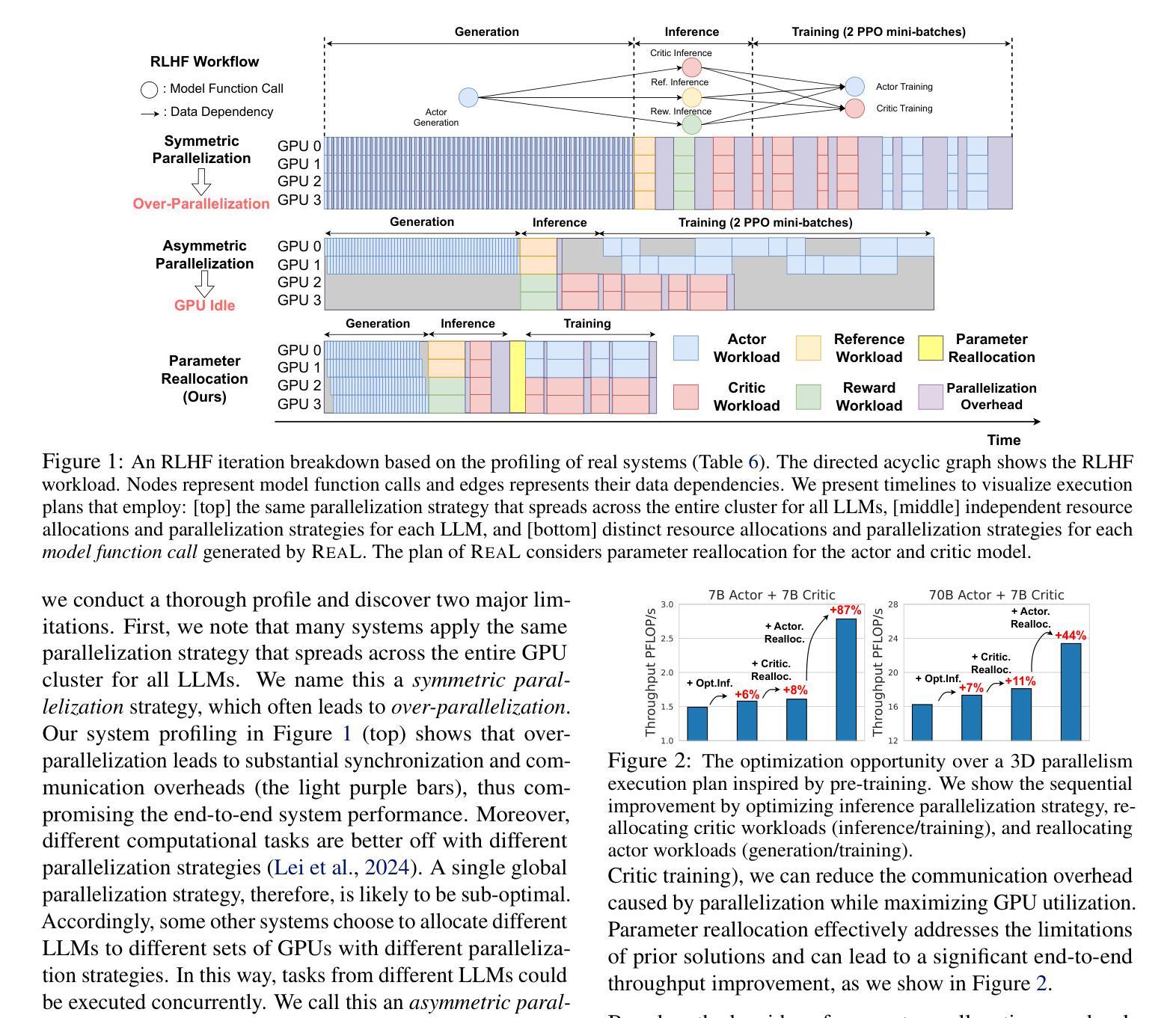
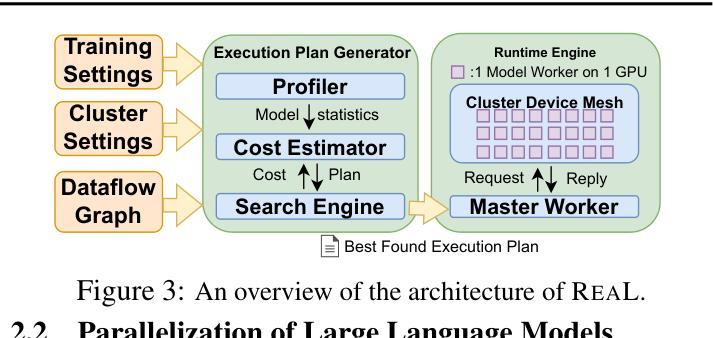
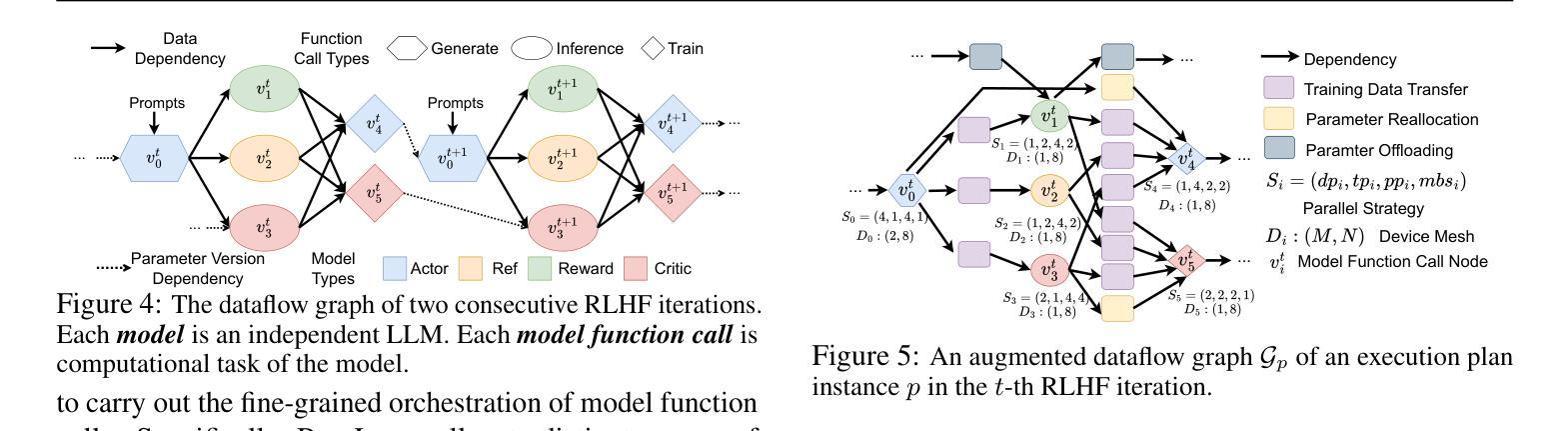
Unlocking Large Language Model’s Planning Capabilities with Maximum Diversity Fine-tuning
Authors:Wenjun Li, Changyu Chen, Pradeep Varakantham
Large language models (LLMs) have demonstrated impressive task-solving capabilities through prompting techniques and system designs, including solving planning tasks (e.g., math proofs, basic travel planning) when sufficient data is available online and used during pre-training. However, for planning tasks with limited prior data (e.g., blocks world, advanced travel planning), the performance of LLMs, including proprietary models like GPT and Gemini, is poor. This paper investigates the impact of fine-tuning on the planning capabilities of LLMs, revealing that LLMs can achieve strong performance in planning through substantial (tens of thousands of specific examples) fine-tuning. Yet, this process incurs high economic, time, and computational costs for each planning problem variation. To address this, we propose Clustering-Based Maximum Diversity Sampling (CMDS), which selects diverse and representative data to enhance sample efficiency and the model’s generalization capability. Extensive evaluations demonstrate that CMDS-l, a baseline method combining CMDS with language embeddings, outperforms random sampling. Furthermore, we introduce a novel algorithm, CMDS-g, which encodes planning task instances with their graph representations into the embedding space. Empirical results show that CMDS-g consistently outperforms baseline methods across various scales and multiple benchmark domains.
