⚠️ 以下所有内容总结都来自于 大语言模型的能力,如有错误,仅供参考,谨慎使用
🔴 请注意:千万不要用于严肃的学术场景,只能用于论文阅读前的初筛!
💗 如果您觉得我们的项目对您有帮助 ChatPaperFree ,还请您给我们一些鼓励!⭐️ HuggingFace免费体验
2025-04-29 更新
SSD-Poser: Avatar Pose Estimation with State Space Duality from Sparse Observations
Authors:Shuting Zhao, Linxin Bai, Liangjing Shao, Ye Zhang, Xinrong Chen
The growing applications of AR/VR increase the demand for real-time full-body pose estimation from Head-Mounted Displays (HMDs). Although HMDs provide joint signals from the head and hands, reconstructing a full-body pose remains challenging due to the unconstrained lower body. Recent advancements often rely on conventional neural networks and generative models to improve performance in this task, such as Transformers and diffusion models. However, these approaches struggle to strike a balance between achieving precise pose reconstruction and maintaining fast inference speed. To overcome these challenges, a lightweight and efficient model, SSD-Poser, is designed for robust full-body motion estimation from sparse observations. SSD-Poser incorporates a well-designed hybrid encoder, State Space Attention Encoders, to adapt the state space duality to complex motion poses and enable real-time realistic pose reconstruction. Moreover, a Frequency-Aware Decoder is introduced to mitigate jitter caused by variable-frequency motion signals, remarkably enhancing the motion smoothness. Comprehensive experiments on the AMASS dataset demonstrate that SSD-Poser achieves exceptional accuracy and computational efficiency, showing outstanding inference efficiency compared to state-of-the-art methods.
