⚠️ 以下所有内容总结都来自于 大语言模型的能力,如有错误,仅供参考,谨慎使用
🔴 请注意:千万不要用于严肃的学术场景,只能用于论文阅读前的初筛!
💗 如果您觉得我们的项目对您有帮助 ChatPaperFree ,还请您给我们一些鼓励!⭐️ HuggingFace免费体验
2025-04-29 更新
TRACE Back from the Future: A Probabilistic Reasoning Approach to Controllable Language Generation
Authors:Gwen Yidou Weng, Benjie Wang, Guy Van den Broeck
As large language models (LMs) advance, there is an increasing need to control their outputs to align with human values (e.g., detoxification) or desired attributes (e.g., personalization, topic). However, autoregressive models focus on next-token predictions and struggle with global properties that require looking ahead. Existing solutions either tune or post-train LMs for each new attribute - expensive and inflexible - or approximate the Expected Attribute Probability (EAP) of future sequences by sampling or training, which is slow and unreliable for rare attributes. We introduce TRACE (Tractable Probabilistic Reasoning for Adaptable Controllable gEneration), a novel framework that efficiently computes EAP and adapts to new attributes through tractable probabilistic reasoning and lightweight control. TRACE distills a Hidden Markov Model (HMM) from an LM and pairs it with a small classifier to estimate attribute probabilities, enabling exact EAP computation over the HMM’s predicted futures. This EAP is then used to reweigh the LM’s next-token probabilities for globally compliant continuations. Empirically, TRACE achieves state-of-the-art results in detoxification with only 10% decoding overhead, adapts to 76 low-resource personalized LLMs within seconds, and seamlessly extends to composite attributes.
随着大型语言模型(LMs)的不断发展,越来越需要控制其输出以符合人类价值观(例如去毒)或所需属性(例如个性化、主题)。然而,自回归模型专注于下一个标记的预测,并努力应对需要前瞻性的全局属性。现有解决方案要么针对每个新属性调整或进行后训练大型语言模型——这既昂贵又缺乏灵活性——要么通过抽样或训练来近似未来序列的预期属性概率(EAP),这对于罕见属性而言既缓慢又不可靠。我们引入了TRACE(用于可控可适应生成的可靠概率推理框架),这是一种新型框架,能够高效计算EAP并根据新的属性进行适应,通过可靠的概率推理和轻量级控制来实现。TRACE通过语言模型蒸馏出隐藏马尔可夫模型(HMM),并将其与小型分类器配对以估计属性概率,从而在HMM预测的未来发展上实现精确的EAP计算。然后,使用此EAP重新权衡语言模型的下一个标记概率,以生成全局合规的延续文本。经验表明,TRACE在仅10%的解码开销下实现了最先进的去毒效果,可在几秒内适应76个低资源个性化大型语言模型,并无缝扩展到复合属性。
论文及项目相关链接
Summary
本文介绍了随着大型语言模型(LMs)的发展,如何控制其输出以符合人类价值观和期望属性。现有方法在处理需要前瞻的全局属性时存在困难,且调整或后训练每个新属性既昂贵又不灵活。为此,作者提出了TRACE框架,它通过可行的概率推理和轻量级控制来适应可控生成。TRACE通过从LM中提炼隐马尔可夫模型(HMM)并配以小型分类器来估计属性概率,能够精确计算HMM预测未来的期望属性概率(EAP)。随后,该EAP被用于重新调整LM的下一个标记概率,以生成全局合规的延续文本。实验表明,TRACE在解毒任务上取得了最佳效果,仅增加了10%的解码开销,并能快速适应低资源个性化LLM,且能无缝扩展到复合属性。
Key Takeaways
- 大型语言模型(LMs)的输出需要控制,以符合人类价值观和期望属性。
- 现有方法在处理需要前瞻的全局属性时存在困难,且调整或后训练每个新属性成本高昂且不灵活。
- TRACE框架通过隐马尔可夫模型(HMM)和分类器来估计属性概率,精确计算期望属性概率(EAP)。
- TRACE实现了对全局合规延续文本的控制,使用EAP重新调整LM的下一个标记概率。
- TRACE在解毒任务上表现最佳,且仅有10%的解码开销。
- TRACE能快速适应低资源个性化LLM。
点此查看论文截图
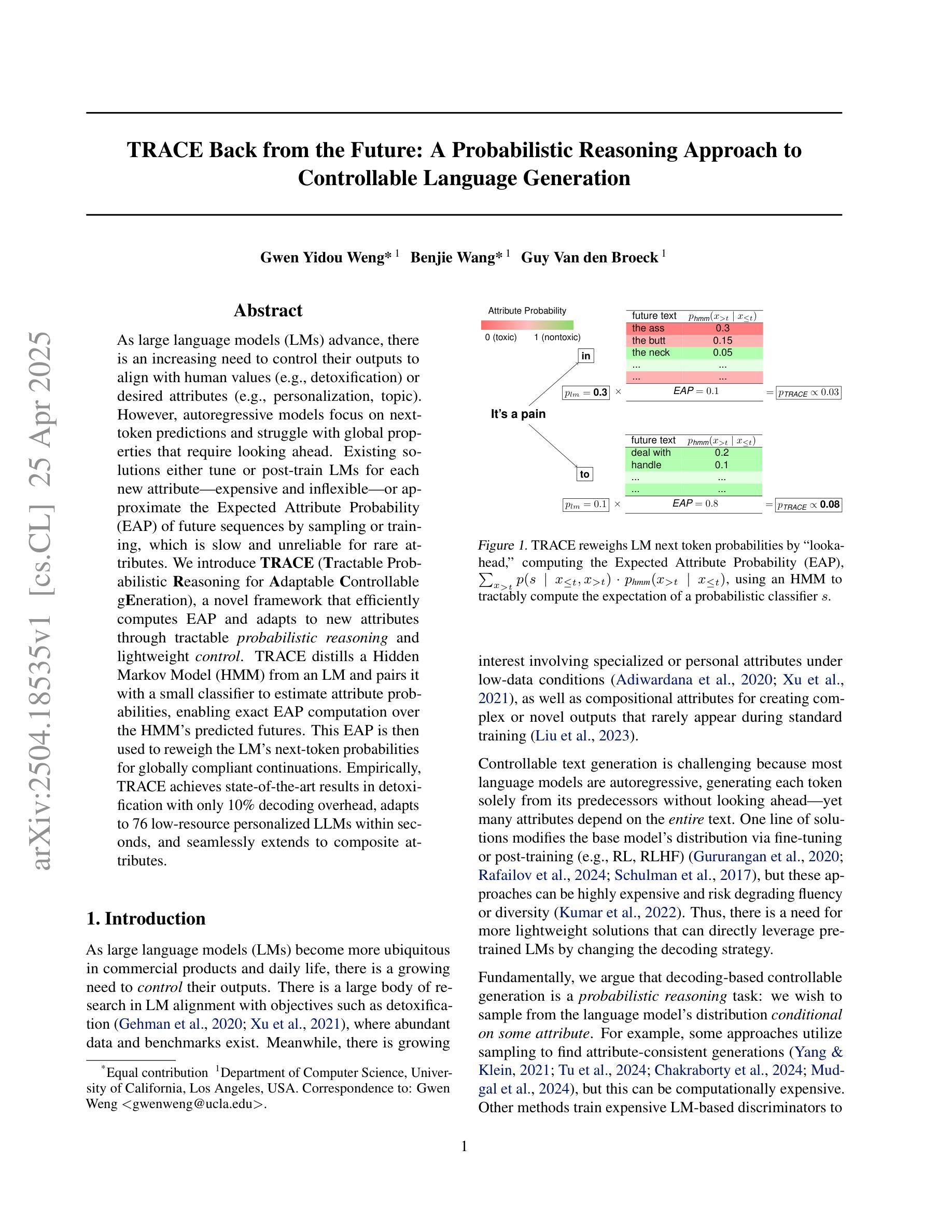
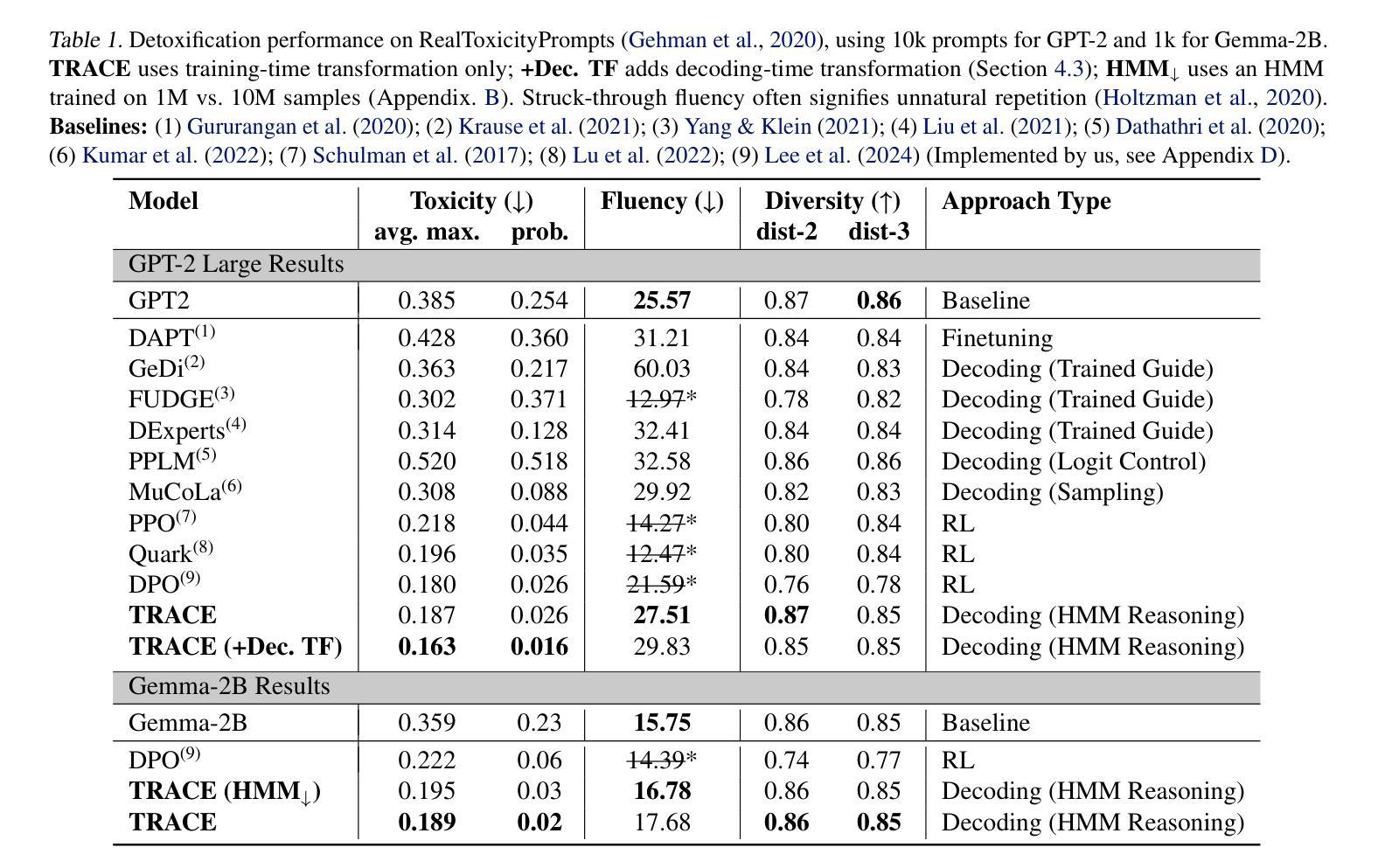
Fast-Slow Thinking for Large Vision-Language Model Reasoning
Authors:Wenyi Xiao, Leilei Gan, Weilong Dai, Wanggui He, Ziwei Huang, Haoyuan Li, Fangxun Shu, Zhelun Yu, Peng Zhang, Hao Jiang, Fei Wu
Recent advances in large vision-language models (LVLMs) have revealed an \textit{overthinking} phenomenon, where models generate verbose reasoning across all tasks regardless of questions. To address this issue, we present \textbf{FAST}, a novel \textbf{Fa}st-\textbf{S}low \textbf{T}hinking framework that dynamically adapts reasoning depth based on question characteristics. Through empirical analysis, we establish the feasibility of fast-slow thinking in LVLMs by investigating how response length and data distribution affect performance. We develop FAST-GRPO with three components: model-based metrics for question characterization, an adaptive thinking reward mechanism, and difficulty-aware KL regularization. Experiments across seven reasoning benchmarks demonstrate that FAST achieves state-of-the-art accuracy with over 10% relative improvement compared to the base model, while reducing token usage by 32.7-67.3% compared to previous slow-thinking approaches, effectively balancing reasoning length and accuracy.
近期大型视觉语言模型(LVLMs)的进步揭示了一种“过度思考”现象,即模型会在所有任务中生成冗长的推理,而无论问题如何。为了解决这一问题,我们提出了FAST,一种新型的Fast-Slow Thinking框架,它根据问题的特性动态适应推理深度。通过实证分析,我们通过在研究响应长度和数据分布如何影响性能的情况下,证实了快速-慢速思考在LVLMs中的可行性。我们开发了FAST-GRPO,包含三个组件:基于模型的度量进行问题特征化、自适应思考奖励机制和难度感知KL正则化。在七个推理基准测试上的实验表明,FAST实现了最先进的准确性,与基础模型相比提高了超过10%的相对改善率。与之前需要缓慢思考的推理方法相比,FAST减少了32.7-67.3%的令牌使用,有效地平衡了推理长度和准确性。
论文及项目相关链接
PDF 16 pages, 5 figures, and 12 tables
Summary
大型视觉语言模型(LVLMs)中的“过度思考”现象引发了广泛关注,该问题导致模型在所有任务中生成冗长的推理,无视问题的实际需求。为解决这一问题,本文提出了名为“FAST”的新型快慢思考框架,该框架能够根据问题的特性动态调整推理深度。通过实证研究,本文验证了快慢思考在LVLMs中的可行性,并探讨了响应长度和数据分布对性能的影响。此外,本文开发了包含三个组件的FAST-GRPO:基于模型的指标进行问题特征化、自适应思考奖励机制和难度感知KL正则化。在七个推理基准测试上的实验表明,FAST实现了最先进的准确性,相较于基础模型有超过10%的相对改进,同时相较于之前的慢思考方法减少了32.7-67.3%的令牌使用,有效平衡了推理长度和准确性。
Key Takeaways
- 大型视觉语言模型(LVLMs)存在“过度思考”现象,即生成冗长推理的问题。
- FAST框架旨在根据问题的特性动态调整推理深度。
- 实证研究验证了快慢思考在LVLMs中的可行性。
- FAST-GRPO包含三个组件:问题特征化、自适应思考奖励机制和难度感知KL正则化。
- 实验表明,FAST在多个推理基准测试上实现了先进准确性。
- FAST相较于基础模型有显著的性能改进,相对改进超过10%。
点此查看论文截图
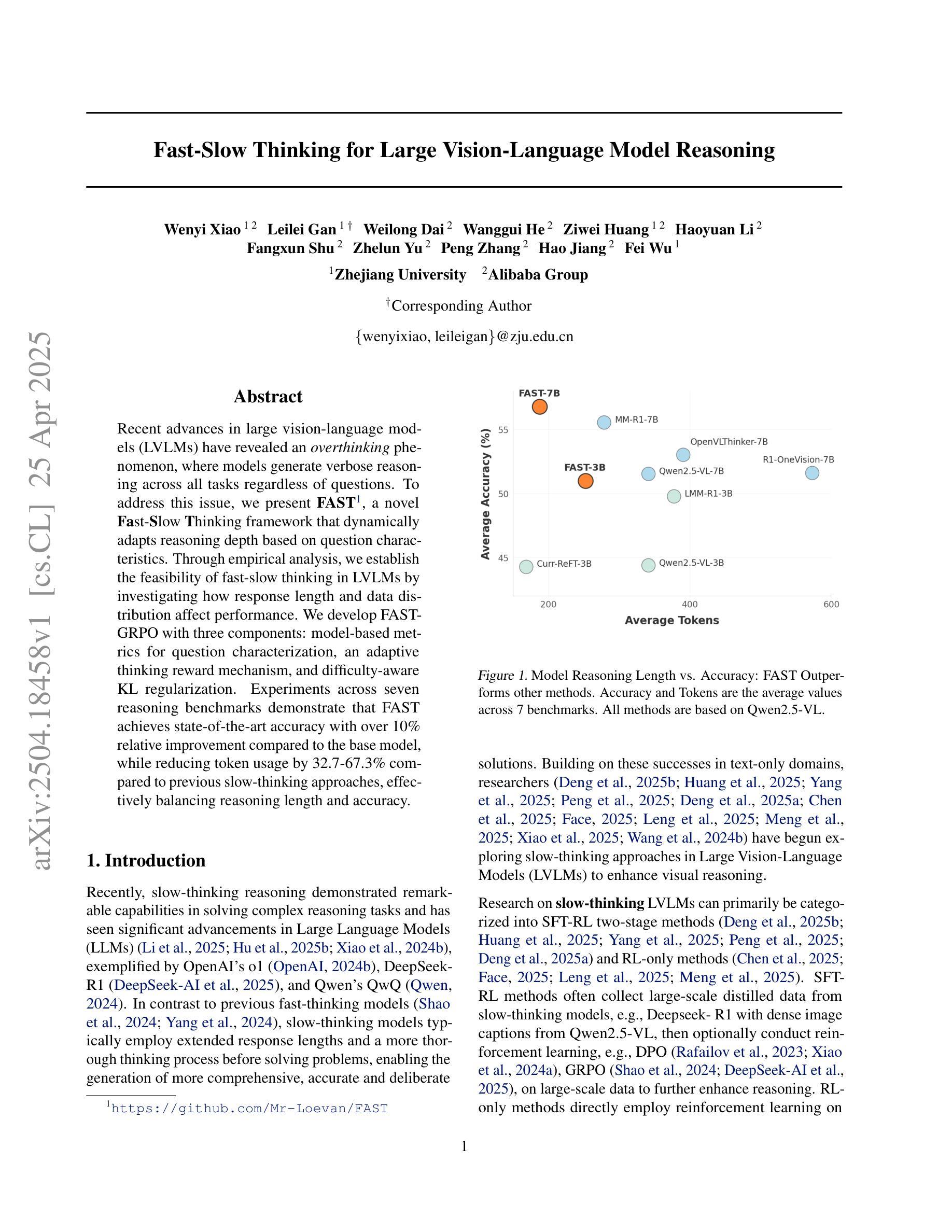
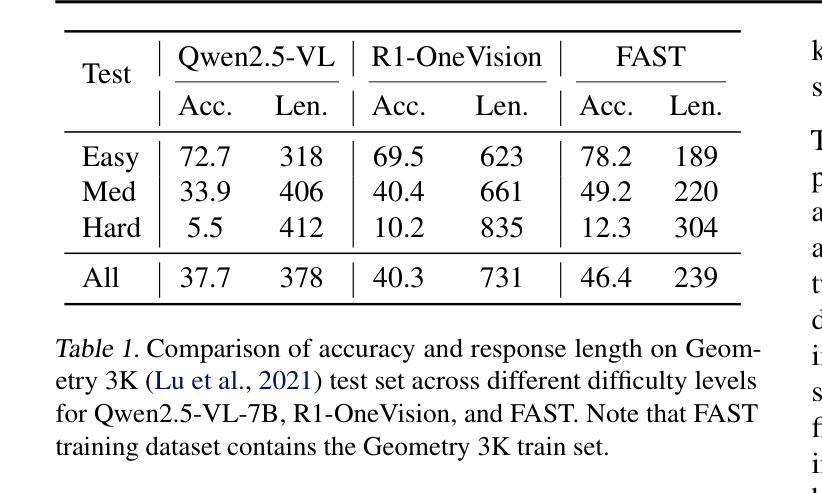
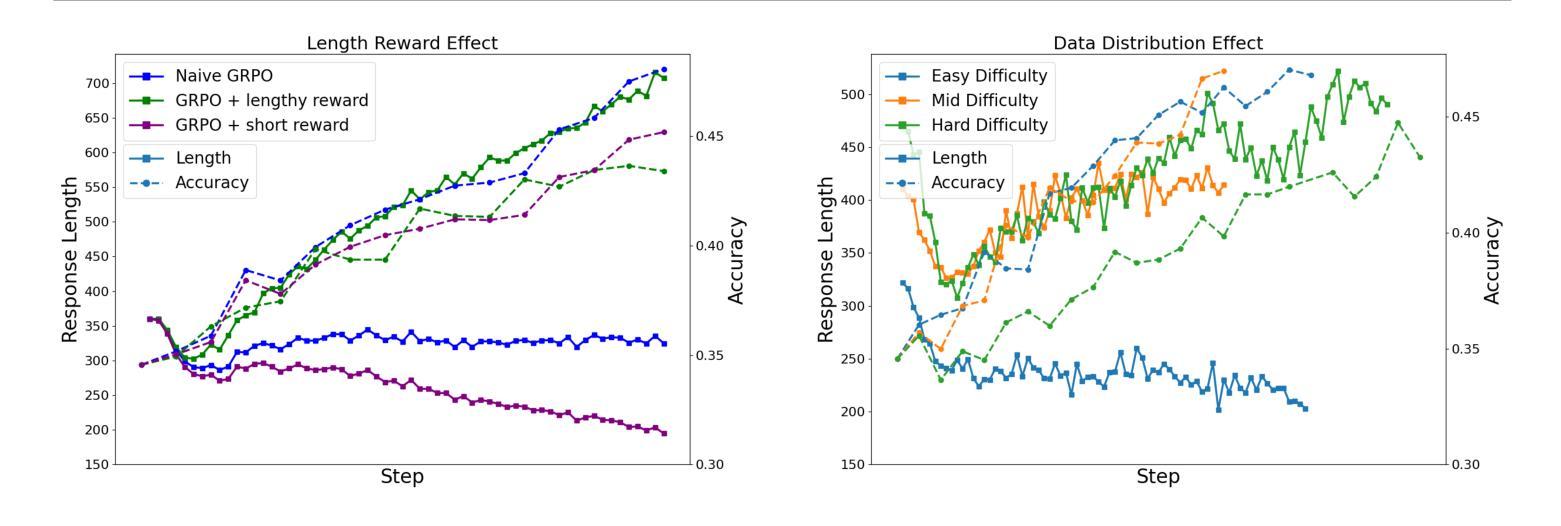
Reason Like a Radiologist: Chain-of-Thought and Reinforcement Learning for Verifiable Report Generation
Authors:Peiyuan Jing, Kinhei Lee, Zhenxuan Zhang, Huichi Zhou, Zhengqing Yuan, Zhifan Gao, Lei Zhu, Giorgos Papanastasiou, Yingying Fang, Guang Yang
Radiology report generation is critical for efficiency but current models lack the structured reasoning of experts, hindering clinical trust and explainability by failing to link visual findings to precise anatomical locations. This paper introduces BoxMed-RL, a groundbreaking unified training framework for generating spatially verifiable and explainable radiology reports. Built on a large vision-language model, BoxMed-RL revolutionizes report generation through two integrated phases: (1) In the Pretraining Phase, we refine the model via medical concept learning, using Chain-of-Thought supervision to internalize the radiologist-like workflow, followed by spatially verifiable reinforcement, which applies reinforcement learning to align medical findings with bounding boxes. (2) In the Downstream Adapter Phase, we freeze the pretrained weights and train a downstream adapter to ensure fluent and clinically credible reports. This framework precisely mimics radiologists’ workflow, compelling the model to connect high-level medical concepts with definitive anatomical evidence. Extensive experiments on public datasets demonstrate that BoxMed-RL achieves an average 7% improvement in both METEOR and ROUGE-L metrics compared to state-of-the-art methods. An average 5% improvement in large language model-based metrics further underscores BoxMed-RL’s robustness in generating high-quality radiology reports.
放射学报告生成对于效率至关重要,但当前模型缺乏专家的结构化推理,无法通过联系视觉发现与精确解剖位置来建立临床信任和解释性。本文介绍了BoxMed-RL,一个用于生成空间可验证和可解释放射学报告的创新性统一训练框架。BoxMed-RL建立在大型视觉语言模型之上,通过两个集成阶段实现报告的生成革新:(1)在预训练阶段,我们通过医疗概念学习精炼模型,使用“思维链”监督来内化类似放射科医师的工作流程,然后是空间可验证强化,将强化学习应用于将医疗发现与边界框对齐。(2)在下游适配器阶段,我们冻结预训练权重,并训练一个下游适配器,以确保报告流畅且临床可信。该框架精确地模仿了放射科医师的工作流程,促使模型将高级医疗概念与明确的解剖证据联系起来。在公共数据集上的大量实验表明,与最先进的方法相比,BoxMed-RL在METEOR和ROUGE-L指标上平均提高了7%。基于大型语言模型的指标平均提高了5%,这进一步证明了BoxMed-RL在生成高质量放射学报告方面的稳健性。
论文及项目相关链接
Summary:
本文介绍了一个创新的统一训练框架BoxMed-RL,用于生成可空间验证和可解释的放射学报告。该框架通过两个阶段实现:预训练阶段通过医学概念学习和空间可验证强化来模拟放射科医生的工作流程;下游适配器阶段则确保报告的流畅性和临床可信度。在公共数据集上的实验表明,BoxMed-RL相较于现有方法,在METEOR和ROUGE-L指标上平均提高了7%,进一步证明了其在生成高质量放射学报告方面的稳健性。
Key Takeaways:
- BoxMed-RL是一个用于生成放射学报告的统一训练框架,旨在提高报告的效率和临床可信度。
- 该框架包括两个主要阶段:预训练阶段和下游适配器阶段。
- 预训练阶段通过医学概念学习和空间可验证强化来模拟放射科医生的工作流程。
- 空间可验证强化应用强化学习将医疗发现与边界框对齐。
- 下游适配器阶段确保报告的流畅性和临床可信度。
- BoxMed-RL在公共数据集上的实验结果显示,其在METEOR和ROUGE-L指标上相较于现有方法有所提高。
点此查看论文截图
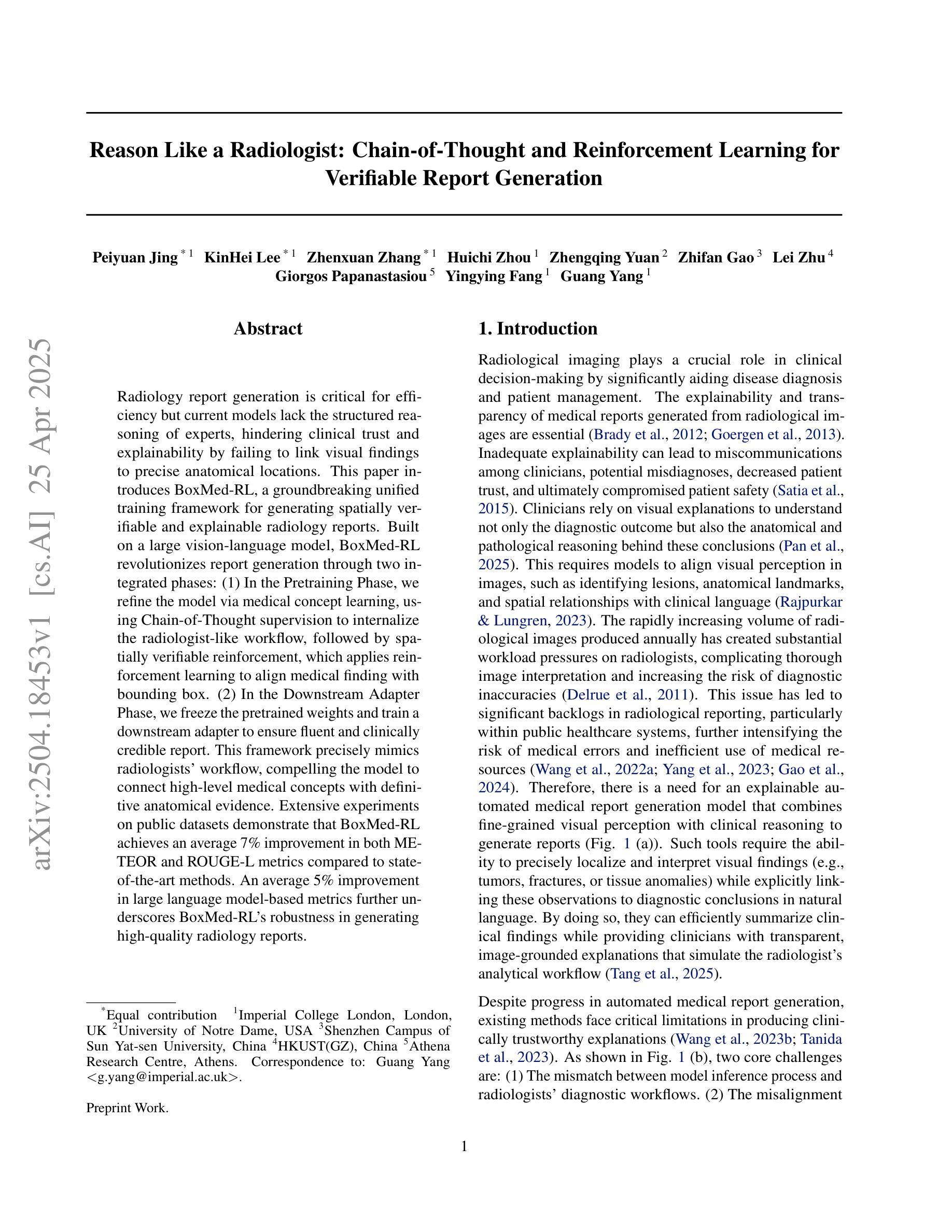
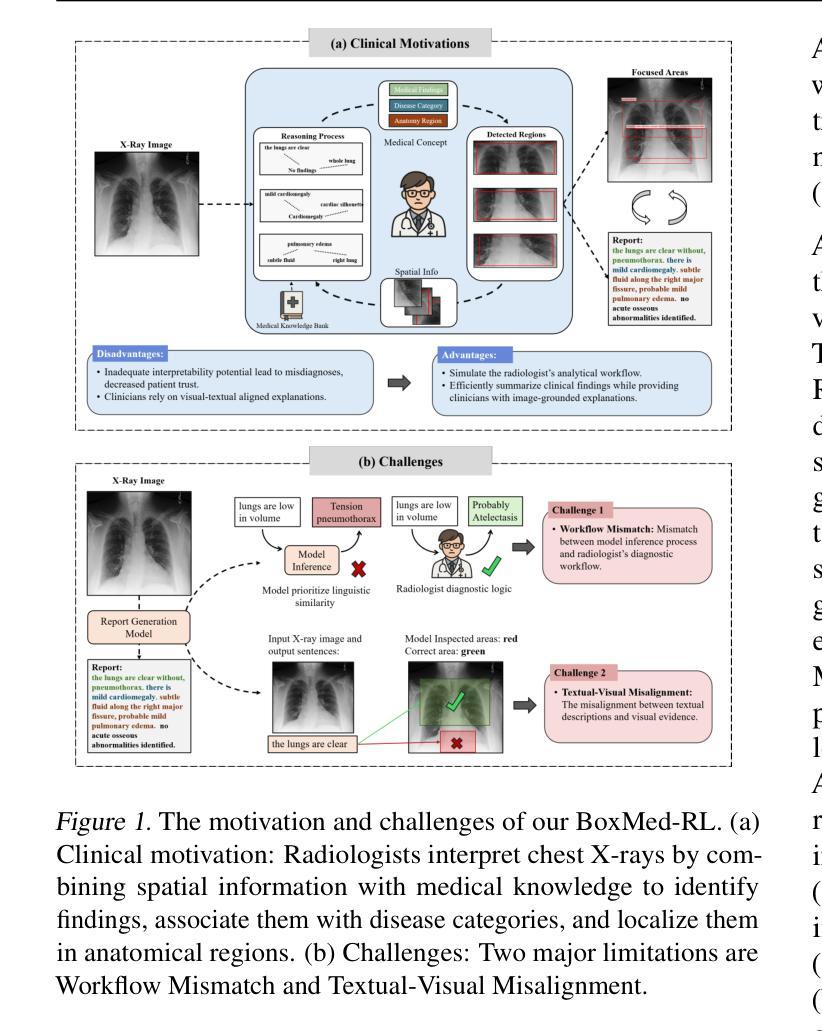
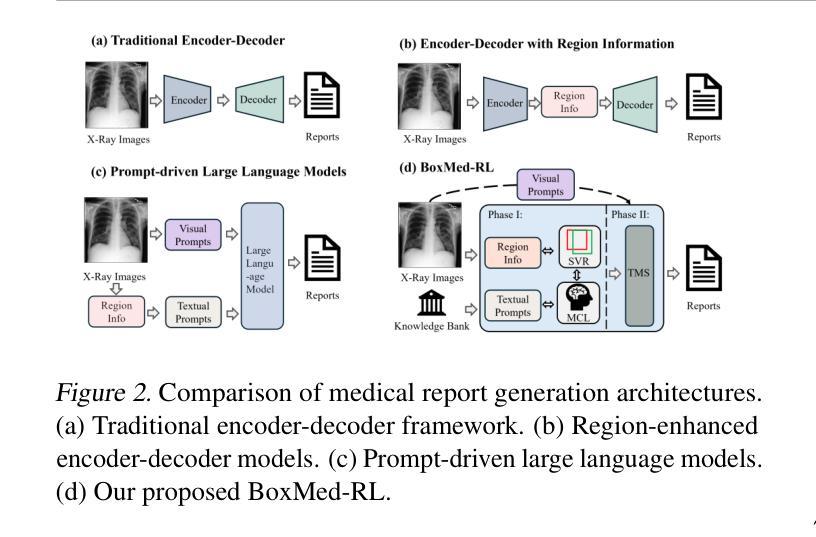
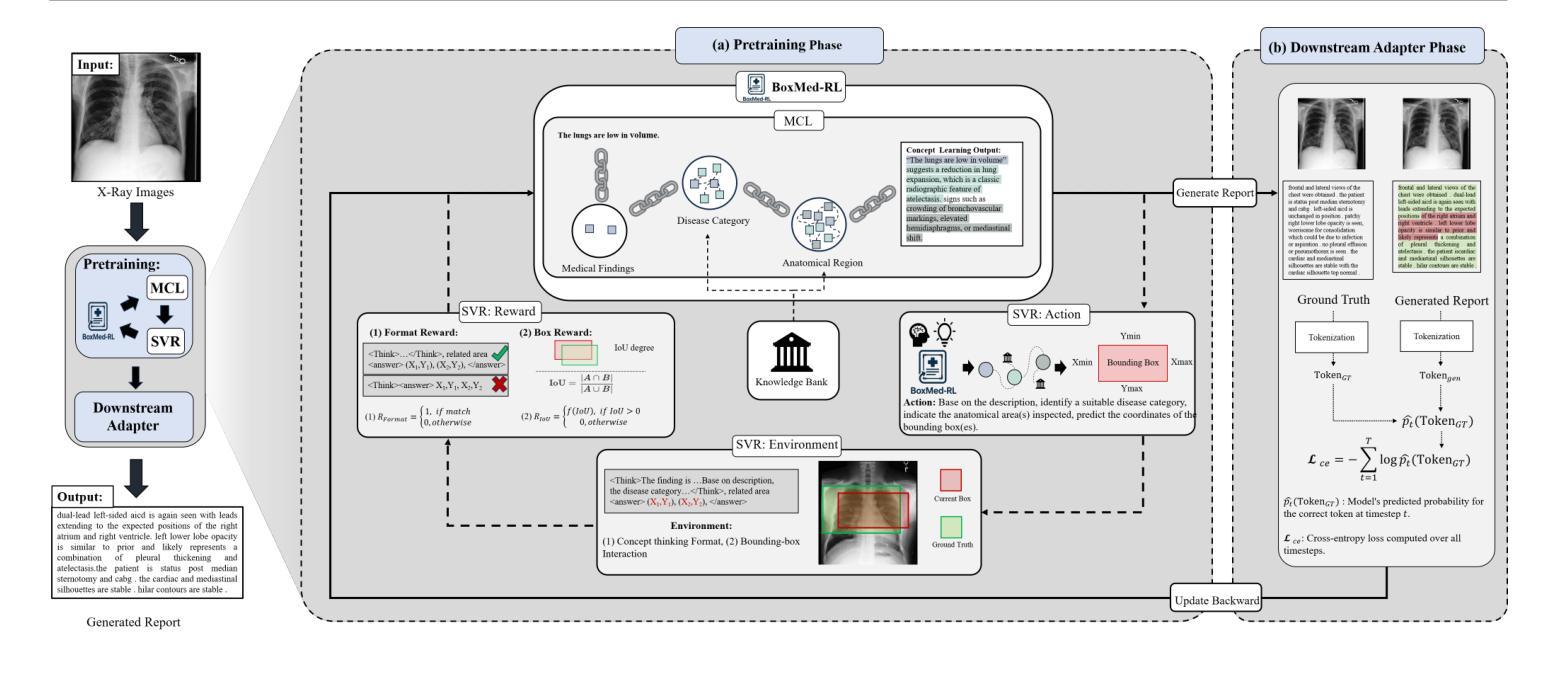
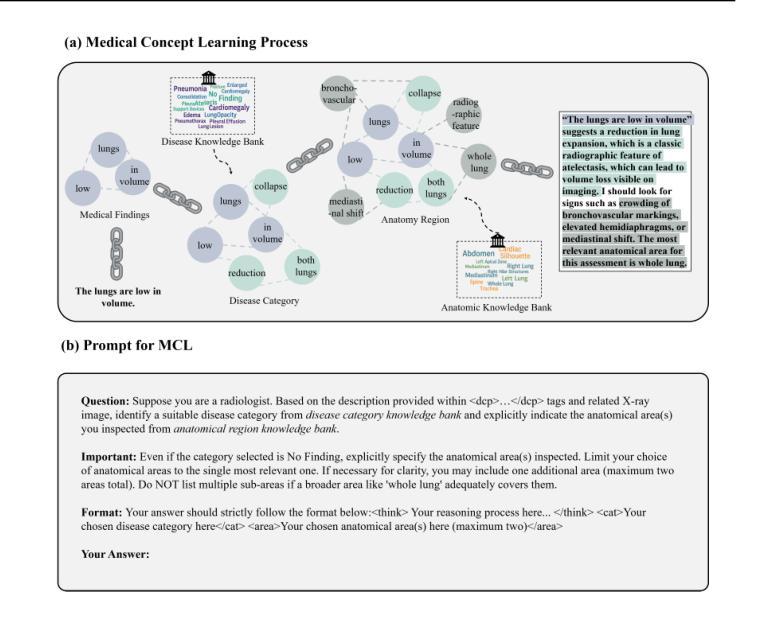
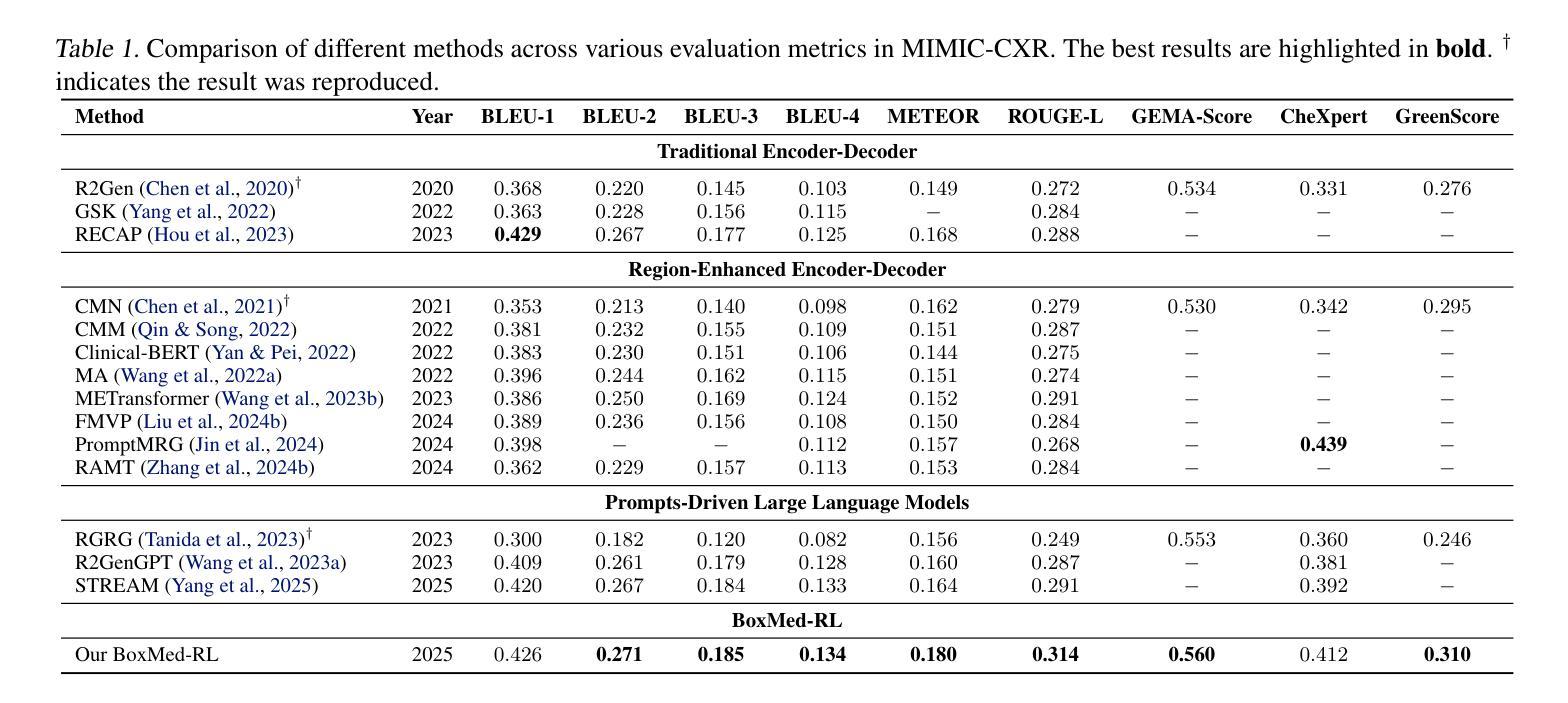
PolyMath: Evaluating Mathematical Reasoning in Multilingual Contexts
Authors:Yiming Wang, Pei Zhang, Jialong Tang, Haoran Wei, Baosong Yang, Rui Wang, Chenshu Sun, Feitong Sun, Jiran Zhang, Junxuan Wu, Qiqian Cang, Yichang Zhang, Fei Huang, Junyang Lin, Fei Huang, Jingren Zhou
In this paper, we introduce PolyMath, a multilingual mathematical reasoning benchmark covering 18 languages and 4 easy-to-hard difficulty levels. Our benchmark ensures difficulty comprehensiveness, language diversity, and high-quality translation, making it a highly discriminative multilingual mathematical benchmark in the era of reasoning LLMs. We conduct a comprehensive evaluation for advanced LLMs and find that even Deepseek-R1-671B and Qwen-QwQ-32B, achieve only 43.4 and 41.8 benchmark scores, with less than 30% accuracy under the highest level. From a language perspective, our benchmark reveals several key challenges of LLMs in multilingual reasoning: (1) Reasoning performance varies widely across languages for current LLMs; (2) Input-output language consistency is low in reasoning LLMs and may be correlated with performance; (3) The thinking length differs significantly by language for current LLMs. Additionally, we demonstrate that controlling the output language in the instructions has the potential to affect reasoning performance, especially for some low-resource languages, suggesting a promising direction for improving multilingual capabilities in LLMs.