大型语言模型(LLM)通过提示技术和系统设计,展示了令人印象深刻的任务解决能力,包括在在线数据充足并用于预训练时,解决规划任务(例如数学证明、基本旅行规划)。然而,对于具有有限先验数据的规划任务(例如积木世界、高级旅行规划),包括GPT和双子座等专有模型在内的LLM表现不佳。本文研究了微调对LLM规划能力的影响,发现LLM可以通过大量的(数以万计的具体例子)微调实现强大的规划能力。然而,这个过程为每个规划问题变体带来了高昂的经济、时间和计算成本。为解决这一问题,我们提出了基于聚类的最大多样性采样(CMDS),该方法选择多样且具有代表性的数据来提高样本效率和模型的泛化能力。广泛的评估表明,CMDS-l(一种将CMDS与语言嵌入相结合的基线方法)优于随机抽样。此外,我们引入了一种新型算法CMDS-g,它将规划任务实例与其图形表示编码到嵌入空间中。实证结果表明,CMDS-g在各种规模和多个基准领域上始终优于基线方法。
论文及项目相关链接
PDF 8 pages of main paper, 2 pages of references
Summary
大型语言模型(LLM)在足够的在线数据和预训练支持的情况下,能展现解决规划任务(如数学证明、基本旅行规划)的能力。然而,对于缺乏先验数据的规划任务,LLM的表现较差。本文通过微调研究LLM的规划能力,发现通过大量的特定例子微调,LLM可以在规划任务中取得良好的表现。为解决经济、时间和计算成本高昂的问题,本文提出了基于聚类的最大多样性采样(CMDS)方法,该方法能选择多样且具有代表性的数据,提高样本效率和模型的泛化能力。评估结果显示,CMDS与语言嵌入结合的基线方法CMDS-l优于随机采样方法,而结合规划任务实例的图表示进入嵌入空间的CMDS-g新算法则在不同规模和多个基准领域上始终优于基线方法。
Key Takeaways
- LLM具备解决规划任务的能力,但在缺乏先验数据的情况下表现不佳。
- 通过大量的特定例子微调,LLM可以在规划任务中取得良好的表现。
- 提出了基于聚类的最大多样性采样(CMDS)方法以提高样本效率和模型泛化能力。
- CMDS-l作为结合CMDS与语言嵌入的基线方法,表现优于随机采样。
- CMDS-g新算法结合规划任务实例的图表示进入嵌入空间,表现优异。
- CMDS-g在不同规模和多个基准领域上的表现均优于基线方法。
点此查看论文截图