随着AR/VR应用的不断增长,对头戴式显示器(HMDs)实时全身姿态估计的需求也在增加。虽然HMDs提供了头部和手部关节信号,但由于下半身不受约束,重建全身姿态仍然具有挑战性。最近的进展通常依赖于传统神经网络和生成模型来提高此任务性能,如Transformer和扩散模型。然而,这些方法在精确姿态重建和快速推理速度之间取得平衡方面遇到了困难。为了克服这些挑战,设计了一个轻便高效的模型SSD-Poser,用于从稀疏观测中进行稳健的全身运动估计。SSD-Poser融入了一个精心设计混合编码器——状态空间注意力编码器,以适应状态空间双态到复杂的运动姿态,并实现实时逼真的姿态重建。此外,引入了一种频率感知解码器,以减轻由变频率运动信号引起的抖动,从而显著提高了运动平滑度。在AMASS数据集上的综合实验表明,SSD-Poser在准确性和计算效率方面表现出卓越性能,与最新方法相比,显示出出色的推理效率。
论文及项目相关链接
PDF 9 pages, 6 figures, conference ICMR 2025
Summary
新一代AR/VR应用中全身姿态估计的重要性,难点在于HMD对于下半身的监测不充足。针对该问题,研究人员推出新型模型SSD-Poser解决精准快速的运动估计挑战。它使用State Space Attention Encoders来处理复杂的运动姿态和从稀疏观察中进行实时重建,以及利用Frequency-Aware Decoder解决由不同频率信号导致的抖动问题。在AMASS数据集上的实验证明SSD-Poser准确高效,具备出色的推理效率。