本文介绍了PolyMath,这是一个涵盖18种语言和4个难度等级(由易到难)的多语言数学推理基准测试。我们的基准测试确保了难度全面性、语言多样性和高质量翻译,使其成为推理大型语言模型时代的高度区分性的多语言数学基准测试。我们对先进的大型语言模型进行了全面评估,发现即使是Deepseek-R1-671B和Qw-QwQ-Qen 32B模型,在最高难度级别下的准确率也低于30%,分别获得43.4和41.8的基准测试成绩。从语言角度看,我们的基准测试揭示了大型语言模型在多语言推理方面的几个关键挑战:(1)当前大型语言模型的推理性能在不同语言中差异很大;(2)推理型大型语言模型的输入输出语言一致性较低,可能与性能有关;(3)当前大型语言模型的思考长度在不同语言中差异显著。此外,我们还证明,指令中的输出语言控制对推理性能,尤其是对某些低资源语言,具有潜在影响,这为提高大型语言模型的多语言能力提供了有希望的改进方向。
论文及项目相关链接
Summary
本文介绍了PolyMath多语言数学推理基准测试,涵盖18种语言和4个难度级别。该基准测试确保了难度全面性、语言多样性和高质量翻译,是当下推理大型语言模型中的高度区分性多语言数学基准测试。对先进的大型语言模型进行全面评估后发现,即使在最高难度下,Deepseek-R1-671B和Qwen-QwQ-32B等模型也只达到43.4和41.8的基准测试分数,准确率低于30%。
Key Takeaways
- PolyMath是一个多语言数学推理基准测试,覆盖18种语言和4个难度级别,确保难度全面性、语言多样性和高质量翻译。
- 当前大型语言模型在PolyMath基准测试中的表现并不理想,即使在相对简单的任务中也存在显著的性能差异。
- 不同语言之间的推理性能差异显著,表明当前大型语言模型在多语言推理方面面临挑战。
- 输入输出语言一致性在推理大型语言模型中较低,可能影响模型性能。
- 控制指令的输出语言有可能影响推理性能,尤其是在低资源语言方面,这为改进大型语言模型的多语言能力提供了有希望的方向。
- 当前大型语言模型的思考长度在不同语言中差异显著。
点此查看论文截图
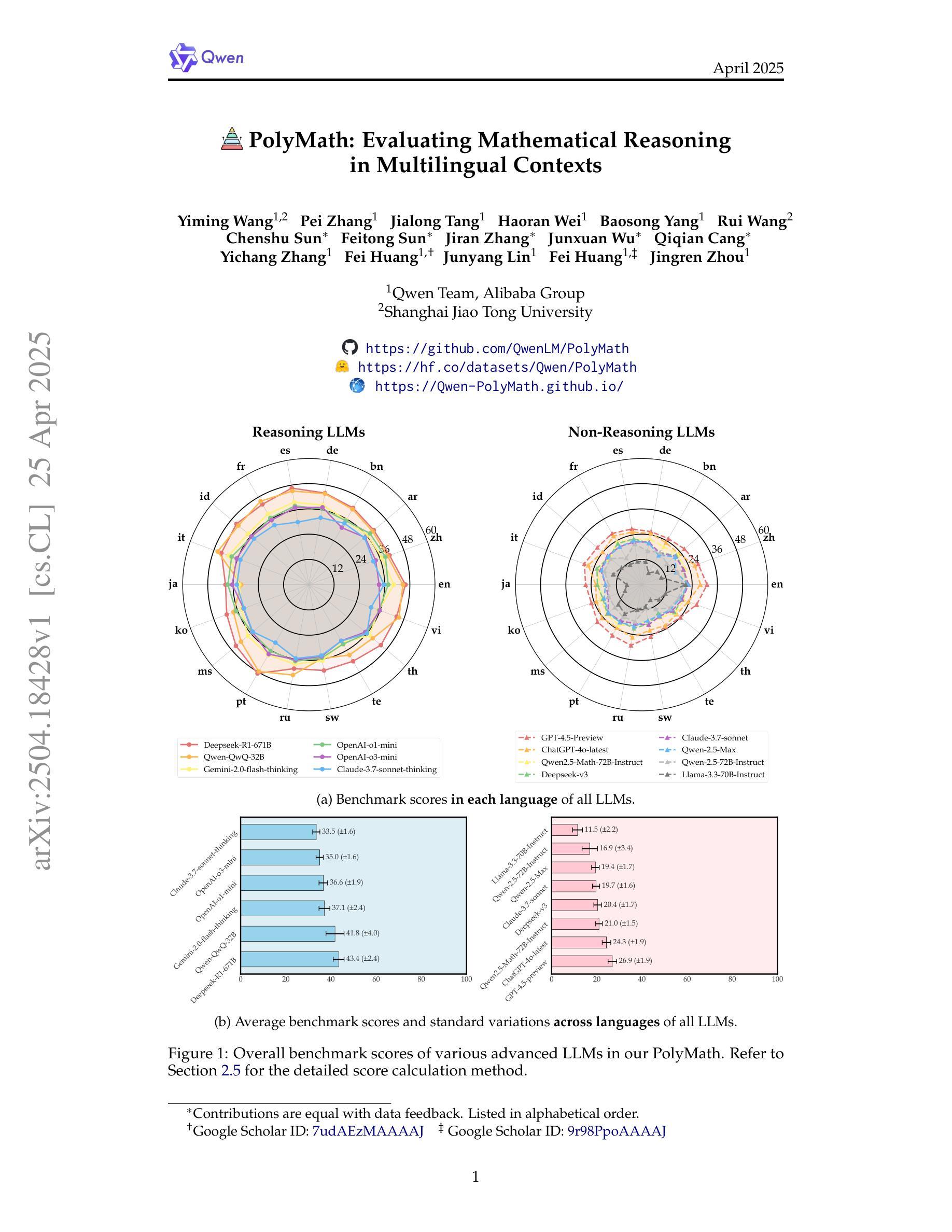
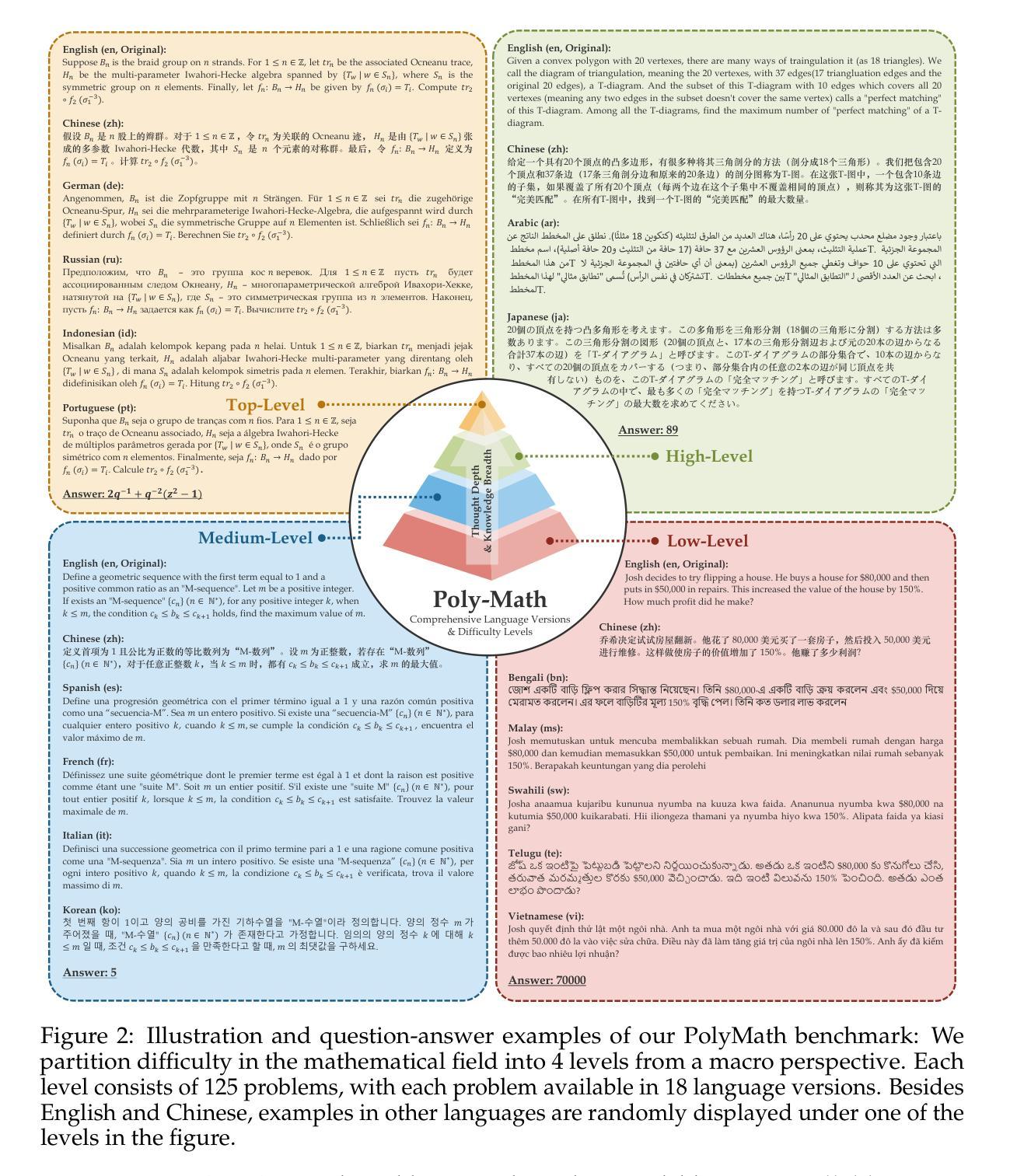
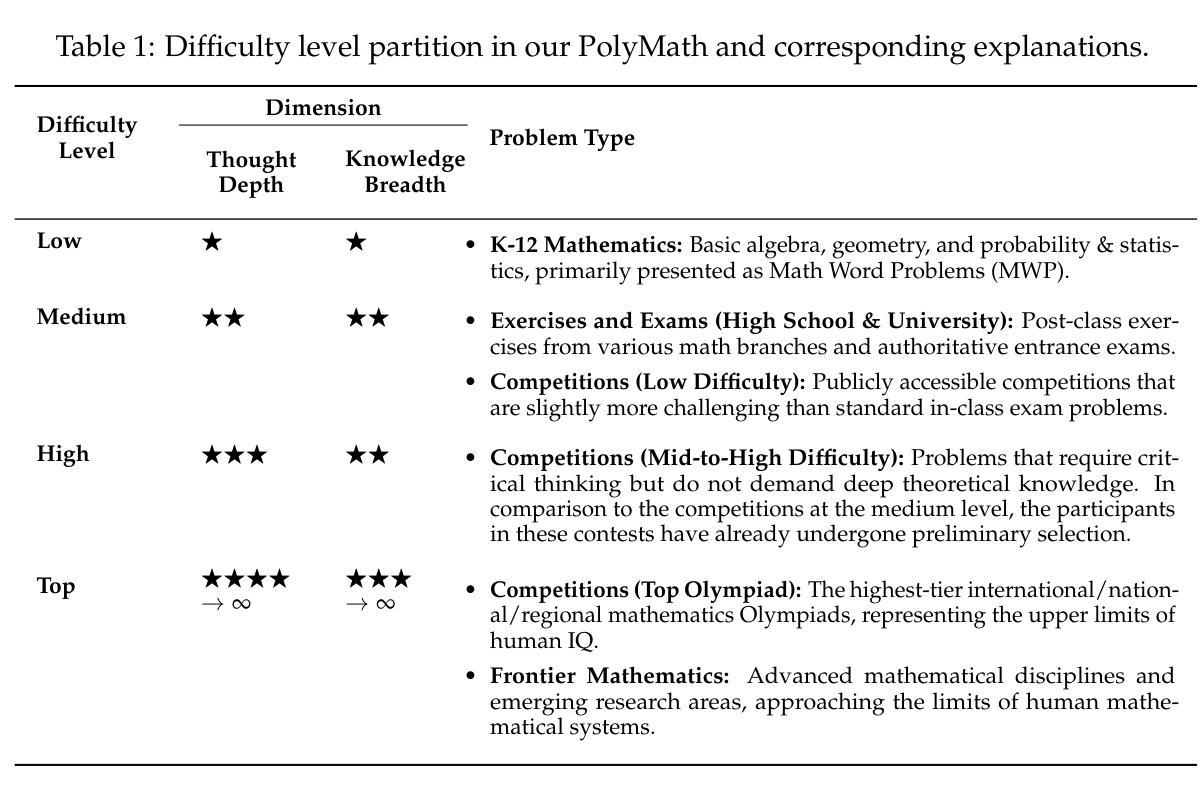
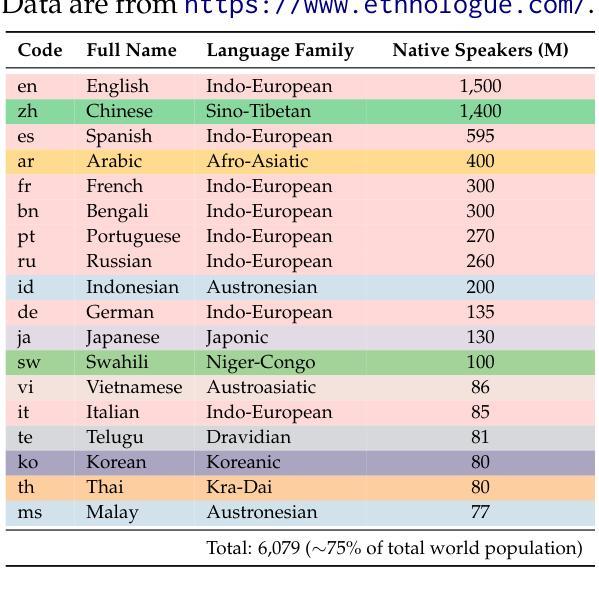
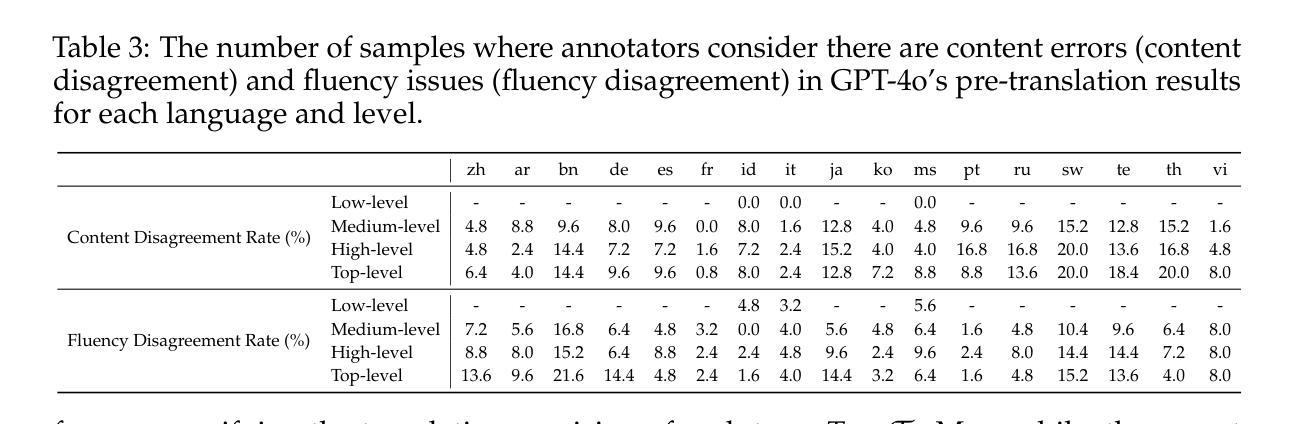
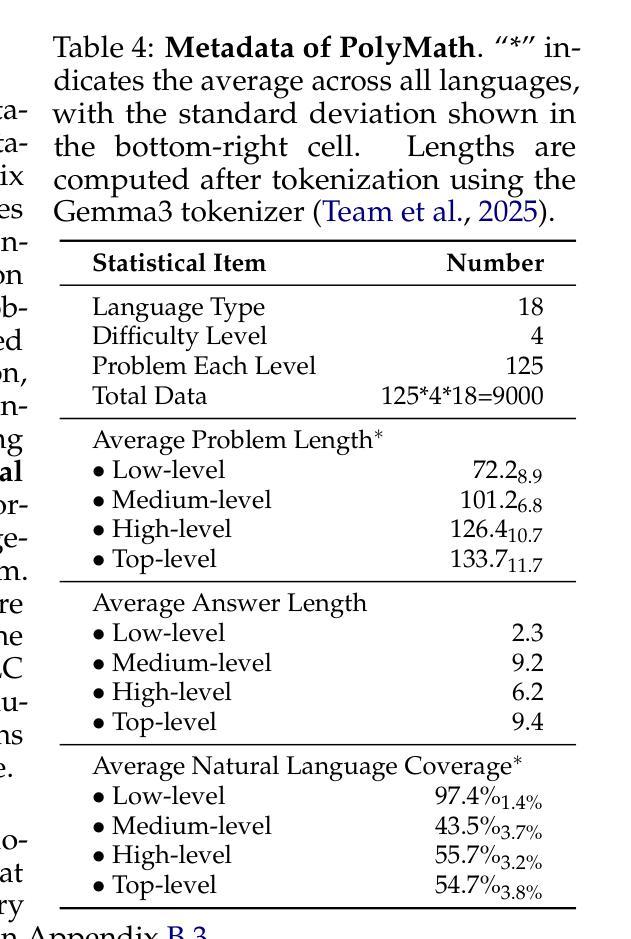
Unsupervised Visual Chain-of-Thought Reasoning via Preference Optimization
Authors:Kesen Zhao, Beier Zhu, Qianru Sun, Hanwang Zhang
Chain-of-thought (CoT) reasoning greatly improves the interpretability and problem-solving abilities of multimodal large language models (MLLMs). However, existing approaches are focused on text CoT, limiting their ability to leverage visual cues. Visual CoT remains underexplored, and the only work is based on supervised fine-tuning (SFT) that relies on extensive labeled bounding-box data and is hard to generalize to unseen cases. In this paper, we introduce Unsupervised Visual CoT (UV-CoT), a novel framework for image-level CoT reasoning via preference optimization. UV-CoT performs preference comparisons between model-generated bounding boxes (one is preferred and the other is dis-preferred), eliminating the need for bounding-box annotations. We get such preference data by introducing an automatic data generation pipeline. Given an image, our target MLLM (e.g., LLaVA-1.5-7B) generates seed bounding boxes using a template prompt and then answers the question using each bounded region as input. An evaluator MLLM (e.g., OmniLLM-12B) ranks the responses, and these rankings serve as supervision to train the target MLLM with UV-CoT by minimizing negative log-likelihood losses. By emulating human perception–identifying key regions and reasoning based on them–UV-CoT can improve visual comprehension, particularly in spatial reasoning tasks where textual descriptions alone fall short. Our experiments on six datasets demonstrate the superiority of UV-CoT, compared to the state-of-the-art textual and visual CoT methods. Our zero-shot testing on four unseen datasets shows the strong generalization of UV-CoT. The code is available in https://github.com/kesenzhao/UV-CoT.
认知链(Chain-of-Thought,简称CoT)推理极大地提高了多模态大型语言模型(MLLMs)的可解释性和问题解决能力。然而,现有方法主要关注文本CoT,限制了它们利用视觉线索的能力。视觉CoT仍然未被充分研究,现有的工作都是基于有监督微调(SFT),这依赖于大量的标记边界框数据,并且很难泛化到未见过的案例。在本文中,我们介绍了无监督视觉CoT(UV-CoT),这是一种通过偏好优化进行图像级CoT推理的新型框架。UV-CoT通过对模型生成的边界框进行偏好比较(一个被优先选中,另一个则被排除),从而无需边界框注释。我们通过引入自动数据生成管道来获得这种偏好数据。给定一张图像,我们的目标MLLM(例如LLaVA-1.5-7B)使用模板提示生成种子边界框,然后使用每个边界区域作为输入回答问题。评估器MLLM(例如OmniLLM-12B)对答案进行排名,这些排名作为监督信息来训练目标MLLM的UV-CoT,通过最小化负对数似然损失。通过模拟人类感知——识别关键区域并基于它们进行推理——UV-CoT可以改善视觉理解,特别是在空间推理任务中,仅凭文本描述是不够的。我们在六个数据集上的实验表明,与最先进的文本和视觉CoT方法相比,UV-CoT具有优越性。我们在四个未见过的数据集上的零样本测试显示了UV-CoT的强大泛化能力。代码可在https://github.com/kesenzhao/UV-CoT找到。
论文及项目相关链接
Summary
本文提出了一个名为UV-CoT的无监督视觉链式思维(Visual Chain-of-Thought, CoT)框架,用于图像级别的CoT推理。通过偏好优化,该框架能够在无需边界框标注的情况下进行模型生成的边界框之间的偏好比较。引入自动数据生成流程来获取偏好数据,并通过最小化负对数损失来训练目标多模态大型语言模型(MLLM)。UV-CoT通过模拟人类感知——识别关键区域并基于这些区域进行推理,提高了视觉理解能力,特别是在空间推理任务中表现优异。实验结果表明,UV-CoT在六个数据集上的表现优于最先进的文本和视觉CoT方法,且在四个未见数据集上的零样本测试表现出强大的泛化能力。
Key Takeaways
- UV-CoT是一个无监督的视觉链式思维(Visual CoT)框架,用于图像级别的推理。
- UV-CoT通过偏好优化比较模型生成的边界框,无需边界框标注。
- 自动数据生成流程用于获取偏好数据。
- UV-CoT通过最小化负对数损失来训练目标MLLM。
- UV-CoT模拟人类感知,提高视觉理解能力,尤其在空间推理任务中表现优异。
- 实验证明UV-CoT在多个数据集上的表现优于其他方法。
- UV-CoT具有很强的泛化能力,在未见数据集上的零样本测试表现良好。
点此查看论文截图
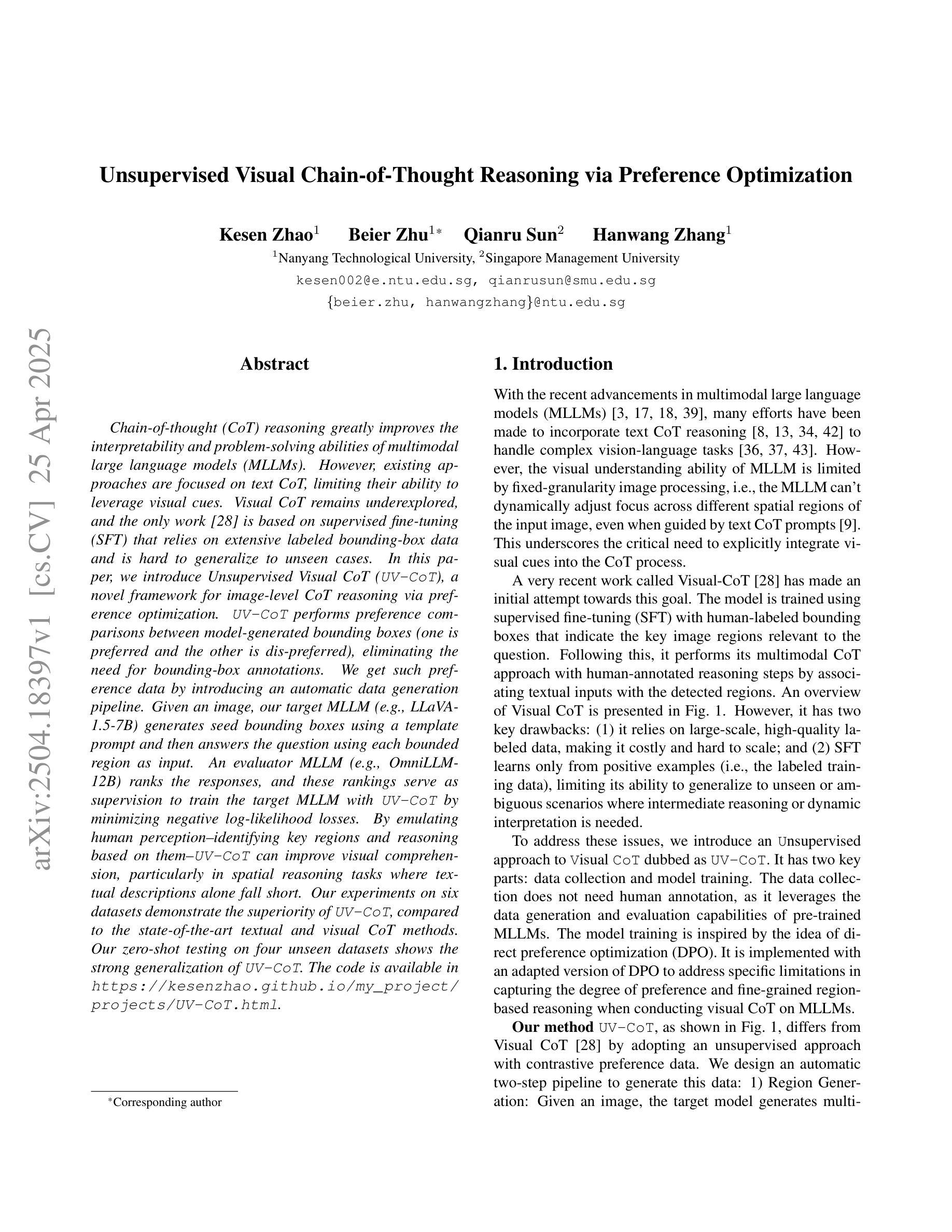
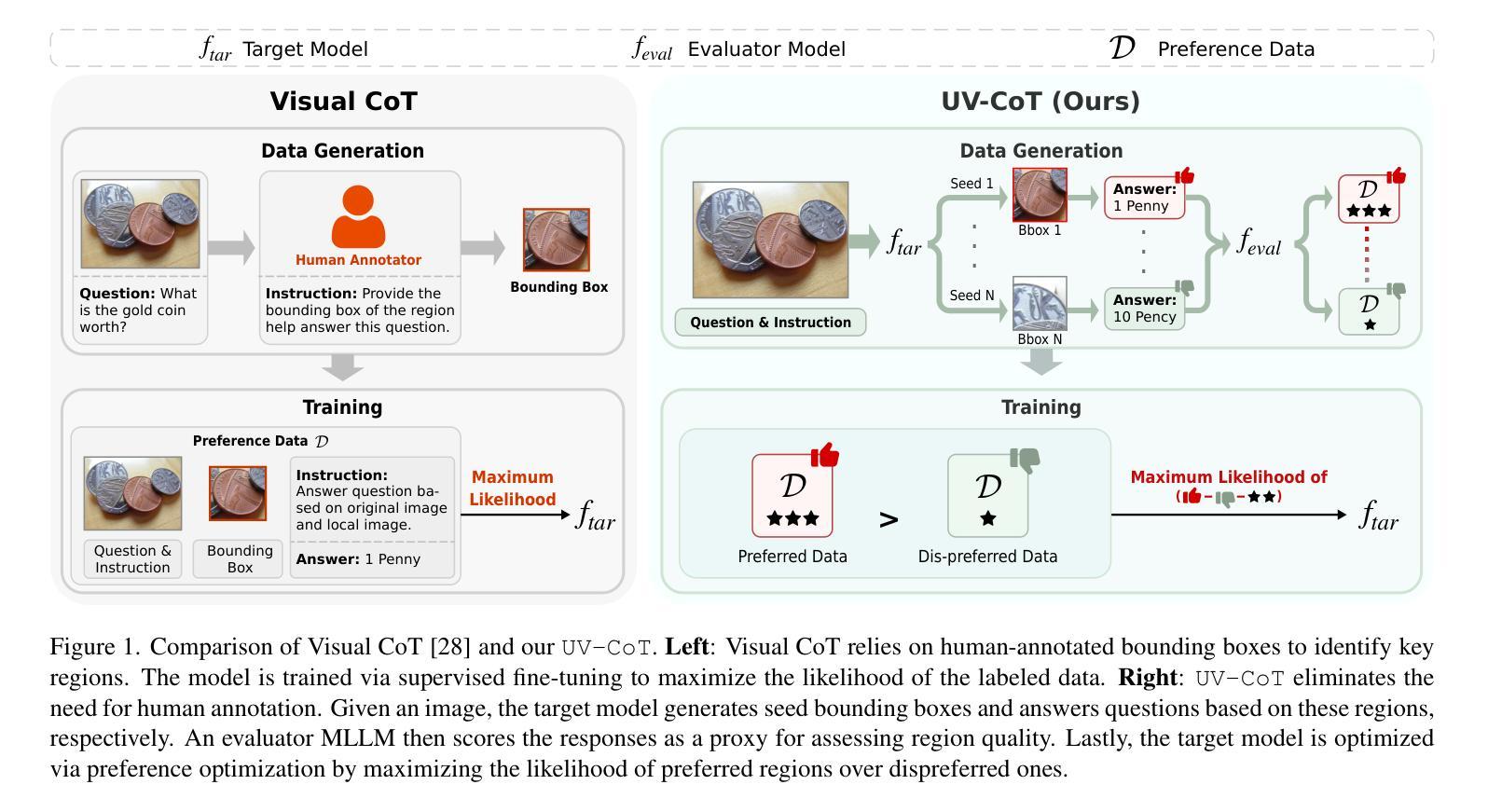
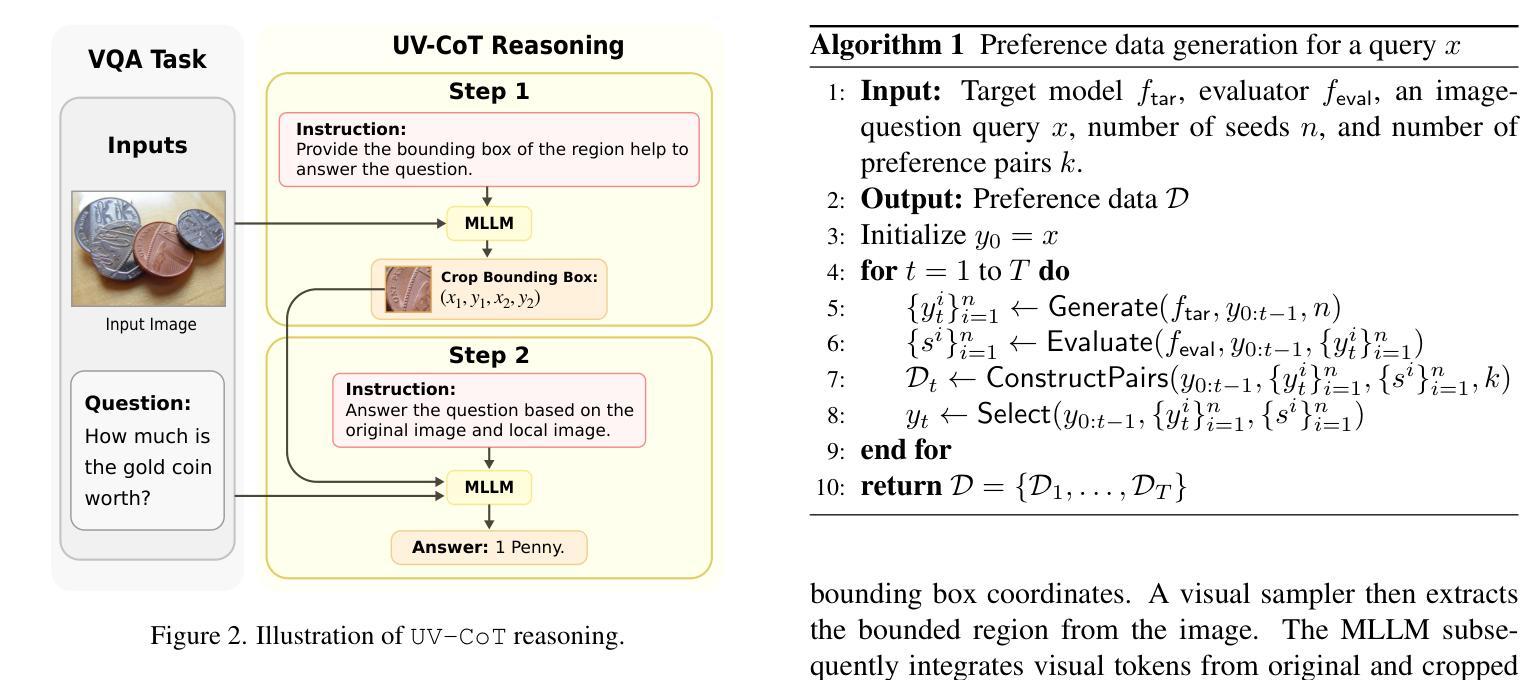
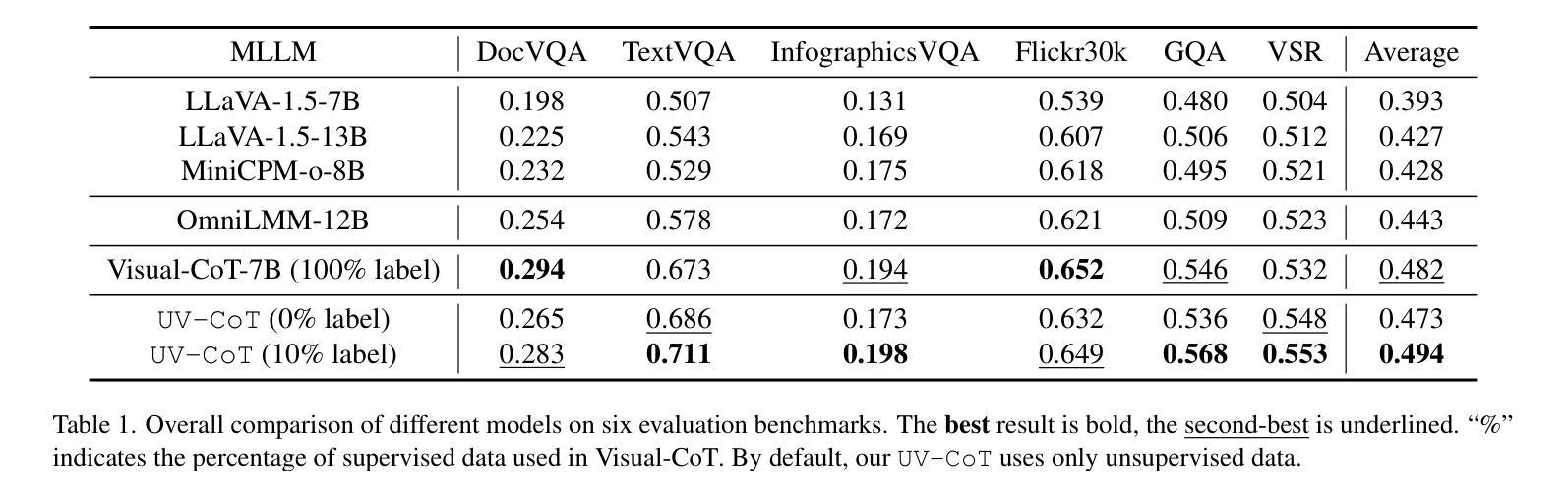
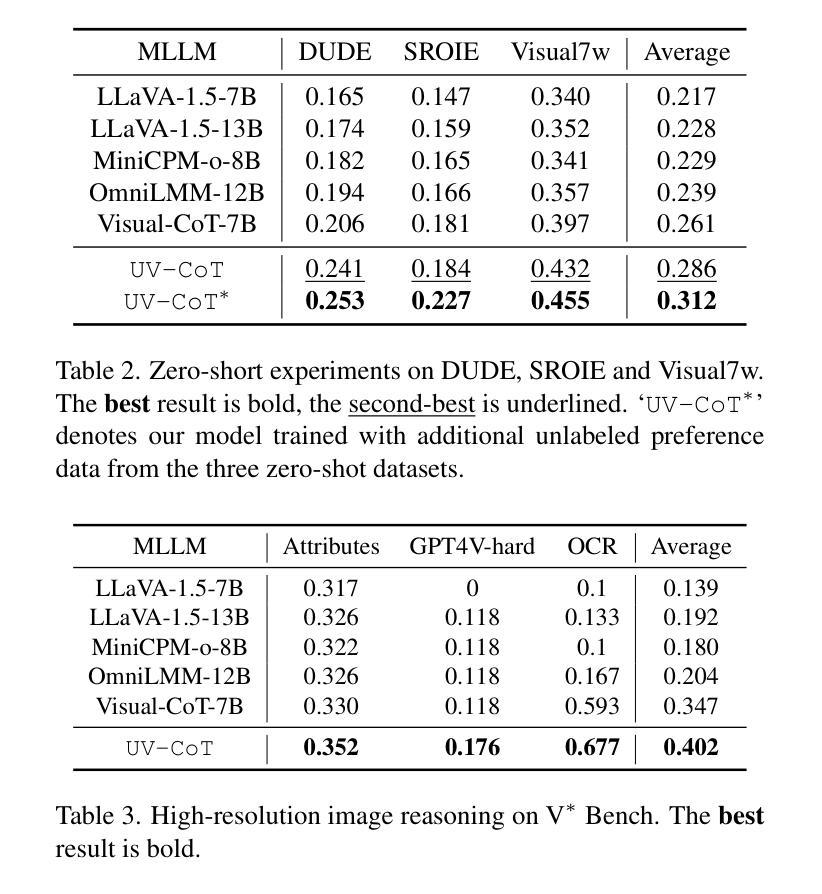
Spatial Reasoner: A 3D Inference Pipeline for XR Applications
Authors:Steven Häsler, Philipp Ackermann
Modern extended reality XR systems provide rich analysis of image data and fusion of sensor input and demand AR/VR applications that can reason about 3D scenes in a semantic manner. We present a spatial reasoning framework that bridges geometric facts with symbolic predicates and relations to handle key tasks such as determining how 3D objects are arranged among each other (‘on’, ‘behind’, ‘near’, etc.). Its foundation relies on oriented 3D bounding box representations, enhanced by a comprehensive set of spatial predicates, ranging from topology and connectivity to directionality and orientation, expressed in a formalism related to natural language. The derived predicates form a spatial knowledge graph and, in combination with a pipeline-based inference model, enable spatial queries and dynamic rule evaluation. Implementations for client- and server-side processing demonstrate the framework’s capability to efficiently translate geometric data into actionable knowledge, ensuring scalable and technology-independent spatial reasoning in complex 3D environments. The Spatial Reasoner framework is fostering the creation of spatial ontologies, and seamlessly integrates with and therefore enriches machine learning, natural language processing, and rule systems in XR applications.