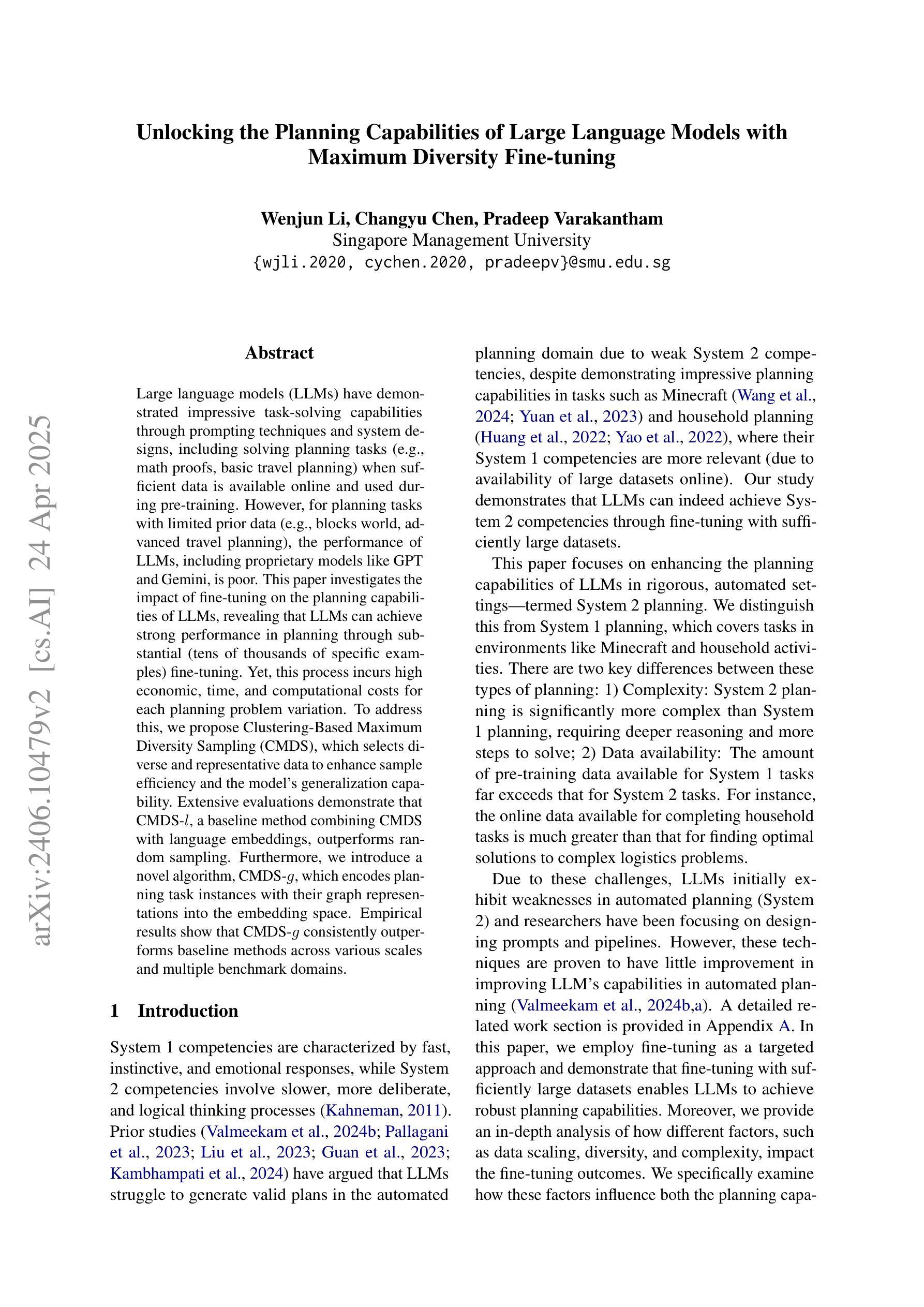
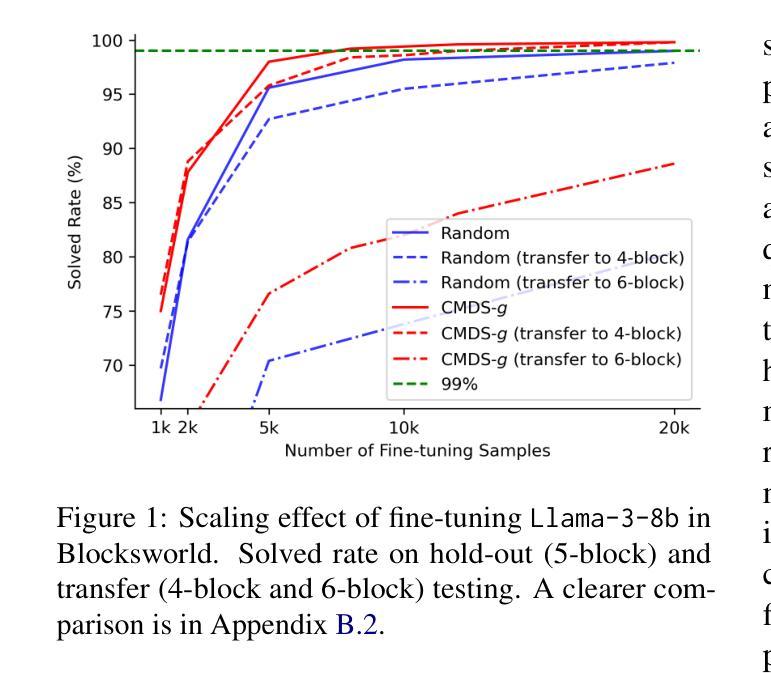
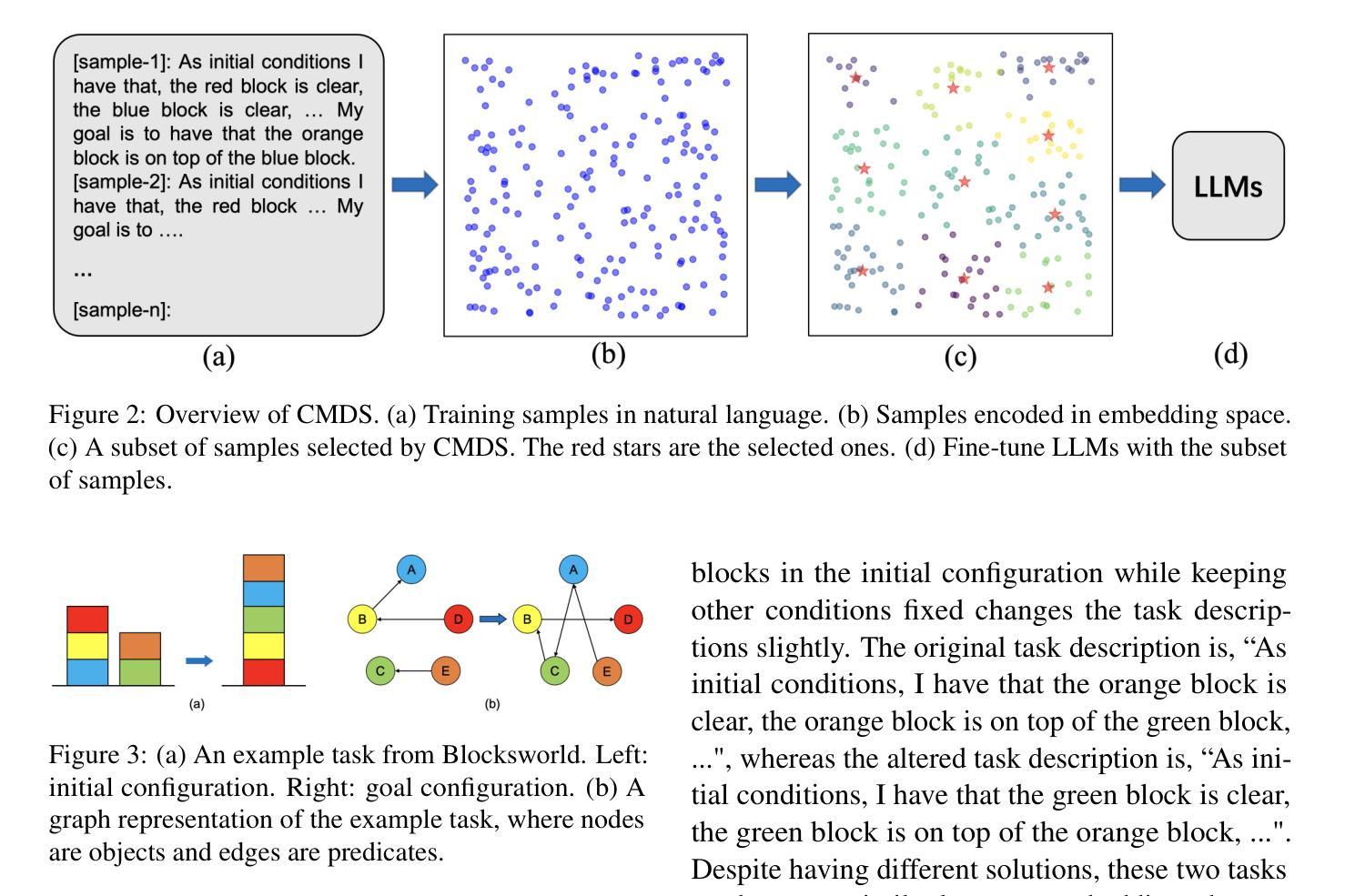
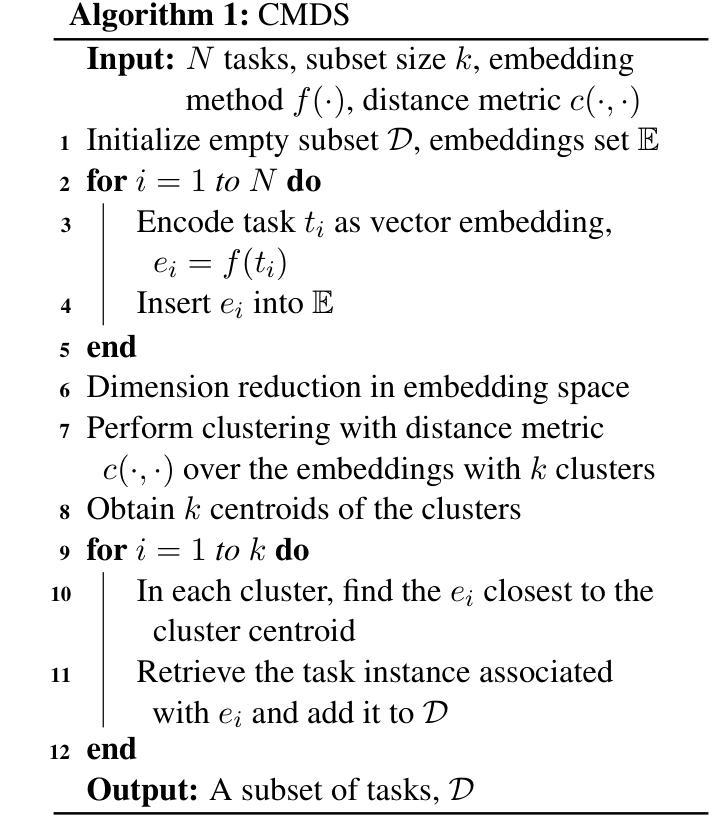
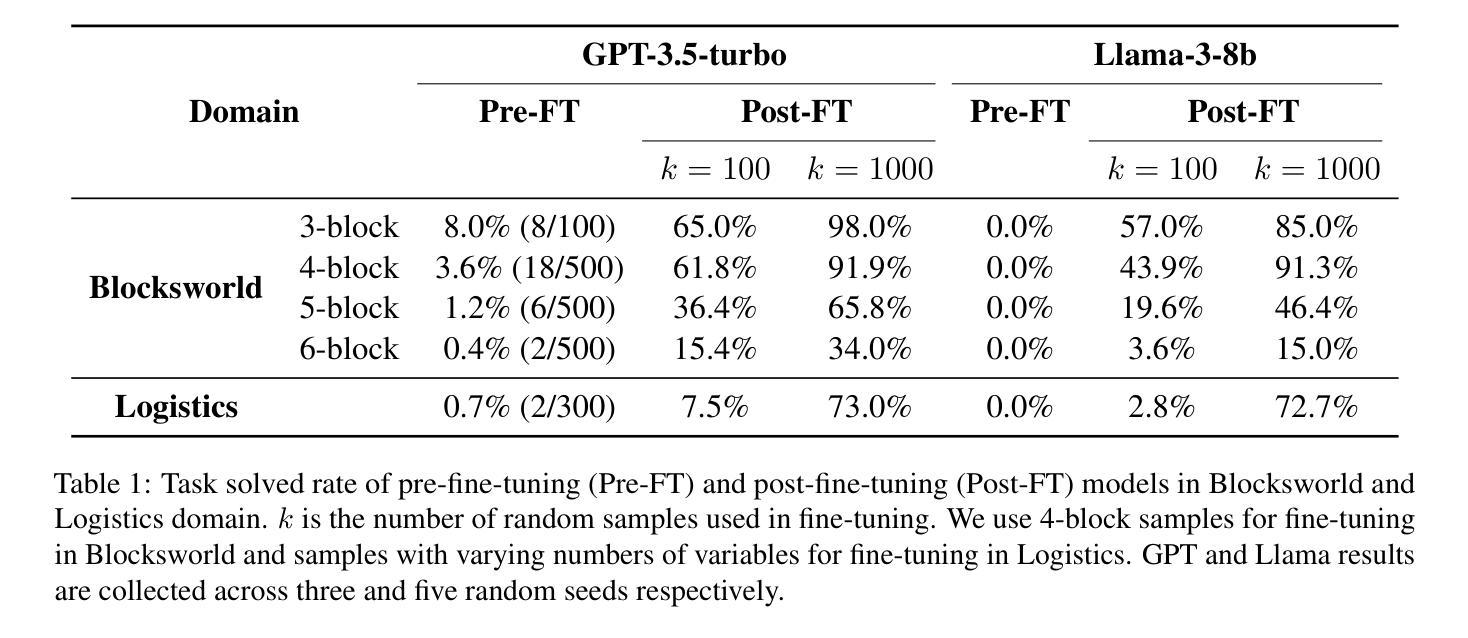
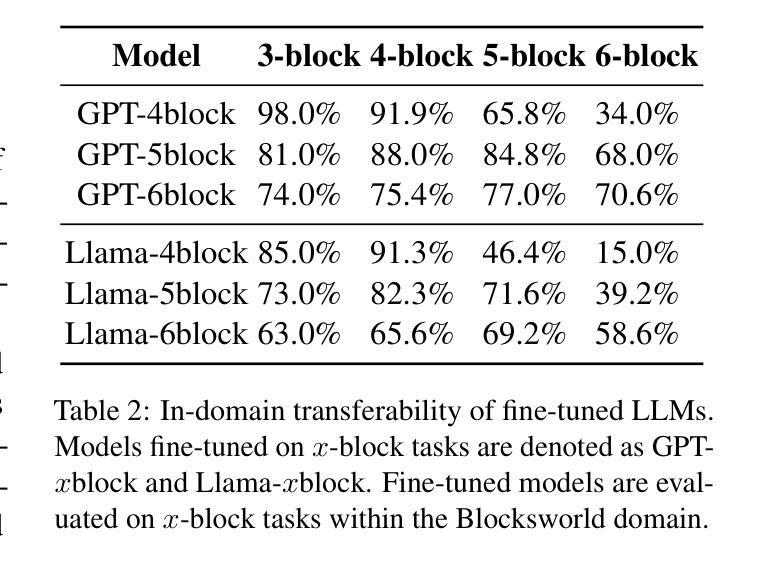
TextSquare: Scaling up Text-Centric Visual Instruction Tuning
Authors:Jingqun Tang, Chunhui Lin, Zhen Zhao, Shu Wei, Binghong Wu, Qi Liu, Hao Feng, Yang Li, Siqi Wang, Lei Liao, Wei Shi, Yuliang Liu, Hao Liu, Yuan Xie, Xiang Bai, Can Huang
Text-centric visual question answering (VQA) has made great strides with the development of Multimodal Large Language Models (MLLMs), yet open-source models still fall short of leading models like GPT4V and Gemini, partly due to a lack of extensive, high-quality instruction tuning data. To this end, we introduce a new approach for creating a massive, high-quality instruction-tuning dataset, Square-10M, which is generated using closed-source MLLMs. The data construction process, termed Square, consists of four steps: Self-Questioning, Answering, Reasoning, and Evaluation. Our experiments with Square-10M led to three key findings: 1) Our model, TextSquare, considerably surpasses open-source previous state-of-the-art Text-centric MLLMs and sets a new standard on OCRBench(62.2%). It even outperforms top-tier models like GPT4V and Gemini in 6 of 10 text-centric benchmarks. 2) Additionally, we demonstrate the critical role of VQA reasoning data in offering comprehensive contextual insights for specific questions. This not only improves accuracy but also significantly mitigates hallucinations. Specifically, TextSquare scores an average of 75.1% across four general VQA and hallucination evaluation datasets, outperforming previous state-of-the-art models. 3) Notably, the phenomenon observed in scaling text-centric VQA datasets reveals a vivid pattern: the exponential increase of instruction tuning data volume is directly proportional to the improvement in model performance, thereby validating the necessity of the dataset scale and the high quality of Square-10M.
