⚠️ 以下所有内容总结都来自于 大语言模型的能力,如有错误,仅供参考,谨慎使用
🔴 请注意:千万不要用于严肃的学术场景,只能用于论文阅读前的初筛!
💗 如果您觉得我们的项目对您有帮助 ChatPaperFree ,还请您给我们一些鼓励!⭐️ HuggingFace免费体验
2025-04-29 更新
MASF-YOLO: An Improved YOLOv11 Network for Small Object Detection on Drone View
Authors:Liugang Lu, Dabin He, Congxiang Liu, Zhixiang Deng
With the rapid advancement of Unmanned Aerial Vehicle (UAV) and computer vision technologies, object detection from UAV perspectives has emerged as a prominent research area. However, challenges for detection brought by the extremely small proportion of target pixels, significant scale variations of objects, and complex background information in UAV images have greatly limited the practical applications of UAV. To address these challenges, we propose a novel object detection network Multi-scale Context Aggregation and Scale-adaptive Fusion YOLO (MASF-YOLO), which is developed based on YOLOv11. Firstly, to tackle the difficulty of detecting small objects in UAV images, we design a Multi-scale Feature Aggregation Module (MFAM), which significantly improves the detection accuracy of small objects through parallel multi-scale convolutions and feature fusion. Secondly, to mitigate the interference of background noise, we propose