⚠️ 以下所有内容总结都来自于 大语言模型的能力,如有错误,仅供参考,谨慎使用
🔴 请注意:千万不要用于严肃的学术场景,只能用于论文阅读前的初筛!
💗 如果您觉得我们的项目对您有帮助 ChatPaperFree ,还请您给我们一些鼓励!⭐️ HuggingFace免费体验
2025-04-29 更新
Iterative Event-based Motion Segmentation by Variational Contrast Maximization
Authors:Ryo Yamaki, Shintaro Shiba, Guillermo Gallego, Yoshimitsu Aoki
Event cameras provide rich signals that are suitable for motion estimation since they respond to changes in the scene. As any visual changes in the scene produce event data, it is paramount to classify the data into different motions (i.e., motion segmentation), which is useful for various tasks such as object detection and visual servoing. We propose an iterative motion segmentation method, by classifying events into background (e.g., dominant motion hypothesis) and foreground (independent motion residuals), thus extending the Contrast Maximization framework. Experimental results demonstrate that the proposed method successfully classifies event clusters both for public and self-recorded datasets, producing sharp, motion-compensated edge-like images. The proposed method achieves state-of-the-art accuracy on moving object detection benchmarks with an improvement of over 30%, and demonstrates its possibility of applying to more complex and noisy real-world scenes. We hope this work broadens the sensitivity of Contrast Maximization with respect to both motion parameters