文本聚焦的视觉问答(VQA)随着多模态大型语言模型(MLLMs)的发展而取得了巨大进步。然而,开源模型仍然无法赶超如GPT4V和Gemini等领先模型,部分原因在于缺乏广泛的高质量指令调整数据。为此,我们介绍了一种创建大规模高质量指令调整数据集Square-10M的新方法,该数据集是利用封闭源代码的MLLMs生成的。数据构建过程称为Square,分为四个步骤:自我提问、回答、推理和评价。我们利用Square-10M进行的实验得到了三个关键发现:
- 我们的模型TextSquare显著超越了先前的开源顶尖文本聚焦型MLLMs,并在OCRBench上设定了新标准(62.2%)。它甚至在10个文本聚焦的基准测试中胜出其中六个与GPT4V和Gemini等顶尖模型一较高下。
- 此外,我们证明了VQA推理数据在提供特定问题的全面背景信息方面的关键作用。这不仅提高了准确性,而且有效减轻了幻觉现象。具体来说,TextSquare在四个通用VQA和幻觉评估数据集上的平均得分为75.1%,超过了之前的顶尖模型。
- 值得注意的是,在扩展文本聚焦的VQA数据集时观察到的现象呈现出了一种鲜明模式:指令调整数据量的指数增长与模型性能的提高直接相关,从而验证了数据集规模及其高质量的必要性和Square-10M的高品质。
论文及项目相关链接
摘要
利用闭源多模态大型语言模型(MLLMs)创建大规模高质量指令调整数据集Square-10M,通过自我提问、回答、推理和评价四步构建数据。实验表明,TextSquare模型在OCRBench上超越开放源代码的文本中心型MLLMs并设定新标准,并在多个文本中心型基准测试中表现优异。此外,VQA推理数据对于提供特定问题的全面背景信息至关重要,可提高准确性并显著降低幻想结果的出现。最后,数据集规模的扩大与模型性能的提升呈正比,验证了Square-10M数据集规模和高质量的重要性。
关键见解
- 引入新型指令调整数据集Square-10M,利用闭源多模态大型语言模型(MLLMs)创建,包含自我提问、回答、推理和评价四步构建。
- TextSquare模型在OCRBench上的表现超越现有开源的文本中心型MLLMs,并在多个基准测试中表现优异。
- VQA推理数据对于提供全面背景信息至关重要,能提高模型准确性并降低幻想结果的出现。
- Square-10M数据集的规模对于模型性能的提升至关重要,数据量的增加与模型性能提升呈正比。
点此查看论文截图
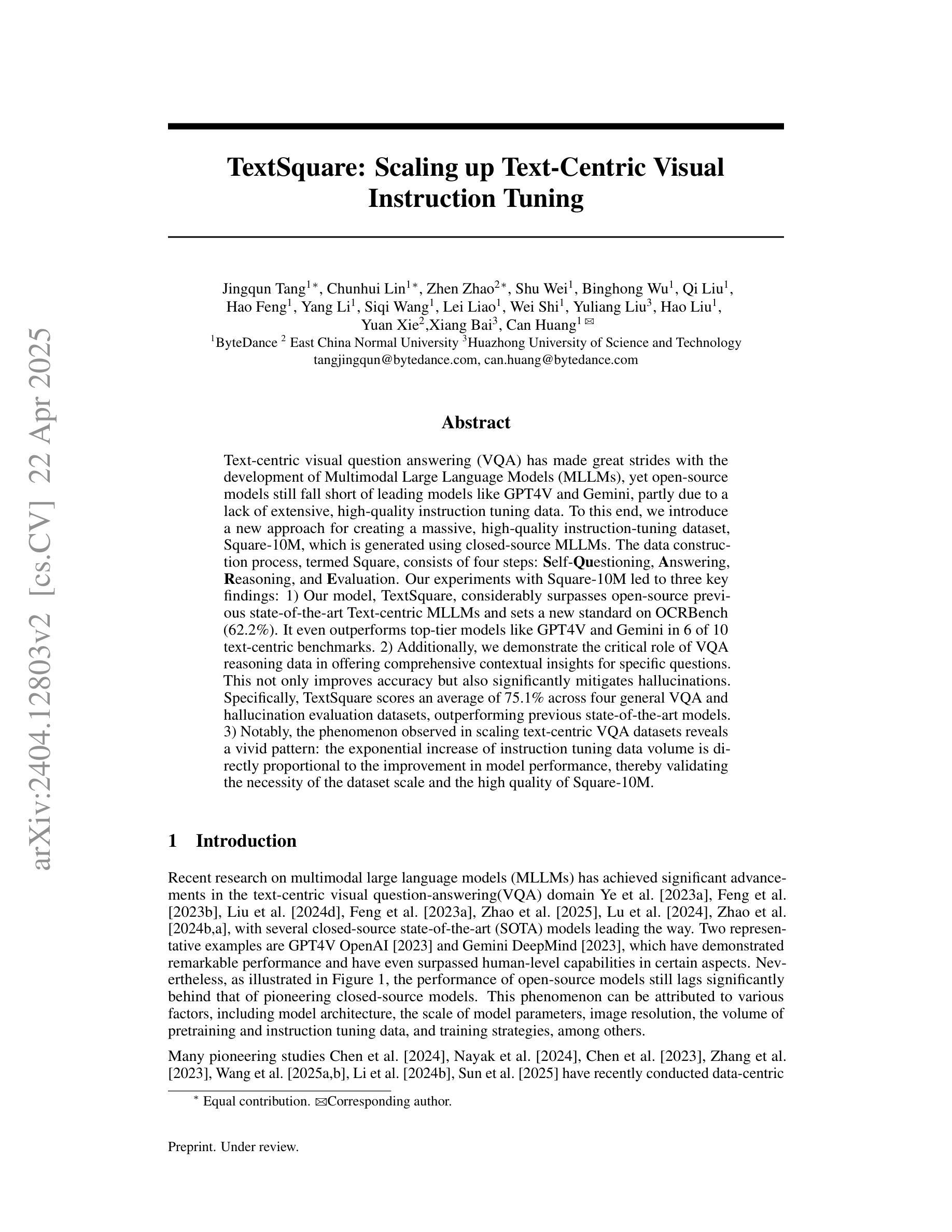
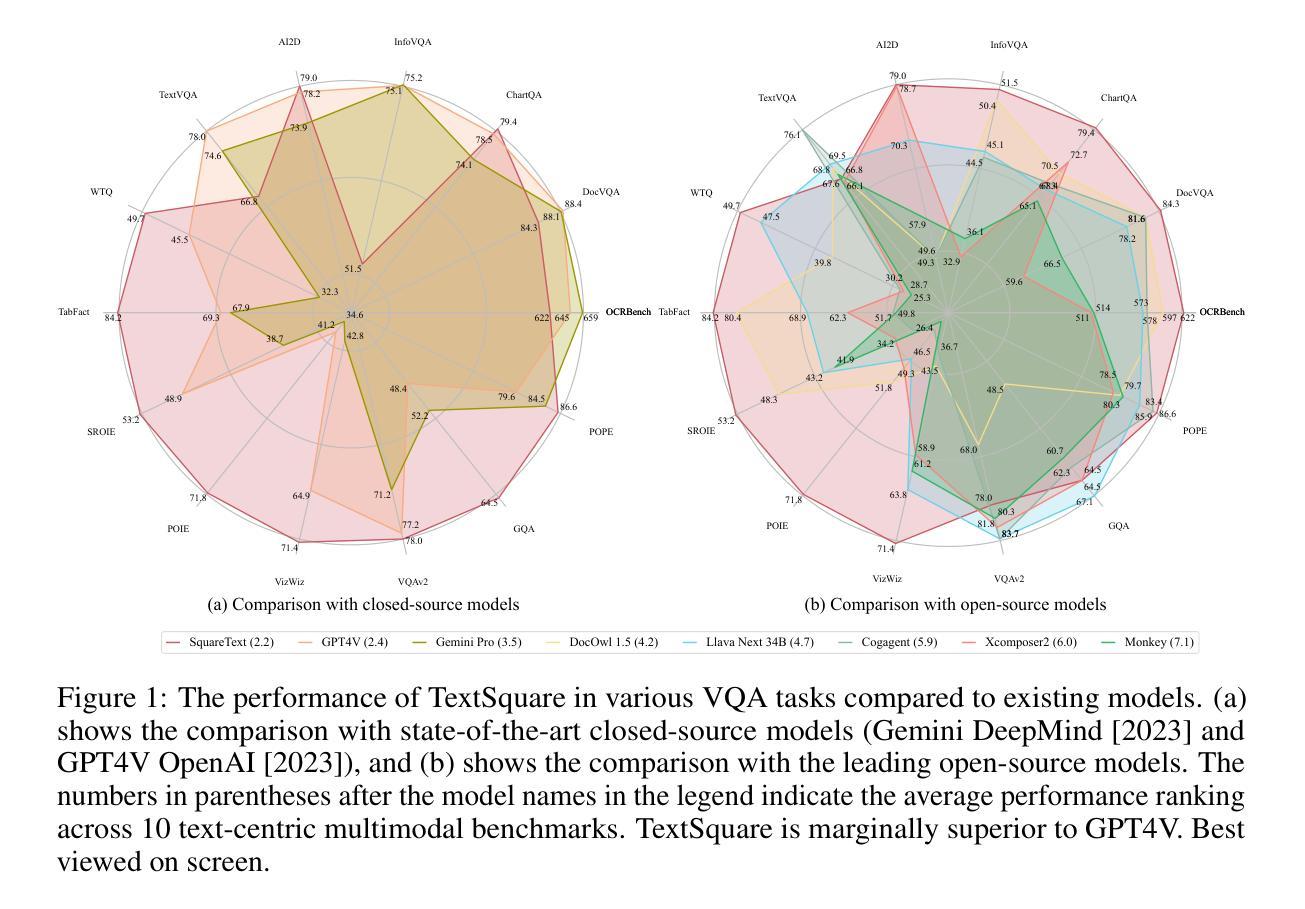
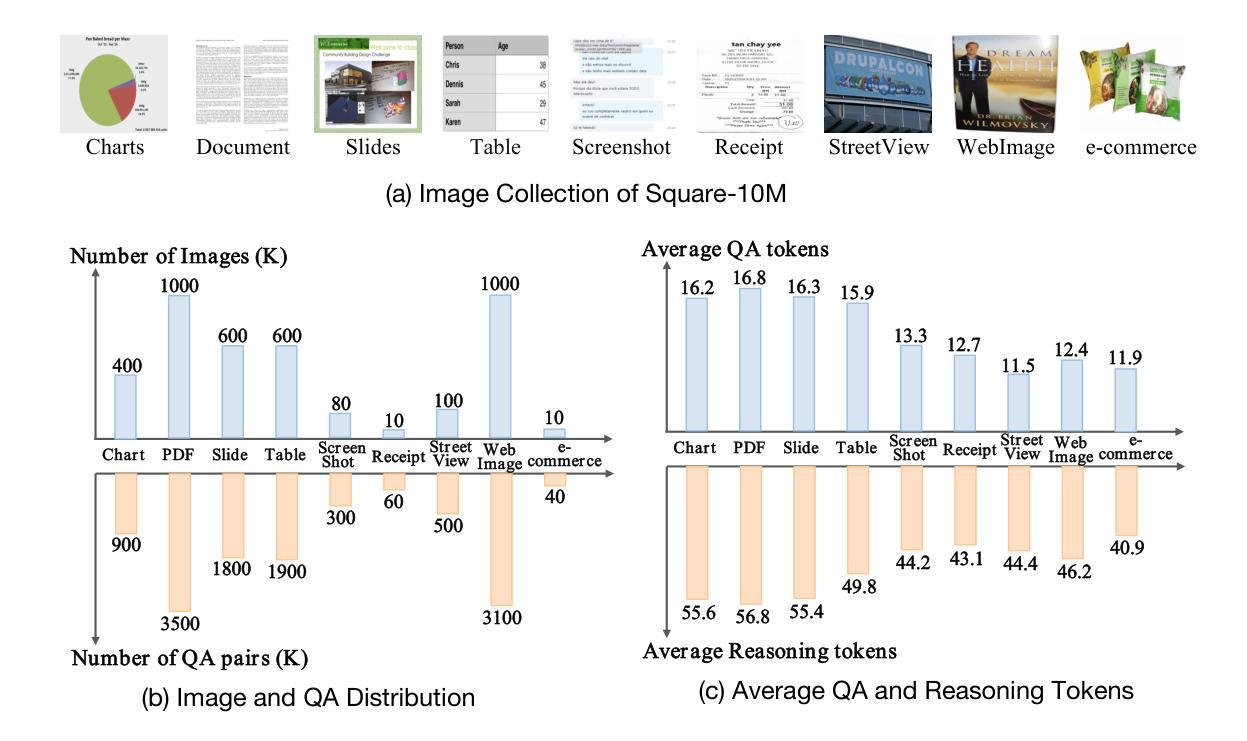
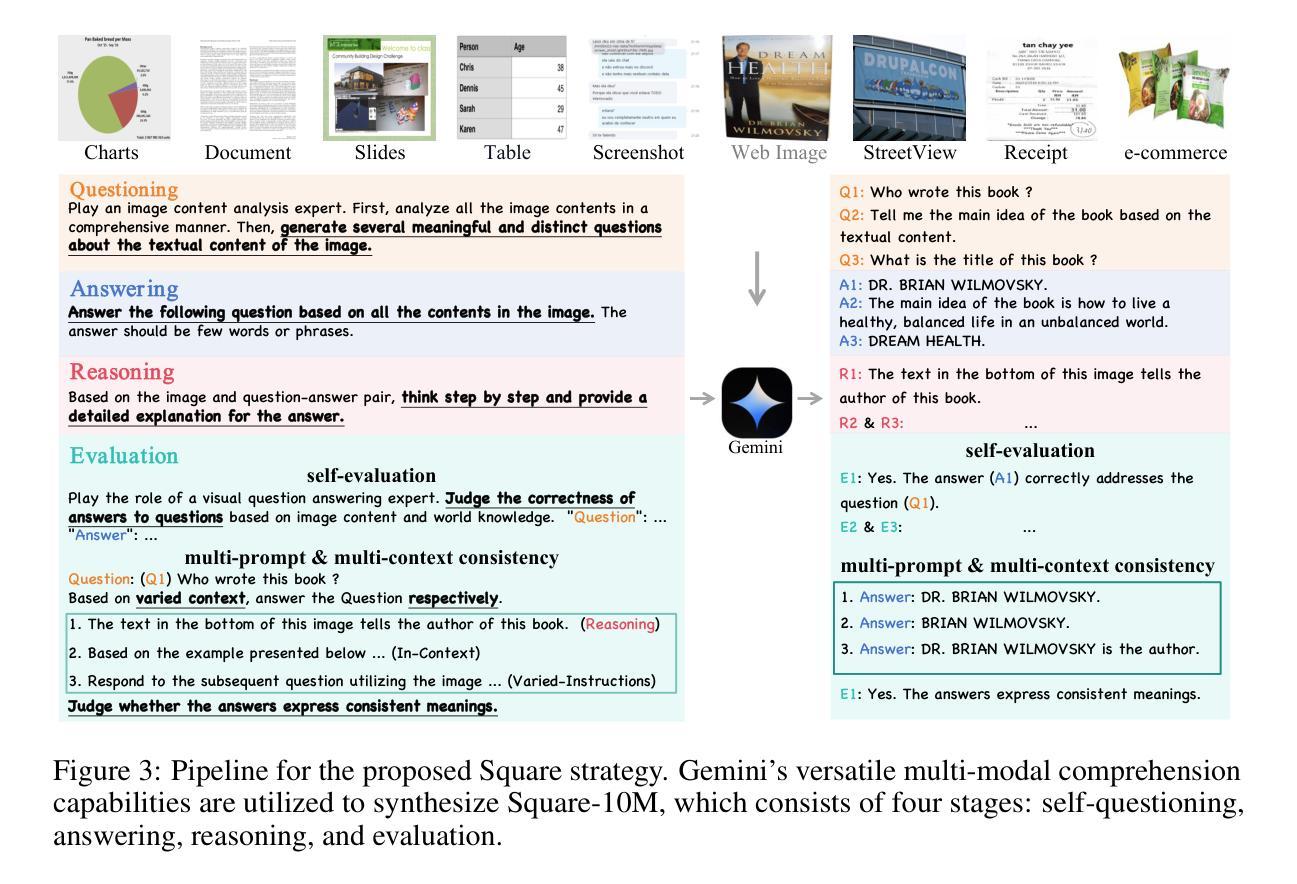
Potential Societal Biases of ChatGPT in Higher Education: A Scoping Review
Authors:Ming Li, Ariunaa Enkhtur, Beverley Anne Yamamoto, Fei Cheng, Lilan Chen
Purpose:Generative Artificial Intelligence (GAI) models, such as ChatGPT, may inherit or amplify societal biases due to their training on extensive datasets. With the increasing usage of GAI by students, faculty, and staff in higher education institutions (HEIs), it is urgent to examine the ethical issues and potential biases associated with these technologies. Design/Approach/Methods:This scoping review aims to elucidate how biases related to GAI in HEIs have been researched and discussed in recent academic publications. We categorized the potential societal biases that GAI might cause in the field of higher education. Our review includes articles written in English, Chinese, and Japanese across four main databases, focusing on GAI usage in higher education and bias. Findings:Our findings reveal that while there is meaningful scholarly discussion around bias and discrimination concerning LLMs in the AI field, most articles addressing higher education approach the issue superficially. Few articles identify specific types of bias under different circumstances, and there is a notable lack of empirical research. Most papers in our review focus primarily on educational and research fields related to medicine and engineering, with some addressing English education. However, there is almost no discussion regarding the humanities and social sciences. Additionally, a significant portion of the current discourse is in English and primarily addresses English-speaking contexts. Originality/Value:To the best of our knowledge, our study is the first to summarize the potential societal biases in higher education. This review highlights the need for more in-depth studies and empirical work to understand the specific biases that GAI might introduce or amplify in educational settings, guiding the development of more ethical AI applications in higher education.