an Improved Efficient Multi-scale Attention Module (IEMA), which enhances the focus on target regions through feature grouping, parallel sub-networks, and cross-spatial learning. Thirdly, we introduce a Dimension-Aware Selective Integration Module (DASI), which further enhances multi-scale feature fusion capabilities by adaptively weighting and fusing low-dimensional features and high-dimensional features. Finally, we conducted extensive performance evaluations of our proposed method on the VisDrone2019 dataset. Compared to YOLOv11-s, MASFYOLO-s achieves improvements of 4.6% in mAP@0.5 and 3.5% in mAP@0.5:0.95 on the VisDrone2019 validation set. Remarkably, MASF-YOLO-s outperforms YOLOv11-m while requiring only approximately 60% of its parameters and 65% of its computational cost. Furthermore, comparative experiments with state-of-the-art detectors confirm that MASF-YOLO-s maintains a clear competitive advantage in both detection accuracy and model efficiency.
随着无人机(UAV)和计算机视觉技术的快速发展,从无人机视角进行目标检测已成为一个突出的研究领域。然而,目标像素比例极小、物体尺寸变化显著以及无人机图像中背景信息复杂等检测挑战,极大地限制了无人机的实际应用。为了解决这些挑战,我们提出了一种新型的目标检测网络——多尺度上下文聚合和自适应尺度融合YOLO(MASF-YOLO),该网络是在YOLOv11的基础上开发的。首先,为了解决无人机图像中检测小物体的困难,我们设计了一个多尺度特征聚合模块(MFAM),通过并行多尺度卷积和特征融合,显著提高小物体的检测精度。