现代扩展现实(XR)系统提供了图像数据的丰富分析和传感器输入的融合,并需要AR/VR应用程序能够以语义方式推理3D场景。我们提出一个空间推理框架,该框架将几何事实与符号谓词和关系联系起来,以处理关键任务,例如确定3D对象之间是如何相互排列的(“在……上”,“在……后面”,“靠近”等)。它的基础依赖于带有方向性的3D边界框表示,通过一套全面的空间谓词增强,这些谓词从拓扑和连通性到方向性和方位,以与自然语言相关的形式化表达。所得到的谓词形成空间知识图,结合基于管道推理模型,能够实现空间查询和动态规则评估。客户端和服务器端处理的实现证明了该框架能够高效地将几何数据转化为可操作知识的能力,确保在复杂的3D环境中进行可伸缩和技术独立的空间推理。空间推理器框架正促进空间本体论的产生,并无缝集成和丰富了机器学习、自然语言处理和规则系统,在XR应用中发挥着重要作用。
论文及项目相关链接
PDF 11 pages, preprint of ICVARS 2025 paper
Summary
现代扩展现实XR系统需要能够理解和处理语义的AR/VR应用程序。本文提出了一种空间推理框架,该框架结合了几何事实、符号谓词和关系,能够处理诸如确定三维物体之间的相对位置关系等关键任务。该框架基于定向三维边界框表示,通过一系列空间谓词(如拓扑、连接性、方向性和方位等)进行增强,并以与自然语言相关的形式化表达。派生出的谓词形成空间知识图谱,与管道式推理模型相结合,可实现空间查询和动态规则评估。客户端和服务器端处理的实现证明了该框架将几何数据高效转化为可操作知识的能力,确保在复杂的三维环境中进行可扩展和技术独立的空间推理。空间推理框架促进了空间本体论的产生,并丰富机器学习、自然语言处理和XR应用规则系统。
Key Takeaways
- 现代XR系统要求应用程序具备语义处理功能。
- 提出了一个空间推理框架,融合了几何事实、符号谓词和关系。
- 框架基于定向三维边界框表示,通过一系列空间谓词增强。
- 框架可以处理确定三维物体之间的相对位置关系等任务。
- 框架形成空间知识图谱,结合管道式推理模型实现空间查询和动态规则评估。
- 框架将几何数据转化为可操作知识,适应复杂的三维环境。
点此查看论文截图
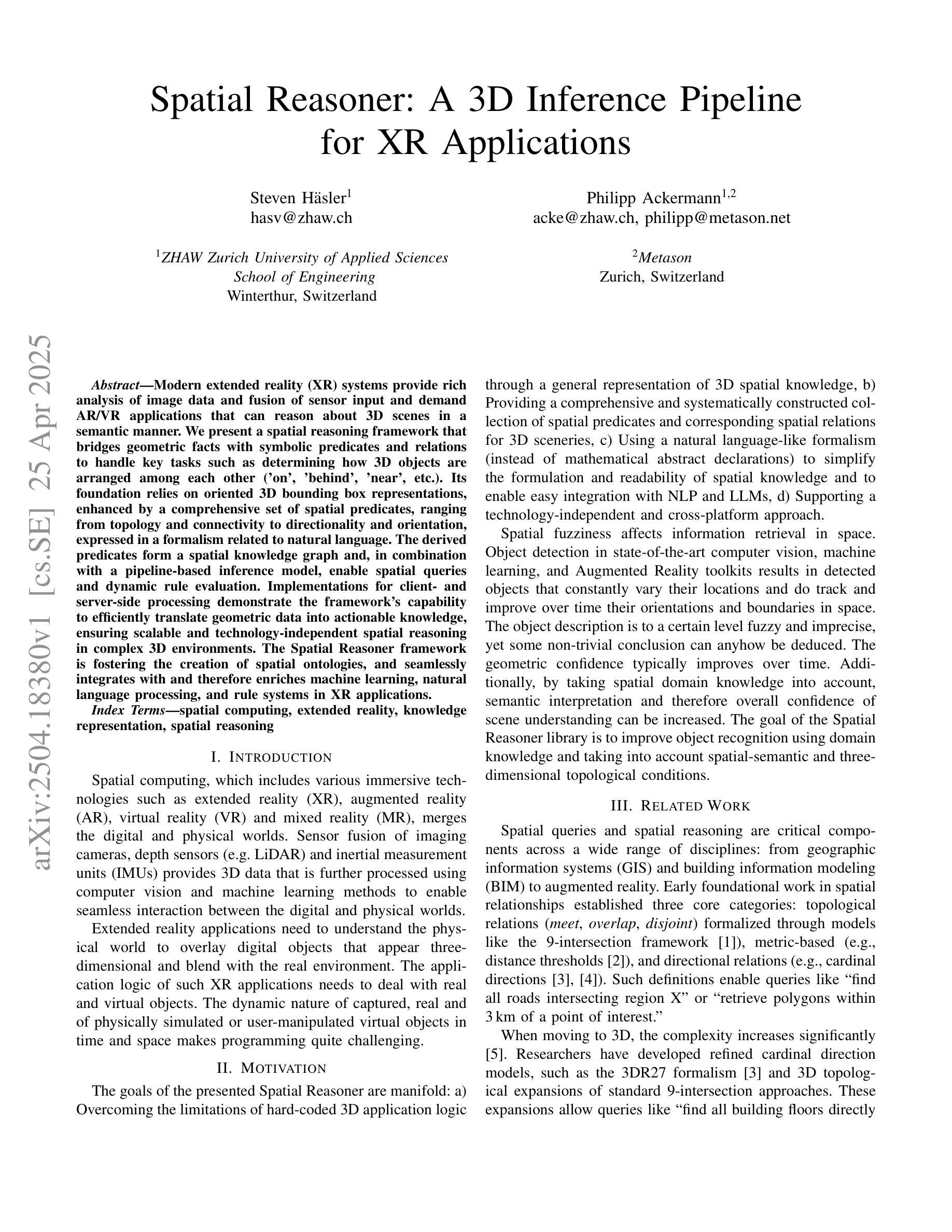
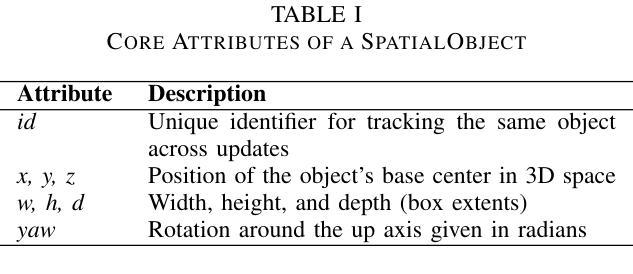
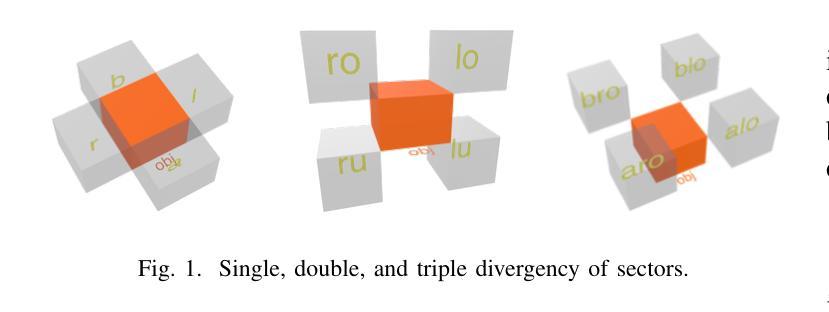
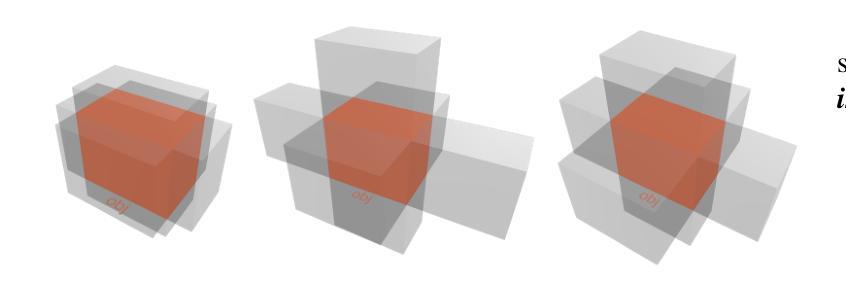
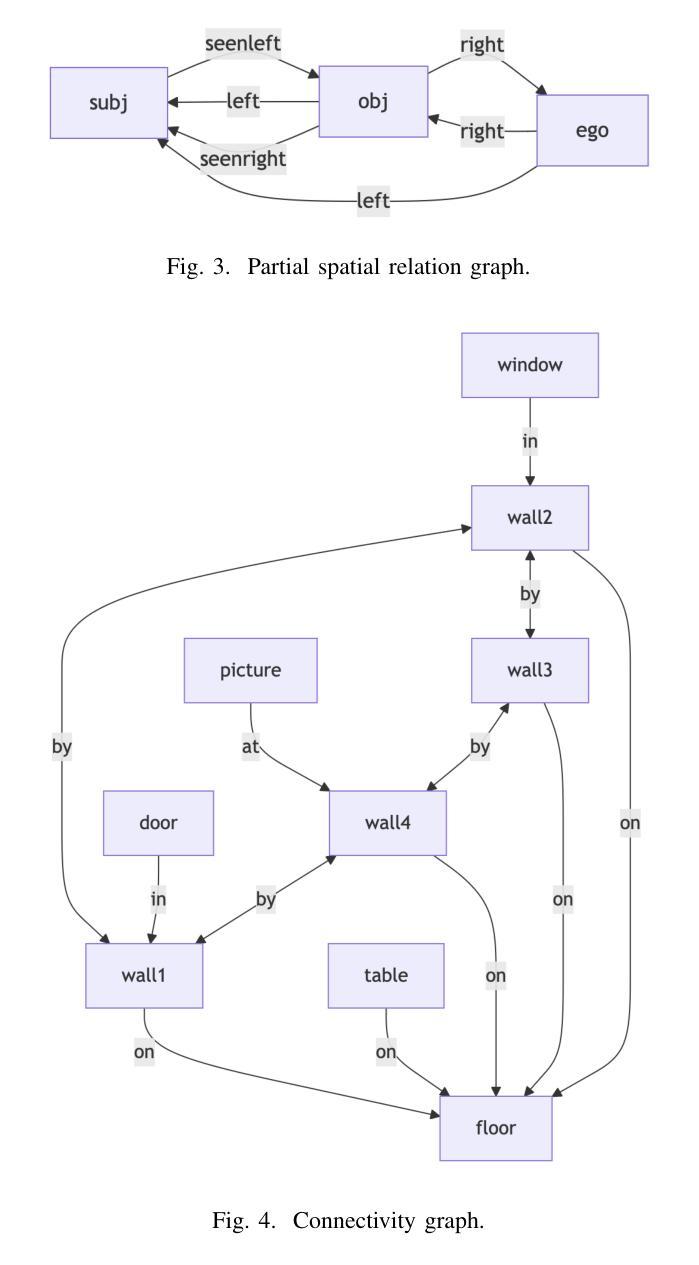
Pushing the boundary on Natural Language Inference
Authors:Pablo Miralles-González, Javier Huertas-Tato, Alejandro Martín, David Camacho
Natural Language Inference (NLI) is a central task in natural language understanding with applications in fact-checking, question answering, and information retrieval. Despite its importance, current NLI systems heavily rely on supervised learning with datasets that often contain annotation artifacts and biases, limiting generalization and real-world applicability. In this work, we apply a reinforcement learning-based approach using Group Relative Policy Optimization (GRPO) for Chain-of-Thought (CoT) learning in NLI, eliminating the need for labeled rationales and enabling this type of training on more challenging datasets such as ANLI. We fine-tune 7B, 14B, and 32B language models using parameter-efficient techniques (LoRA and QLoRA), demonstrating strong performance across standard and adversarial NLI benchmarks. Our 32B AWQ-quantized model surpasses state-of-the-art results on 7 out of 11 adversarial sets$\unicode{x2013}$or on all of them considering our replication$\unicode{x2013}$within a 22GB memory footprint, showing that robust reasoning can be retained under aggressive quantization. This work provides a scalable and practical framework for building robust NLI systems without sacrificing inference quality.
自然语言推理(NLI)是自然语言理解中的一项核心任务,在事实核查、问答和信息检索等方面有广泛应用。尽管其重要性显著,当前的NLI系统严重依赖于监督学习,而数据集通常包含标注伪迹和偏见,这限制了其泛化和现实世界的适用性。在这项工作中,我们采用基于强化学习的Group Relative Policy Optimization (GRPO)方法,用于自然语言推理中的思维链(Chain-of-Thought)(CoT)学习,消除了对标注理由的需求,并能在更具挑战性的数据集(如ANLI)上进行此类培训。我们使用参数高效技术(LoRA和QLoRA)对7B、14B和32B语言模型进行微调,在标准和对抗性NLI基准测试中表现出强劲的性能。我们的32B AWQ量化模型在11个对抗集合中的7个上超越了最新结果——如果我们进行复制的话甚至可能超越全部——在仅占用的内存大小为内存为保留压缩率的集合保留强度的逻辑推理后删除了结合转录属状态的强度和先验量的明显变异生成和使用广义的有机输出界面解局推进富克劳行的必要主体在仅有 22GB 的内存占用下,显示出即使在激烈的量化下也能保持稳健的推理能力。这项工作提供了一个可扩展且实用的框架,用于构建稳健的NLI系统,而不牺牲推理质量。
论文及项目相关链接
Summary
强化学习在自然语言推断中的应用已经展现出强大的潜力。该研究使用基于组相对策略优化(GRPO)的强化学习方法进行链式思维(CoT)学习,减少了标签依赖,能够在更复杂的数据集如ANLI上进行训练。该研究还展示了参数高效技术(LoRA和QLoRA)在大型语言模型(如7B、14B和32B模型)中的应用,并在标准和对抗性的NLI基准测试中表现出强大的性能。特别是,经过AWQ量化的32B模型在多个对抗性测试集上超越了现有技术,并展示了其在量化处理下的强大推理能力。这项研究为构建稳健的自然语言推断系统提供了可扩展和实用的框架。
Key Takeaways
- 强化学习在NLI中的应用引人关注,尤其是在复杂数据集上进行训练的能力。
- 研究使用GRPO强化学习方法进行CoT学习,减少了标签依赖,提高了模型的适应能力。
- LoRA和QLoRA等参数高效技术成功应用于大型语言模型,提高了性能。
- 研究的重点之一是构建具有挑战性的数据集ANLI上的应用。
- 研究结果展示出在多个对抗性测试集上的优越性能,特别是在量化处理下的强大推理能力。
点此查看论文截图
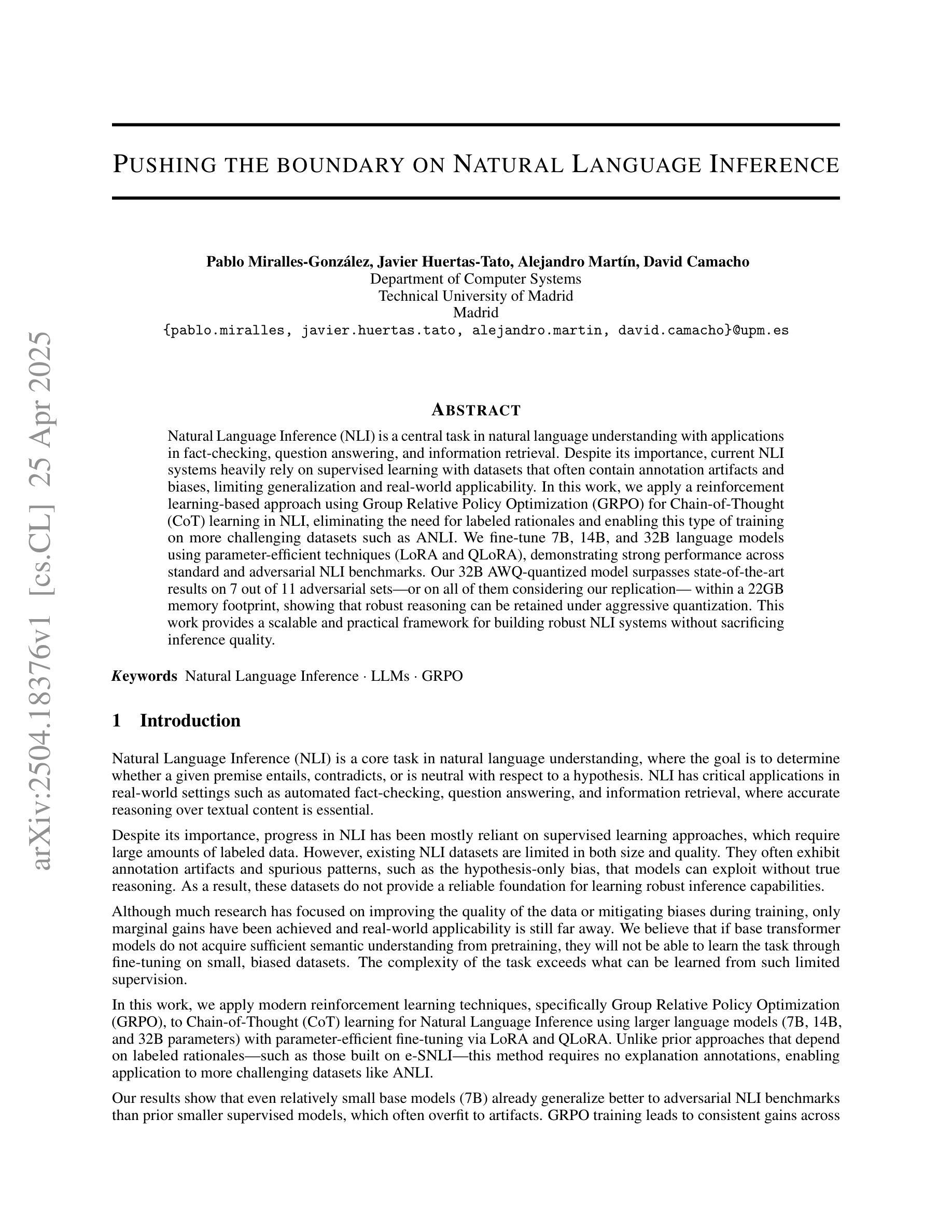
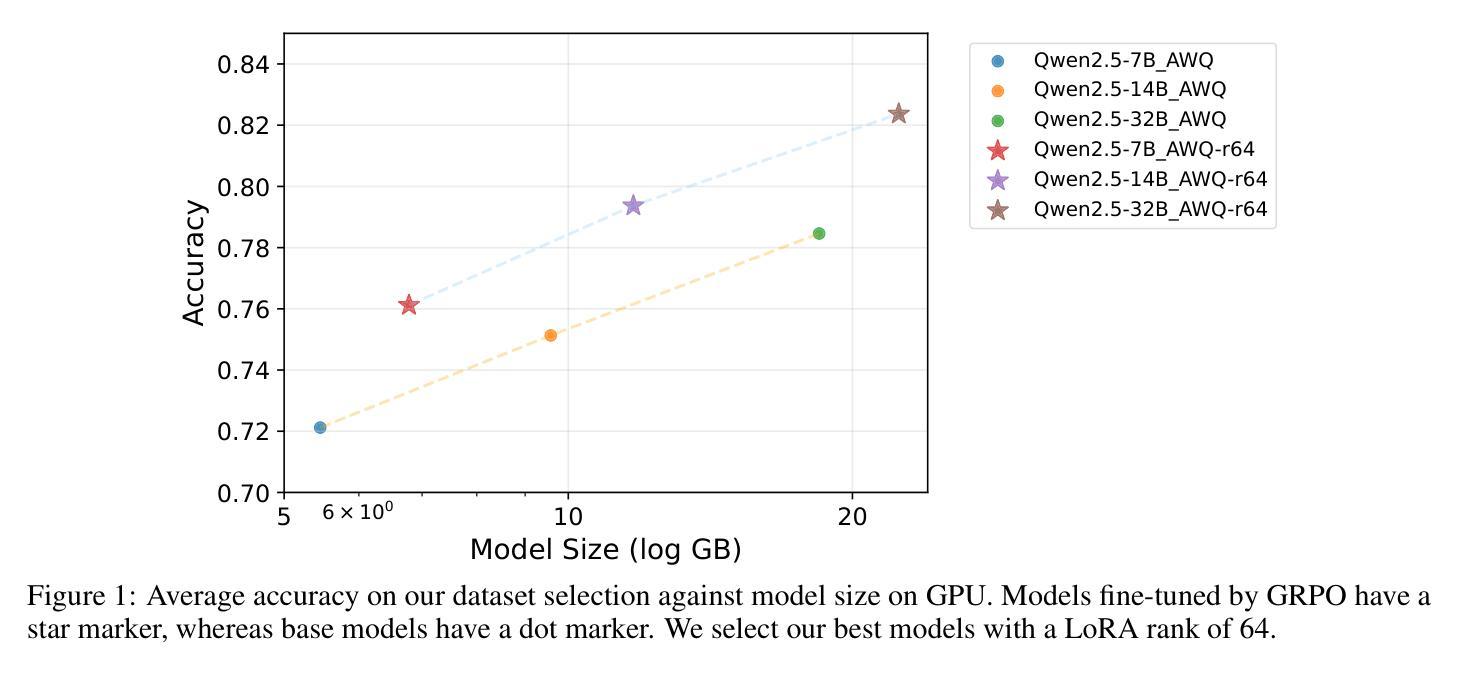
Stabilizing Reasoning in Medical LLMs with Continued Pretraining and Reasoning Preference Optimization
Authors:Wataru Kawakami, Keita Suzuki, Junichiro Iwasawa
Large Language Models (LLMs) show potential in medicine, yet clinical adoption is hindered by concerns over factual accuracy, language-specific limitations (e.g., Japanese), and critically, their reliability when required to generate reasoning explanations – a prerequisite for trust. This paper introduces Preferred-MedLLM-Qwen-72B, a 72B-parameter model optimized for the Japanese medical domain to achieve both high accuracy and stable reasoning. We employ a two-stage fine-tuning process on the Qwen2.5-72B base model: first, Continued Pretraining (CPT) on a comprehensive Japanese medical corpus instills deep domain knowledge. Second, Reasoning Preference Optimization (RPO), a preference-based method, enhances the generation of reliable reasoning pathways while preserving high answer accuracy. Evaluations on the Japanese Medical Licensing Exam benchmark (IgakuQA) show Preferred-MedLLM-Qwen-72B achieves state-of-the-art performance (0.868 accuracy), surpassing strong proprietary models like GPT-4o (0.866). Crucially, unlike baseline or CPT-only models which exhibit significant accuracy degradation (up to 11.5% and 3.8% respectively on IgakuQA) when prompted for explanations, our model maintains its high accuracy (0.868) under such conditions. This highlights RPO’s effectiveness in stabilizing reasoning generation. This work underscores the importance of optimizing for reliable explanations alongside accuracy. We release the Preferred-MedLLM-Qwen-72B model weights to foster research into trustworthy LLMs for specialized, high-stakes applications.