and input events, thus contributing to theoretical advancements in event-based motion segmentation estimation. https://github.com/aoki-media-lab/event_based_segmentation_vcmax
事件相机提供的丰富信号非常适合于运动估计,因为它们对场景的变化作出反应。由于场景中的任何视觉变化都会产生事件数据,因此将数据分类为不同的运动(即运动分割)至关重要,这对于对象检测和视觉伺服等各种任务都很有用。我们提出了一种迭代运动分割方法,通过将事件分类为背景(例如,主要运动假设)和前景(独立运动残差),从而扩展了对比度最大化框架。实验结果表明,该方法成功地对公共和自我记录的数据集的事件集群进行了分类,产生了清晰、运动补偿的边缘图像。该方法在运动对象检测基准测试上达到了最先进的准确性,提高了30%以上,并证明了其在更复杂和嘈杂的现实场景中的应用可能性。我们希望这项工作能扩大对比度最大化对运动参数和输入事件的敏感性,从而为基于事件的运动分割估计理论做出贡献。详情请参见:https://github.com/aoki-media-lab/event_based_segmentation_vcmax。
论文及项目相关链接
PDF 11 pages, 9 figures, 3 tables, CVPR Workshop 2025
Summary
事件相机提供的丰富信号非常适合用于运动估计,因为它们对场景的变化作出反应。场景中的任何视觉变化都会产生事件数据,因此将数据分类为不同的运动(即运动分割)至关重要,这有助于各种任务,如目标检测和视觉伺服。本文提出一种迭代运动分割方法,通过事件分类为背景(例如主导运动假设)和前景(独立运动残差),扩展对比最大化框架。实验结果表明,该方法成功地对公共和自我记录的数据集的事件集群进行分类,产生清晰、运动补偿的边缘图像。该方法在移动目标检测基准测试中实现了最先进的准确性,提高了超过30%,并证明其适用于更复杂和嘈杂的现实世界场景。本文希望这项工作能提高对比最大化对运动参数和输入事件的敏感性,从而为事件基础运动分割估计的理论发展做出贡献。
Key Takeaways
- 事件相机响应场景变化产生丰富的事件数据,适用于运动估计。
- 运动分割是分类事件数据的关键步骤,有助于目标检测和视觉伺服等任务。
- 提出一种基于迭代方法的运动分割技术,通过分类事件数据(背景和前景)扩展对比最大化框架。
- 实验证明该方法在公共和自我记录的数据集上有效分类事件集群。
- 所提出的方法在移动目标检测方面达到了最先进的准确性,并显著提高性能。
- 该方法适应于复杂和嘈杂的现实世界场景。
点此查看论文截图
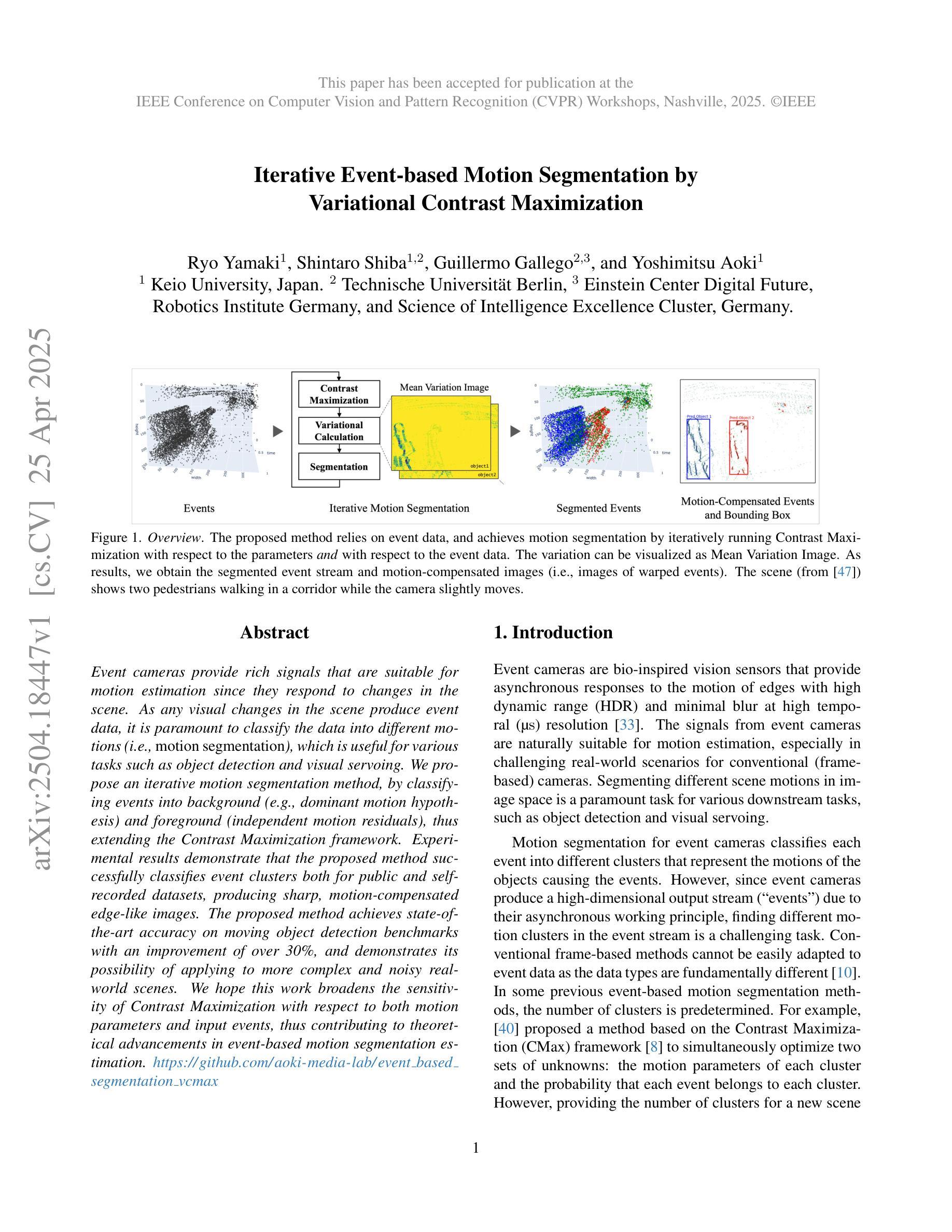
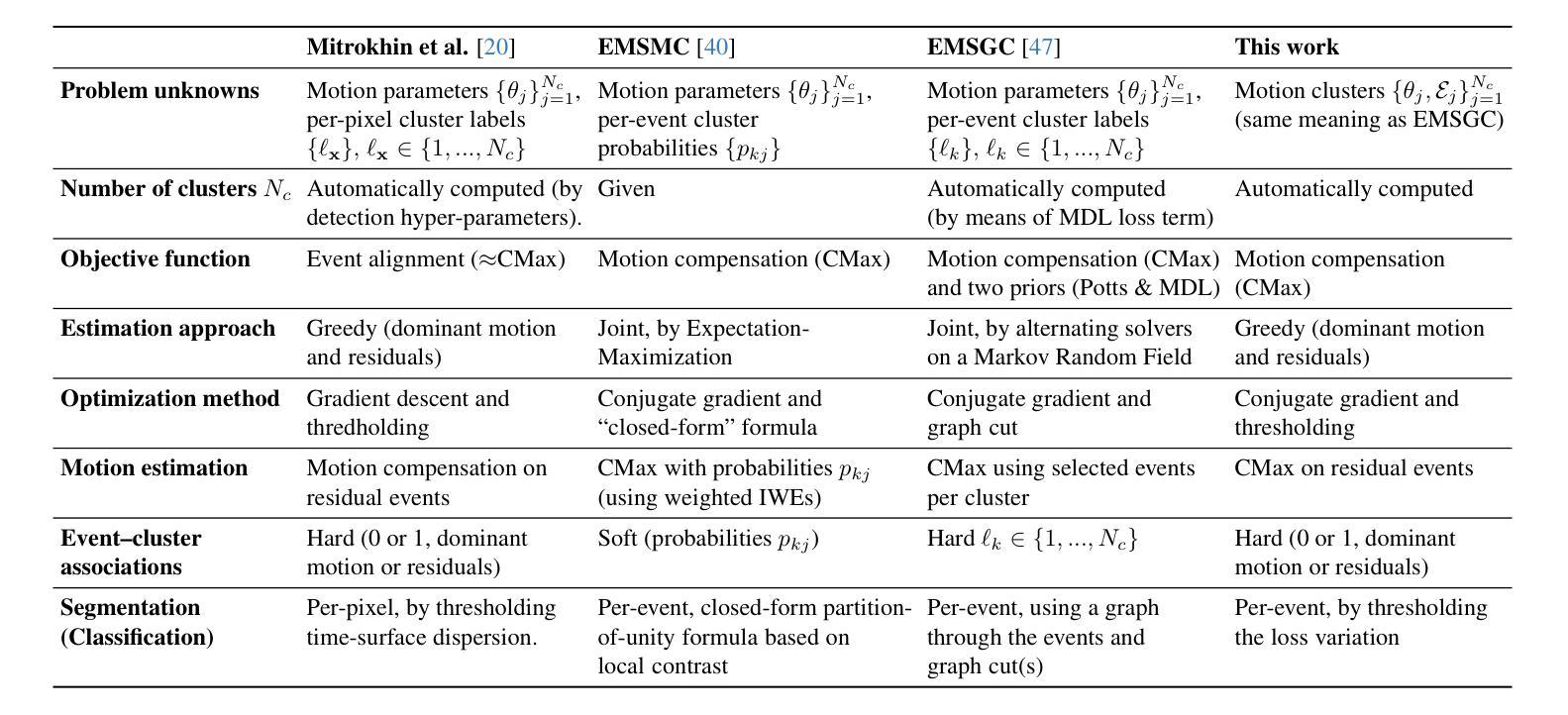
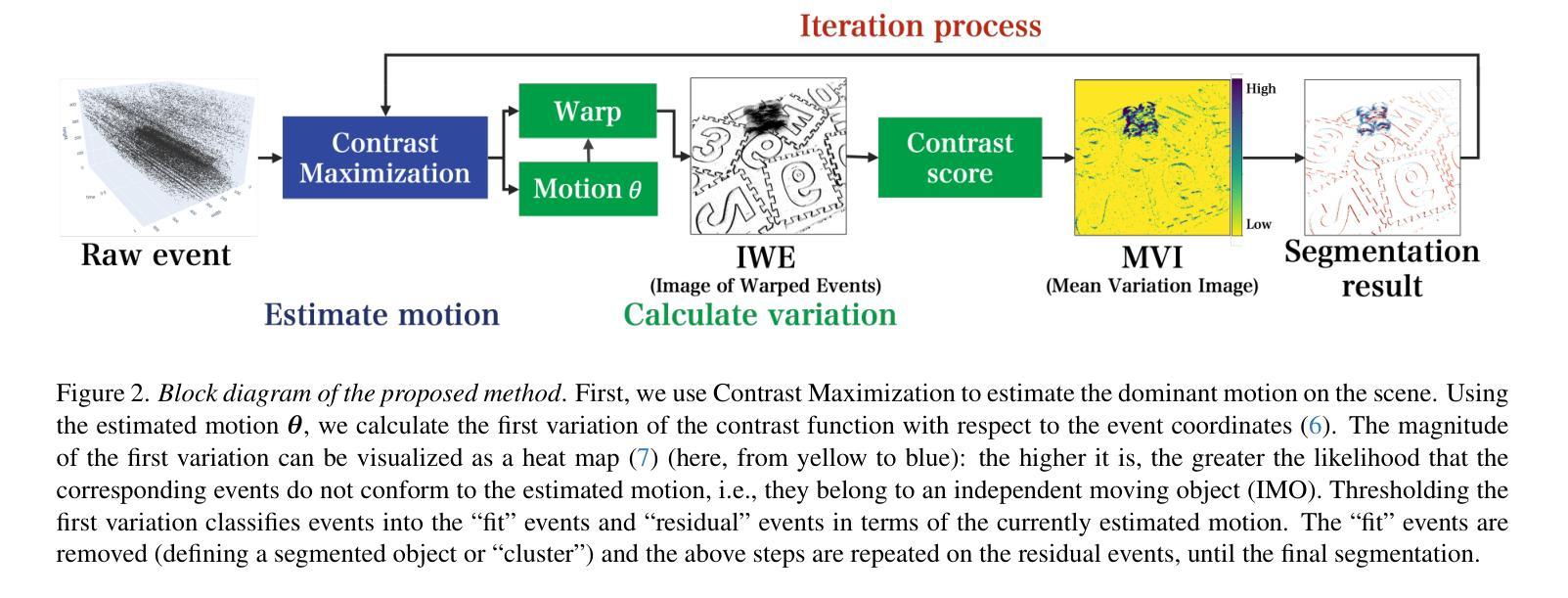
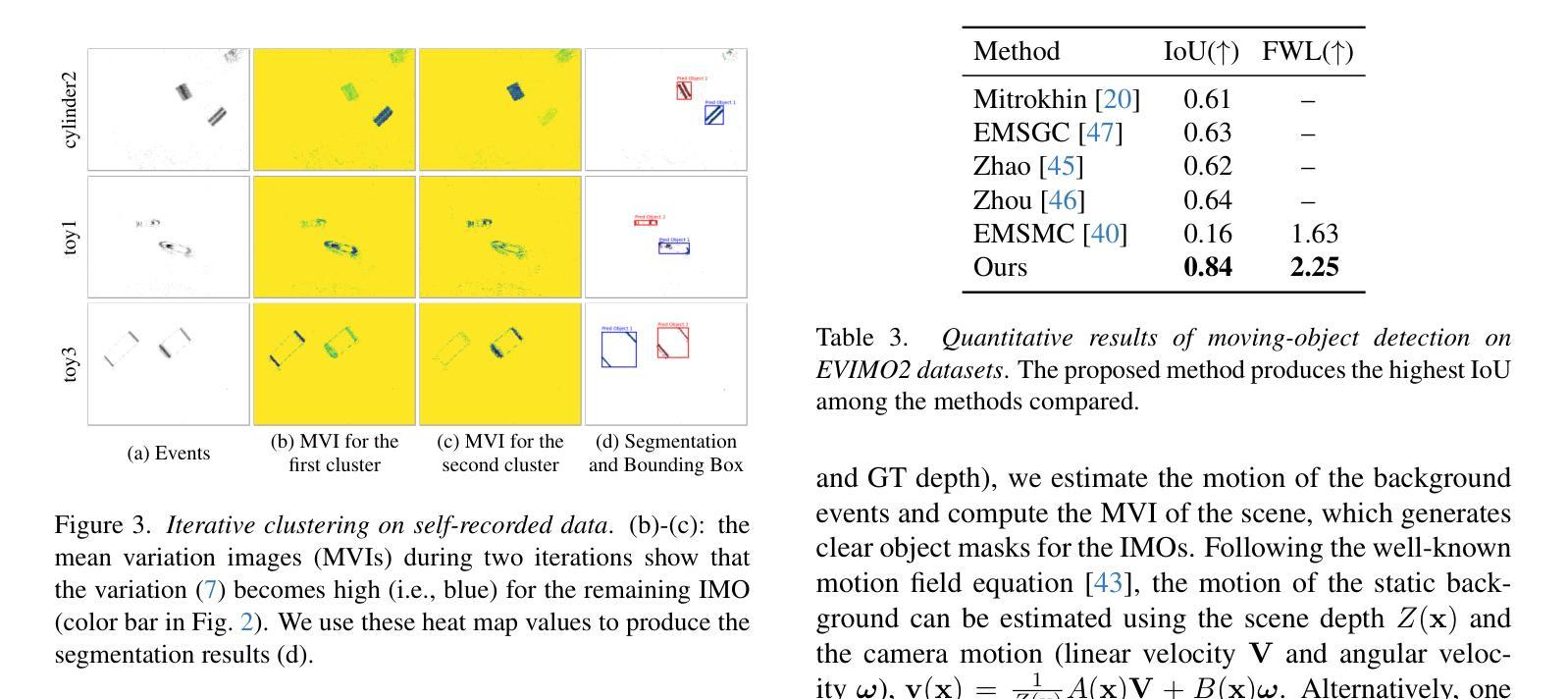