其次,为了减轻背景噪声的干扰,我们提出了一个改进的高效多尺度注意模块(IEMA),它通过特征分组、并行子网络和跨空间学习来增强对目标区域的关注。第三,我们引入了一个维度感知选择性集成模块(DASI),通过自适应加权融合低维特征和高维特征,进一步增强了多尺度特征融合能力。最后,我们在VisDrone2019数据集上对我们的方法进行了性能评估。与YOLOv11-s相比,MASFYOLO-s在VisDrone2019验证集上的mAP@0.5提高了4.6%,在mAP@0.5:0.95上提高了3.5%。值得注意的是,MASF-YOLO-s在参数和计算成本方面仅占用YOLOv11-m的大约60%,但性能却优于后者。此外,与最新检测器的对比实验证实,MASF-YOLO-s在检测精度和模型效率方面均保持明显竞争优势。
论文及项目相关链接
Summary:随着无人机和计算机视觉技术的快速发展,无人机视角下的目标检测已成为一个热门研究领域。针对无人机图像中目标像素比例极小、目标对象尺度变化大以及背景信息复杂等挑战,提出了基于YOLOv1的新型目标检测网络MASF-YOLO。该网络通过设计多尺度特征聚合模块、改进的多尺度注意力模块以及维度感知选择性集成模块,提高了小目标的检测精度,减轻了背景噪声的干扰,增强了多尺度特征融合能力。在VisDrone2019数据集上的实验表明,MASF-YOLO-s相较于YOLOv11在检测精度上有所提升,同时模型效率和参数需求也更为优秀。