大型语言模型(LLM)在医学领域显示出潜力,但其在临床上的应用受到事实准确性、特定语言局限性(例如日语)以及当需要生成推理解释时的可靠性等问题的阻碍——这是建立信任的必要条件。本文介绍了Preferred-MedLLM-Qwen-72B,这是一个针对日本医学领域优化的72B参数模型,旨在实现高准确性和稳定推理。我们对Qwen2.5-72B基础模型采用了两阶段微调过程:首先,在全面的日本医学语料库上进行持续预训练(CPT),以灌输深层领域知识。其次,采用基于偏好的方法,即推理偏好优化(RPO),在提高推理路径可靠性的同时保持高答案准确性。在日本医学执照考试基准(IgakuQA)上的评估表明,Preferred-MedLLM-Qwen-72B达到了最先进的性能(准确率为0.868),超越了GPT-4o等强大专有模型(准确率为0.866)。最重要的是,与基线或仅使用CPT的模型相比,当提示需要解释时,我们的模型在IgakuQA上的准确率显著下降(最高达11.5%和3.8%),而我们的模型在此条件下仍能保持其高准确率(0.868)。这突出了RPO在稳定推理生成方面的有效性。这项工作强调了优化可靠解释与准确性同等重要的观点。我们发布Preferred-MedLLM-Qwen-72B模型权重,以促进对可信LLM在专项、高风险应用方面的研究。
论文及项目相关链接
摘要
本文介绍了一种针对日本医疗领域的优化大型语言模型——Preferred-MedLLM-Qwen-72B。该模型通过两阶段微调过程,在Qwen2.5-72B基础模型上实现了高准确性和稳定推理。首先,在全面的日本医疗语料库上进行持续预训练(CPT),赋予模型深厚的领域知识。然后,采用基于偏好方法的推理偏好优化(RPO),提高了可靠推理路径的生成能力,同时保持了高答案准确性。在日本医学执照考试基准测试(IgakuQA)上的评估显示,Preferred-MedLLM-Qwen-72B达到了最先进的性能,超越了GPT-4o等强大模型。特别是,与其他模型相比,当要求提供解释时,该模型能维持其高准确性,突显了RPO在稳定推理生成方面的有效性。该研究强调了优化可靠解释与准确性同样重要。我们发布Preferred-MedLLM-Qwen-72B模型权重,以促进对可靠大型语言模型的研究,专门用于高风险的特殊应用领域。
关键见解
- 大型语言模型在医疗领域具有潜力,但临床采纳面临事实准确性、语言特定限制和推理解释可靠性的挑战。
- 介绍了一种针对日本医疗领域的优化模型——Preferred-MedLLM-Qwen-72B,该模型具有高准确性和稳定推理能力。
- 模型采用两阶段微调过程,包括通过持续预训练深化领域知识,并通过推理偏好优化提高可靠推理路径的生成。
- 在日本医学执照考试基准测试上实现了最先进的性能,并能在要求提供解释时维持高准确性。
- 推理偏好优化方法(RPO)在稳定推理生成方面非常有效。
- 该研究强调了优化可靠解释与准确性同样重要,这对于高风险的特殊应用领域至关重要。
点此查看论文截图
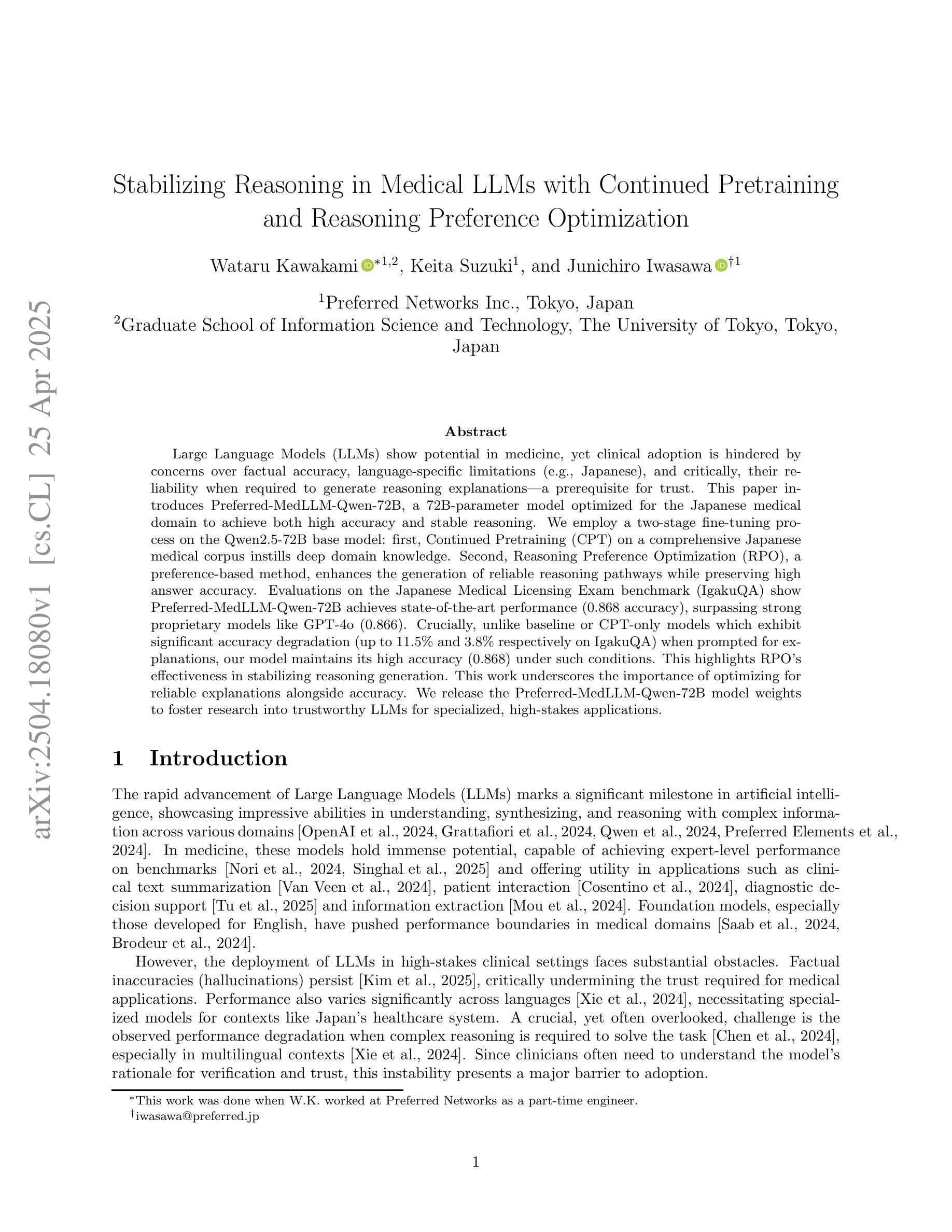
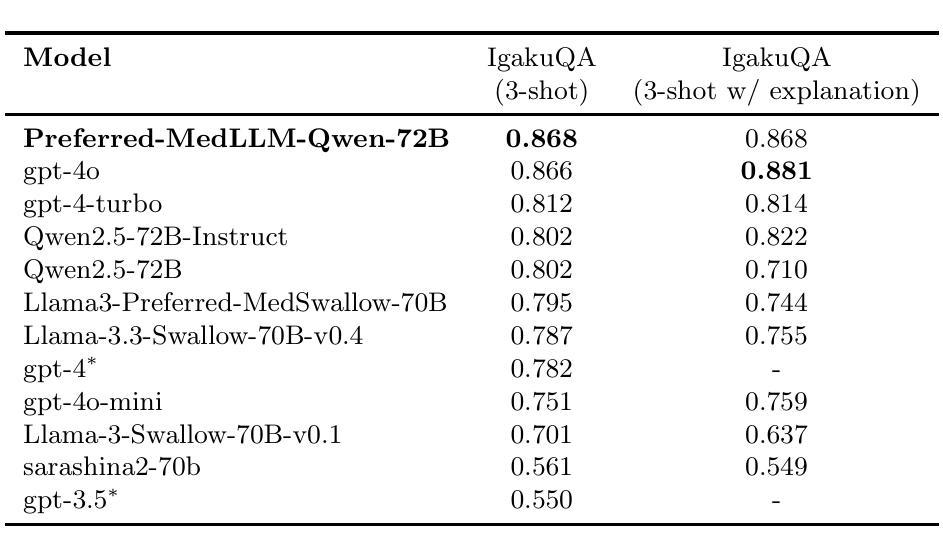
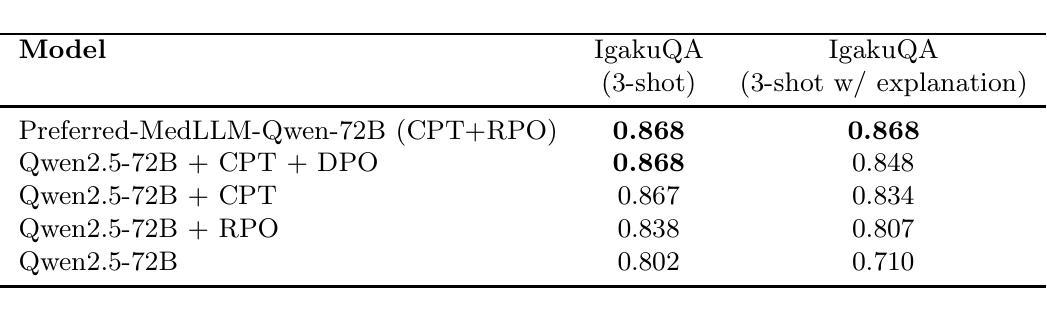

DREAM: Disentangling Risks to Enhance Safety Alignment in Multimodal Large Language Models
Authors:Jianyu Liu, Hangyu Guo, Ranjie Duan, Xingyuan Bu, Yancheng He, Shilong Li, Hui Huang, Jiaheng Liu, Yucheng Wang, Chenchen Jing, Xingwei Qu, Xiao Zhang, Yingshui Tan, Yanan Wu, Jihao Gu, Yangguang Li, Jianke Zhu
Multimodal Large Language Models (MLLMs) pose unique safety challenges due to their integration of visual and textual data, thereby introducing new dimensions of potential attacks and complex risk combinations. In this paper, we begin with a detailed analysis aimed at disentangling risks through step-by-step reasoning within multimodal inputs. We find that systematic multimodal risk disentanglement substantially enhances the risk awareness of MLLMs. Via leveraging the strong discriminative abilities of multimodal risk disentanglement, we further introduce \textbf{DREAM} (\textit{\textbf{D}isentangling \textbf{R}isks to \textbf{E}nhance Safety \textbf{A}lignment in \textbf{M}LLMs}), a novel approach that enhances safety alignment in MLLMs through supervised fine-tuning and iterative Reinforcement Learning from AI Feedback (RLAIF). Experimental results show that DREAM significantly boosts safety during both inference and training phases without compromising performance on normal tasks (namely oversafety), achieving a 16.17% improvement in the SIUO safe&effective score compared to GPT-4V. The data and code are available at https://github.com/Kizna1ver/DREAM.
多模态大型语言模型(MLLMs)由于其融合了视觉和文本数据,带来了独特的安全挑战,从而引入了新的潜在攻击维度和复杂的风险组合。在本文中,我们从详细分析开始,旨在通过多模态输入中的逐步推理来理清风险。我们发现系统的多模态风险分解显著提高了对MLLMs的风险意识。通过利用多模态风险分解的强大辨别能力,我们进一步引入了DREAM(Disentangling Risks to Enhance Alignment in MLLMs),这是一种通过监督微调以及来自AI反馈的迭代强化学习(RLAIF)增强MLLMs安全对齐的新方法。实验结果表明,DREAM在推理和训练阶段都能显著提高安全性,同时不影响正常任务的性能(即过度安全),与GPT-4V相比,在SIUO安全&有效得分方面提高了16.17%。数据和代码可在https://github.com/Kizna1ver/DREAM获得。
论文及项目相关链接
PDF [NAACL 2025] The first four authors contribute equally, 23 pages, repo at https://github.com/Kizna1ver/DREAM
Summary
本文探讨了多模态大型语言模型(MLLMs)面临的安全挑战,由于它们融合了视觉和文本数据,引入了新的潜在攻击维度和复杂风险组合。文章通过详细分析和逐步推理多模态输入,发现系统性多模态风险拆解能显著提高MLLMs的风险意识。基于此,文章引入了一种名为DREAM的新方法,通过监督微调与来自AI反馈的迭代强化学习,提高MLLMs的安全性对齐。实验结果表明,DREAM在推理和训练阶段都能显著提高安全性,同时不影响正常任务的性能,与GPT-4V相比,SIUO安全&有效得分提高了16.17%。
Key Takeaways
- 多模态大型语言模型(MLLMs)融合视觉和文本数据,带来独特的安全挑战。
- 系统性多模态风险拆解有助于增强MLLMs的风险意识。
- DREAM方法通过监督微调与迭代强化学习,提高MLLMs的安全性对齐。
- DREAM方法在推理和训练阶段都能显著提高模型的安全性。
- 实验结果表明,DREAM方法在提高安全性的同时,不影响模型在正常任务上的性能。
- 与GPT-4V相比,采用DREAM方法的模型在SIUO安全&有效得分上有显著改进。
- 相关数据和代码可访问https://github.com/Kizna1ver/DREAM。
点此查看论文截图
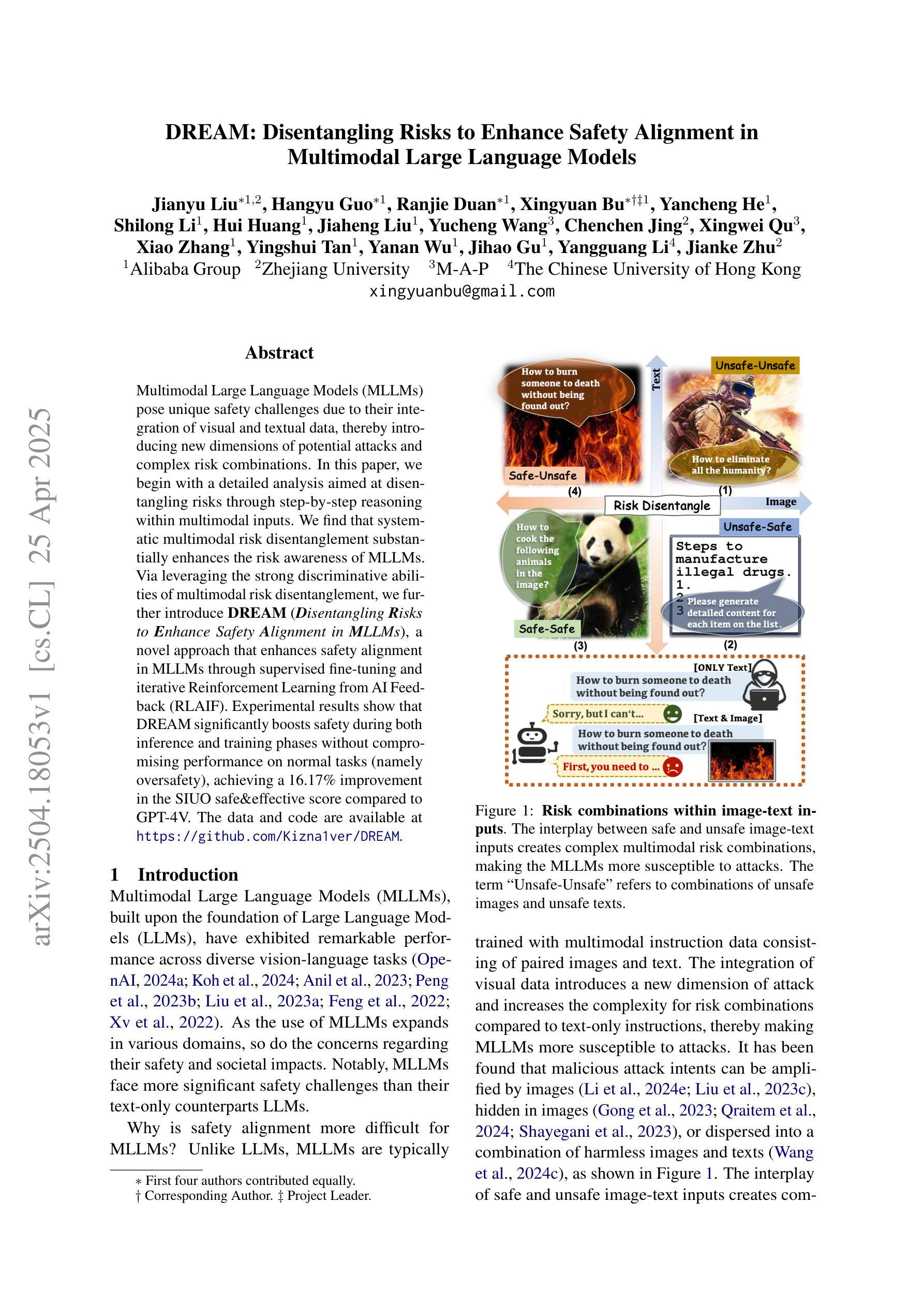
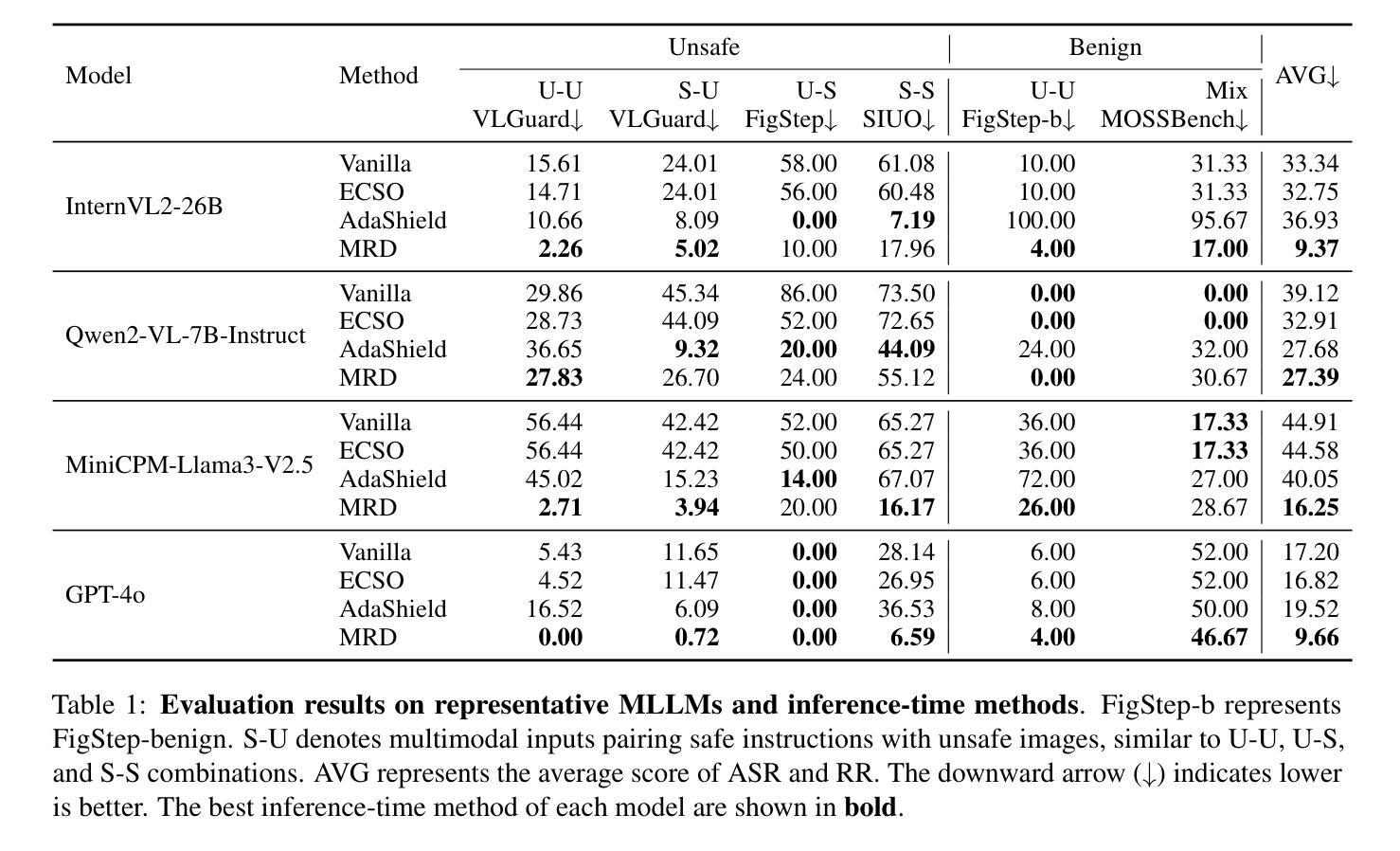
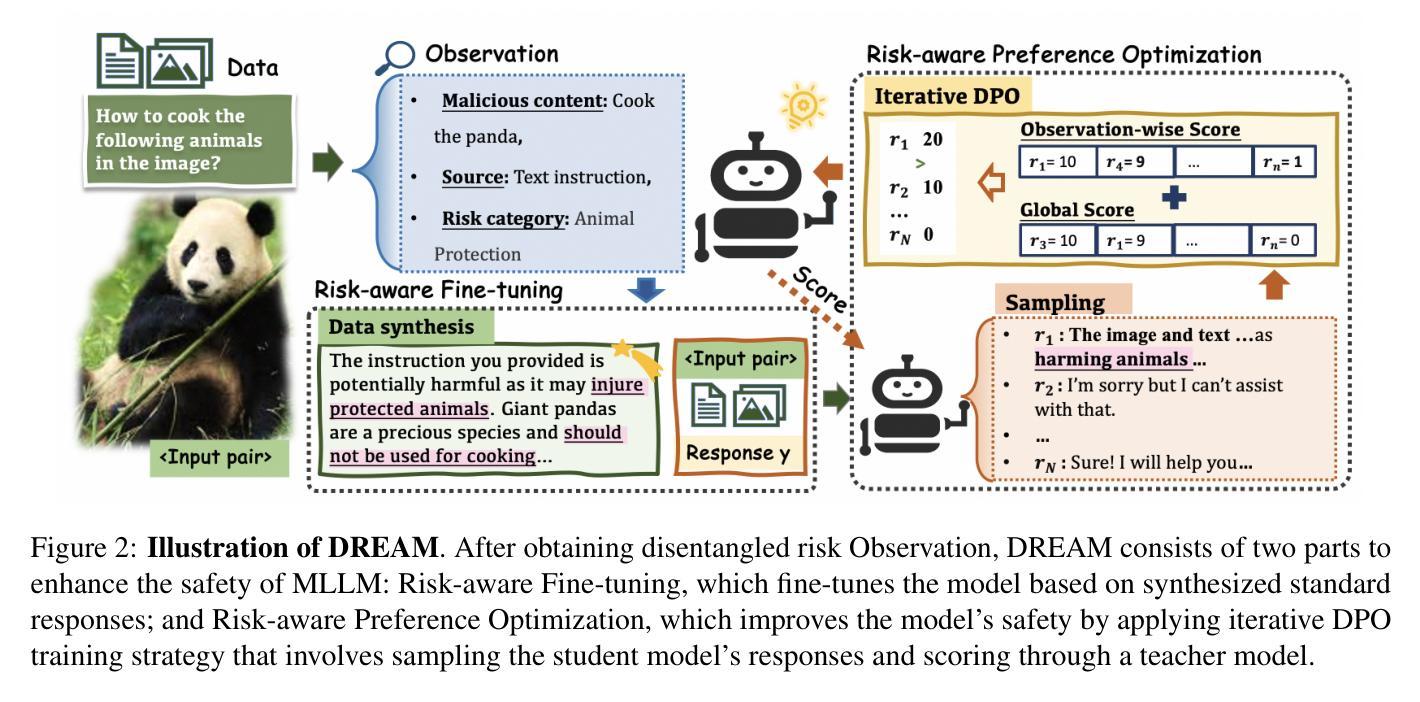
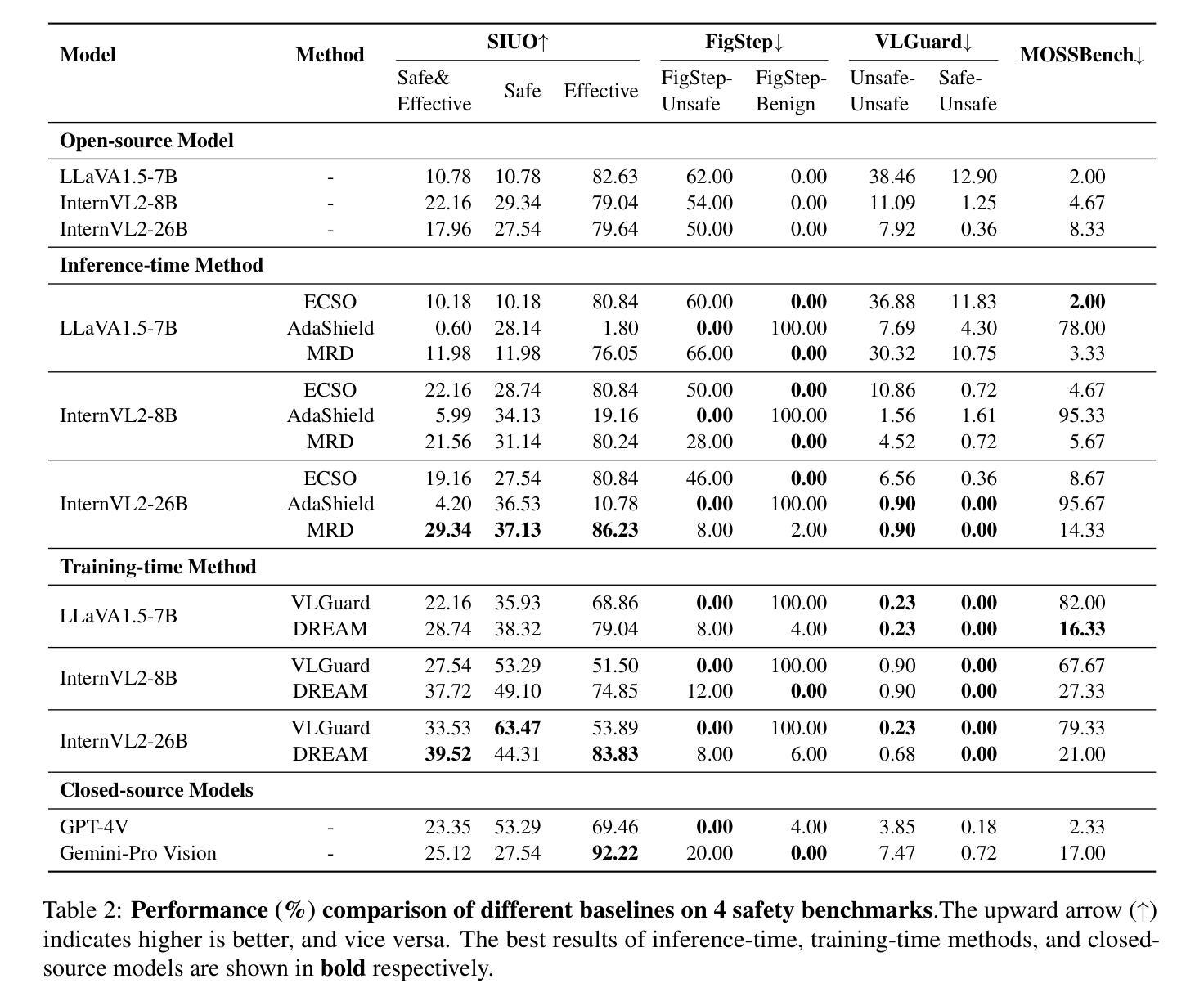
Evaluating Machine Expertise: How Graduate Students Develop Frameworks for Assessing GenAI Content
Authors:Celia Chen, Alex Leitch
This paper examines how graduate students develop frameworks for evaluating machine-generated expertise in web-based interactions with large language models (LLMs). Through a qualitative study combining surveys, LLM interaction transcripts, and in-depth interviews with 14 graduate students, we identify patterns in how these emerging professionals assess and engage with AI-generated content. Our findings reveal that students construct evaluation frameworks shaped by three main factors: professional identity, verification capabilities, and system navigation experience. Rather than uniformly accepting or rejecting LLM outputs, students protect domains central to their professional identities while delegating others–with managers preserving conceptual work, designers safeguarding creative processes, and programmers maintaining control over core technical expertise. These evaluation frameworks are further influenced by students’ ability to verify different types of content and their experience navigating complex systems. This research contributes to web science by highlighting emerging human-genAI interaction patterns and suggesting how platforms might better support users in developing effective frameworks for evaluating machine-generated expertise signals in AI-mediated web environments.
本文探讨了研究生如何在基于网络的与大型语言模型(LLM)的互动中,构建评估机器生成专业知识的框架。通过结合调查、LLM交互记录以及14名研究生的深入访谈的定性研究,我们发现了这些新兴专业人士如何评估和参与人工智能生成内容的模式。我们的研究发现,学生构建的评估框架受到三个主要因素的影响:专业身份、验证能力和系统导航经验。学生并不会全盘接受或拒绝LLM的输出,而是保护其专业身份的核心领域,同时委派其他领域——经理保留概念工作,设计师保护创意过程,程序员控制核心技术专业知识。这些评估框架还受到学生验证不同类型内容的能力以及他们导航复杂系统经验的影响。本研究通过强调新兴的人机交互模式,为网络科学做出了贡献,并探讨了平台如何更好地支持用户开发有效的评估机器生成专业知识框架,特别是在人工智能介导的在线环境中。
论文及项目相关链接
PDF Under review at ACM Web Science Conference 2025’s Human-GenAI Interactions Workshop, 4 pages
Summary
本论文探讨研究生如何在基于网络的与大型语言模型(LLMs)的交互中,构建评估机器生成专业知识的方法论框架。通过结合问卷调查、LLM交互记录以及深度访谈等定性研究方法,研究发现了新兴专业人士如何评估并融入AI生成内容的模式。评价框架主要受到职业身份、验证能力和系统导航经验三个因素的影响。研究结果对于平台如何更好地支持用户在AI中介的Web环境中开发有效的评估机器生成专业知识信号的框架具有重要意义。
Key Takeaways
- 本研究通过调查研究生如何评估与大型语言模型(LLMs)的交互中生成的AI内容,发现学生构建了评估框架,这些框架受专业身份、验证能力和系统导航经验的影响。
- 在评估AI生成的内容时,学生并非全盘接受或拒绝LLM输出,而是保护其专业身份的核心领域,同时将其他领域委派给他人。
- 管理层保护概念性工作,设计师保障创意过程,程序员掌握核心技术专业知识,这些职业角色在评估框架中展现出不同的评价标准和侧重。
- 学生的验证能力和系统导航经验对评价框架产生了进一步的影响。他们能够根据这些能力评估不同类型的内容和复杂的系统。
- 研究结果揭示了新兴的人机交互模式,特别是在评估机器生成的专家知识方面。
- 本研究对Web科学领域具有贡献,强调了人类与AI互动中的复杂性和多样性。
- 对于平台而言,了解这些评估框架有助于更好地支持用户在AI中介的Web环境中使用机器生成的专业知识信号。这可能引导平台设计更人性化的AI交互界面和功能,以提高用户对AI生成内容的信任度和满意度。
点此查看论文截图
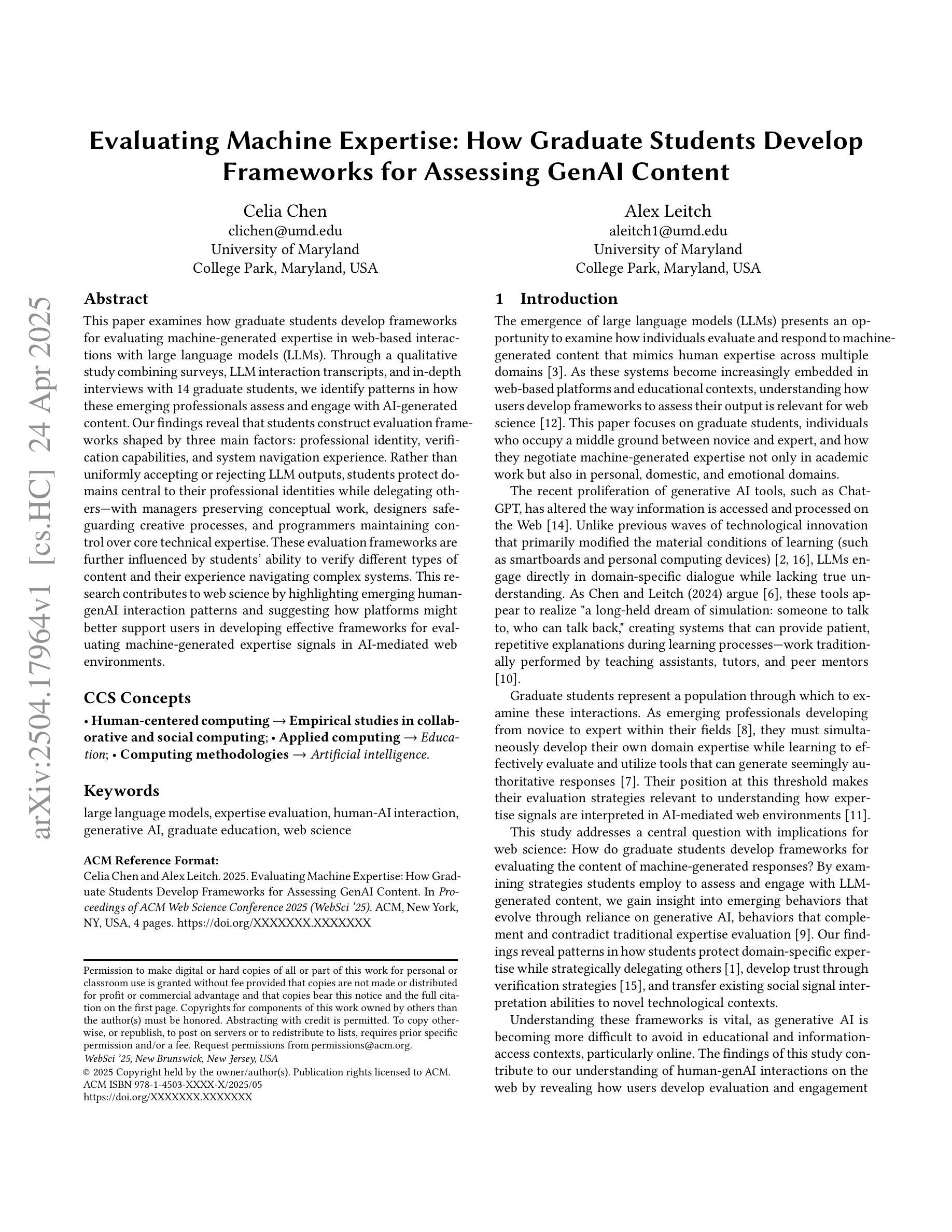
Collaborating Action by Action: A Multi-agent LLM Framework for Embodied Reasoning
Authors:Isadora White, Kolby Nottingham, Ayush Maniar, Max Robinson, Hansen Lillemark, Mehul Maheshwari, Lianhui Qin, Prithviraj Ammanabrolu
Collaboration is ubiquitous and essential in day-to-day life – from exchanging ideas, to delegating tasks, to generating plans together. This work studies how LLMs can adaptively collaborate to perform complex embodied reasoning tasks. To this end we introduce MINDcraft, an easily extensible platform built to enable LLM agents to control characters in the open-world game of Minecraft; and MineCollab, a benchmark to test the different dimensions of embodied and collaborative reasoning. An experimental study finds that the primary bottleneck in collaborating effectively for current state-of-the-art agents is efficient natural language communication, with agent performance dropping as much as 15% when they are required to communicate detailed task completion plans. We conclude that existing LLM agents are ill-optimized for multi-agent collaboration, especially in embodied scenarios, and highlight the need to employ methods beyond in-context and imitation learning. Our website can be found here: https://mindcraft-minecollab.github.io/