目的:生成式人工智能(GAI)模型,如ChatGPT,由于其基于大量数据集进行训练,可能会继承或放大社会偏见。随着高等教育机构(HEIs)的学生、教师和员工对GAI的越来越广泛的使用,亟需审查与这些技术相关的伦理问题和潜在偏见。设计/方法:本综述旨在阐明近年来在学术出版物中如何研究和讨论高等教育机构中GAI的偏见问题。我们对GAI在高等教育领域可能产生的潜在社会偏见进行了分类。我们的综述包括四个主要数据库的英文、中文和日语文章,重点关注人工智能领域的大型语言模型(LLM)在高等教育中的使用情况和偏见问题。发现:我们的研究发现,虽然人工智能领域的偏见和歧视问题已有相当多的学术讨论,但大多数针对高等教育的文章都仅限于表面。很少有文章能识别出不同情况下的特定偏见类型,并且缺乏实证研究的明显。我们评审的论文主要集中在医学和工程相关的教育和研究领域,其中一些涉及英语教育。然而,关于人文和社会科学的讨论几乎不存在。此外,当前的大部分讨论都是英语,主要关注英语语境。创新性/价值:据我们所知,我们的研究是首次总结高等教育中潜在的社会偏见。本综述强调了需要更深入的研究和实证工作,以了解GAI在教育环境中可能引入或放大的特定偏见,为高等教育中更伦理的人工智能应用发展提供指导。
论文及项目相关链接
PDF Open Praxis
Summary