Key Takeaways:
- UAV视角下的目标检测是一个重要的研究领域。
- 面临目标像素比例小、目标尺度变化和复杂背景等挑战。
- 提出了基于YOLOv1的新型网络MASF-YOLO来解决这些挑战。
- MASF-YOLO通过设计多尺度特征聚合模块提高了小目标的检测精度。
- 改进的多尺度注意力模块减轻了背景噪声的干扰。
- 维度感知选择性集成模块增强了多尺度特征融合能力。
- 在VisDrone2019数据集上的实验表明,MASF-YOLO-s在检测精度和模型效率上均有所优势。
点此查看论文截图
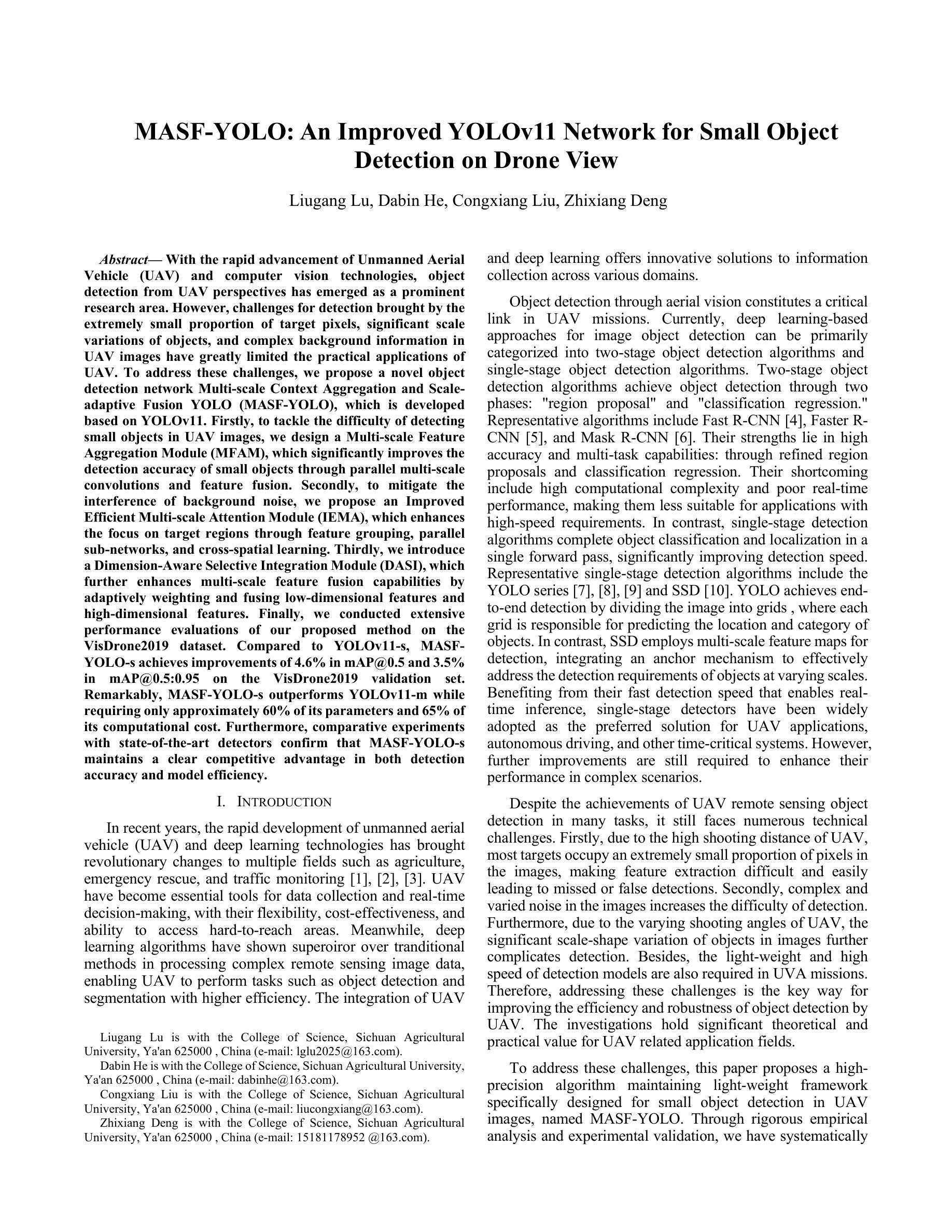
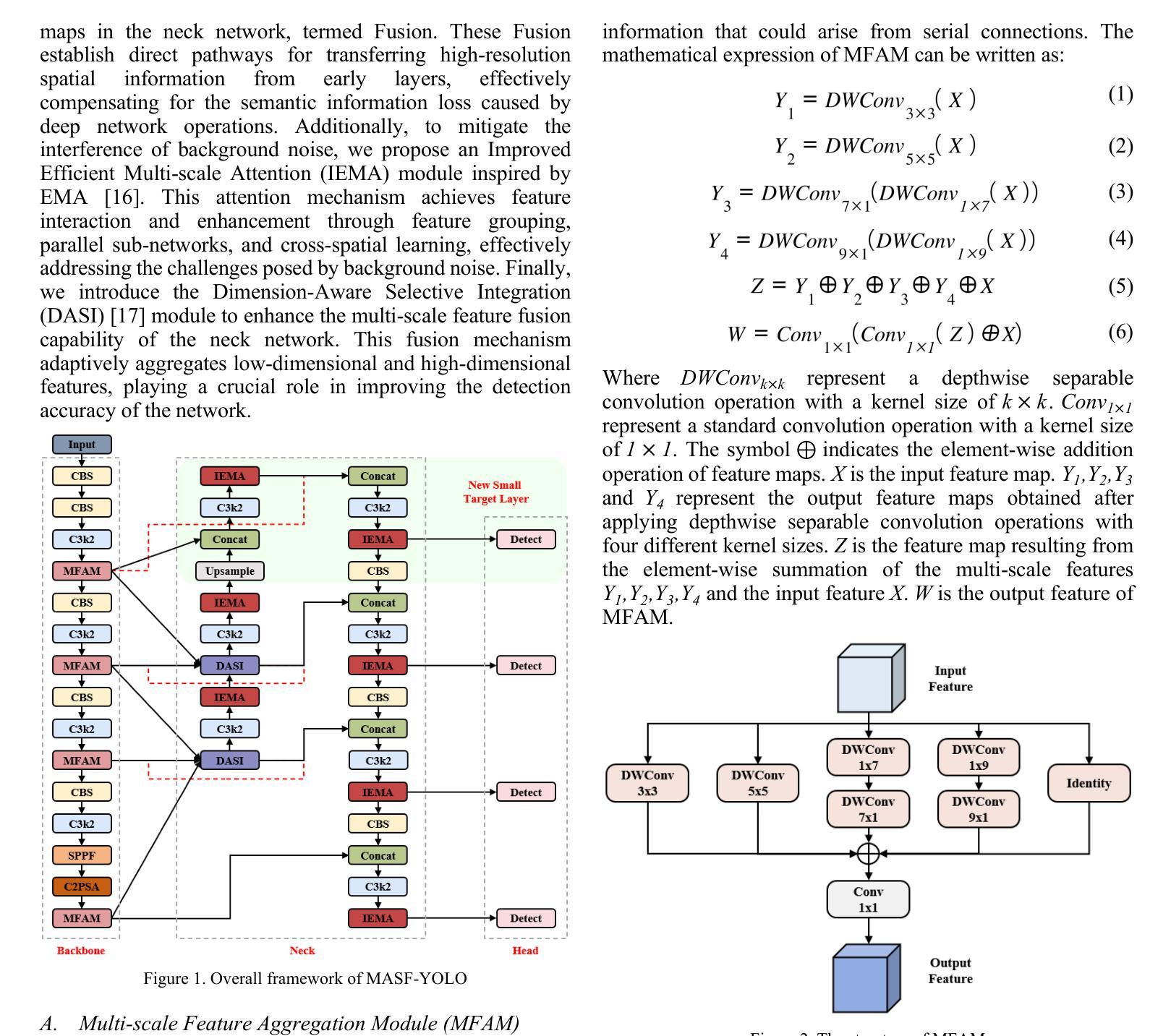
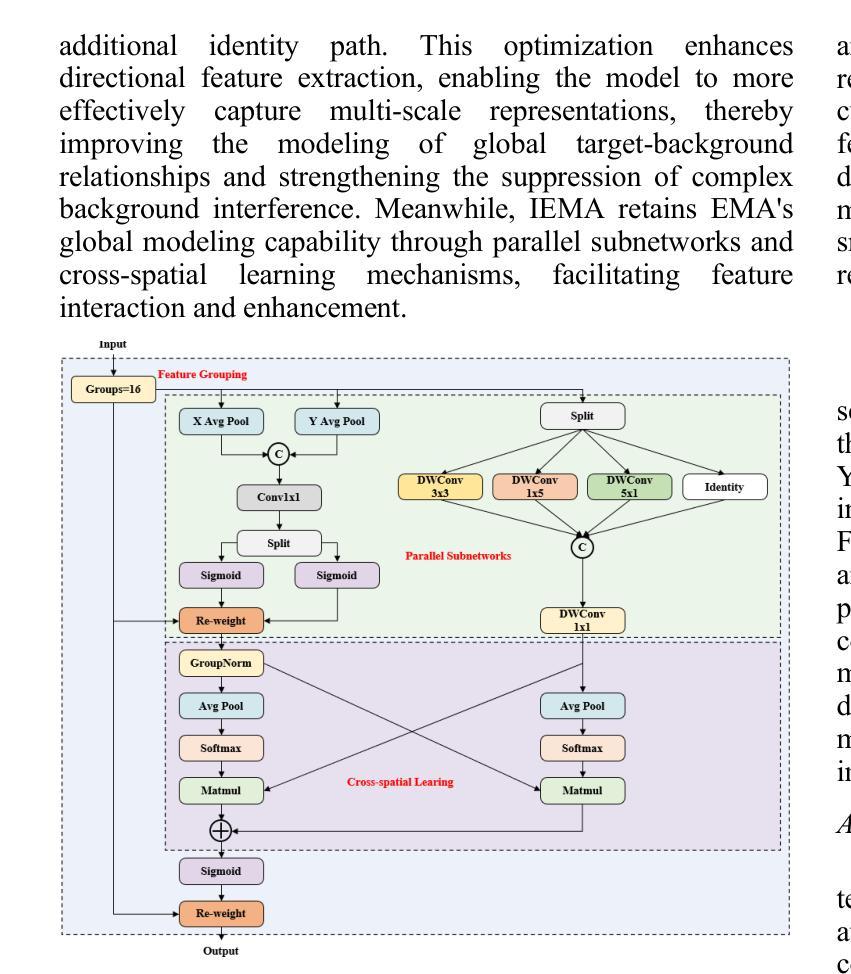
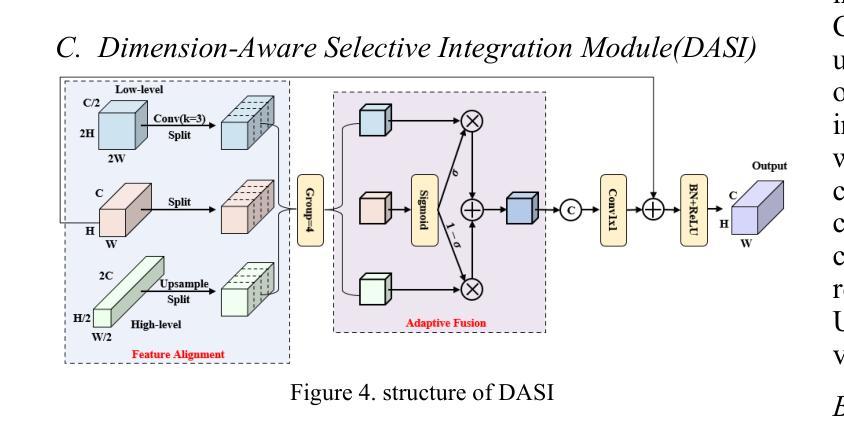
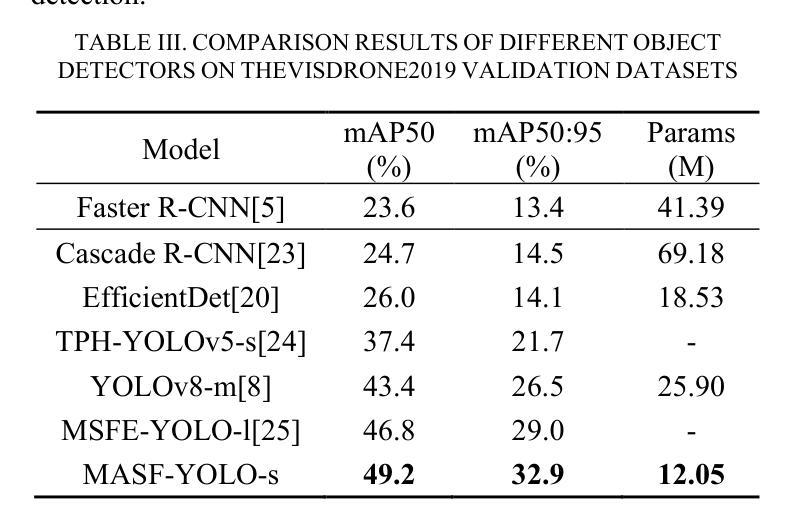
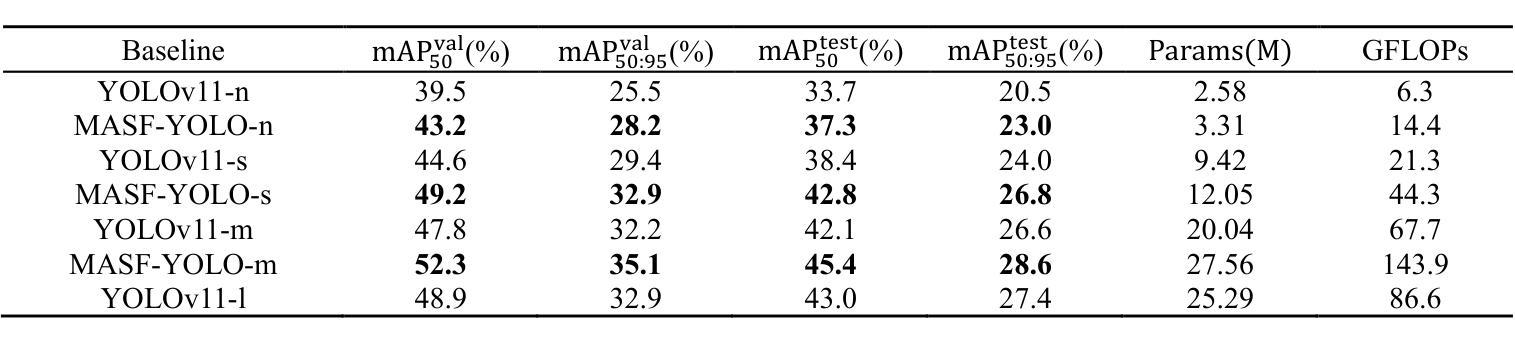
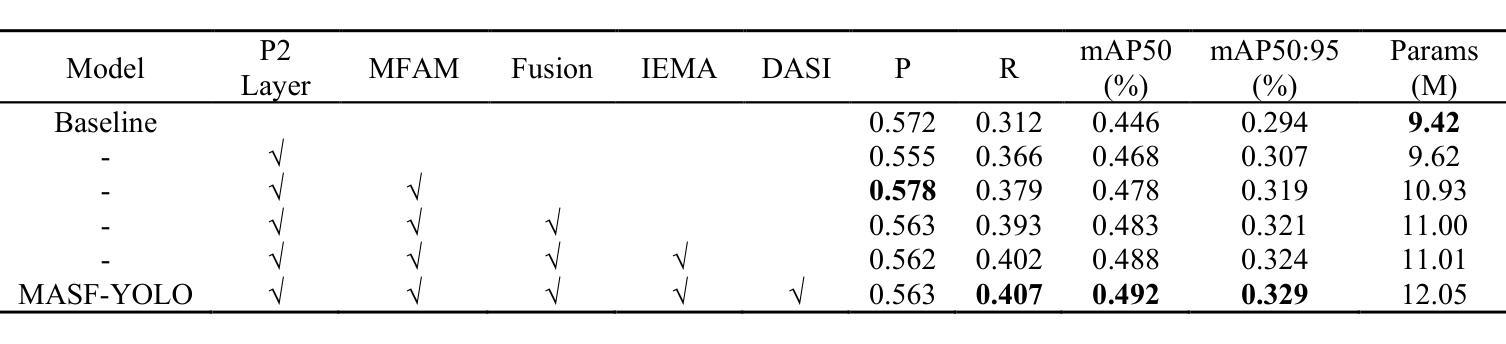
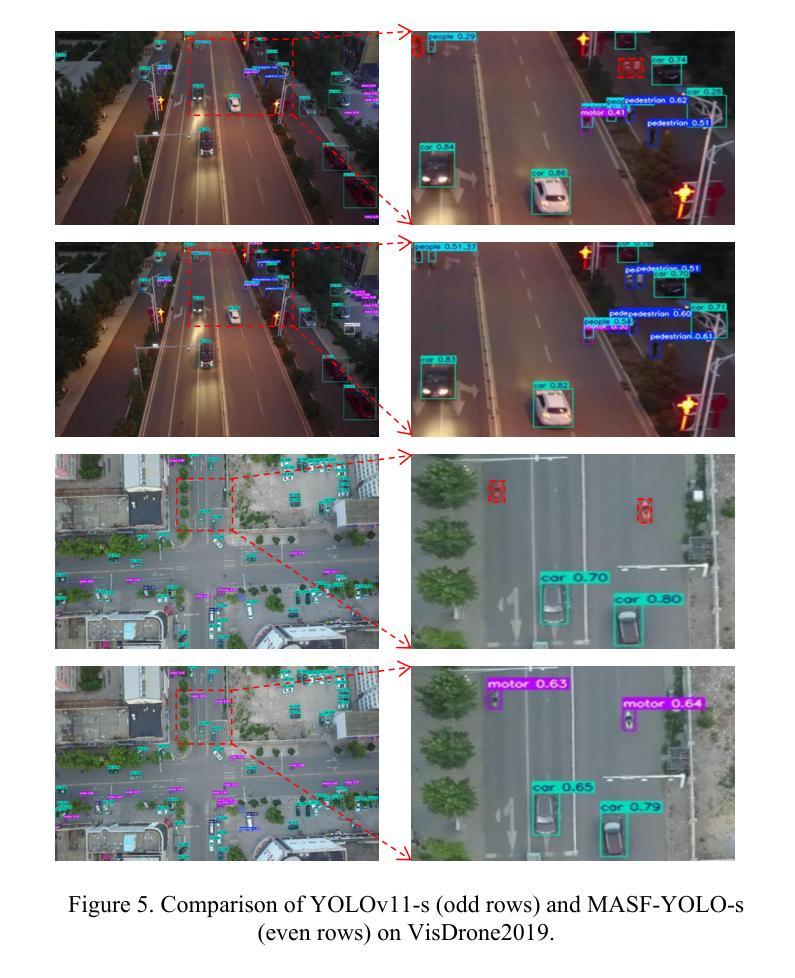