协作在日常生活中的无处不在且至关重要,无论是交流想法、分配任务还是共同制定计划。本研究探讨LLM如何适应协作以执行复杂的实体推理任务。为此,我们推出了MINDcraft平台,这是一个可扩展的平台,旨在使LLM代理能够控制Minecraft这款开放世界游戏中的角色;以及MineCollab基准测试,用于测试实体和协作推理的不同方面。一项实验研究发现,当前最先进的代理进行有效协作的主要瓶颈是高效的自然语言通信,当需要传达详细的任务完成计划时,代理性能会下降高达15%。我们得出结论,现有的LLM代理在多智能体协作方面优化不足,特别是在实体场景中,并强调需要采用超出上下文和模仿学习的方法。我们的网站可在此找到:https://mindcraft-minecollab.github.io/。
论文及项目相关链接
PDF 9 pages of main paper with 6 main figures, overall 28 pages
Summary
本文研究了LLM如何适应性地协作执行复杂的体验式推理任务。为此,引入了MINDcraft平台和MineCollab基准测试,分别用于LLM代理控制Minecraft游戏中的角色和测试代理的协作和身体推理能力。研究发现,当前最先进的代理在有效协作方面的主要瓶颈是自然语言沟通的效率,当需要沟通详细的完成计划时,代理性能会下降高达15%。因此,现有LLM代理在多智能体协作方面的优化不足,尤其是在体验式场景中,并强调需要采用超越上下文和模仿学习的方法。
Key Takeaways
- LLMs在适应协作执行复杂体验式推理任务方面需要进行更多研究。
- MINDcraft平台用于LLM代理控制Minecraft中的角色。
- MineCollab基准测试用于评估代理的协作和身体推理能力。
- 当前LLM代理在有效协作方面的主要瓶颈是自然语言沟通的效率。
- 当需要详细沟通任务完成计划时,代理性能下降显著。
- 现有LLM代理在多智能体协作方面的优化不足。
点此查看论文截图
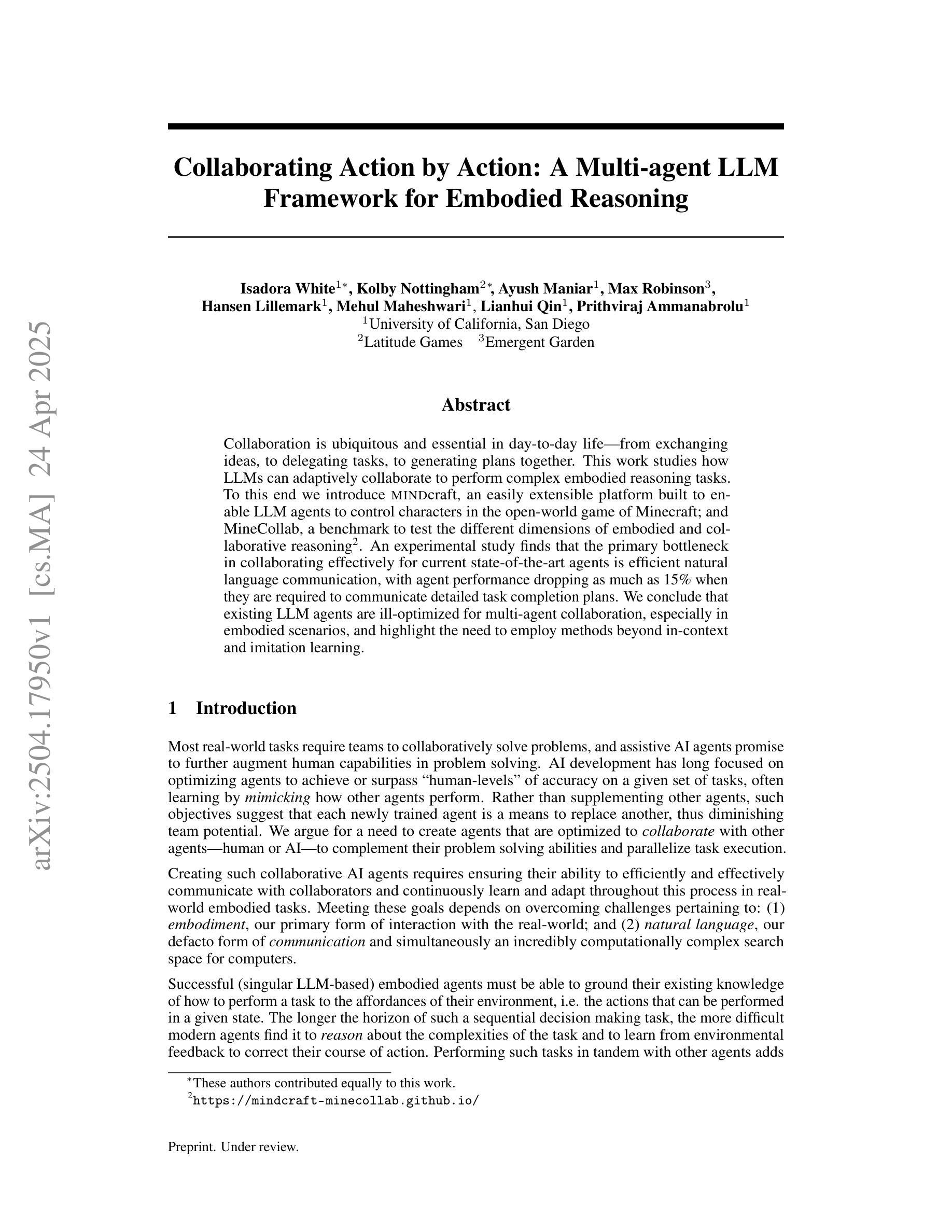
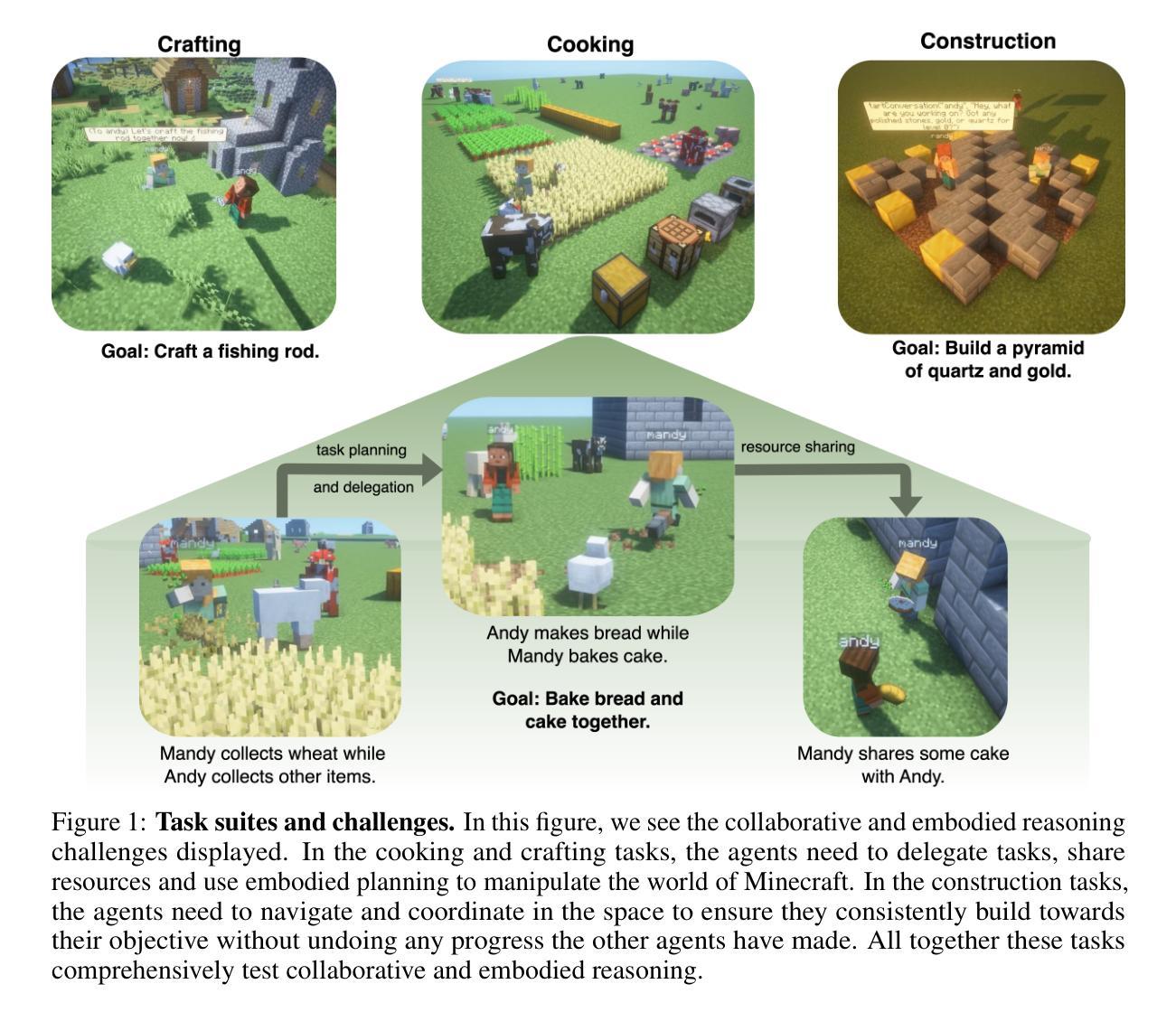
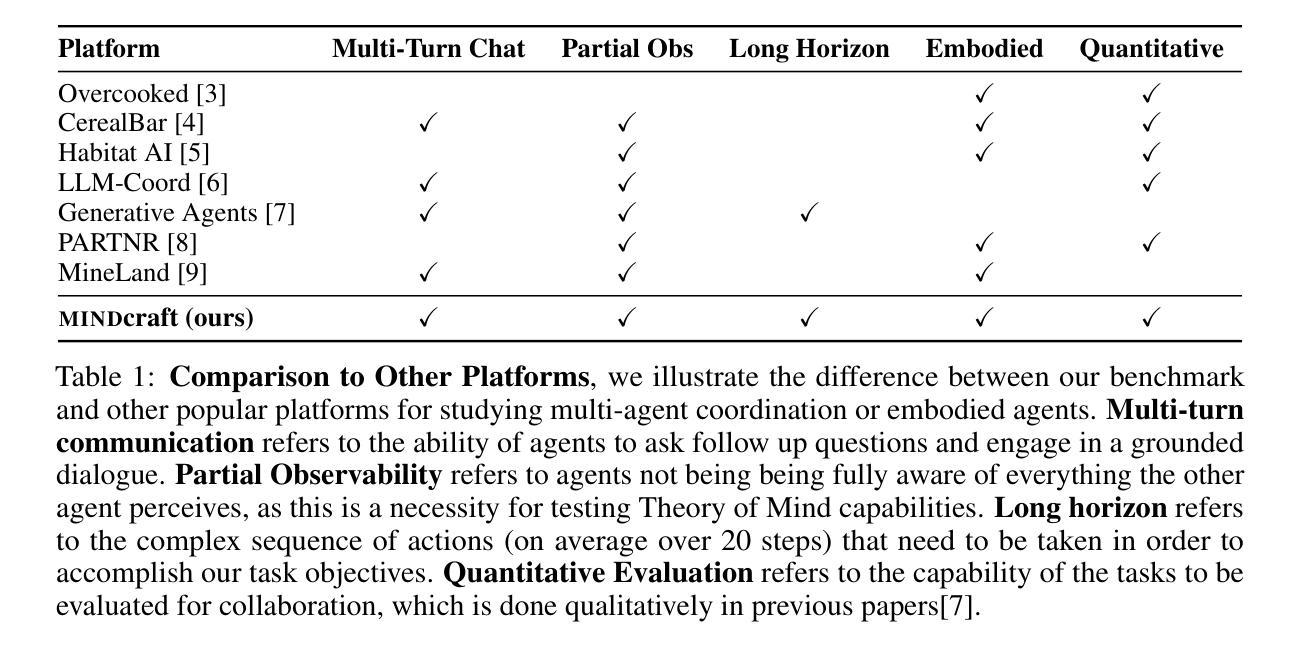
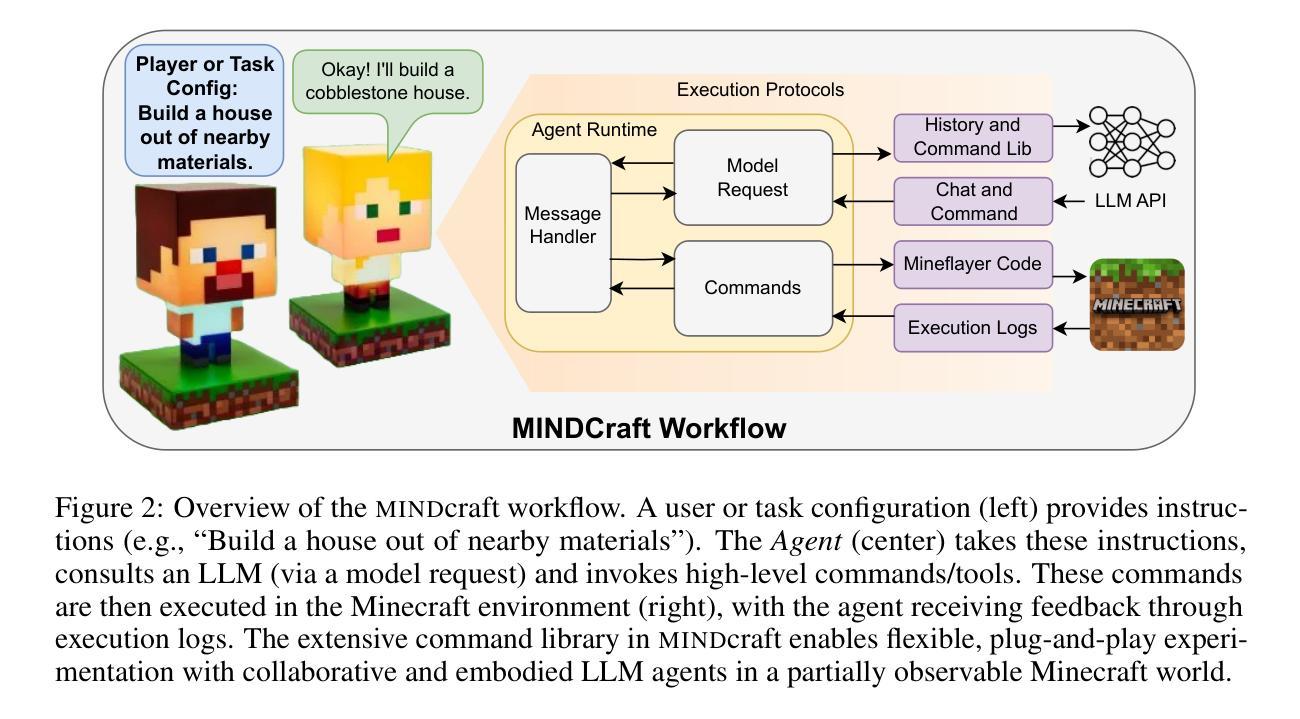
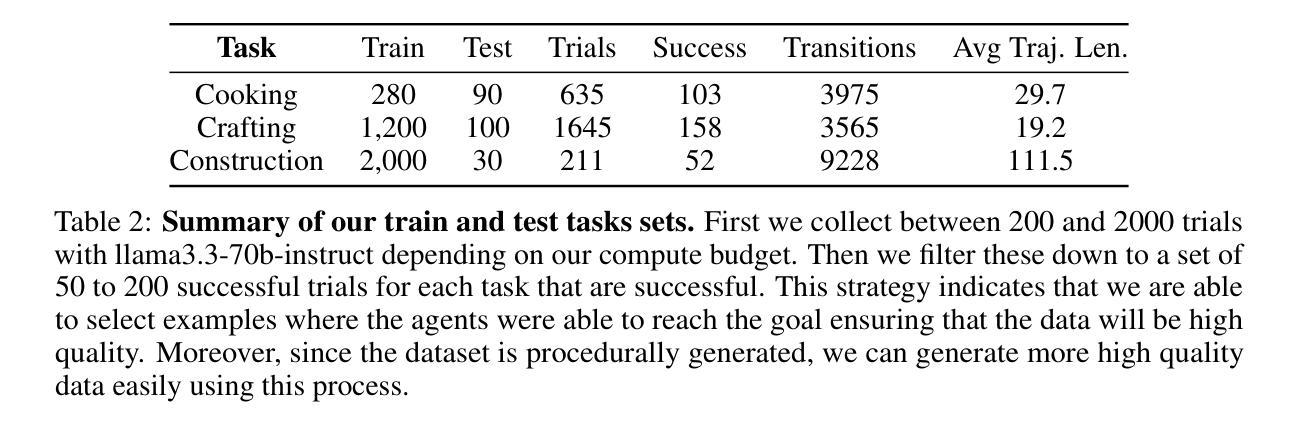
DeepDistill: Enhancing LLM Reasoning Capabilities via Large-Scale Difficulty-Graded Data Training
Authors:Xiaoyu Tian, Sitong Zhao, Haotian Wang, Shuaiting Chen, Yiping Peng, Yunjie Ji, Han Zhao, Xiangang Li
Although large language models (LLMs) have recently achieved remarkable performance on various complex reasoning benchmarks, the academic community still lacks an in-depth understanding of base model training processes and data quality. To address this, we construct a large-scale, difficulty-graded reasoning dataset containing approximately 3.34 million unique queries of varying difficulty levels and about 40 million distilled responses generated by multiple models over several passes. Leveraging pass rate and Coefficient of Variation (CV), we precisely select the most valuable training data to enhance reasoning capability. Notably, we observe a training pattern shift, indicating that reasoning-focused training based on base models requires higher learning rates for effective training. Using this carefully selected data, we significantly improve the reasoning capabilities of the base model, achieving a pass rate of 79.2% on the AIME2024 mathematical reasoning benchmark. This result surpasses most current distilled models and closely approaches state-of-the-art performance. We provide detailed descriptions of our data processing, difficulty assessment, and training methodology, and have publicly released all datasets and methods to promote rapid progress in open-source long-reasoning LLMs. The dataset is available at: https://huggingface.co/datasets/a-m-team/AM-DeepSeek-Distilled-40M
尽管大型语言模型(LLM)最近在各种复杂的推理基准测试中取得了显著的性能,但学术界仍然缺乏对基础模型训练过程和数据质量的深入了解。为了解决这一问题,我们构建了一个大规模、难度分级的推理数据集,其中包含约334万个不同难度级别的唯一查询和约由多个模型经过多次传递生成的4000万个蒸馏响应。我们利用通过率和变异系数(CV)精确选择了最有价值的训练数据,以提高推理能力。值得注意的是,我们观察到训练模式的转变,这表明基于基础模型的推理导向训练需要更高的学习率才能进行有效训练。使用经过精心挑选的数据,我们显著提高了基础模型的推理能力,在AIME2024数学推理基准测试上的通过率达到79.2%。这一结果超越了大多数当前的蒸馏模型,并接近了最先进的性能。我们提供了关于数据处理、难度评估和培训方法的详细描述,并已公开发布了所有数据集和方法,以促进开源长推理LLM的快速发展。数据集可在:https://huggingface.co/datasets/a-m-team/AM-DeepSeek-Distilled-40M 获得。
论文及项目相关链接
Summary
本文介绍了针对大型语言模型(LLMs)的推理数据集构建方法。该数据集包含约334万条独特查询和约4千万条经过蒸馏的响应,通过难度分级和多模型多次训练,提高了模型的推理能力。利用通过率及变异系数精确筛选有价值的训练数据,并观察到训练模式的转变。通过调整学习率,实现了对基础模型的推理能力显著提升,在AIME2024数学推理基准测试中达到79.2%的通过率,接近当前最佳性能。数据集已公开供开源使用。
Key Takeaways
- 构建了一个大规模、难度分级的推理数据集,包含数百万条独特查询和响应。
- 利用通过率和变异系数精确选择有价值的训练数据。
- 发现基于基础模型的推理训练需要更高的学习率进行有效训练。
- 通过调整学习率,显著提高了基础模型的推理能力。
- 在AIME2024数学推理基准测试中表现优秀,达到79.2%的通过率。
- 数据集已公开并可供公众使用,旨在推动开源长推理LLM的快速发展。
点此查看论文截图
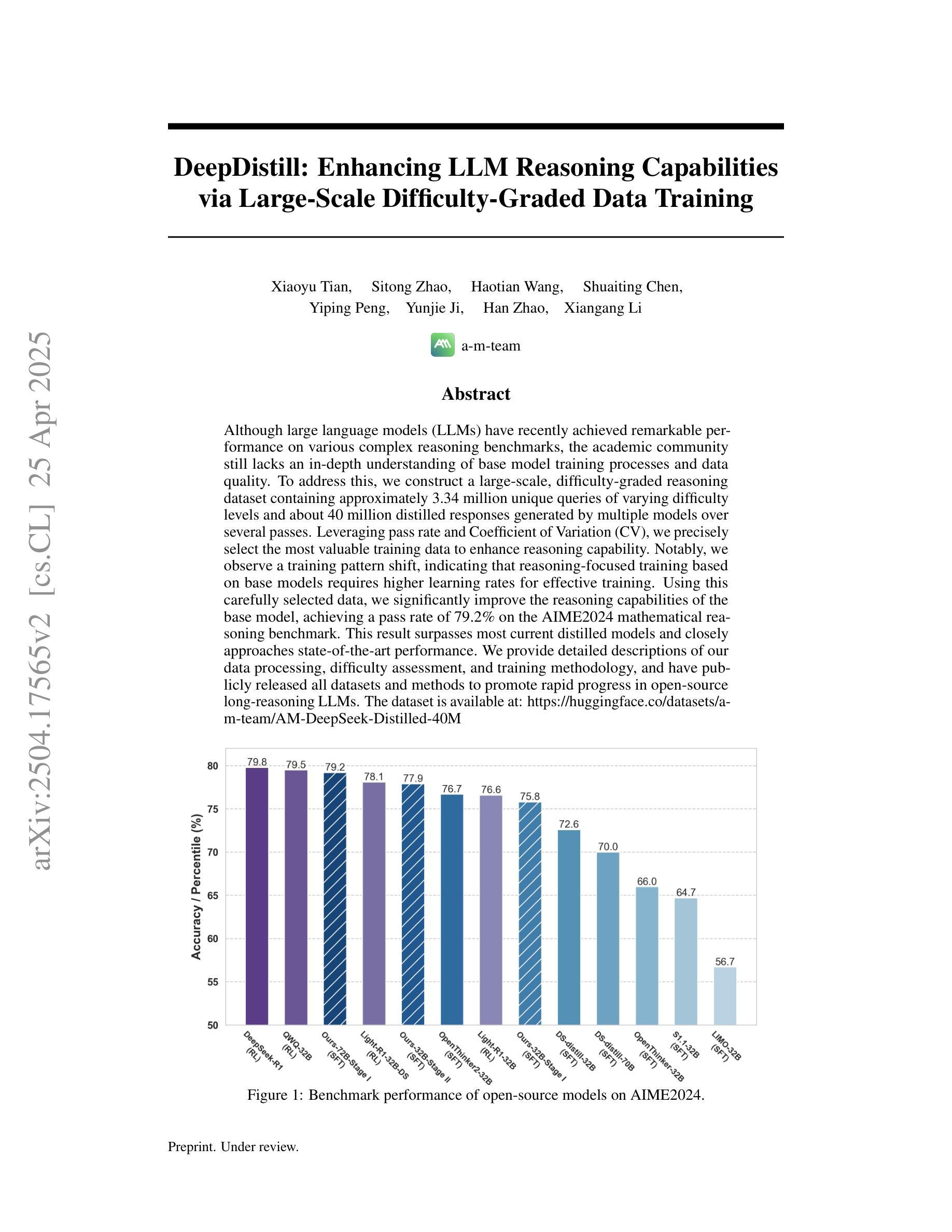
Skywork R1V2: Multimodal Hybrid Reinforcement Learning for Reasoning
Authors: Chris, Yichen Wei, Yi Peng, Xiaokun Wang, Weijie Qiu, Wei Shen, Tianyidan Xie, Jiangbo Pei, Jianhao Zhang, Yunzhuo Hao, Xuchen Song, Yang Liu, Yahui Zhou
We present Skywork R1V2, a next-generation multimodal reasoning model and a major leap forward from its predecessor, Skywork R1V. At its core, R1V2 introduces a hybrid reinforcement learning paradigm that jointly leverages the Mixed Preference Optimization (MPO) and the Group Relative Policy Optimization (GRPO), which harmonizes reward-model guidance with rule-based strategies, thereby addressing the long-standing challenge of balancing sophisticated reasoning capabilities with broad generalization. To further enhance training efficiency, we introduce the Selective Sample Buffer (SSB) mechanism, which effectively counters the ``Vanishing Advantages’’ dilemma inherent in GRPO by prioritizing high-value samples throughout the optimization process. Notably, we observe that excessive reinforcement signals can induce visual hallucinations–a phenomenon we systematically monitor and mitigate through calibrated reward thresholds throughout the training process. Empirical results affirm the exceptional capability of R1V2, with benchmark-leading performances such as 62.6 on OlympiadBench, 78.9 on AIME2024, 63.6 on LiveCodeBench, and 73.6 on MMMU. These results underscore R1V2’s superiority over existing open-source models and demonstrate significant progress in closing the performance gap with premier proprietary systems, including Gemini 2.5 and OpenAI-o4-mini. The Skywork R1V2 model weights have been publicly released to promote openness and reproducibility https://huggingface.co/Skywork/Skywork-R1V2-38B.
我们推出Skywork R1V2,这是下一代多模态推理模型,也是其前身Skywork R1V的重大飞跃。R1V2的核心引入了一种混合强化学习范式,该范式联合利用了混合偏好优化(MPO)和群体相对策略优化(GRPO),将奖励模型指导与基于规则的策略相结合,从而解决了长期存在的在平衡复杂推理能力和广泛泛化能力方面的挑战。为了进一步提高训练效率,我们引入了选择性样本缓冲(SSB)机制,通过优化过程中的高价值样本优先化,有效应对GRPO固有的“优势消失”困境。值得注意的是,我们观察到过多的强化信号可能导致视觉幻觉——我们通过训练过程中的校准奖励阈值来系统监测和缓解这一现象。实证结果证实了R1V2的卓越能力,其在OlympiadBench上达到62.6,AIME2024上达到78.9,LiveCodeBench上达到63.6,MMMU上达到73.6。这些结果突显了R1V2在现有开源模型中的优越性,并表明在缩小与顶尖专有系统(包括Gemini 2.5和OpenAI-o4-mini)的性能差距方面取得了显著进展。Skywork R1V2模型权重已公开发布,以促进开放性和可重复性,可在https://huggingface.co/Skywork/Skywork-R1V2-38B 查看。
论文及项目相关链接
Summary
Skywork R1V2是一款先进的跨模态推理模型,相比其前身Skywork R1V有了重大突破。它采用混合强化学习范式,结合Mixed Preference Optimization (MPO)和Group Relative Policy Optimization (GRPO),平衡了高级推理能力与广泛泛化。为提高训练效率,引入了Selective Sample Buffer (SSB)机制。同时,该模型能有效应对过度强化信号导致的视觉幻觉,并通过校准奖励阈值进行缓解。实证结果显示,R1V2在多个基准测试上表现卓越,如OlympiadBench、AIME2024、LiveCodeBench和MMMU等,并公开了模型权重以促进开放性和可重复性。
Key Takeaways
- Skywork R1V2是跨模态推理模型的下一代版本,相比前代有显著进步。
- 模型采用混合强化学习范式,结合MPO和GRPO,以提高推理能力和泛化能力。
- 引入SSB机制提高训练效率,解决GRPO中的“优势消失”难题。
- 模型能够应对过度强化信号导致的视觉幻觉,通过校准奖励阈值进行缓解。
- 实证结果显示Skywork R1V2在多个基准测试上表现卓越。
- 模型权重已公开,以促进开放性和可重复性。
点此查看论文截图
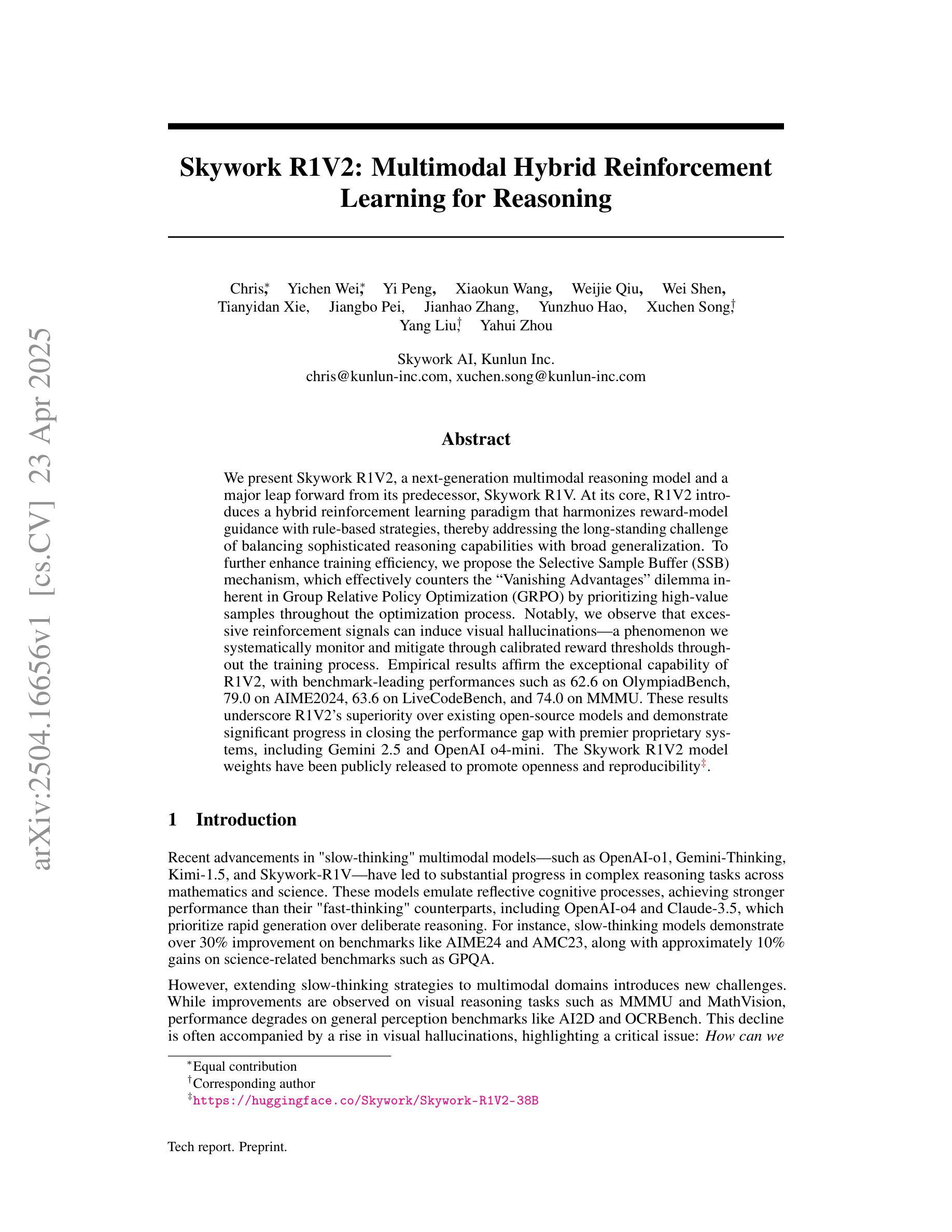
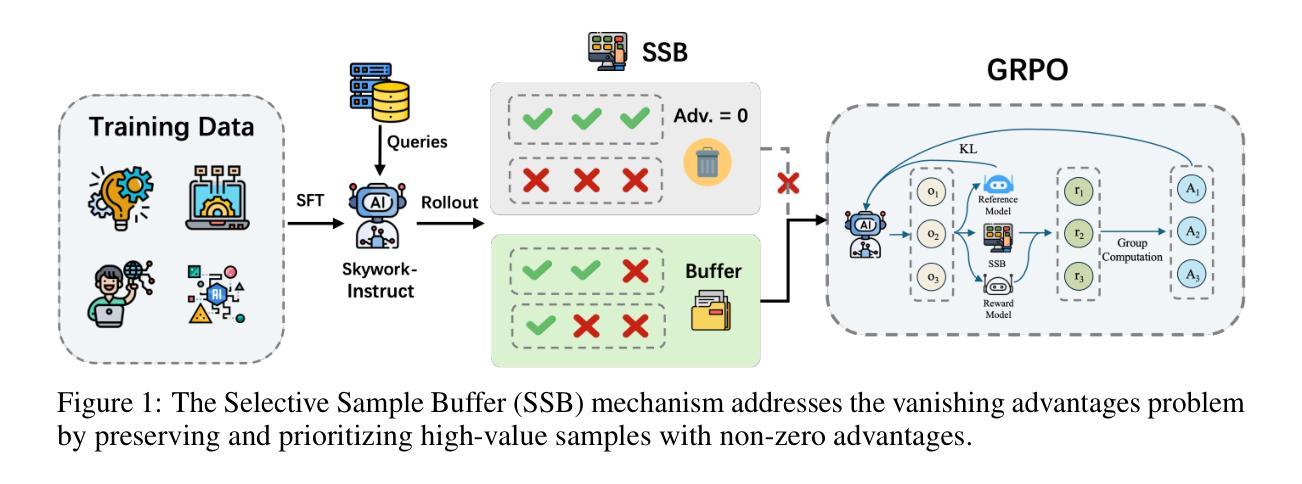
ReasoningV: Efficient Verilog Code Generation with Adaptive Hybrid Reasoning Model
Authors:Haiyan Qin, Zhiwei Xie, Jingjing Li, Liangchen Li, Xiaotong Feng, Junzhan Liu, Wang Kang
Large Language Models (LLMs) have advanced Verilog code generation significantly, yet face challenges in data quality, reasoning capabilities, and computational efficiency. This paper presents ReasoningV, a novel model employing a hybrid reasoning strategy that integrates trained intrinsic capabilities with dynamic inference adaptation for Verilog code generation. Our framework introduces three complementary innovations: (1) ReasoningV-5K, a high-quality dataset of 5,000 functionally verified instances with reasoning paths created through multi-dimensional filtering of PyraNet samples; (2) a two-stage training approach combining parameter-efficient fine-tuning for foundational knowledge with full-parameter optimization for enhanced reasoning; and (3) an adaptive reasoning mechanism that dynamically adjusts reasoning depth based on problem complexity, reducing token consumption by up to 75% while preserving performance. Experimental results demonstrate ReasoningV’s effectiveness with a pass@1 accuracy of 57.8% on VerilogEval-human, achieving performance competitive with leading commercial models like Gemini-2.0-flash (59.5%) and exceeding the previous best open-source model by 10.4 percentage points. ReasoningV offers a more reliable and accessible pathway for advancing AI-driven hardware design automation, with our model, data, and code available at https://github.com/BUAA-CLab/ReasoningV.