在高等教育机构中,生成式人工智能模型(如ChatGPT)可能因训练时使用的庞大数据集而继承或放大社会偏见。随着学生在高等教育机构中对这些技术的使用不断增加,对与之相关的伦理问题和潜在偏见的研究变得迫切。本文通过文献综述方式探讨了高等教育中的人工智能模型的潜在社会偏见问题,发现目前研究多关注医学和工程领域,缺乏对人文学科和社会科学的深入探讨,同时大多数文献是以英语撰写,主要关注英语语境下的讨论。呼吁未来研究需要更深入的了解人工智能模型在教育中引入或放大的具体偏见,以指导更伦理的人工智能应用在高等教育领域的发展。
Key Takeaways
- 生成式人工智能模型(如ChatGPT)在高等教育中的使用日益普及,引发对伦理问题和潜在社会偏见的关注。
- 这些模型可能因训练数据而继承或放大社会偏见。
- 目前的研究主要集中在医学和工程领域的人工智能在教育中的应用,对人文学科和社会科学的关注较少。
- 大多数文献是以英语撰写,主要关注英语语境下的讨论,对其他语言和背景的研究相对缺乏。
- 目前对于人工智能在高等教育中的潜在社会偏见的研究缺乏深入和实证性研究。
- 需要更多的研究来了解人工智能模型在教育中引入或放大的具体偏见。
点此查看论文截图
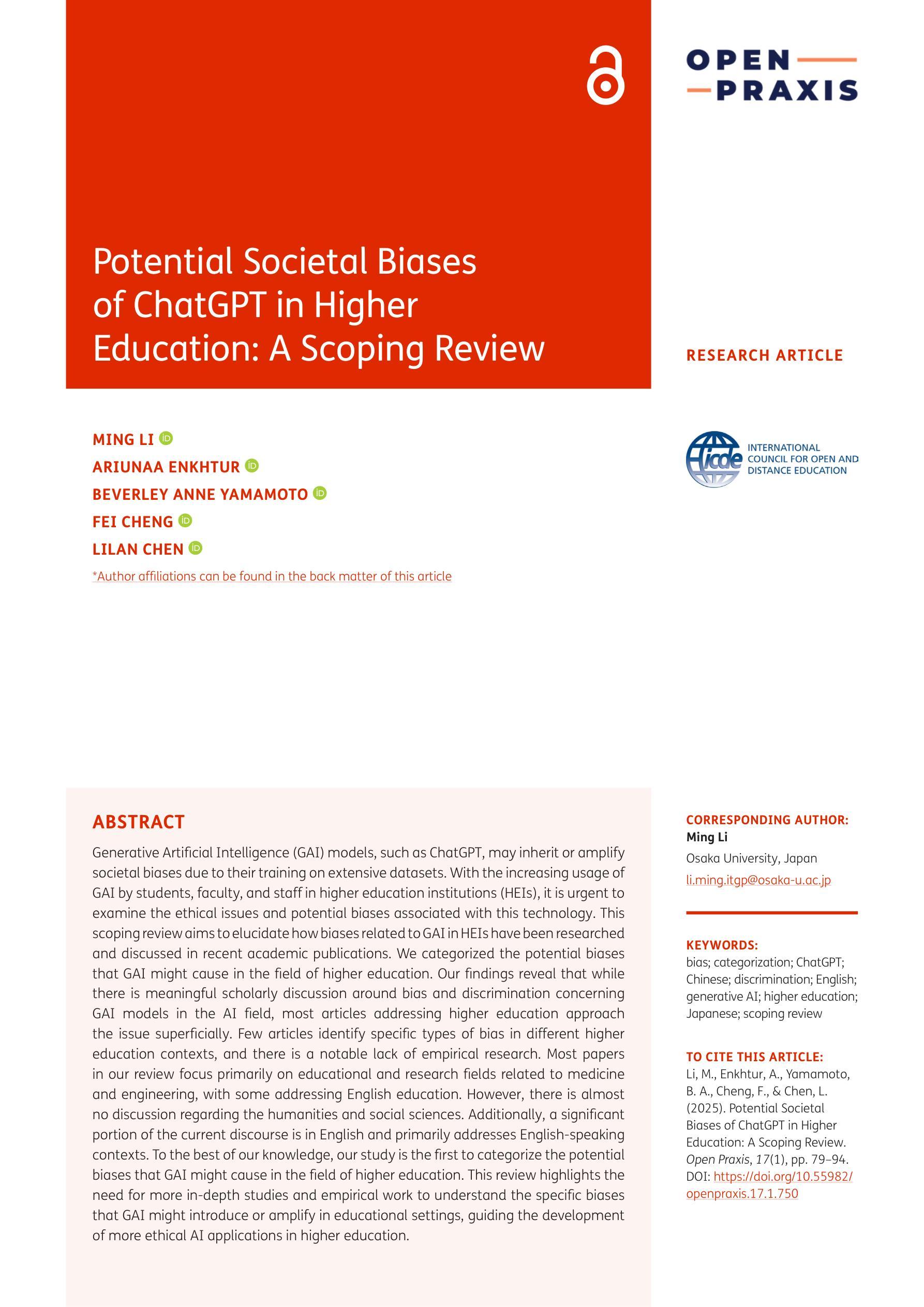
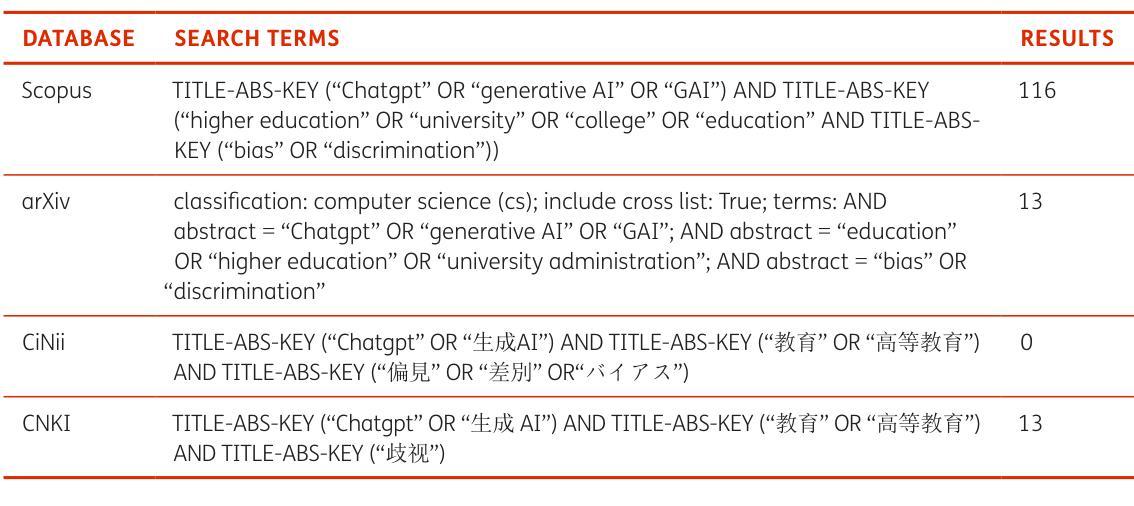
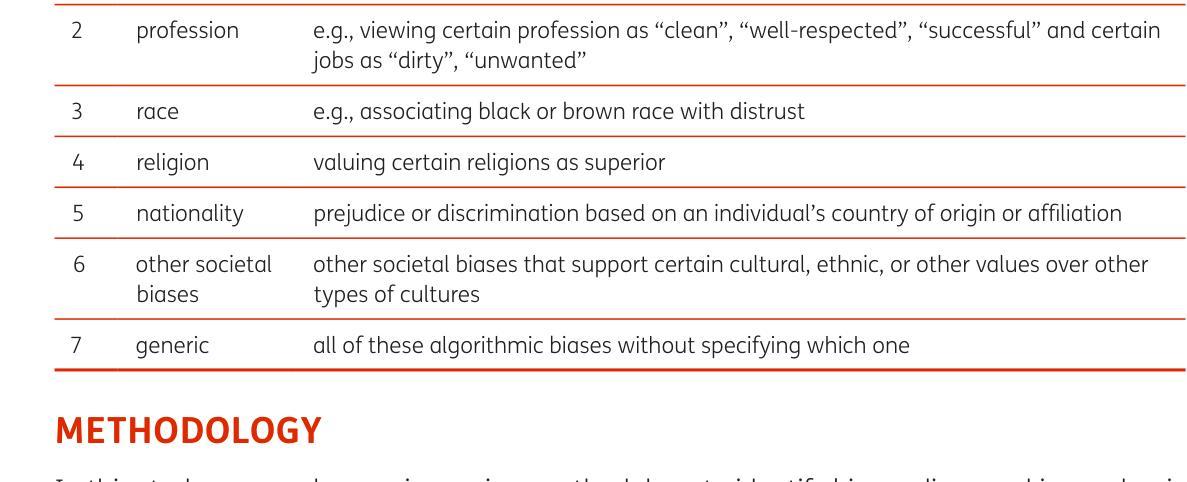
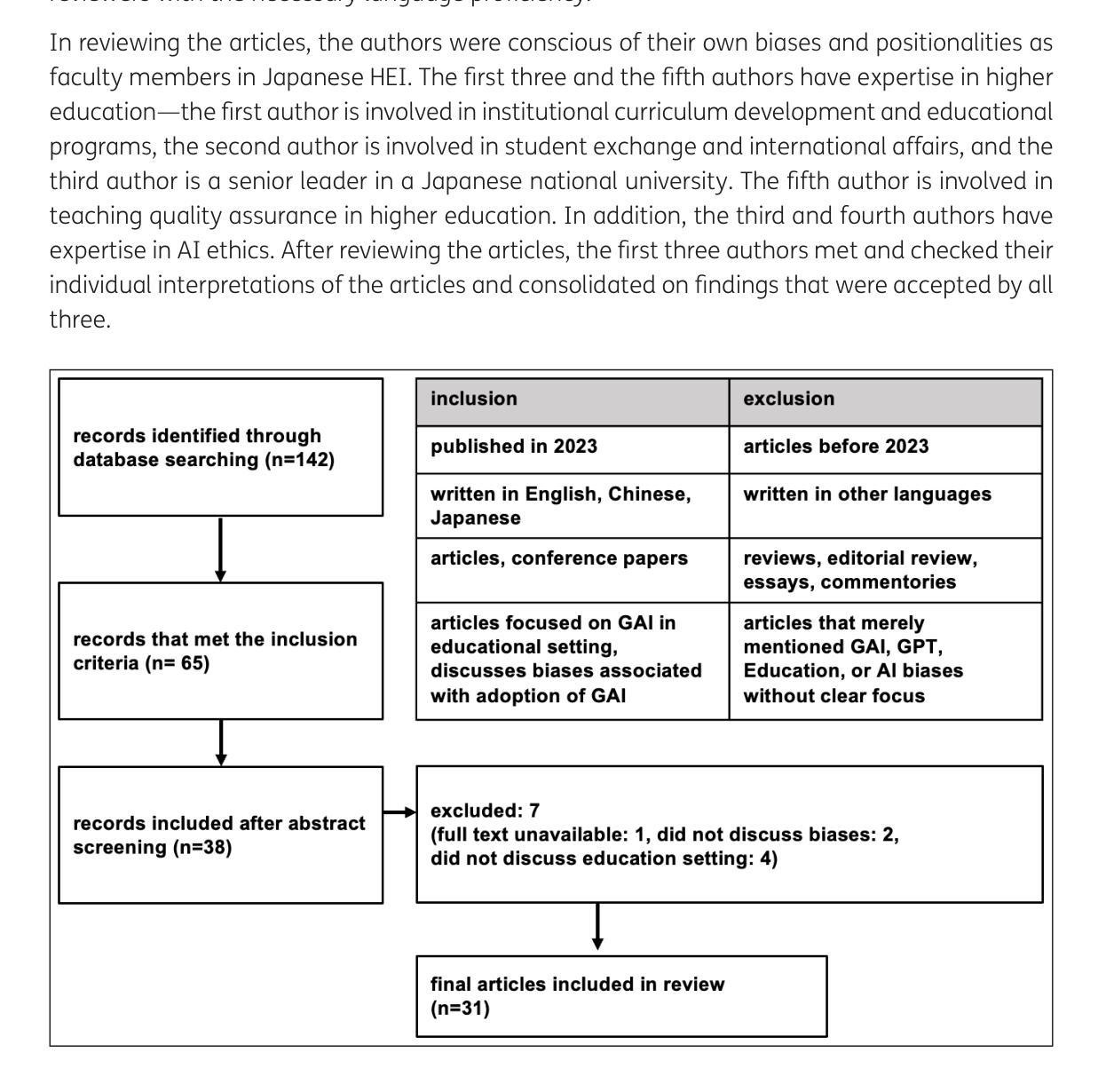