Key Takeaways
- AR/VR应用的增长对全身姿态估计提出了更高要求,特别是在使用头戴式显示器(HMDs)的情况下。
- HMDs提供的头部和手部信号对于全身姿态重建是一个挑战。
- 现有方法如Transformer和扩散模型在精确姿态重建和快速推理速度之间难以取得平衡。
- SSD-Poser模型被设计用于从稀疏观察中进行稳健的全身运动估计。
- SSD-Poser采用State Space Attention Encoders以适应复杂的运动姿态和实时重建。
- Frequency-Aware Decoder用于解决由不同频率运动信号引起的抖动问题,提高了运动平滑度。
点此查看论文截图
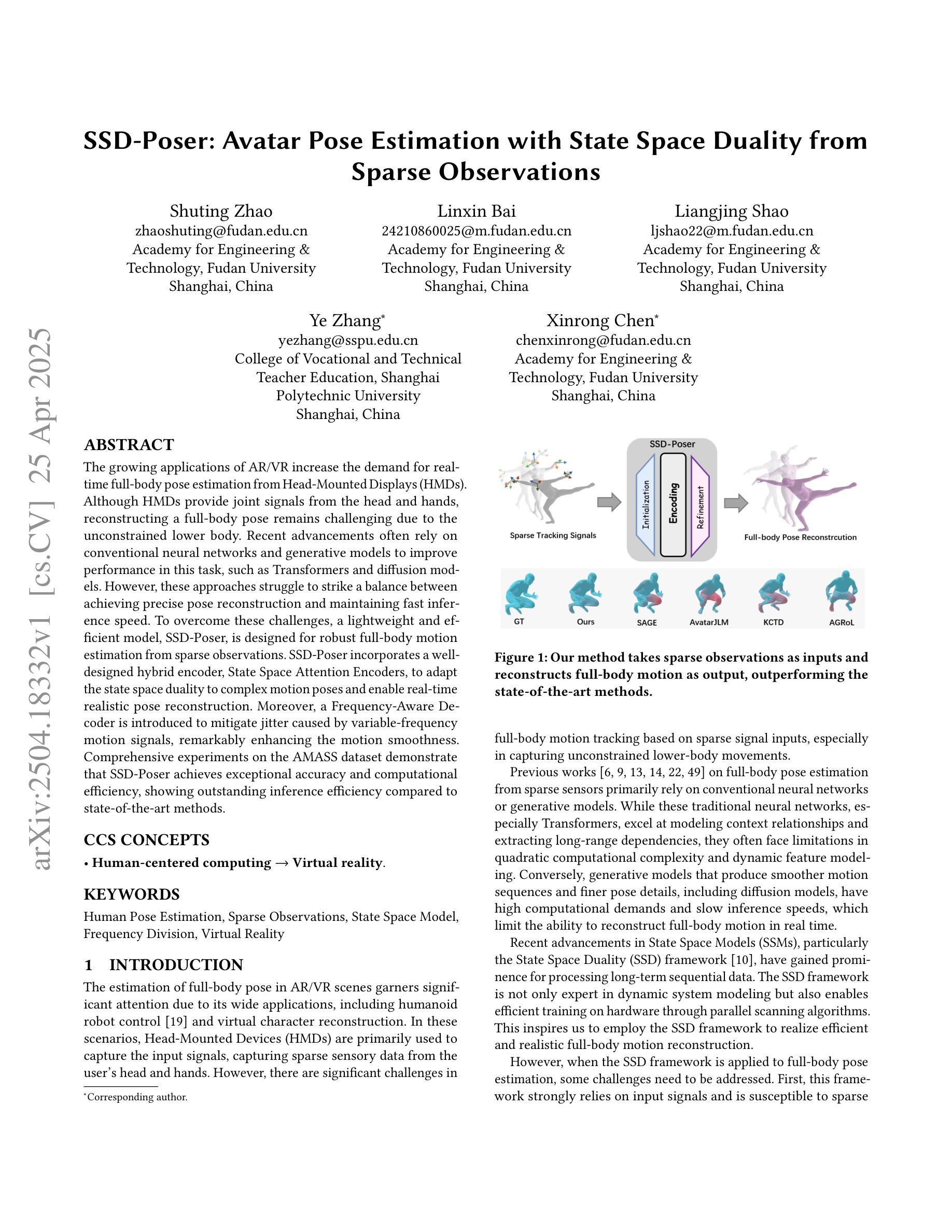
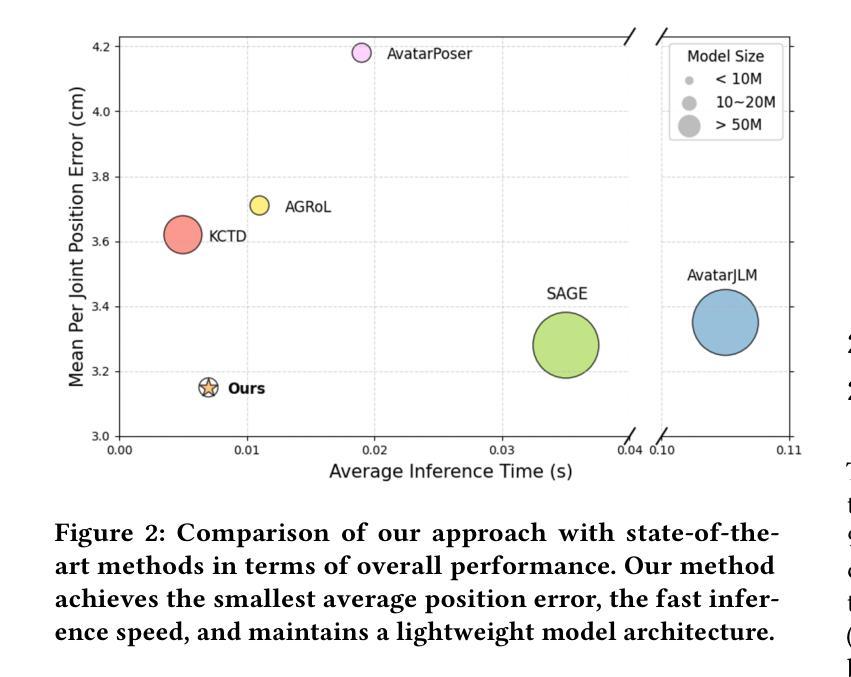
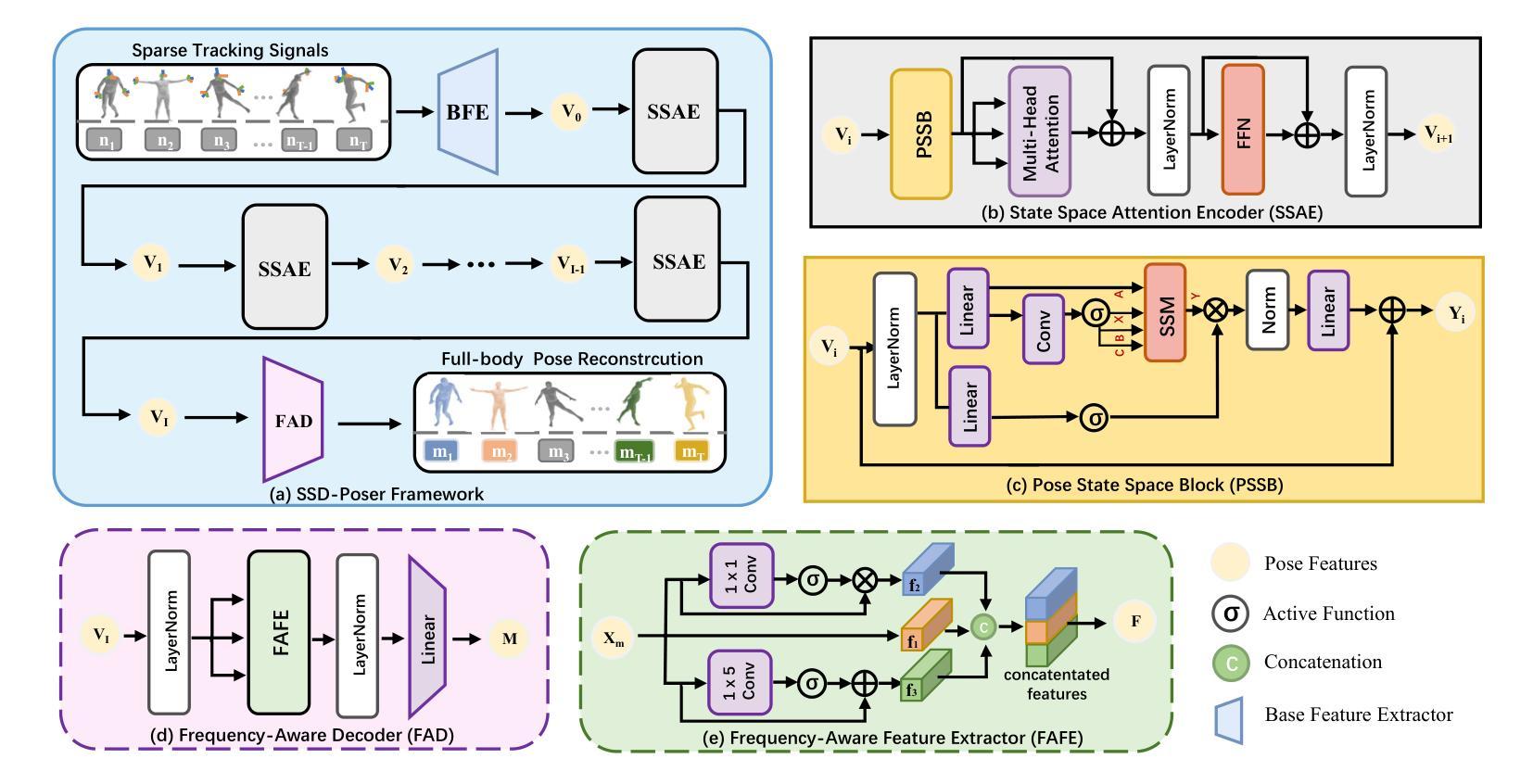
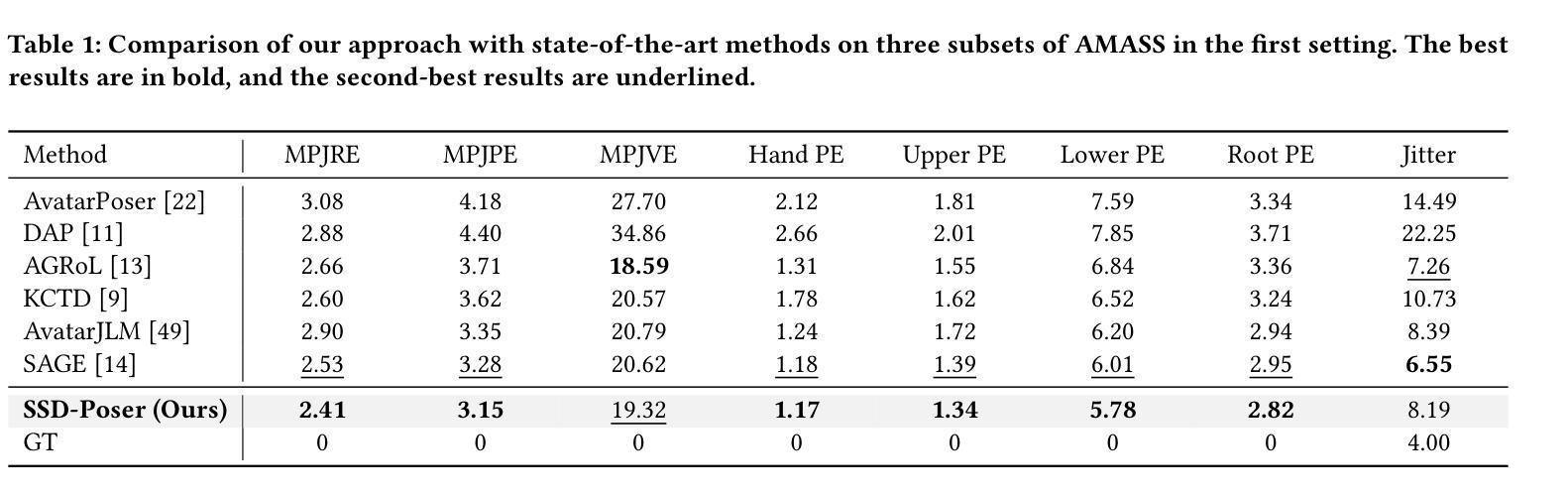
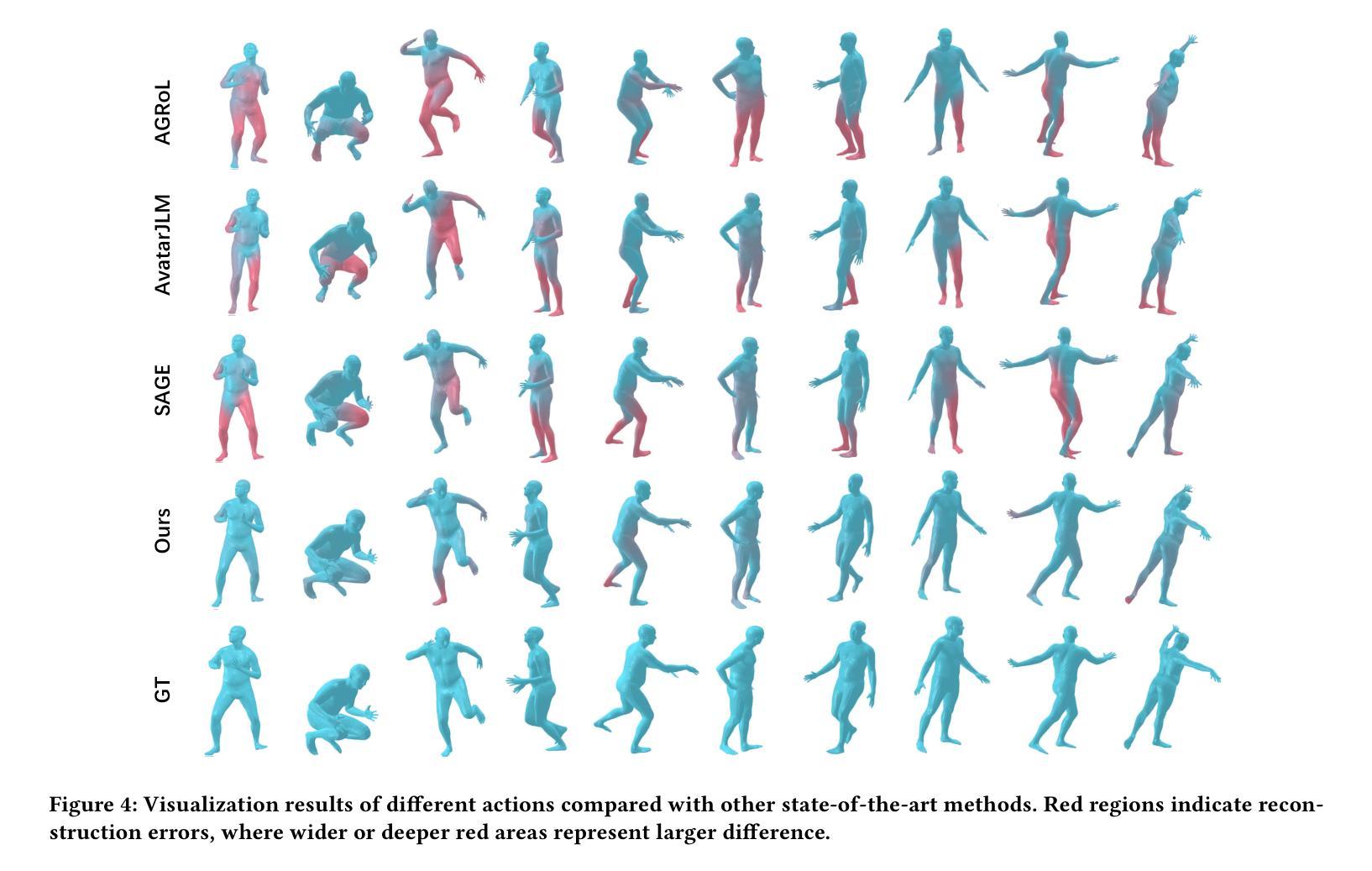