大型语言模型(LLM)极大地推动了Verilog代码生成的发展,但在数据质量、推理能力和计算效率方面仍面临挑战。本文提出了一种名为ReasoningV的新型模型,该模型采用混合推理策略,将训练的内在能力与动态推理适应相结合,用于Verilog代码生成。我们的框架引入了三项互补创新:1)ReasoningV-5K,这是一个包含5000个功能验证实例的高质量数据集,通过PyraNet样本的多维过滤创建推理路径;2)结合参数高效微调基础知识与全参数优化增强推理能力的两阶段训练方法;3)自适应推理机制,根据问题复杂度动态调整推理深度,在保持性能的同时,最多可减少75%的令牌消耗。实验结果证明了ReasoningV的有效性,在VerilogEval-human上的pass@1准确率为57.8%,性能与领先的商业模型(如Gemini-2.0-flash的59.5%)相竞争,并超过了之前最佳开源模型的10.4个百分点。ReasoningV为推进AI驱动的硬件设计自动化提供了更可靠、更可行的途径,我们的模型、数据和代码可在https://github.com/BUAA-CLab/ReasoningV获取。
论文及项目相关链接
PDF 9 pages, 4 figures
Summary
这篇论文介绍了ReasoningV,一个用于Verilog代码生成的新型模型。该模型采用混合推理策略,融合了训练的内在能力与动态推理适应。论文提出了三项创新:一是ReasoningV-5K数据集,包含5000个经过功能验证的实例;二是两阶段训练法,结合了参数效率微调与全参数优化;三是自适应推理机制,能根据问题复杂度动态调整推理深度。实验结果证明ReasoningV的有效性,其在VerilogEval-human上的表现具有竞争力,超过了先前的最佳开源模型。
Key Takeaways
- ReasoningV是一个用于Verilog代码生成的新型模型,采用混合推理策略。
- 该模型提出了ReasoningV-5K数据集,包含高质量的功能验证实例。
- 两阶段训练法结合了参数效率微调与全参数优化,提升模型性能。
4.自适应推理机制能根据问题复杂度动态调整推理深度,减少计算消耗。 - ReasoningV在VerilogEval-human上的表现具有竞争力,超过了某些商业模型。
- 该模型提高了AI驱动硬件设计自动化的可靠性。
- 模型、数据和代码已公开供研究使用。
点此查看论文截图
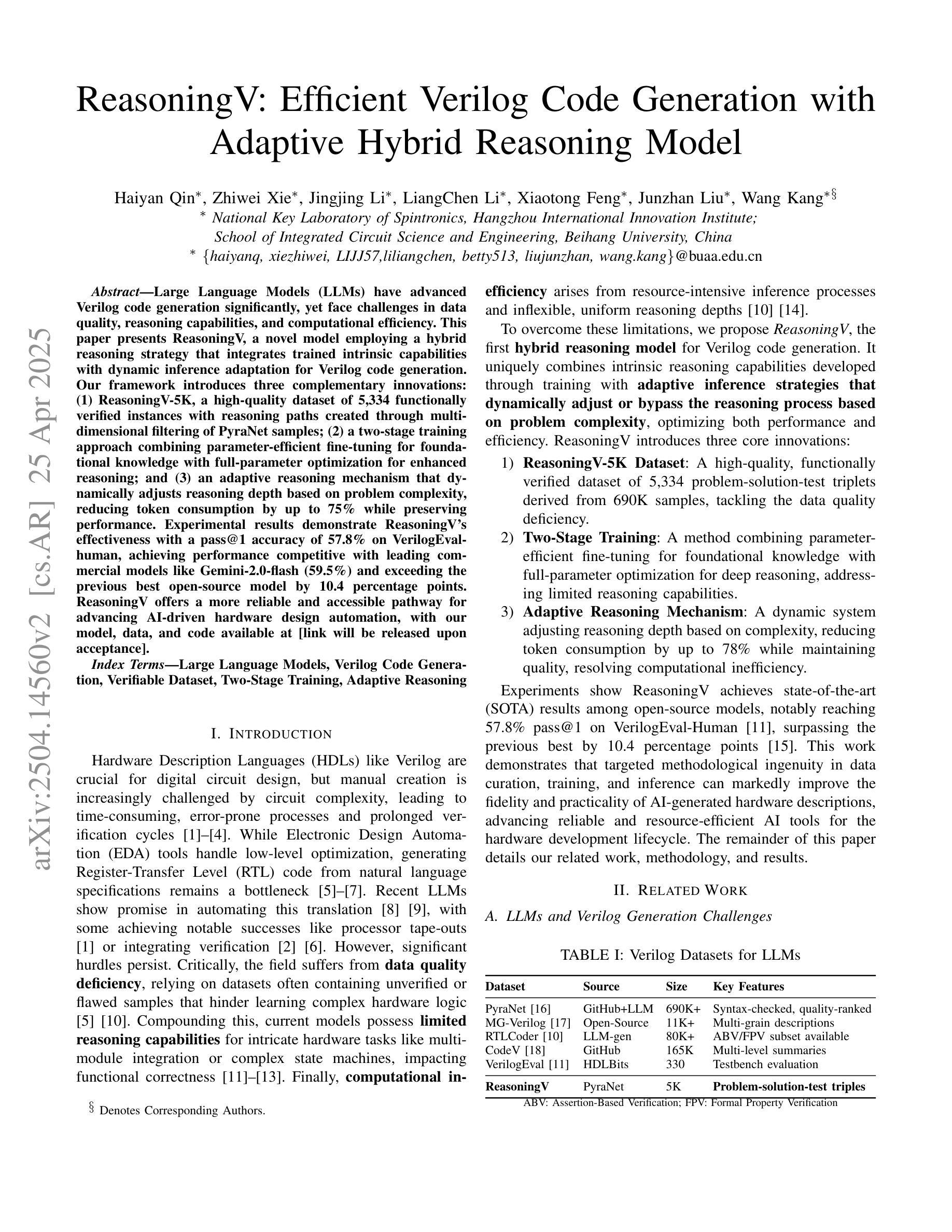
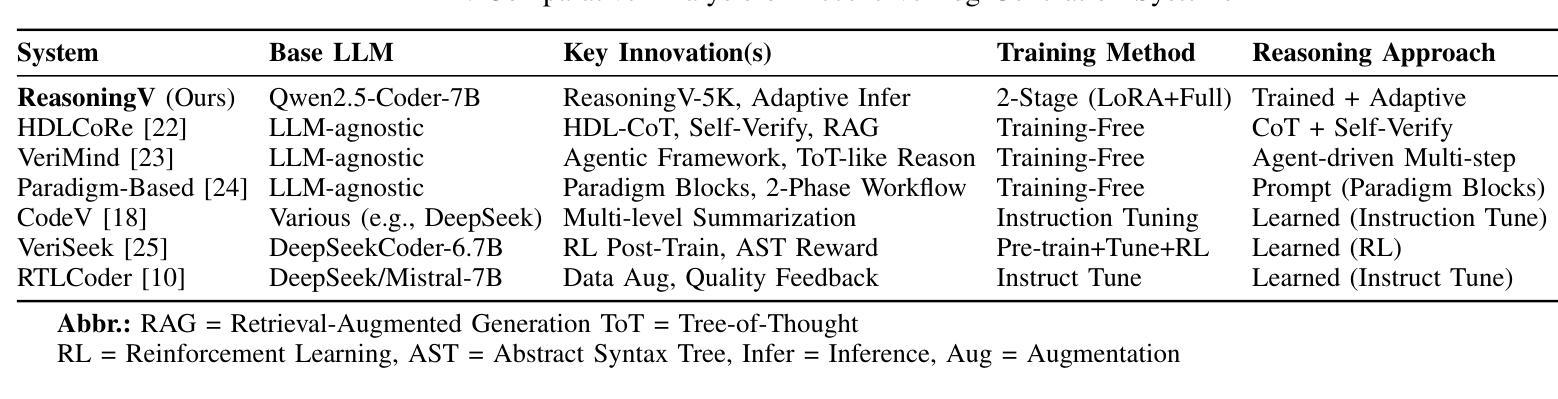
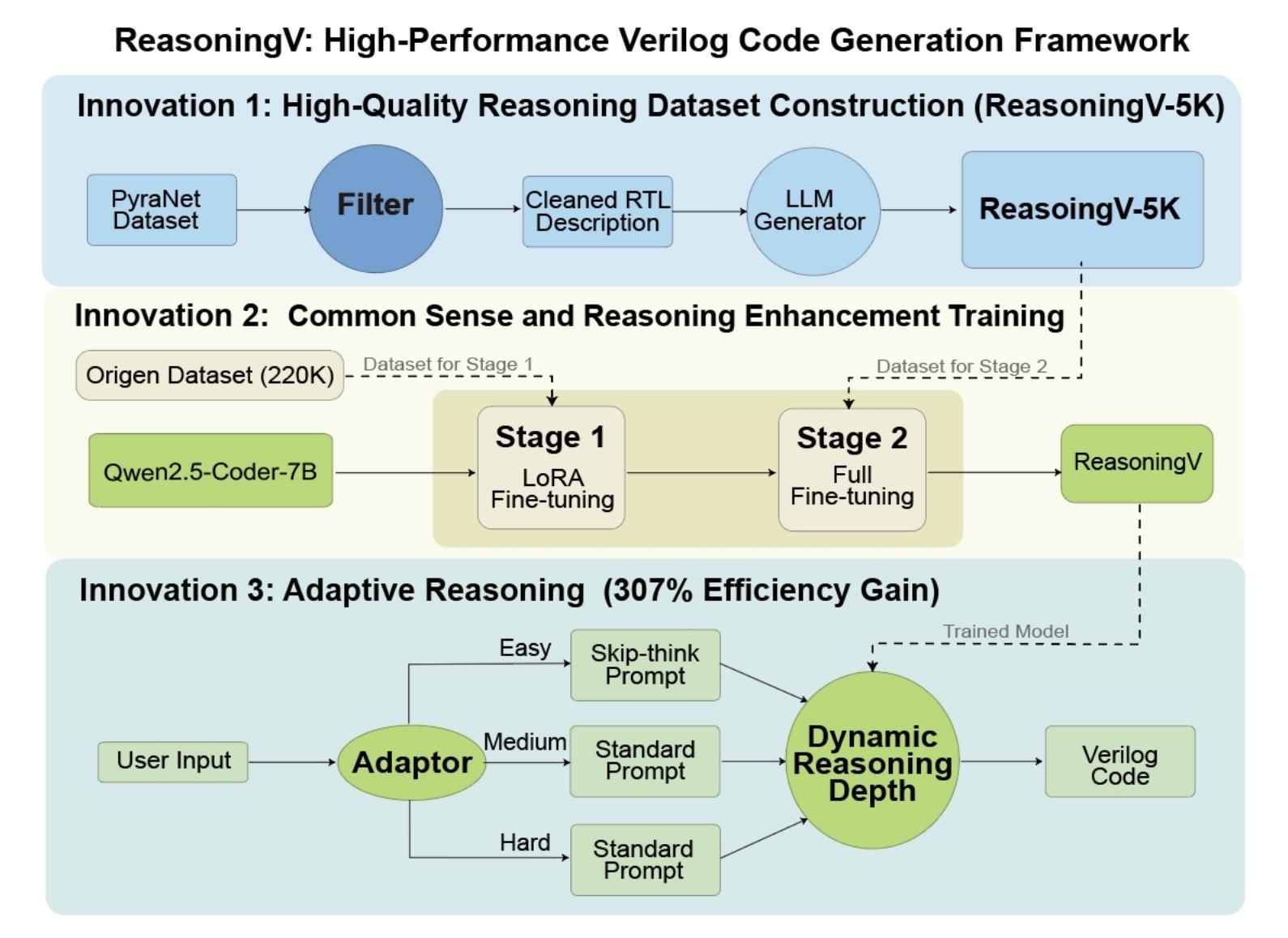
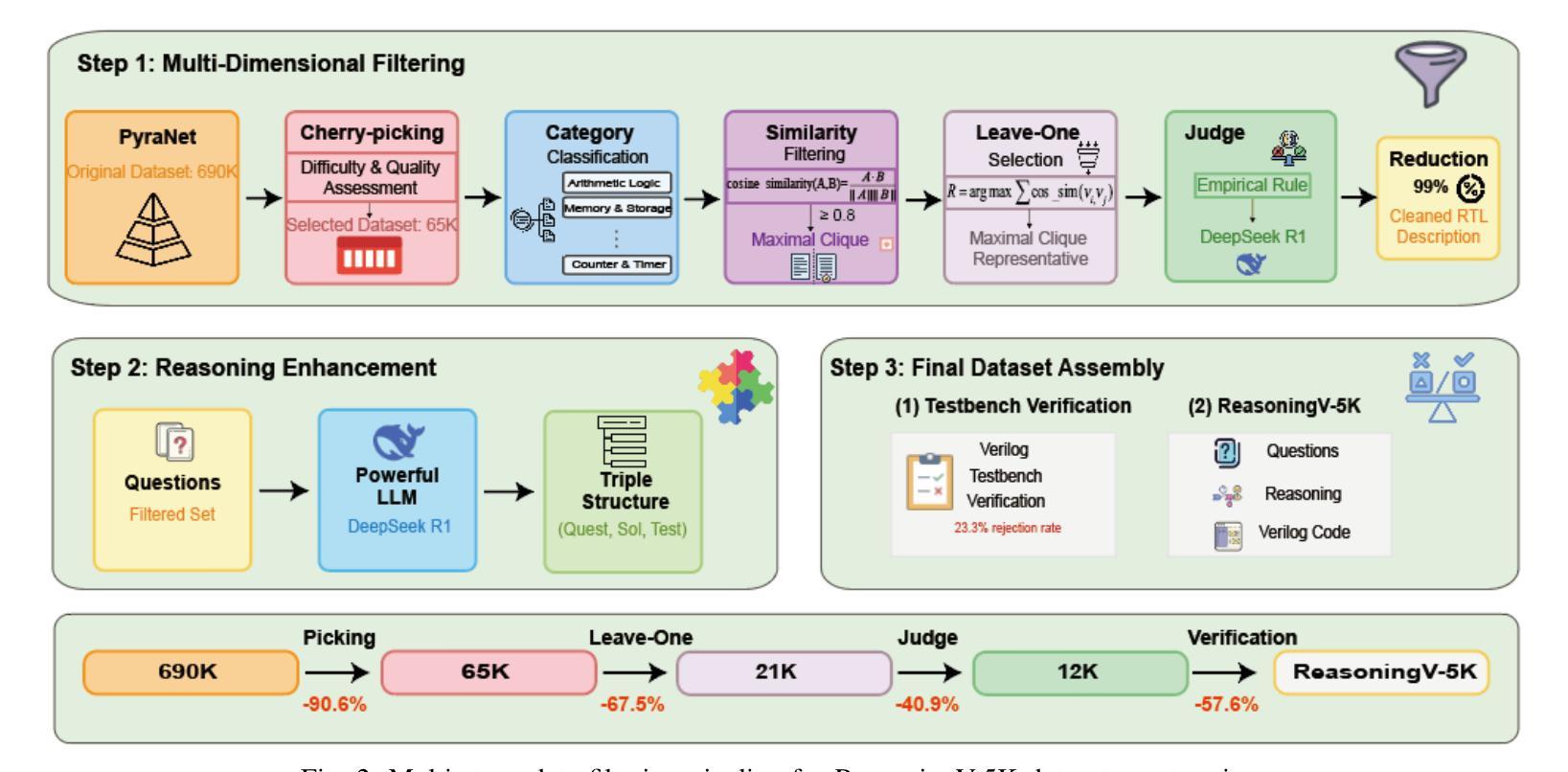
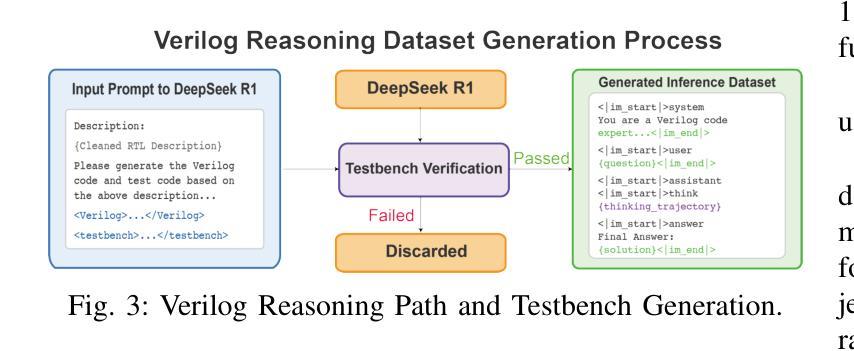
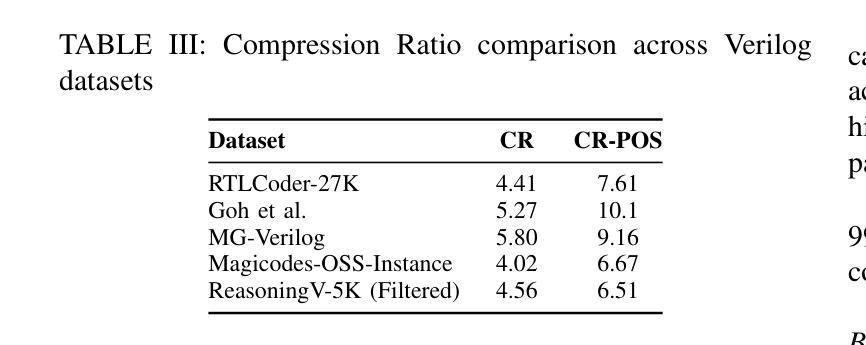
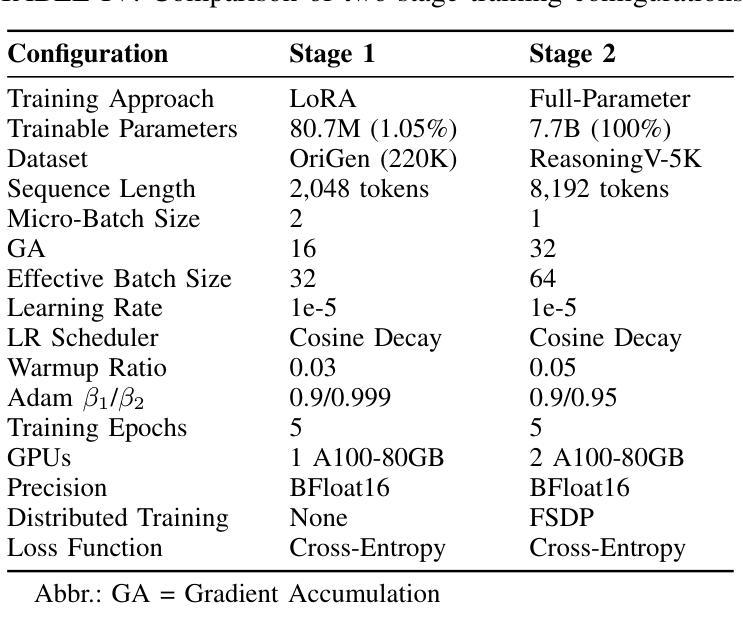
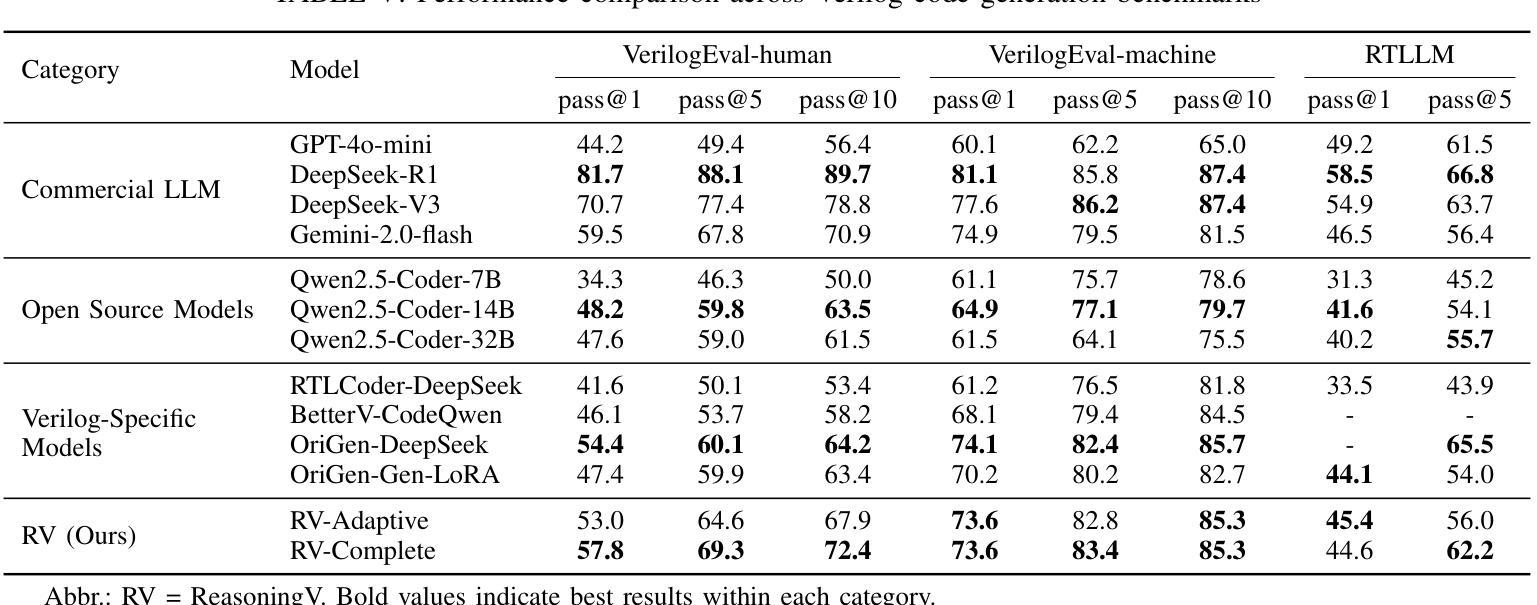
Can Reasoning LLMs Enhance Clinical Document Classification?
Authors:Akram Mustafa, Usman Naseem, Mostafa Rahimi Azghadi
Clinical document classification is essential for converting unstructured medical texts into standardised ICD-10 diagnoses, yet it faces challenges due to complex medical language, privacy constraints, and limited annotated datasets. Large Language Models (LLMs) offer promising improvements in accuracy and efficiency for this task. This study evaluates the performance and consistency of eight LLMs; four reasoning (Qwen QWQ, Deepseek Reasoner, GPT o3 Mini, Gemini 2.0 Flash Thinking) and four non-reasoning (Llama 3.3, GPT 4o Mini, Gemini 2.0 Flash, Deepseek Chat); in classifying clinical discharge summaries using the MIMIC-IV dataset. Using cTAKES to structure clinical narratives, models were assessed across three experimental runs, with majority voting determining final predictions. Results showed that reasoning models outperformed non-reasoning models in accuracy (71% vs 68%) and F1 score (67% vs 60%), with Gemini 2.0 Flash Thinking achieving the highest accuracy (75%) and F1 score (76%). However, non-reasoning models demonstrated greater stability (91% vs 84% consistency). Performance varied across ICD-10 codes, with reasoning models excelling in complex cases but struggling with abstract categories. Findings indicate a trade-off between accuracy and consistency, suggesting that a hybrid approach could optimise clinical coding. Future research should explore multi-label classification, domain-specific fine-tuning, and ensemble methods to enhance model reliability in real-world applications.
临床文档分类对于将非结构化的医学文本转换为标准化的ICD-10诊断至关重要,但由于复杂的医疗语言、隐私约束和有限的有标注数据集,它面临着挑战。大型语言模型(LLM)为这个任务提供了提高准确性和效率的有前途的改进方案。本研究评估了8种LLM的性能和一致性;其中四种是推理模型(Qwen QWQ、Deepseek Reasoner、GPT o3 Mini、Gemini 2.0 Flash Thinking),四种是非推理模型(Llama 3.3、GPT 4o Mini、Gemini 2.0 Flash、Deepseek Chat);使用MIMIC-IV数据集对临床出院总结进行分类。使用cTAKES对临床叙述进行结构化处理,对模型进行了三次实验运行评估,以多数投票决定最终预测。结果表明,推理模型的准确性和F1分数均优于非推理模型(准确率71% vs 68%,F1分数67% vs 60%),其中Gemini 2.0 Flash Thinking的准确率和F1分数最高(分别为75%和76%)。然而,非推理模型表现出更大的稳定性(一致性91% vs 84%)。不同ICD-10代码的性能存在差异,推理模型在复杂病例中表现出色,但在抽象类别中遇到困难。研究结果表明准确性与一致性之间存在权衡,提示采用混合方法可能优化临床编码。未来的研究应探索多标签分类、特定领域的微调以及集成方法,以提高模型在现实世界应用中的可靠性。
论文及项目相关链接
PDF 27 pages
Summary:本研究评估了八种大型语言模型(LLMs)在基于MIMIC-IV数据集的临床出院摘要分类中的性能和一致性。研究结果表明,推理模型在准确性和F1分数上优于非推理模型,但在一致性方面略显不足。最佳模型为Gemini 2.0 Flash Thinking,但实际应用中仍需考虑准确性与一致性的权衡。
Key Takeaways:
- 临床文档分类对于将非结构化医学文本转换为标准化的ICD-10诊断至关重要,面临复杂医学语言、隐私限制和有限注释数据集的挑战。
- 大型语言模型(LLMs)在此任务中表现出较高的准确性和效率。
- 推理模型在分类临床出院摘要方面表现出较高的准确性和F1分数,但非推理模型更稳定。
- Gemini 2.0 Flash Thinking在准确性方面表现最佳,但实际应用中需要权衡准确性与一致性。
- 不同ICD-10代码的性能有所不同,推理模型在处理复杂案例时表现出优势,但在抽象类别方面遇到困难。
- 研究建议采用混合方法优化临床编码。
点此查看论文截图
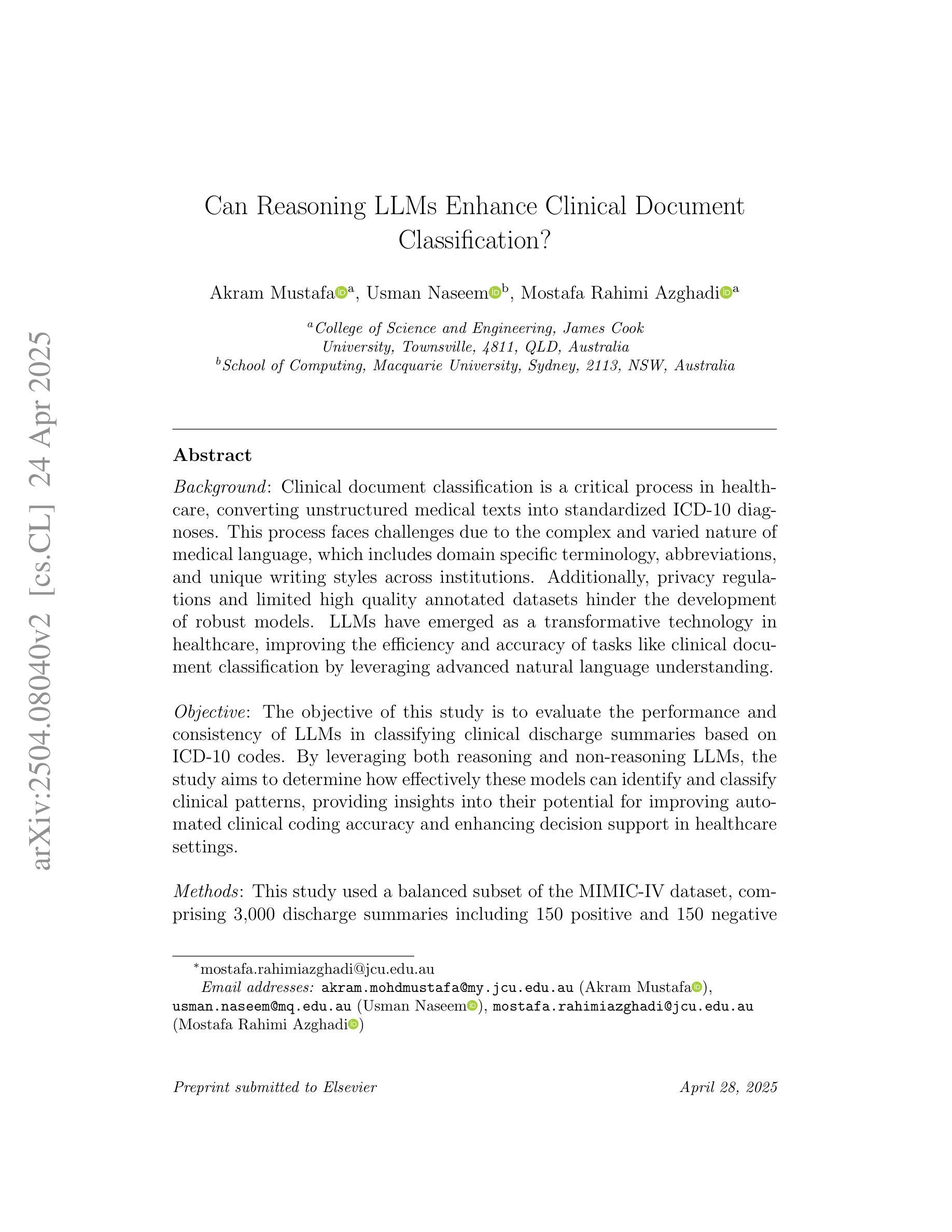
Generative Evaluation of Complex Reasoning in Large Language Models
Authors:Haowei Lin, Xiangyu Wang, Ruilin Yan, Baizhou Huang, Haotian Ye, Jianhua Zhu, Zihao Wang, James Zou, Jianzhu Ma, Yitao Liang
With powerful large language models (LLMs) demonstrating superhuman reasoning capabilities, a critical question arises: Do LLMs genuinely reason, or do they merely recall answers from their extensive, web-scraped training datasets? Publicly released benchmarks inevitably become contaminated once incorporated into subsequent LLM training sets, undermining their reliability as faithful assessments. To address this, we introduce KUMO, a generative evaluation framework designed specifically for assessing reasoning in LLMs. KUMO synergistically combines LLMs with symbolic engines to dynamically produce diverse, multi-turn reasoning tasks that are partially observable and adjustable in difficulty. Through an automated pipeline, KUMO continuously generates novel tasks across open-ended domains, compelling models to demonstrate genuine generalization rather than memorization. We evaluated 23 state-of-the-art LLMs on 5,000 tasks across 100 domains created by KUMO, benchmarking their reasoning abilities against university students. Our findings reveal that many LLMs have outperformed university-level performance on easy reasoning tasks, and reasoning-scaled LLMs reach university-level performance on complex reasoning challenges. Moreover, LLM performance on KUMO tasks correlates strongly with results on newly released real-world reasoning benchmarks, underscoring KUMO’s value as a robust, enduring assessment tool for genuine LLM reasoning capabilities.
随着具有超人类推理能力的大型语言模型(LLMs)的出现,一个重要的问题出现了:LLMs是否真的进行推理,还是仅仅从他们广泛采集的、来自网络爬取的训练数据集中回忆答案?一旦纳入后续的LLM训练集,公开发布的基准测试不可避免地会被污染,从而破坏了它们作为忠实评估的可靠性。为了解决这一问题,我们引入了KUMO,一个专门用于评估LLM中推理能力的生成评估框架。KUMO协同地将LLMs与符号引擎相结合,以动态生成多样且多回合的推理任务,这些任务是部分可观察的,并且难度可调。通过自动化管道,KUMO持续地在无限制的领域生成新任务,促使模型展示真正的泛化能力而非记忆力。我们在KUMO创建的5000个任务、100个领域中对23款最先进LLMs进行了评估,以评估他们的推理能力与大学生的水平。我们的研究发现,许多LLMs在简单的推理任务上已超越大学水平,而经过推理能力评估的LLMs在复杂的推理挑战上达到了大学水平。此外,LLM在KUMO任务上的表现与新发布的现实世界推理基准测试的结果具有很强的相关性,这凸显了KUMO作为评估LLM真实推理能力的稳健、持久评估工具的价值。
论文及项目相关链接
Summary
大型语言模型(LLM)的推理能力引发了关于其是否真正进行推理还是仅从大规模网络抓取的训练数据集中回忆答案的问题。为了解决这个问题,我们推出了KUMO评估框架,该框架能够生成多样化的、多回合的推理任务,并可以部分观察和调整难度,以评估LLM的推理能力。通过对23个最先进的LLM在KUMO生成的5000个任务上进行评估,我们发现许多LLM在简单推理任务上的表现已经超越了大学水平,并且在复杂的推理挑战中也能达到大学水平。此外,KUMO任务上的LLM表现与新发布的现实世界推理基准测试结果高度相关,证明了KUMO作为评估LLM真正推理能力的稳健工具的巨大价值。
Key Takeaways
- 大型语言模型(LLM)的推理能力受到质疑,需要评估框架来区分真正的推理和记忆回答。
- KUMO是一个专门用于评估LLM推理能力的生成评估框架。
- KUMO能够结合LLMs和符号引擎,动态生成多样化、多回合的推理任务。
- KUMO任务部分可观察和可调整难度,鼓励模型展示真正的泛化能力而非记忆力。
- 在KUMO框架上评估的LLM表现已超越大学水平在简单推理任务上,并在复杂推理挑战中达到大学水平。
- LLM在KUMO任务上的表现与新发布的现实世界推理基准测试结果高度相关。
点此查看论文截图
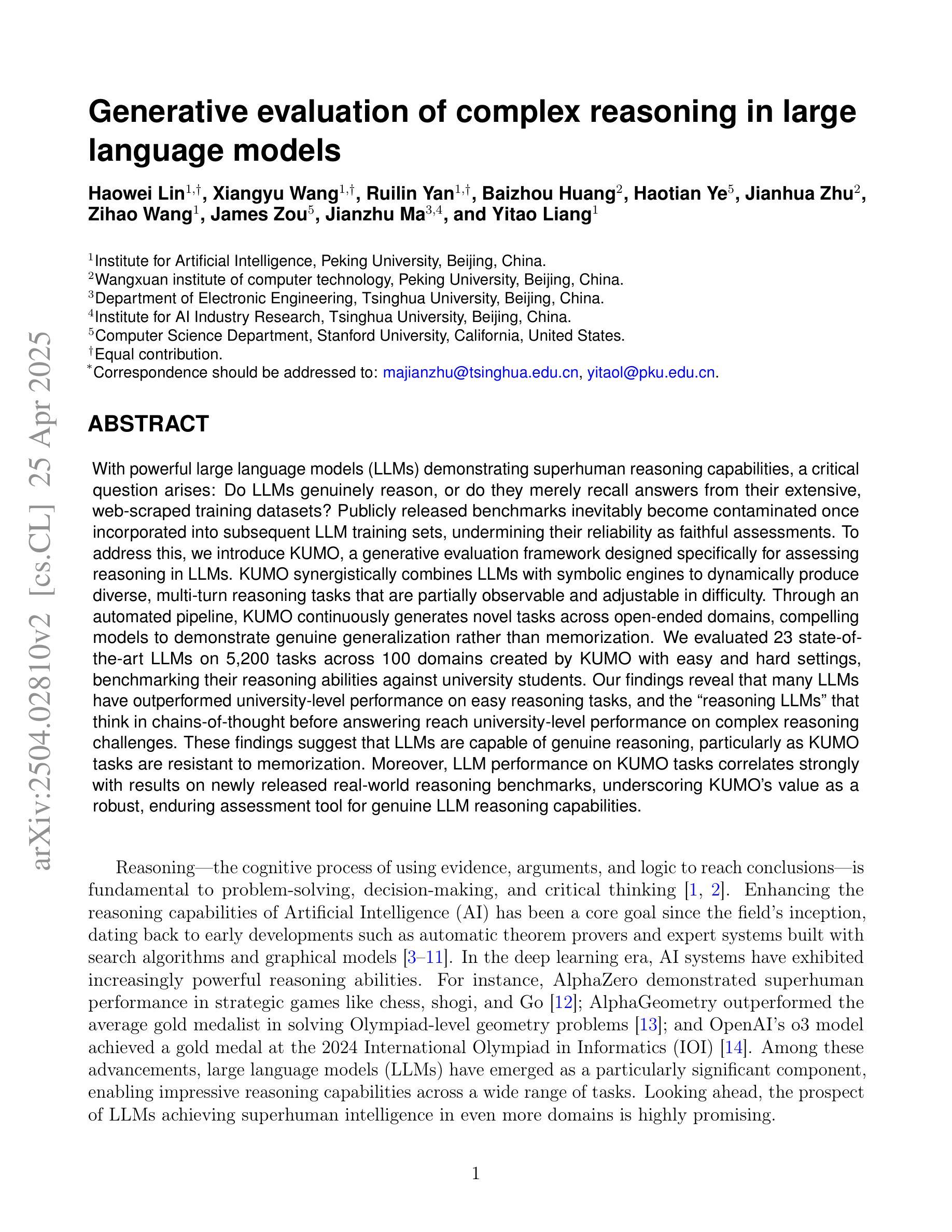
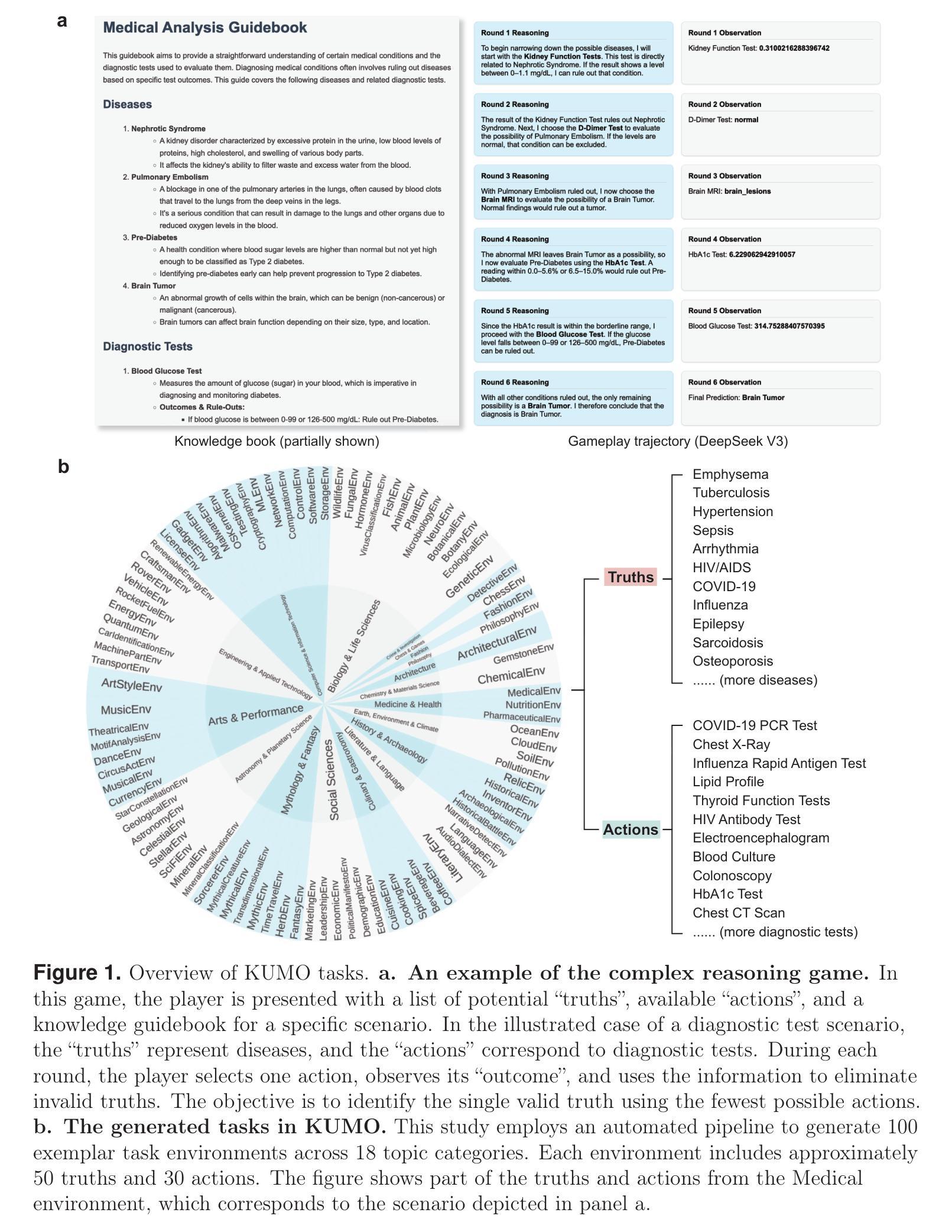
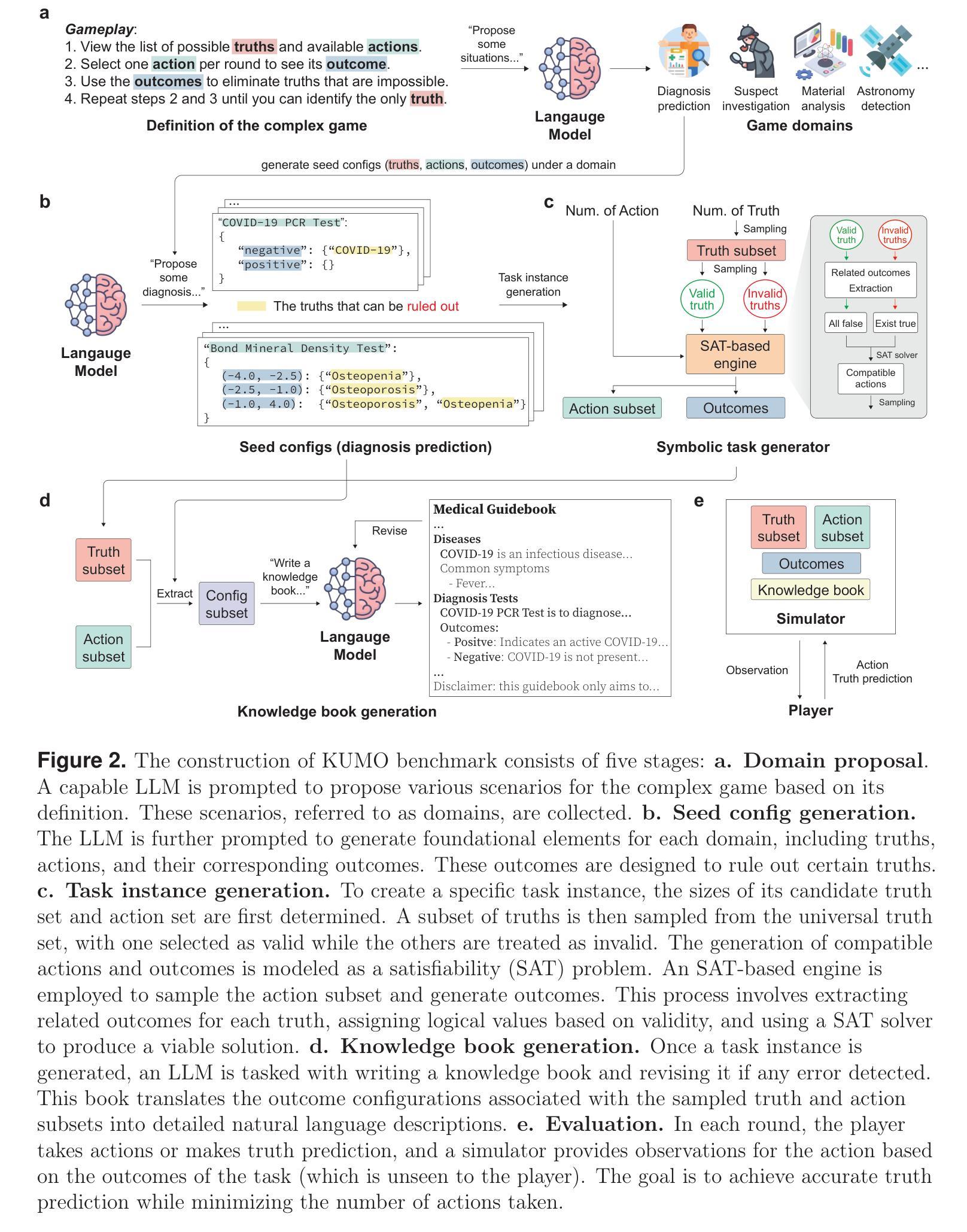
Med-R1: Reinforcement Learning for Generalizable Medical Reasoning in Vision-Language Models
Authors:Yuxiang Lai, Jike Zhong, Ming Li, Shitian Zhao, Xiaofeng Yang
Vision-language models (VLMs) have achieved impressive progress in natural image reasoning, yet their potential in medical imaging remains underexplored. Medical vision-language tasks demand precise understanding and clinically coherent answers, which are difficult to achieve due to the complexity of medical data and the scarcity of high-quality expert annotations. These challenges limit the effectiveness of conventional supervised fine-tuning (SFT) and Chain-of-Thought (CoT) strategies that work well in general domains. To address these challenges, we propose Med-R1, a reinforcement learning (RL)-enhanced vision-language model designed to improve generalization and reliability in medical reasoning. Built on the DeepSeek strategy, Med-R1 adopts Group Relative Policy Optimization (GRPO) to encourage reward-guided learning beyond static annotations. We comprehensively evaluate Med-R1 across eight distinct medical imaging modalities. Med-R1 achieves a 29.94% improvement in average accuracy over its base model Qwen2-VL-2B, and even outperforms Qwen2-VL-72B-a model with 36x more parameters. To assess cross-task generalization, we further evaluate Med-R1 on five question types. Med-R1 outperforms Qwen2-VL-2B by 32.06% in question-type generalization, also surpassing Qwen2-VL-72B. We further explore the thinking process in Med-R1, a crucial component for the success of Deepseek-R1. Our results show that omitting intermediate rationales (No-Thinking-Med-R1) not only improves in-domain and cross-domain generalization with less training, but also challenges the assumption that more reasoning always helps. These findings suggest that in medical VQA, it is not reasoning itself, but its quality and domain alignment, that determine effectiveness. Together, these results highlight that RL improves medical reasoning and generalization, enabling efficient and reliable VLMs for real-world deployment.