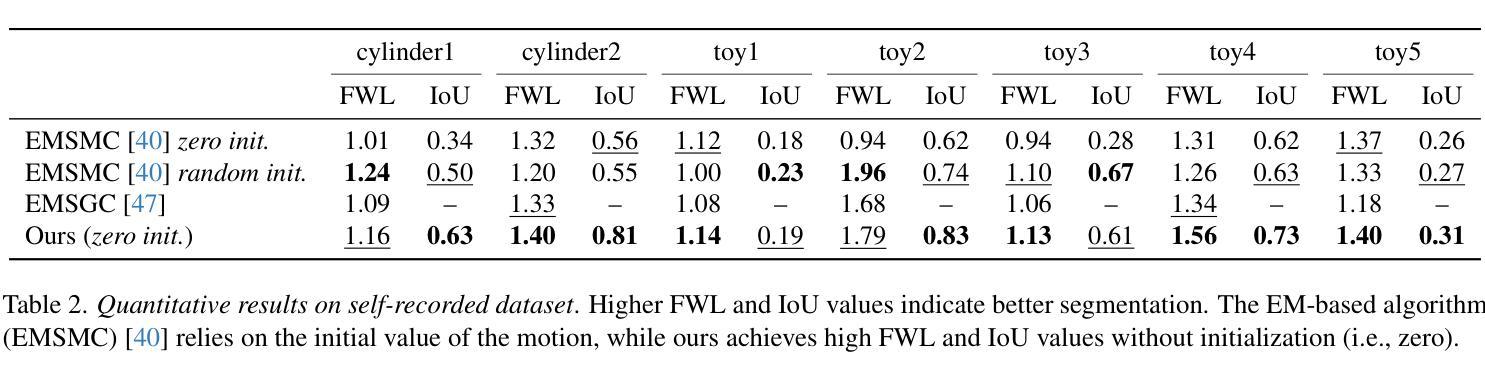
CLOC: Contrastive Learning for Ordinal Classification with Multi-Margin N-pair Loss
Authors:Dileepa Pitawela, Gustavo Carneiro, Hsiang-Ting Chen
In ordinal classification, misclassifying neighboring ranks is common, yet the consequences of these errors are not the same. For example, misclassifying benign tumor categories is less consequential, compared to an error at the pre-cancerous to cancerous threshold, which could profoundly influence treatment choices. Despite this, existing ordinal classification methods do not account for the varying importance of these margins, treating all neighboring classes as equally significant. To address this limitation, we