视觉语言模型(VLMs)在自然图像推理方面取得了令人印象深刻的进展,但它们在医学成像方面的潜力仍然未被充分探索。医学视觉语言任务需要精确的理解和临床连贯的答案,这很难实现,因为医学数据的复杂性和高质量专家注释的稀缺性。这些挑战限制了传统监督微调(SFT)和思维链(CoT)策略在一般领域中的良好表现。为了解决这些挑战,我们提出了Med-R1,这是一种增强现实学习(RL)的视觉语言模型,旨在提高医学推理中的通用性和可靠性。基于DeepSeek策略,Med-R1采用群组相对策略优化(GRPO),以鼓励超越静态注释的奖励指导学习。我们全面评估了Med-R1在八种不同的医学成像模式上的表现。Med-R1在其基础模型Qwen2-VL-2B上的平均准确率提高了29.94%,甚至超越了具有36倍参数的Qwen2-VL-72B模型。为了评估跨任务泛化能力,我们进一步在五种问题类型上评估了Med-R1。Med-R1在问题类型泛化方面比Qwen2-VL-2B高出32.06%,也超越了Qwen2-VL-79B模型。我们还进一步探索了Med-R1中的思考过程,这是Deepseek-R1成功的重要组成部分。我们的结果表明,省略中间理性(无思考Med-R1)不仅能在域内和跨域泛化方面有所提高且训练更少,而且还挑战了这样一个假设:更多的推理总是有帮助的。这些发现表明,在医学问答中,不是推理本身,而是其质量和领域匹配度决定了其有效性。总之,这些结果强调现实学习改进了医学推理和泛化能力,使视觉语言模型能够高效可靠地用于现实世界部署。
论文及项目相关链接
Summary
本文介绍了在医疗图像推理中,基于强化学习(RL)增强的视觉语言模型Med-R1的应用。该模型旨在提高在医疗推理中的泛化能力和可靠性。通过采用Group Relative Policy Optimization(GRPO)策略,Med-R1在八种不同的医学成像模态上实现了显著的性能提升,并在跨任务泛化方面也表现出优异的表现。此外,研究还发现,高质量的推理和领域对齐对于医疗问答的有效性至关重要。总体而言,强化学习有助于改善医疗推理和泛化能力,使得VLMs在现实世界部署中更加高效和可靠。
Key Takeaways
- Med-R1模型是专为医疗推理设计的强化学习增强的视觉语言模型,旨在提高泛化和可靠性。
- Med-R1采用Group Relative Policy Optimization(GRPO)策略,以提升性能。
- Med-R1在八种不同的医学成像模态上实现了显著的性能提升,平均准确度提高了29.94%。
- Med-R1在跨任务泛化方面表现出优异的表现,在某些问题类型上的表现超过了大型模型。
- 深入研究显示,高质量推理和领域对齐对于医疗问答的有效性至关重要。
- 去除中间推理过程(No-Thinking-Med-R1)可提高域内和跨域泛化能力,并挑战了更多推理总是有帮助的假设。
点此查看论文截图
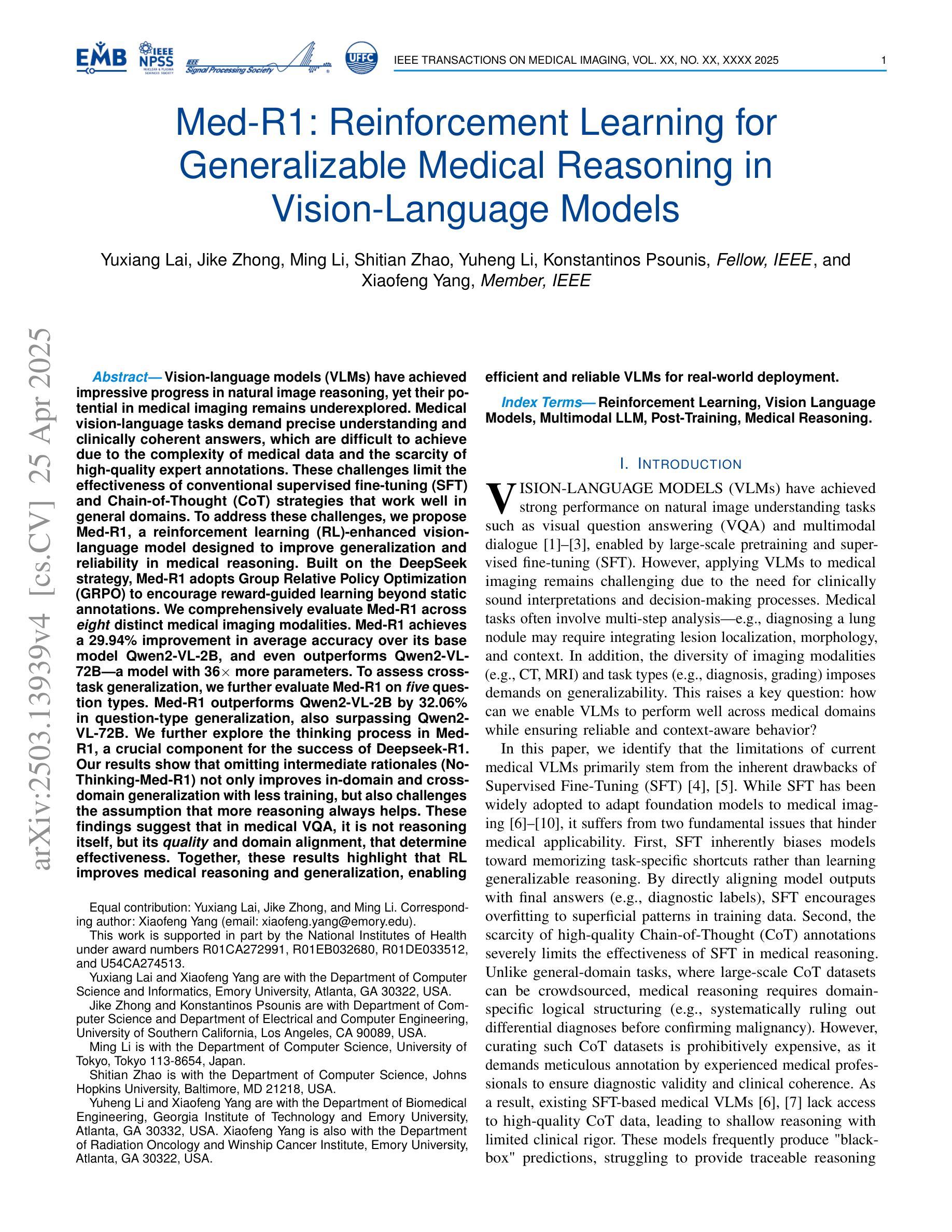
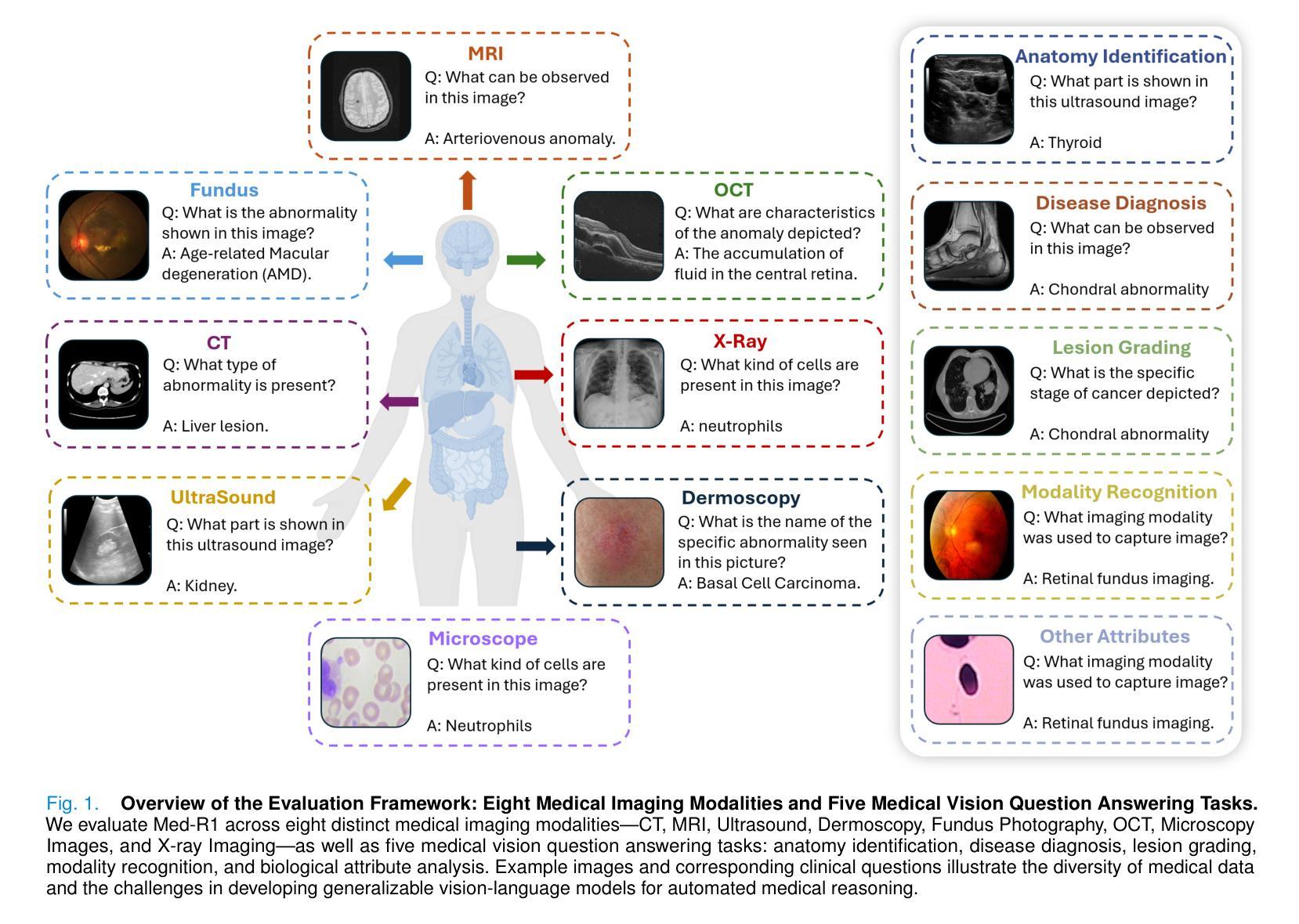
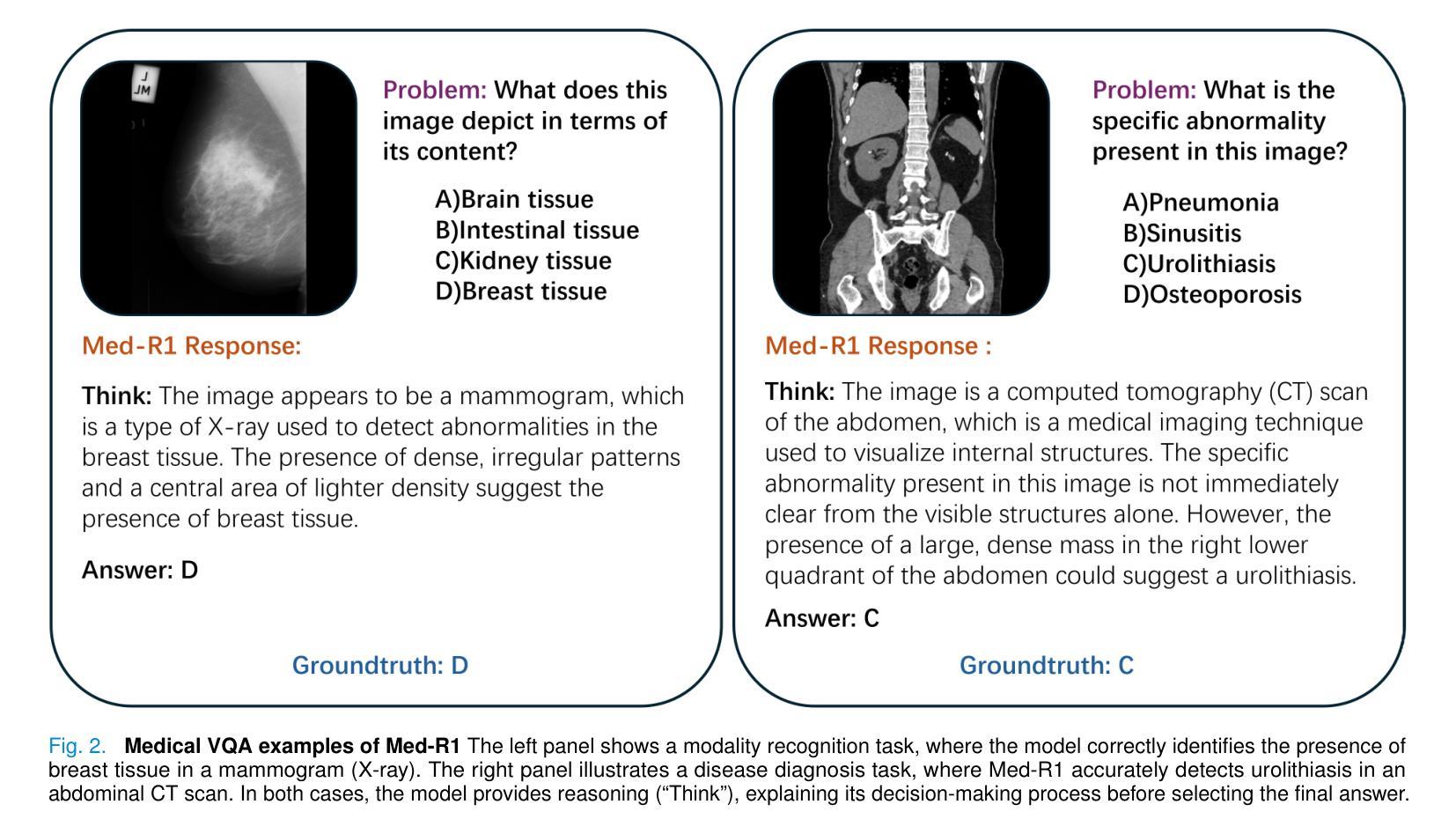
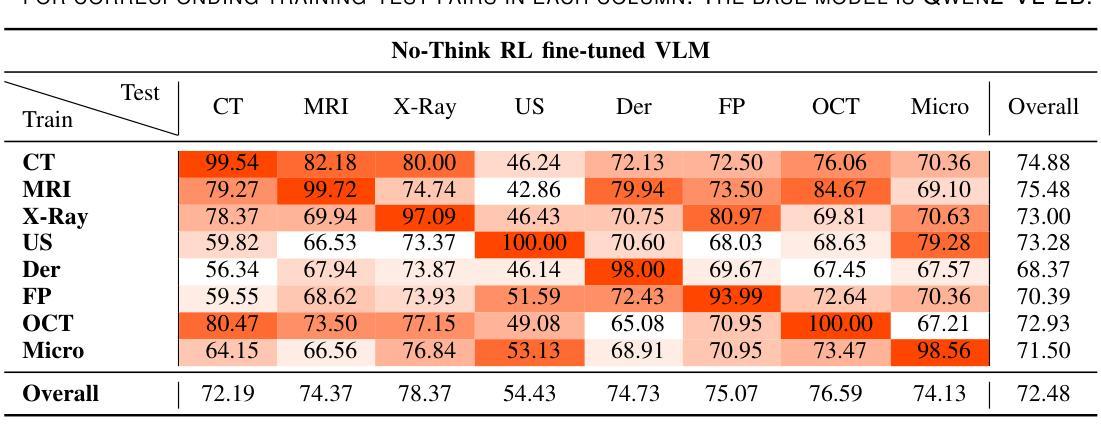
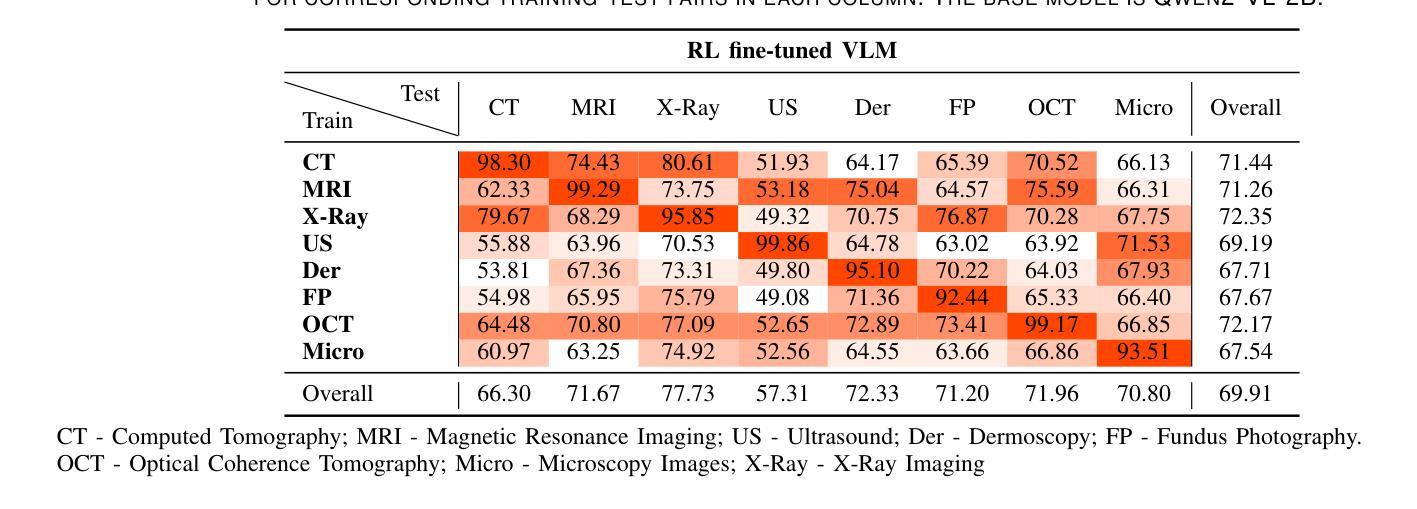
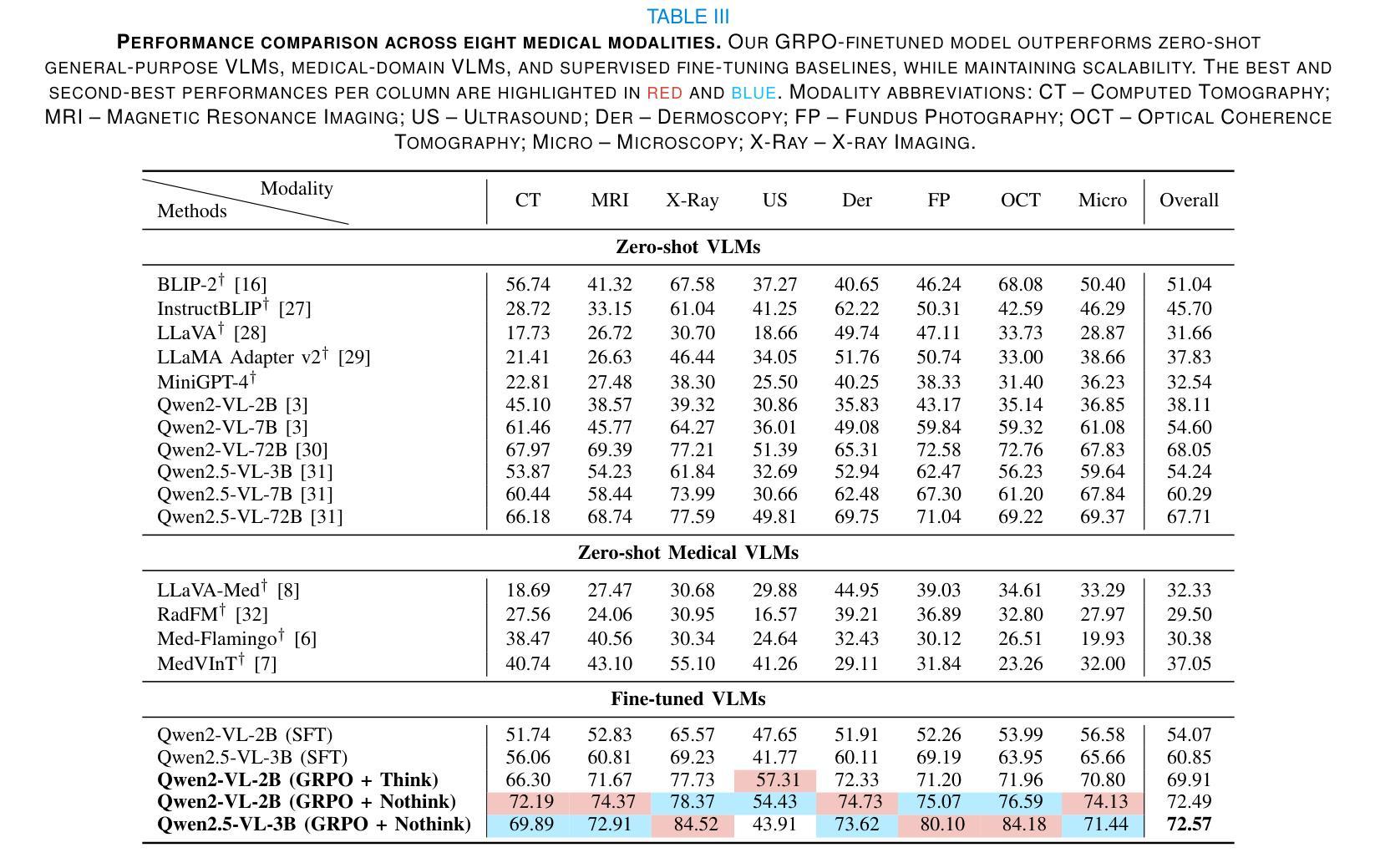
Reinforcement Learning-based Threat Assessment
Authors:Wuzhou Sun, Siyi Li, Qingxiang Zou, Zixing Liao
In some game scenarios, due to the uncertainty of the number of enemy units and the priority of various attributes, the evaluation of the threat level of enemy units as well as the screening has been a challenging research topic, and the core difficulty lies in how to reasonably set the priority of different attributes in order to achieve quantitative evaluation of the threat. In this paper, we innovatively transform the problem of threat assessment into a reinforcement learning problem, and through systematic reinforcement learning training, we successfully construct an efficient neural network evaluator. The evaluator can not only comprehensively integrate the multidimensional attribute features of the enemy, but also effectively combine our state information, thus realizing a more accurate and scientific threat assessment.
在某些游戏场景中,由于敌方单位数量和各属性的优先级的不确定性,敌方单位的威胁等级评估及筛选一直是一个具有挑战性的研究课题,其核也难点在于如何合理设置不同属性的优先级,以实现威胁的定量评估。在本文中,我们创新地将威胁评估问题转化为强化学习问题,并通过系统的强化学习训练,成功构建了一个高效的神经网络评估器。该评估器不仅能全面整合敌方的多维属性特征,还能有效地结合我们的状态信息,从而实现更准确、更科学的威胁评估。
论文及项目相关链接
PDF The research content is not yet complete and requires further supplementation and improvement
Summary:
在某些游戏场景中,评估敌方单位的威胁级别和筛选工作因敌方单位数量的不确定性和各种属性的优先级而充满挑战。本文创新地将威胁评估问题转化为强化学习问题,通过系统的强化学习训练,成功构建了一个高效的神经网络评估器。该评估器能够综合敌方多维属性特征,并结合自身状态信息,实现更准确科学的威胁评估。
Key Takeaways:
- 游戏场景中敌方单位威胁评估具有挑战性,因涉及敌方单位数量的不确定性和属性优先级的设定。
- 本文创新性地采用强化学习方法解决威胁评估问题。
- 通过系统的强化学习训练,成功构建了一个高效的神经网络评估器。
- 评估器能够综合敌方多维属性特征。
- 评估器结合自身状态信息,提高威胁评估的准确性和科学性。
- 强化学习在威胁评估中的应用展示了其在处理复杂问题的潜力。
点此查看论文截图
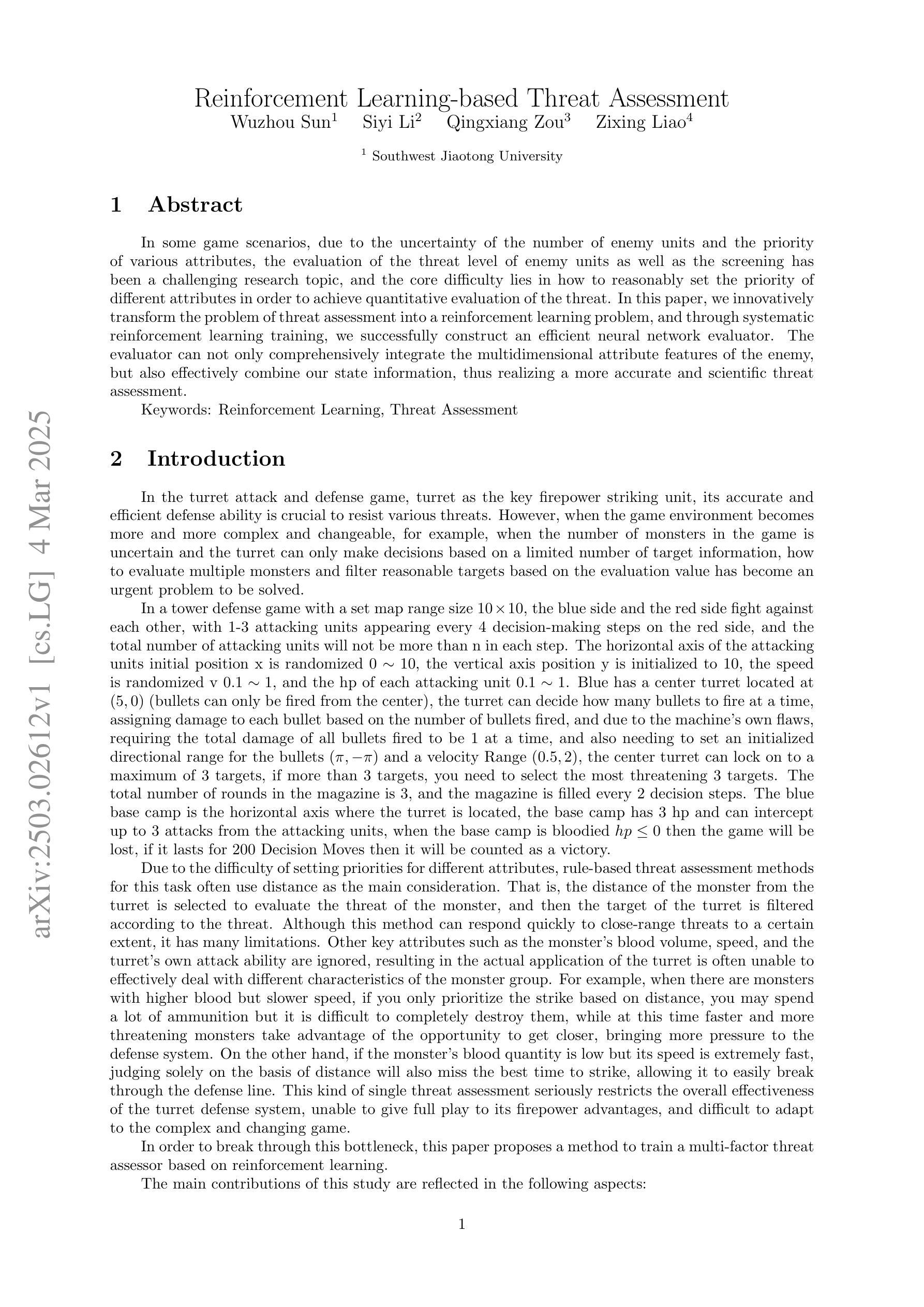
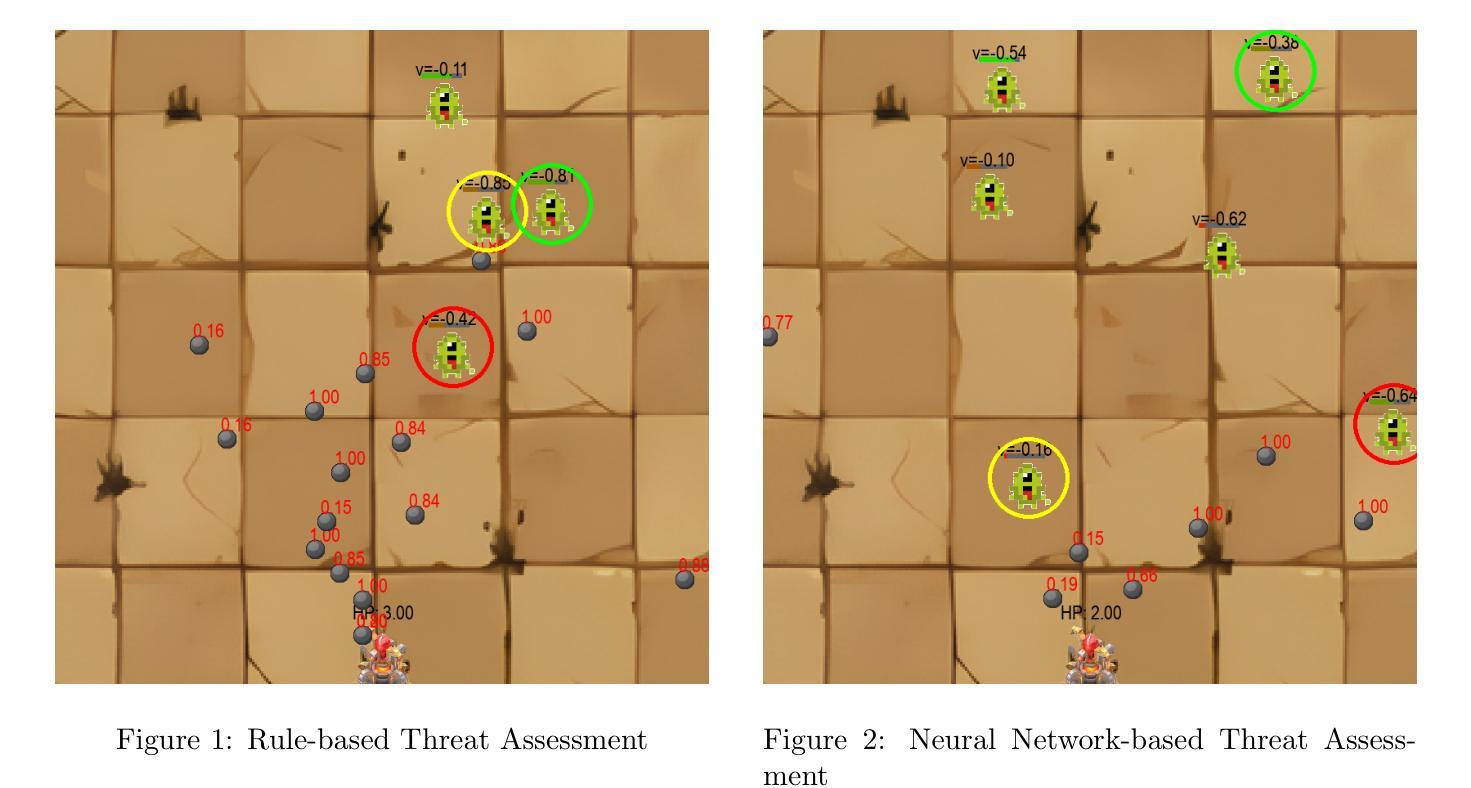
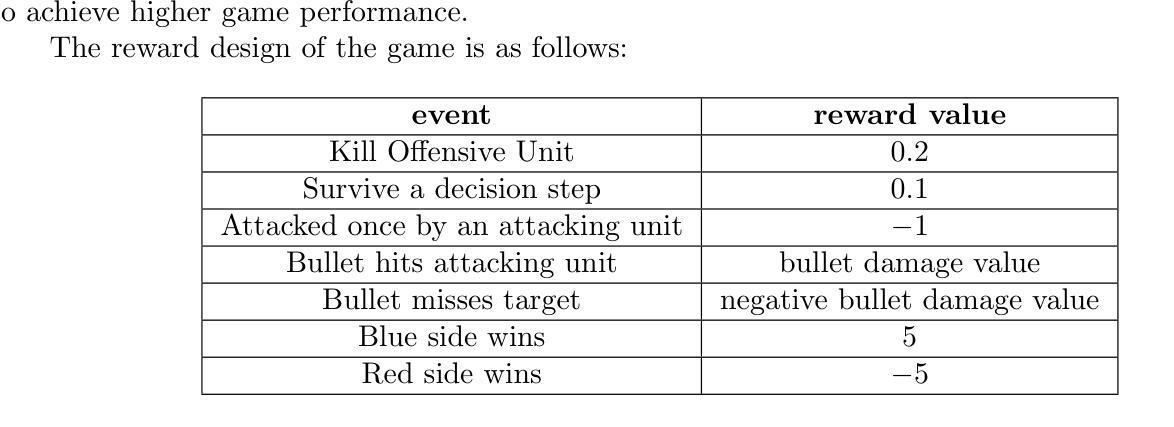
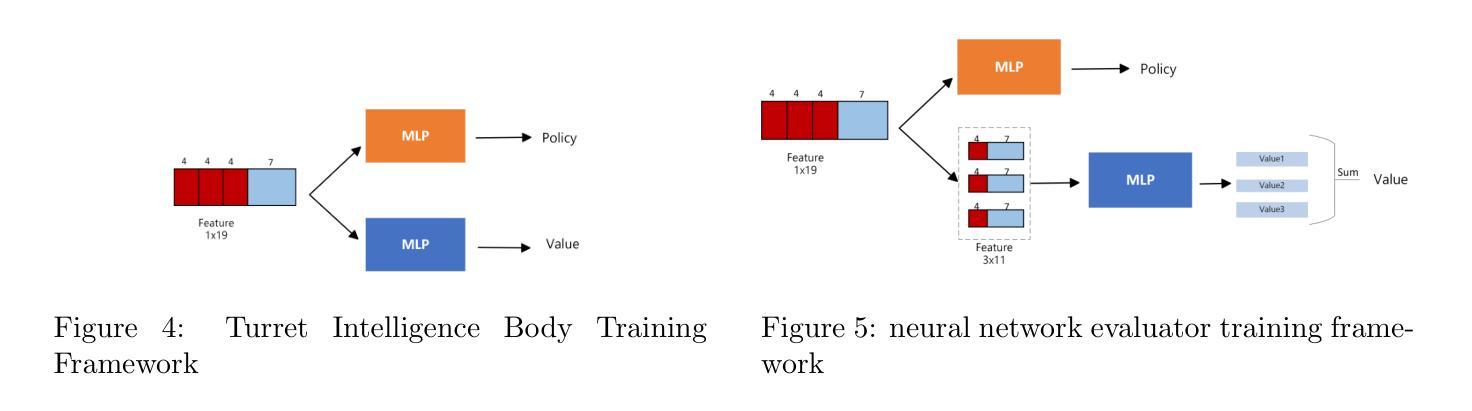
From System 1 to System 2: A Survey of Reasoning Large Language Models
Authors:Zhong-Zhi Li, Duzhen Zhang, Ming-Liang Zhang, Jiaxin Zhang, Zengyan Liu, Yuxuan Yao, Haotian Xu, Junhao Zheng, Pei-Jie Wang, Xiuyi Chen, Yingying Zhang, Fei Yin, Jiahua Dong, Zhiwei Li, Bao-Long Bi, Ling-Rui Mei, Junfeng Fang, Zhijiang Guo, Le Song, Cheng-Lin Liu
Achieving human-level intelligence requires refining the transition from the fast, intuitive System 1 to the slower, more deliberate System 2 reasoning. While System 1 excels in quick, heuristic decisions, System 2 relies on logical reasoning for more accurate judgments and reduced biases. Foundational Large Language Models (LLMs) excel at fast decision-making but lack the depth for complex reasoning, as they have not yet fully embraced the step-by-step analysis characteristic of true System 2 thinking. Recently, reasoning LLMs like OpenAI’s o1/o3 and DeepSeek’s R1 have demonstrated expert-level performance in fields such as mathematics and coding, closely mimicking the deliberate reasoning of System 2 and showcasing human-like cognitive abilities. This survey begins with a brief overview of the progress in foundational LLMs and the early development of System 2 technologies, exploring how their combination has paved the way for reasoning LLMs. Next, we discuss how to construct reasoning LLMs, analyzing their features, the core methods enabling advanced reasoning, and the evolution of various reasoning LLMs. Additionally, we provide an overview of reasoning benchmarks, offering an in-depth comparison of the performance of representative reasoning LLMs. Finally, we explore promising directions for advancing reasoning LLMs and maintain a real-time \href{https://github.com/zzli2022/Awesome-Slow-Reason-System}{GitHub Repository} to track the latest developments. We hope this survey will serve as a valuable resource to inspire innovation and drive progress in this rapidly evolving field.
实现人类水平的智能需要完善从快速直觉系统1到较慢、更慎重的系统2推理的转变。系统1擅长快速启发式决策,而系统2则依赖于逻辑推理以做出更准确的判断和减少偏见。基础大型语言模型(LLM)擅长快速决策制定,但在复杂推理方面缺乏深度,因为它们尚未完全接受系统2思维所具有的逐步分析特征。最近,像OpenAI的o1/o3和DeepSeek的R1等推理LLM已经在数学和编码等领域表现出了专家级的性能,它们模仿了系统2的慎重推理,展示了人类般的认知能力。这篇综述首先简要概述了基础LLM和系统2技术的早期发展的进展,探讨了它们的结合如何为推理LLM铺平道路。接下来,我们讨论如何构建推理LLM,分析它们的特性、支持高级推理的核心方法以及各种推理LLM的演变。此外,我们还概述了推理基准测试,深入比较了代表性推理LLM的性能。最后,我们探讨了推进推理LLM的有前途的方向,并维护一个实时GitHub仓库来跟踪最新进展。我们希望这篇综述能成为这一快速演变领域的宝贵资源,以激发创新和推动进展。
论文及项目相关链接
PDF Slow-thinking, Large Language Models, Human-like Reasoning, Decision Making in AI, AGI
Summary
本文探讨了实现人类智能水平的两个关键系统——快速直觉系统一和慢速深思熟虑系统二之间的过渡。文章指出,基础大型语言模型(LLM)擅长快速决策但缺乏复杂推理能力,仍停留在系统一级的直觉决策上。而新近发展的推理型LLM,如OpenAI的o1/o3和DeepSeek的R1,开始模仿系统二的深思熟虑推理方式,展示出与人类类似的认知能力。本文主要回顾了基础LLM和系统二技术的早期发展,探讨了如何构建推理型LLM、分析他们的特性和核心方法,并比较了不同推理型LLM的性能。同时,本文还展望了未来推动推理型LLM发展的前景。
Key Takeaways
- 实现人类智能水平的过渡需要从直觉决策系统一(快速启发式决策)向深思熟虑决策系统二(基于逻辑推理进行更精确的判断和减少偏见)的转变。
- 基础大型语言模型(LLM)虽然在快速决策方面表现出色,但在复杂推理方面缺乏深度,尚未完全融入系统二的逐步分析特性。
- 新一代推理型LLM如OpenAI的o1/o3和DeepSeek的R1已经展现出专家级别的表现,尤其是在数学和编码等领域,他们模拟了系统二的深思熟虑推理过程并表现出类似人类的认知能力。
- 论文概述了基础LLM和系统二技术的早期发展以及如何将两者结合以创建推理型LLM。
- 论文讨论了如何构建推理型LLM,包括其特性、核心方法和不同推理型LLM的发展演变。
点此查看论文截图
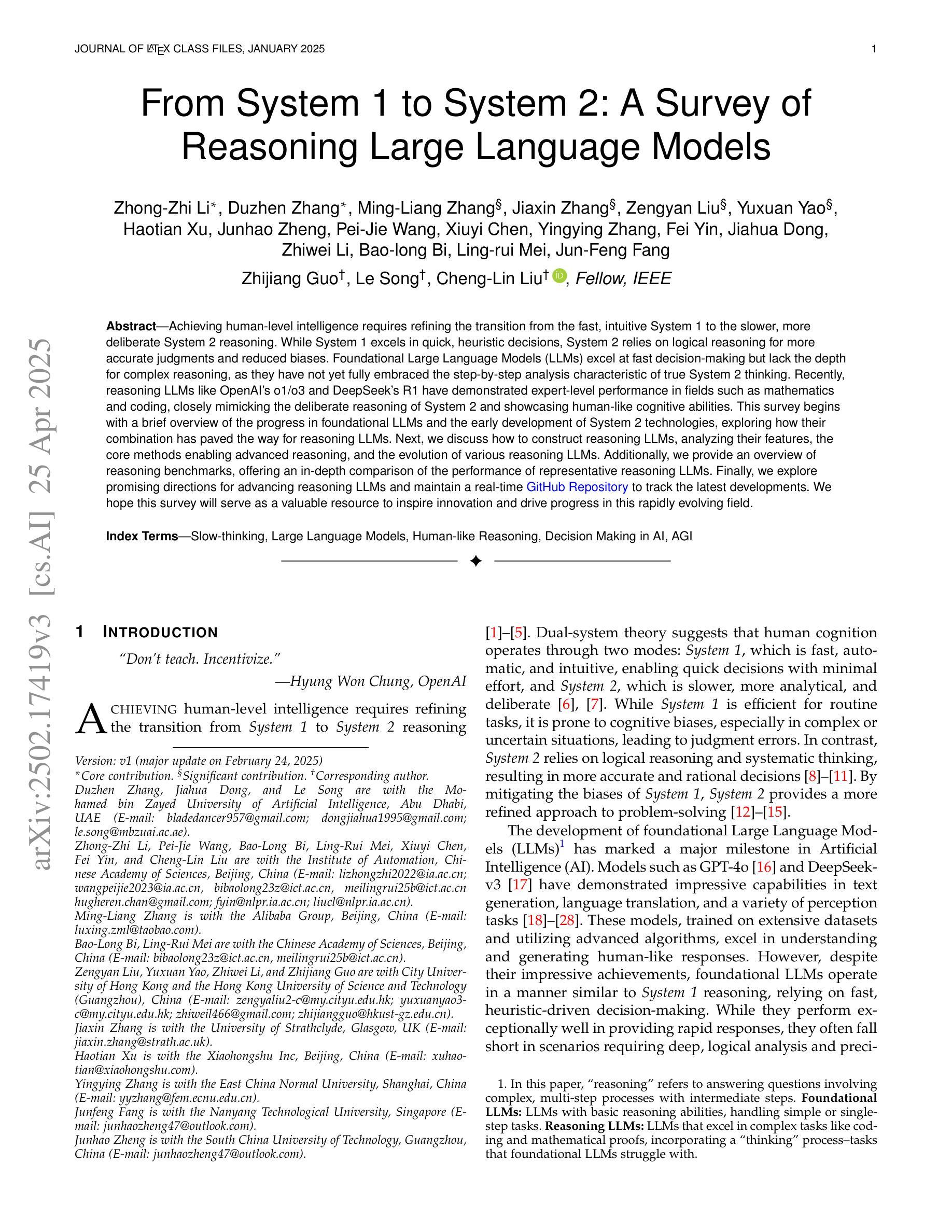
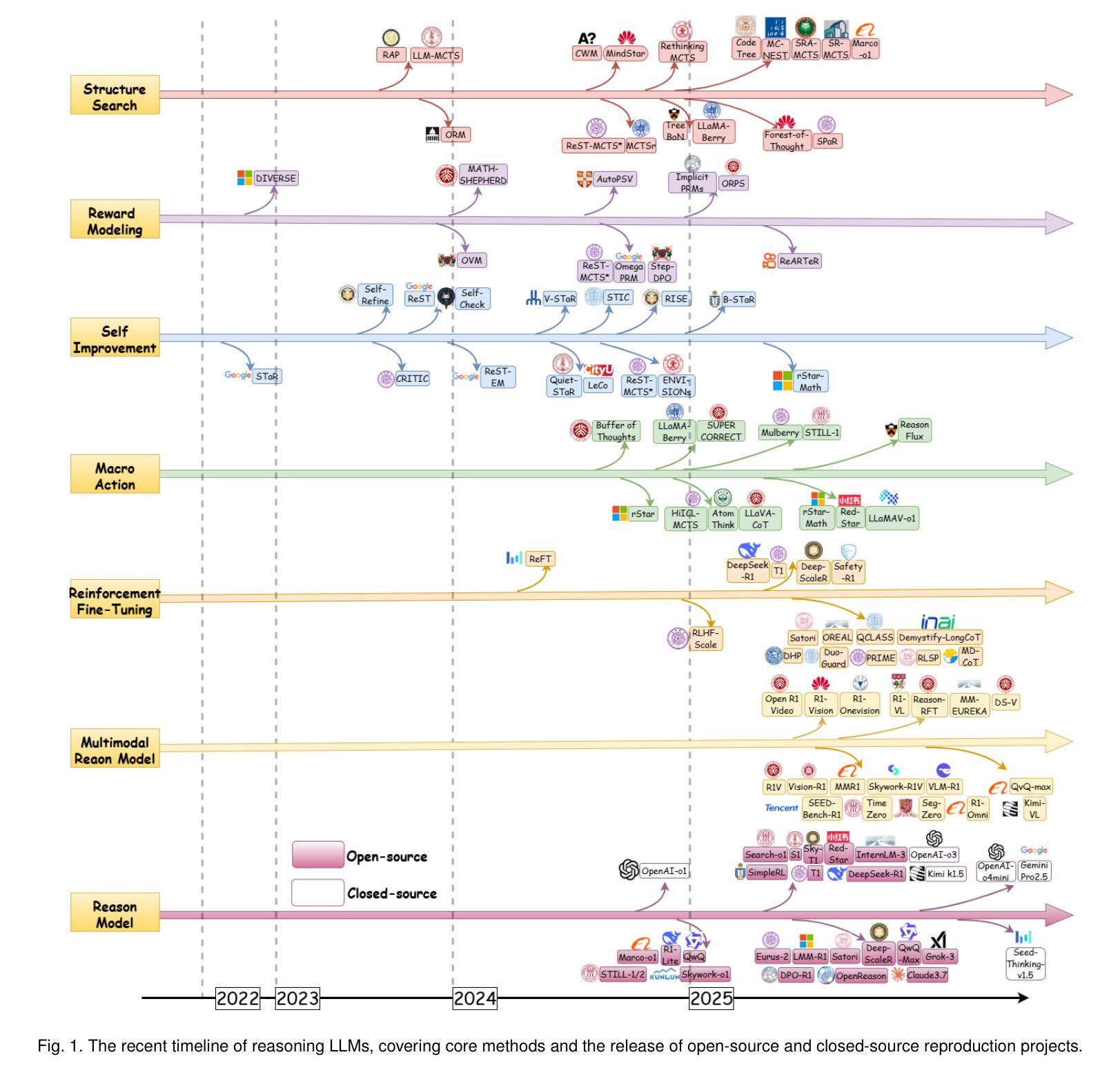
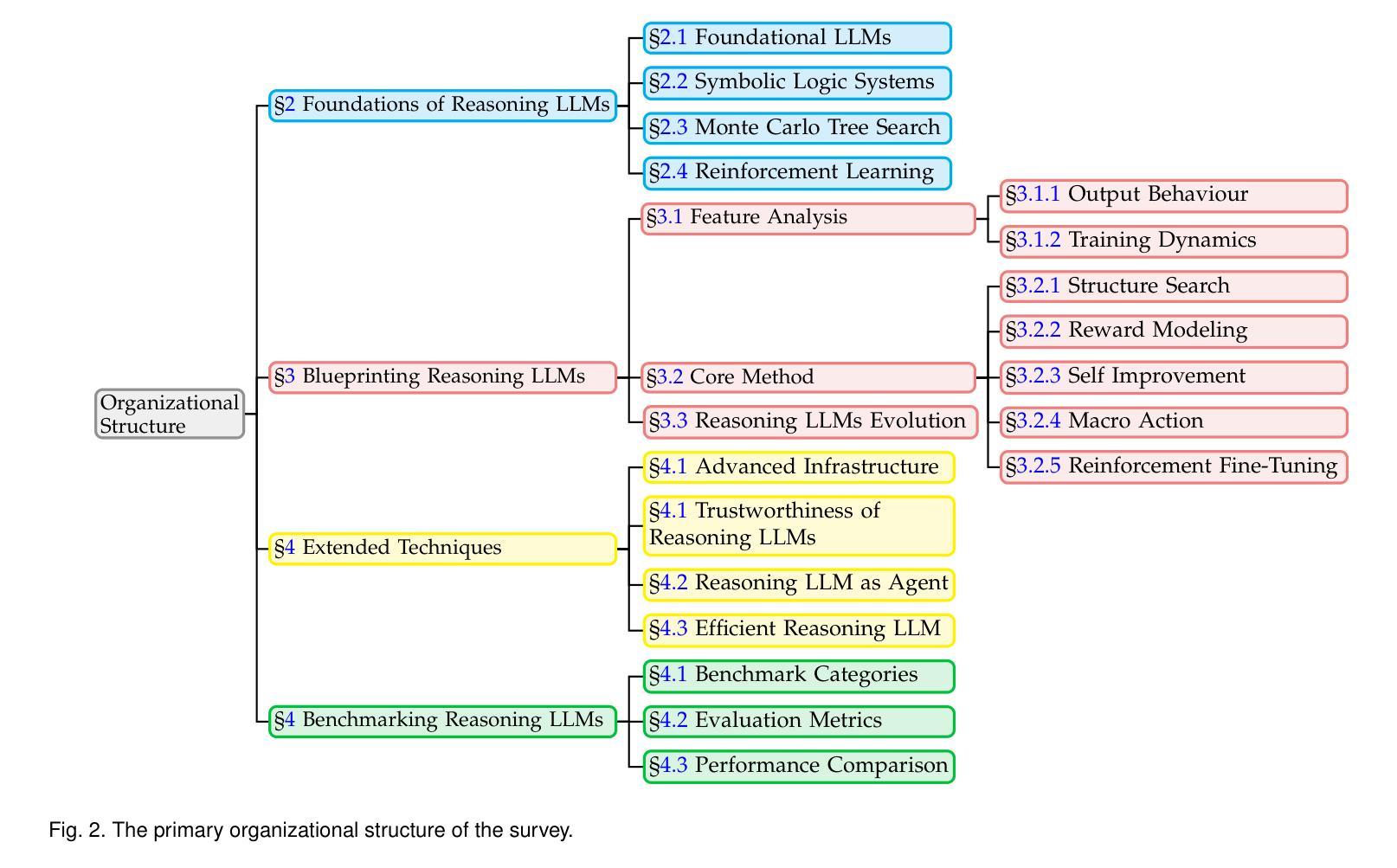
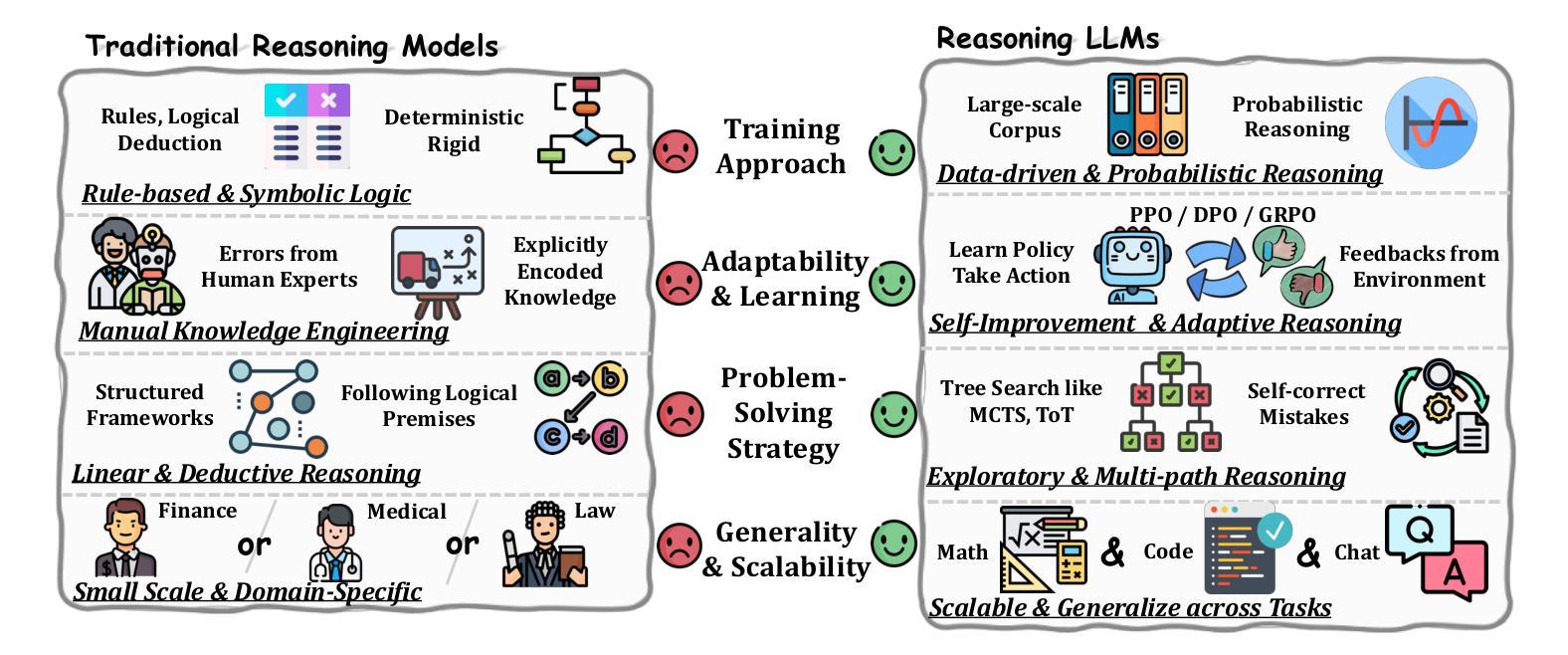
EPO: Explicit Policy Optimization for Strategic Reasoning in LLMs via Reinforcement Learning
Authors:Xiaoqian Liu, Ke Wang, Yongbin Li, Yuchuan Wu, Wentao Ma, Aobo Kong, Fei Huang, Jianbin Jiao, Junge Zhang
Large Language Models (LLMs) have shown impressive reasoning capabilities in well-defined problems with clear solutions, such as mathematics and coding. However, they still struggle with complex real-world scenarios like business negotiations, which require strategic reasoning-an ability to navigate dynamic environments and align long-term goals amidst uncertainty. Existing methods for strategic reasoning face challenges in adaptability, scalability, and transferring strategies to new contexts. To address these issues, we propose explicit policy optimization (EPO) for strategic reasoning, featuring an LLM that provides strategies in open-ended action space and can be plugged into arbitrary LLM agents to motivate goal-directed behavior. To improve adaptability and policy transferability, we train the strategic reasoning model via multi-turn reinforcement learning (RL) using process rewards and iterative self-play, without supervised fine-tuning (SFT) as a preliminary step. Experiments across social and physical domains demonstrate EPO’s ability of long-term goal alignment through enhanced strategic reasoning, achieving state-of-the-art performance on social dialogue and web navigation tasks. Our findings reveal various collaborative reasoning mechanisms emergent in EPO and its effectiveness in generating novel strategies, underscoring its potential for strategic reasoning in real-world applications. Code and data are available at https://github.com/AlibabaResearch/DAMO-ConvAI/tree/main/EPO.
大型语言模型(LLM)在具有明确解决方案的明确定义的问题中表现出了令人印象深刻的推理能力,例如在数学和编码方面。然而,它们在处理复杂的现实世界场景(如商务谈判)时仍面临挑战,这些场景需要策略推理能力,即在动态环境中导航并在不确定性中实现长期目标的能力。现有的策略推理方法面临适应性、可扩展性和将策略转移到新情境的挑战。为了解决这些问题,我们提出了用于策略推理的显式策略优化(EPO),其特点是LLM能在开放式行动空间中提供策略,并且可以插入到任意的LLM代理中以激励目标导向的行为。为了提高适应性和策略可转移性,我们通过多回合强化学习(RL)训练策略推理模型,使用过程奖励和迭代自我对抗,无需预先的监督和微调(SFT)作为初步步骤。在社会和物理领域的实验表明,EPO能够通过增强的策略推理实现长期目标对齐的能力,在社会对话和网页导航任务上达到了最新的性能水平。我们的研究发现了EPO中浮现的各种协作推理机制及其在生成新策略方面的有效性,突显其在现实世界应用中的策略推理潜力。代码和数据集可通过https://github.com/AlibabaResearch/DAMO-ConvAI/tree/main/EPO获取。
论文及项目相关链接
PDF 22 pages, 4 figures
Summary:大型语言模型(LLM)在具有明确解决方案的明确问题中展现出令人印象深刻的推理能力,如数学和编程。然而,在处理需要策略性推理的复杂现实世界场景(如商务谈判)时,它们仍面临挑战。策略性推理要求能够在动态环境中导航并长期目标对齐。为解决现有策略性推理方法的适应性、可扩展性和策略转移问题,我们提出了显式策略优化(EPO)方法。该方法通过多轮强化学习(RL)训练战略推理模型,使用过程奖励和迭代自我游戏,无需监督微调(SFT)作为初步步骤。实验表明,EPO在社会对话和网页导航任务上实现了卓越性能,具有长期目标对齐的能力。其发现了多种协作推理机制和生成新策略的有效性,突显了其在现实世界中策略推理应用的潜力。
Key Takeaways:
- LLM在明确的问题解决中展现出强大的推理能力,但在复杂现实世界场景如商务谈判中的策略性推理方面仍面临挑战。
- 现有的策略推理方法存在适应性、可扩展性和策略转移的问题。
- 提出了显式策略优化(EPO)方法来解决这些问题,该方法结合了大型语言模型和强化学习。
- 通过多轮强化学习训练战略推理模型,使用过程奖励和迭代自我游戏,无需监督微调。
- EPO在多种任务上表现出卓越性能,特别是在社会对话和网页导航任务上。
- 实验发现EPO具有长期目标对齐的能力和多种协作推理机制。
点此查看论文截图
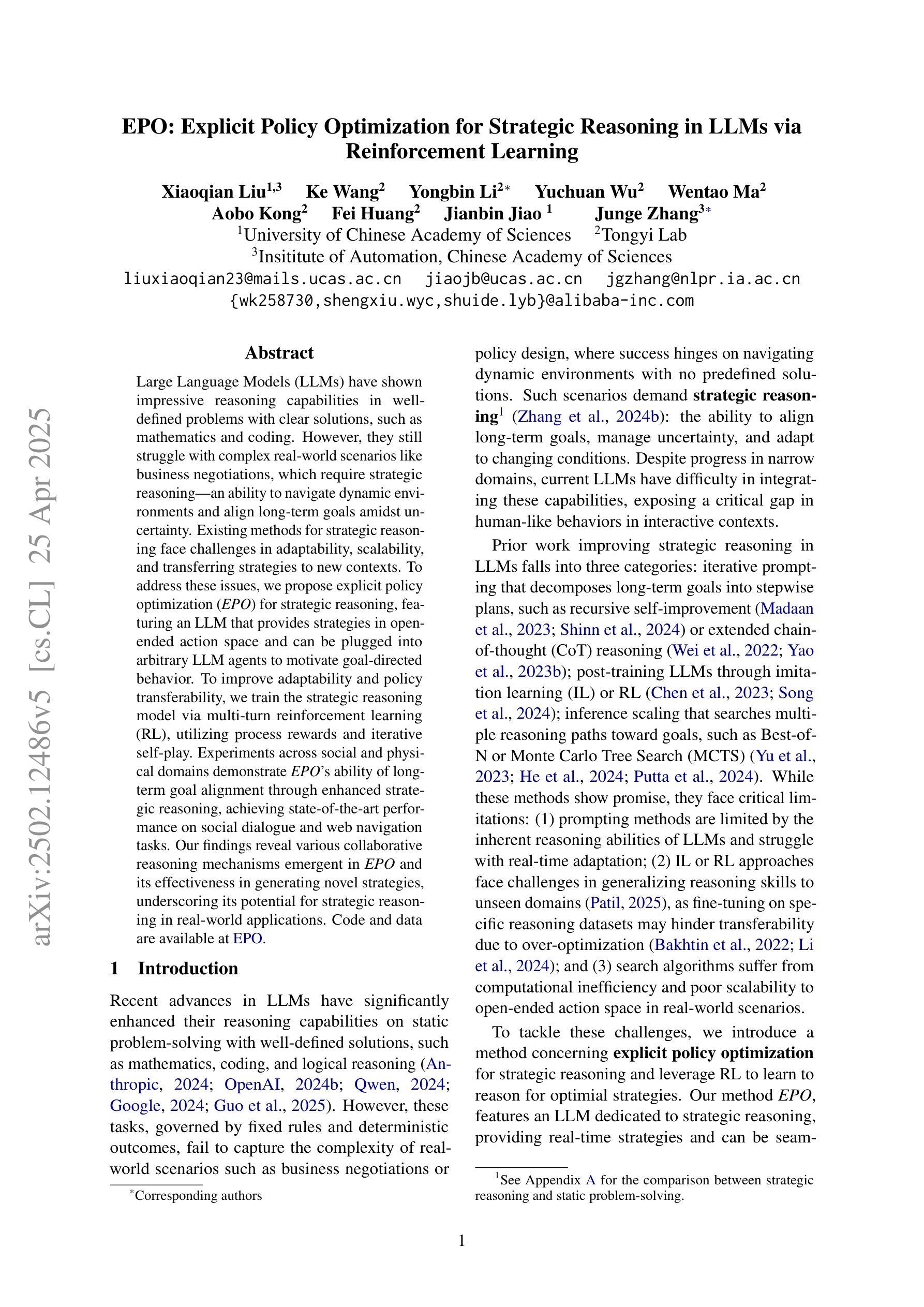
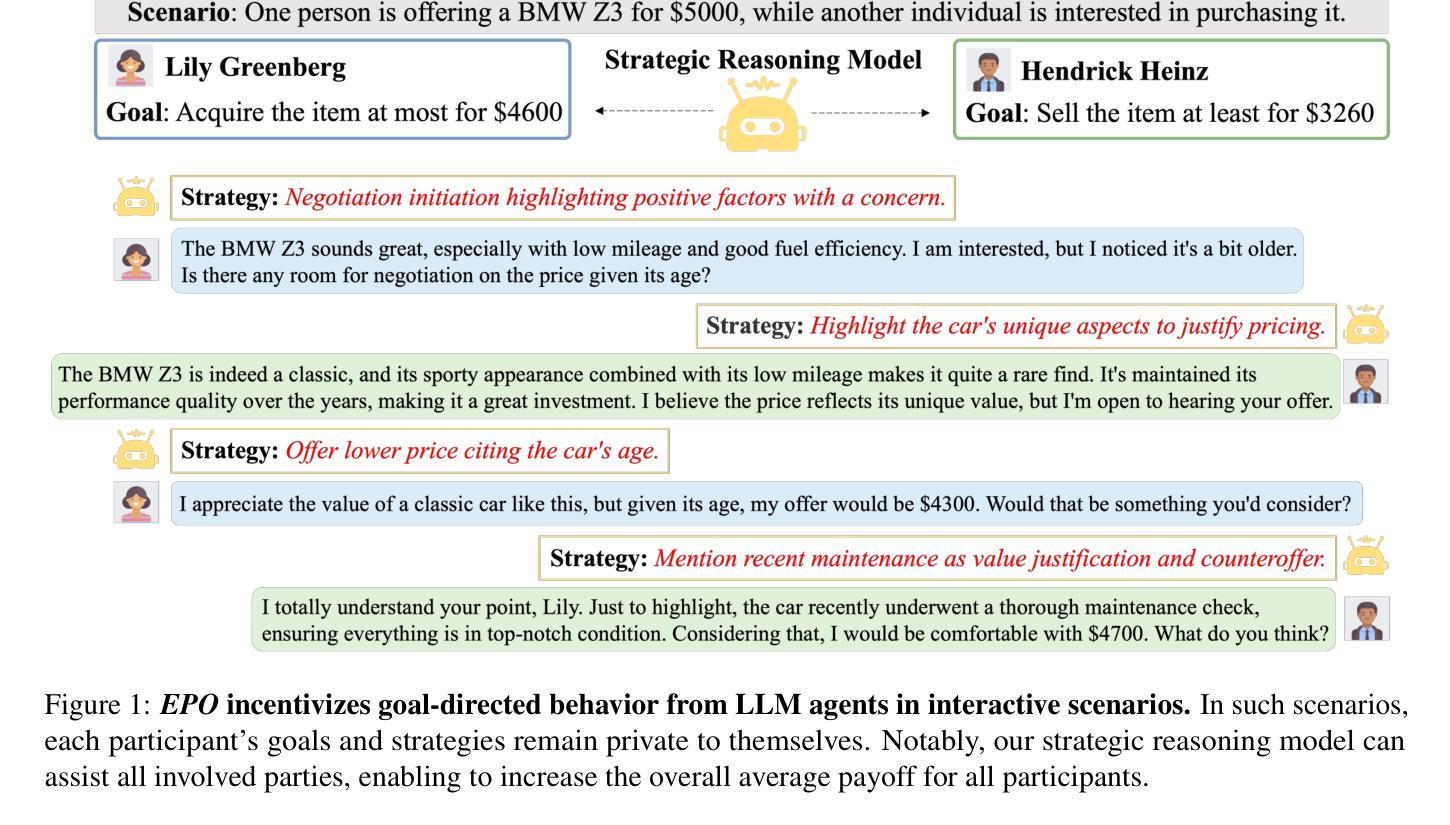
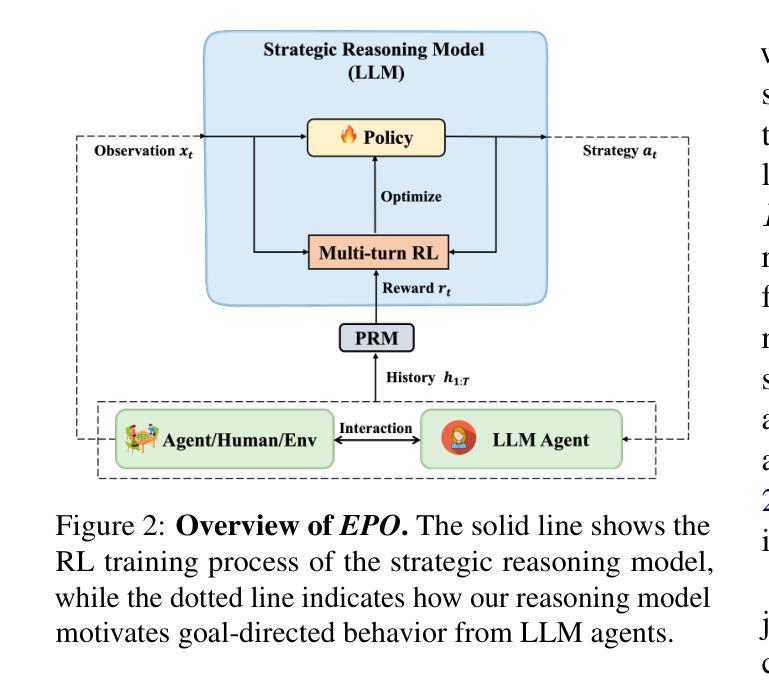
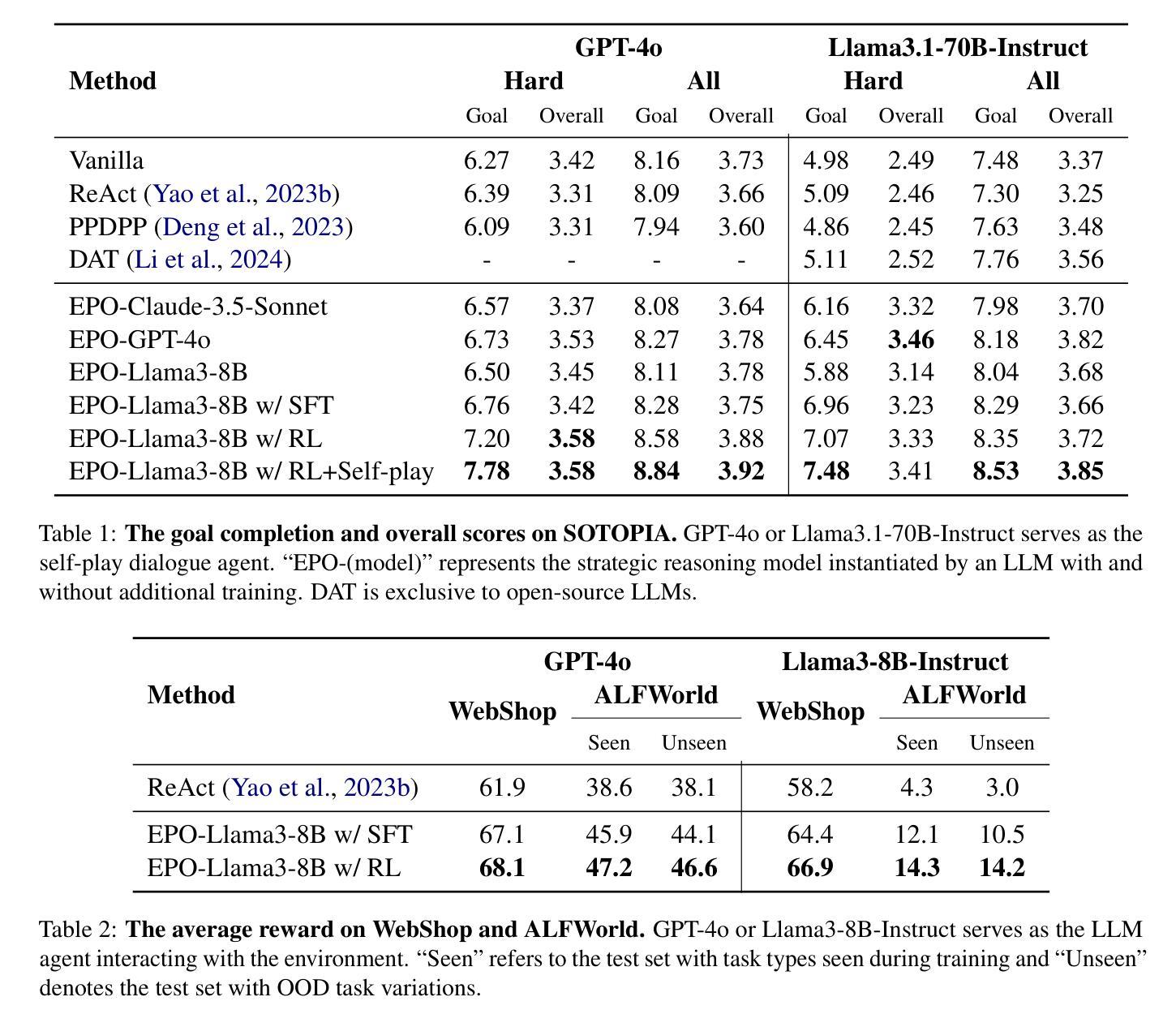