propose CLOC, a new margin-based contrastive learning method for ordinal classification that learns an ordered representation based on the optimization of multiple margins with a novel multi-margin n-pair loss (MMNP). CLOC enables flexible decision boundaries across key adjacent categories, facilitating smooth transitions between classes and reducing the risk of overfitting to biases present in the training data. We provide empirical discussion regarding the properties of MMNP and show experimental results on five real-world image datasets (Adience, Historical Colour Image Dating, Knee Osteoarthritis, Indian Diabetic Retinopathy Image, and Breast Carcinoma Subtyping) and one synthetic dataset simulating clinical decision bias. Our results demonstrate that CLOC outperforms existing ordinal classification methods and show the interpretability and controllability of CLOC in learning meaningful, ordered representations that align with clinical and practical needs.
在顺序分类中,误分类相邻等级是常见的,但这些错误的后果并不相同。例如,误分类良性肿瘤类别相较于在癌前病变到癌症阈值的错误来说,后果较轻。后者可能会深刻影响治疗选择。尽管如此,现有的顺序分类方法并没有考虑到这些边际的不同重要性,将所有相邻类别视为同等重要。为了解决这一局限性,我们提出了CLOC,这是一种新的基于边距的对比学习方法,用于顺序分类。它基于优化多种边距学习有序表示,采用了新型的多边距n对损失(MMNP)。CLOC能够在关键相邻类别之间实现灵活决策边界,促进类之间的平稳过渡,并减少了对训练数据中存在的偏见过拟合的风险。我们针对MMNP的属性进行了实证讨论,并在五个真实图像数据集(Adience、历史彩色图像时代、膝骨关节炎、印度糖尿病视网膜病变图像和乳腺癌亚型)和一个模拟临床决策偏见的合成数据集上展示了实验结果。我们的结果表明,CLOC在顺序分类方法上的表现更为出色,并展示了CLOC在学习符合临床和实际需求的具有意义的有序表示方面的可解释性和可控性。
论文及项目相关链接
PDF Accepted in CVPR 2025
Summary
本文提出一种基于边际对比学习的新方法CLOC,用于序数分类。该方法考虑到不同类别间错误分类后果的差异性,通过优化多个边际与新型多边际n对损失函数(MMNP),学习有序表示。CLOC在关键相邻类别之间设置灵活决策边界,促进类别间平滑过渡,降低对训练数据偏见的拟合风险。实验结果显示,CLOC在多个图像数据集上的性能优于现有序数分类方法,并展示了其在学习符合临床和实际需求的有意义、有序表示中的可解释性和可控性。
Key Takeaways
- CLOC是一种针对序数分类的基于边际对比学习的新方法。
- 现有序数分类方法未考虑不同类别错误分类后果的差异性。
- CLOC通过优化多个边际与新型多边际n对损失函数(MMNP)来学习有序表示。
- CLOC在关键相邻类别之间设置灵活决策边界,促进类别间平滑过渡。
- CLOC降低对训练数据偏见的拟合风险。
- 实验证明,CLOC在多个图像数据集上的性能优于现有序数分类方法。
点此查看论文截图
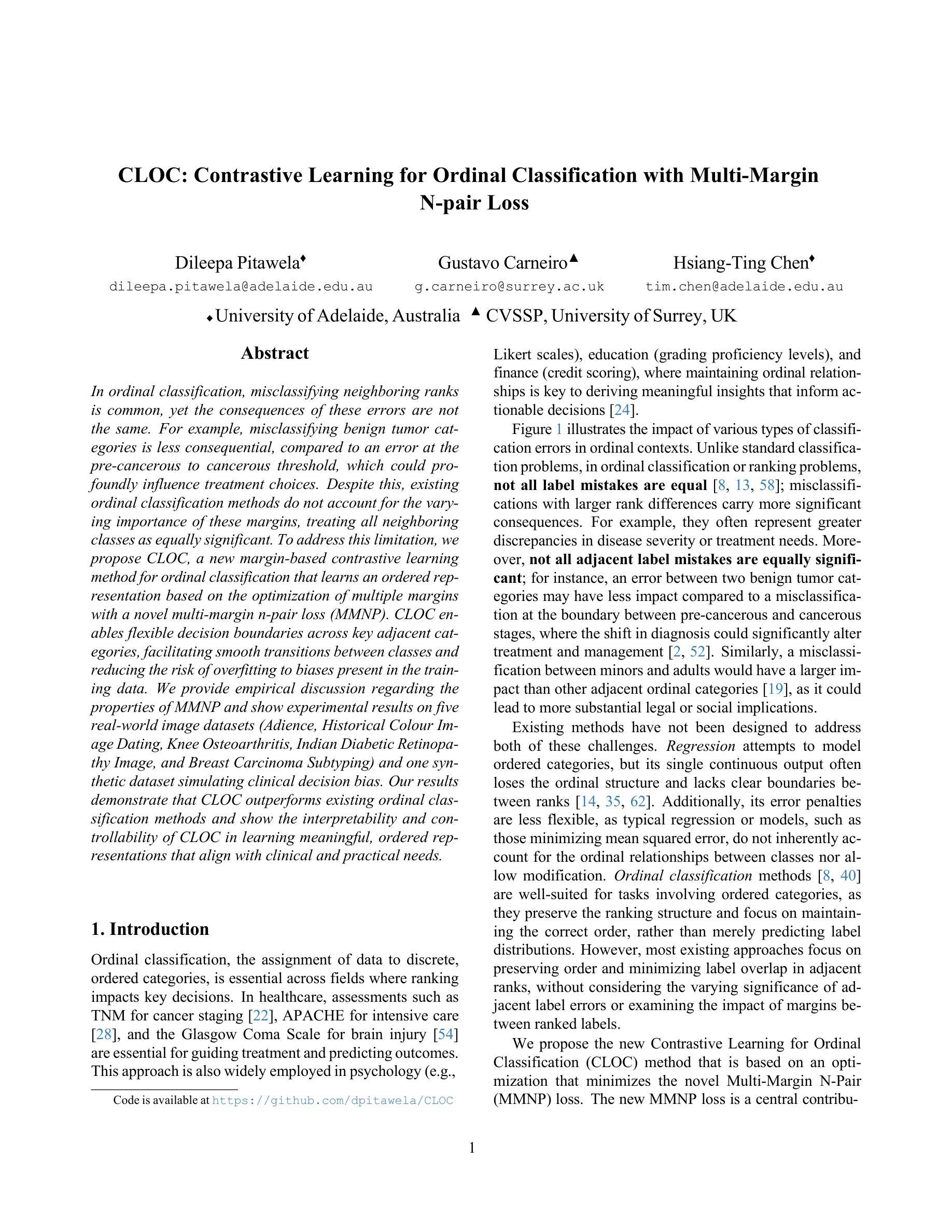
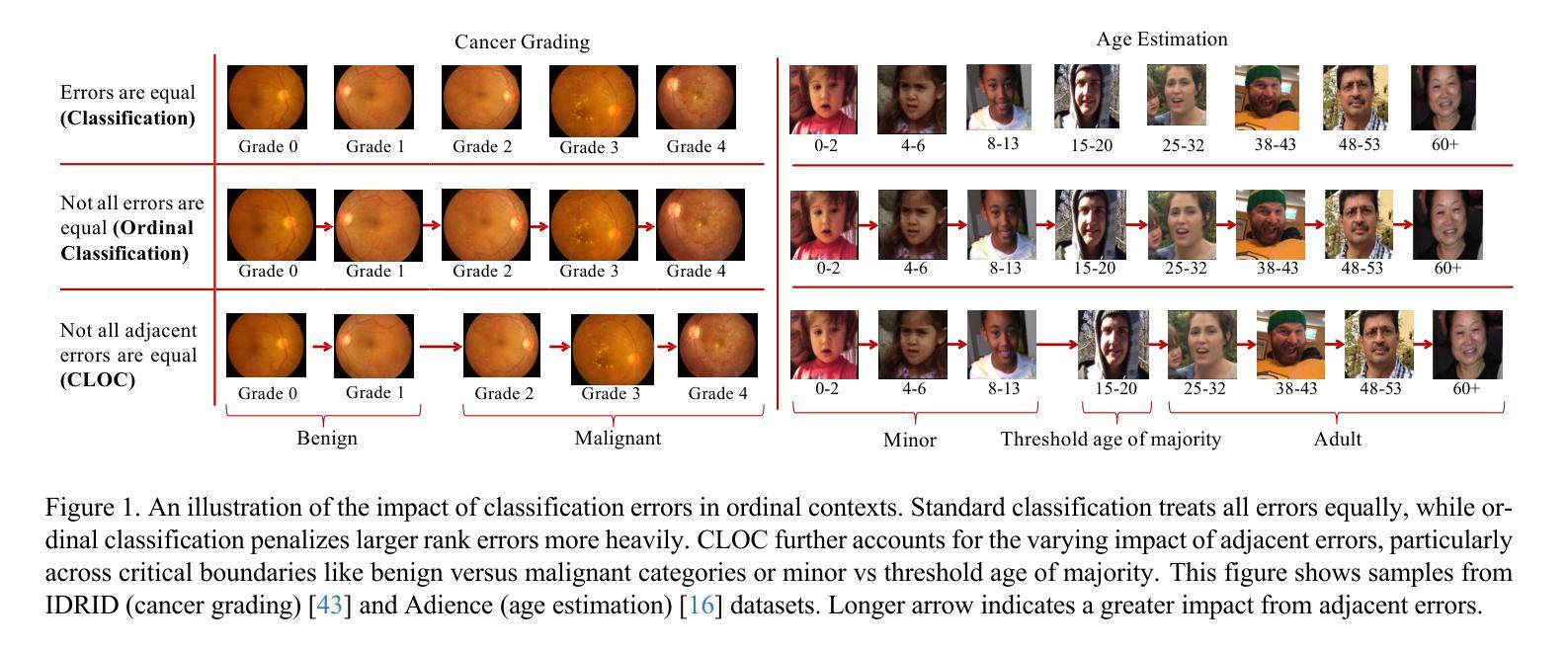
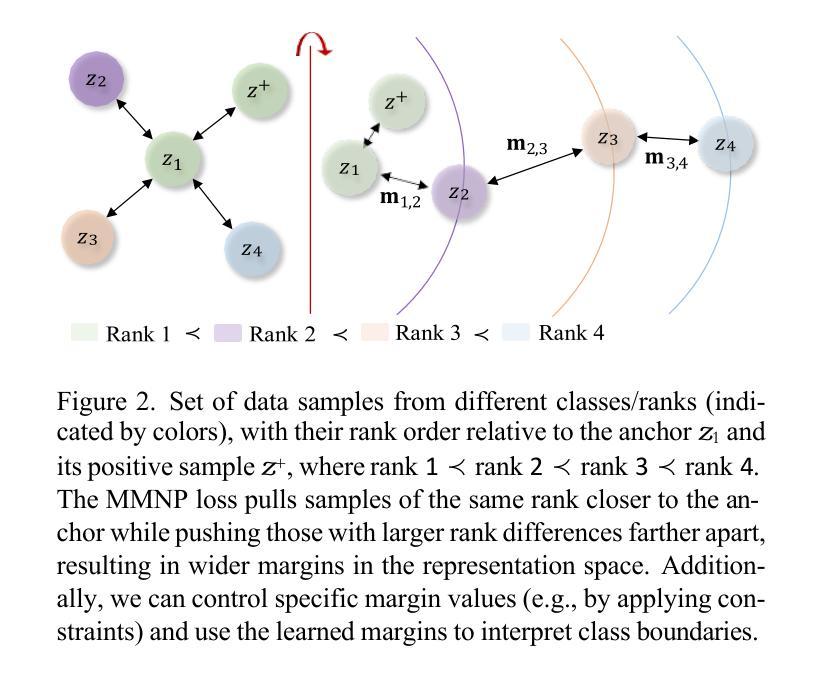
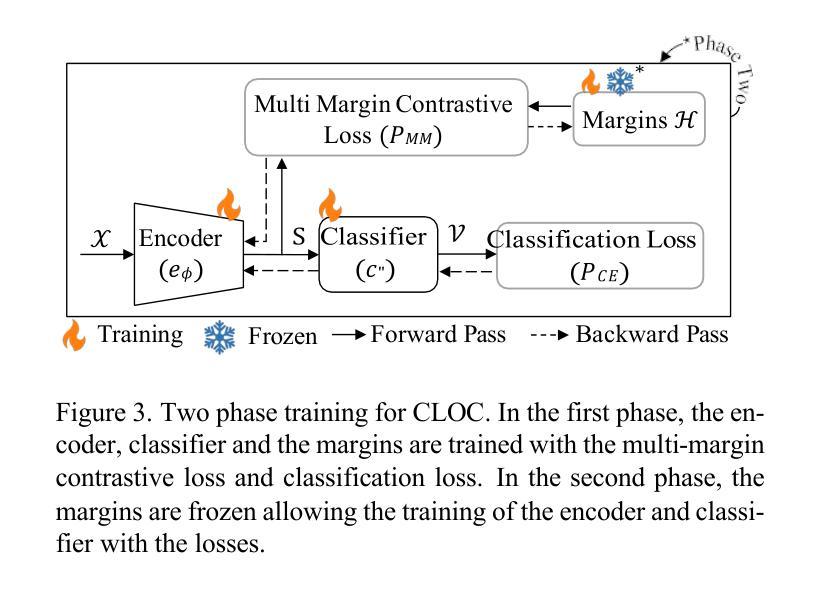
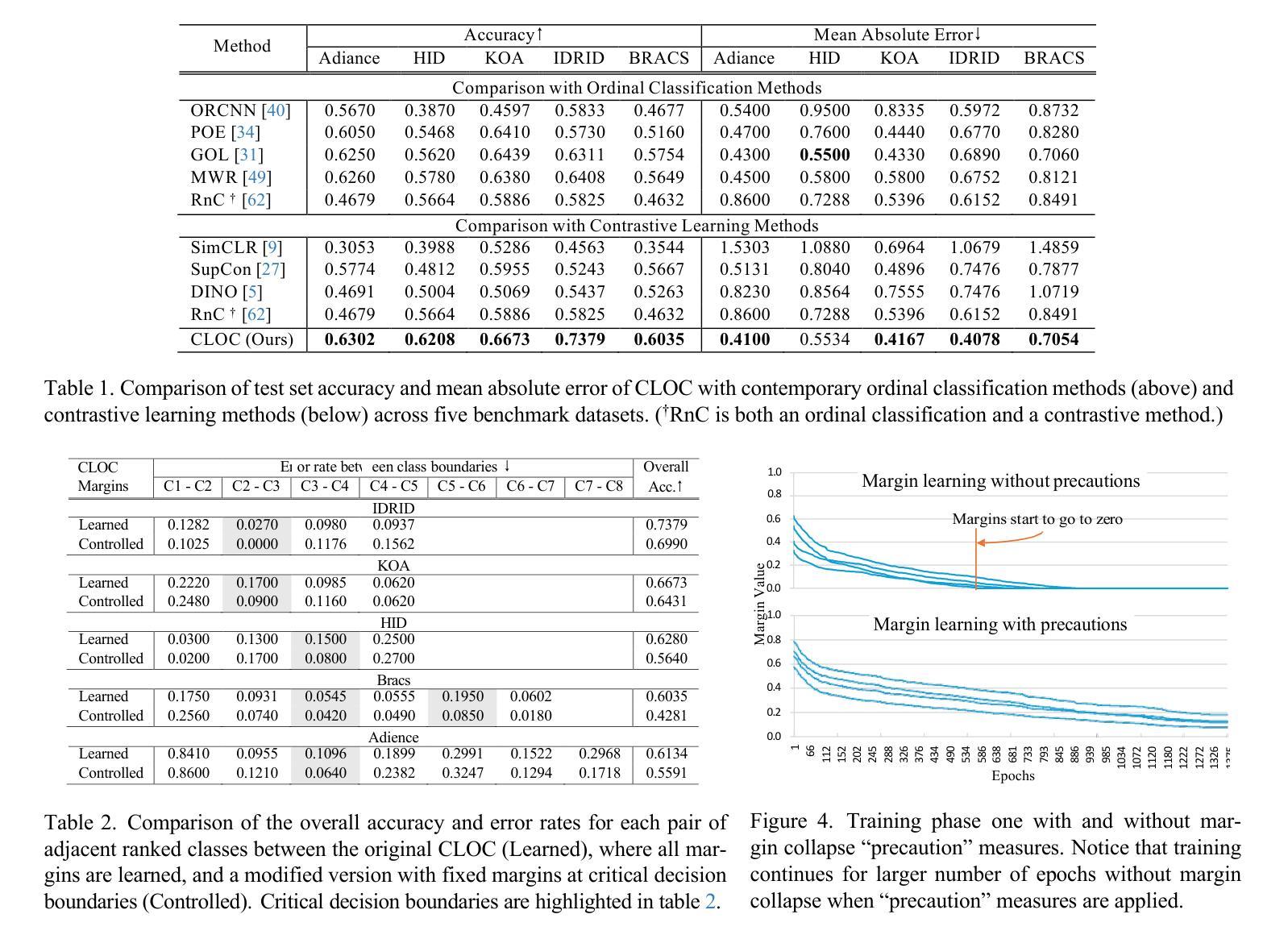