⚠️ 以下所有内容总结都来自于 大语言模型的能力,如有错误,仅供参考,谨慎使用
🔴 请注意:千万不要用于严肃的学术场景,只能用于论文阅读前的初筛!
💗 如果您觉得我们的项目对您有帮助 ChatPaperFree ,还请您给我们一些鼓励!⭐️ HuggingFace免费体验
2025-04-29 更新
Kimi-Audio Technical Report
Authors: KimiTeam, Ding Ding, Zeqian Ju, Yichong Leng, Songxiang Liu, Tong Liu, Zeyu Shang, Kai Shen, Wei Song, Xu Tan, Heyi Tang, Zhengtao Wang, Chu Wei, Yifei Xin, Xinran Xu, Jianwei Yu, Yutao Zhang, Xinyu Zhou, Y. Charles, Jun Chen, Yanru Chen, Yulun Du, Weiran He, Zhenxing Hu, Guokun Lai, Qingcheng Li, Yangyang Liu, Weidong Sun, Jianzhou Wang, Yuzhi Wang, Yuefeng Wu, Yuxin Wu, Dongchao Yang, Hao Yang, Ying Yang, Zhilin Yang, Aoxiong Yin, Ruibin Yuan, Yutong Zhang, Zaida Zhou
We present Kimi-Audio, an open-source audio foundation model that excels in audio understanding, generation, and conversation. We detail the practices in building Kimi-Audio, including model architecture, data curation, training recipe, inference deployment, and evaluation. Specifically, we leverage a 12.5Hz audio tokenizer, design a novel LLM-based architecture with continuous features as input and discrete tokens as output, and develop a chunk-wise streaming detokenizer based on flow matching. We curate a pre-training dataset that consists of more than 13 million hours of audio data covering a wide range of modalities including speech, sound, and music, and build a pipeline to construct high-quality and diverse post-training data. Initialized from a pre-trained LLM, Kimi-Audio is continual pre-trained on both audio and text data with several carefully designed tasks, and then fine-tuned to support a diverse of audio-related tasks. Extensive evaluation shows that Kimi-Audio achieves state-of-the-art performance on a range of audio benchmarks including speech recognition, audio understanding, audio question answering, and speech conversation. We release the codes, model checkpoints, as well as the evaluation toolkits in https://github.com/MoonshotAI/Kimi-Audio.
我们介绍了Kimi-Audio,这是一个开源的音频基础模型,在音频理解、生成和对话方面表现出色。我们详细阐述了构建Kimi-Audio的实践,包括模型架构、数据整理、训练配方、推理部署和评估。具体来说,我们利用12.5Hz的音频标记器,设计了一种新型基于LLM的架构,以连续特征为输入,离散标记为输出,并基于流匹配开发了一种分块流式解标记器。我们整理了一个预训练数据集,包含超过1300万小时的音频数据,涵盖语音、声音和音乐等多种模式,并建立了一条构建高质量和多样化后训练数据的流水线。Kimi-Audio以预训练的LLM为初始值,在音频和文本数据上进行持续预训练,完成了几个精心设计的任务,然后进行微调以支持多种音频相关任务。广泛评估表明,Kimi-Audio在一系列音频基准测试中实现了最先进的性能,包括语音识别、音频理解、音频问答和语音对话。我们在https://github.com/MoonshotAI/Kimi-Audio上发布了代码、模型检查点以及评估工具包。
论文及项目相关链接
Summary:
Kimi-Audio是一个开源的音频基础模型,在音频理解、生成和对话方面表现出色。本文详细介绍了Kimi-Audio的构建实践,包括模型架构、数据收集、训练配方、推理部署和评估。Kimi-Audio利用12.5Hz音频标记器,设计了一种新型LLM架构,以连续特征为输入,离散标记为输出,并基于流匹配开发了一种分块流式去标记器。利用超过1300万小时的音频数据预训练模型,并在多种音频相关任务上进行微调。评估表明,Kimi-Audio在语音识别、音频理解、音频问答和语音对话等音频基准测试上达到了最先进的性能。
Key Takeaways:
- Kimi-Audio是一个开源的音频基础模型,专注于音频理解、生成和对话。
- 采用了12.5Hz的音频标记器,并设计了新型的LLM架构。
- 模型在多种音频任务上表现出色,包括语音识别、音频理解、音频问答和语音对话。
- 使用超过1300万小时的多种模态音频数据进行预训练。
- 采用了流式去标记器来处理音频数据。
- 模型经过连续预训练和微调,以适应不同的音频相关任务。
点此查看论文截图
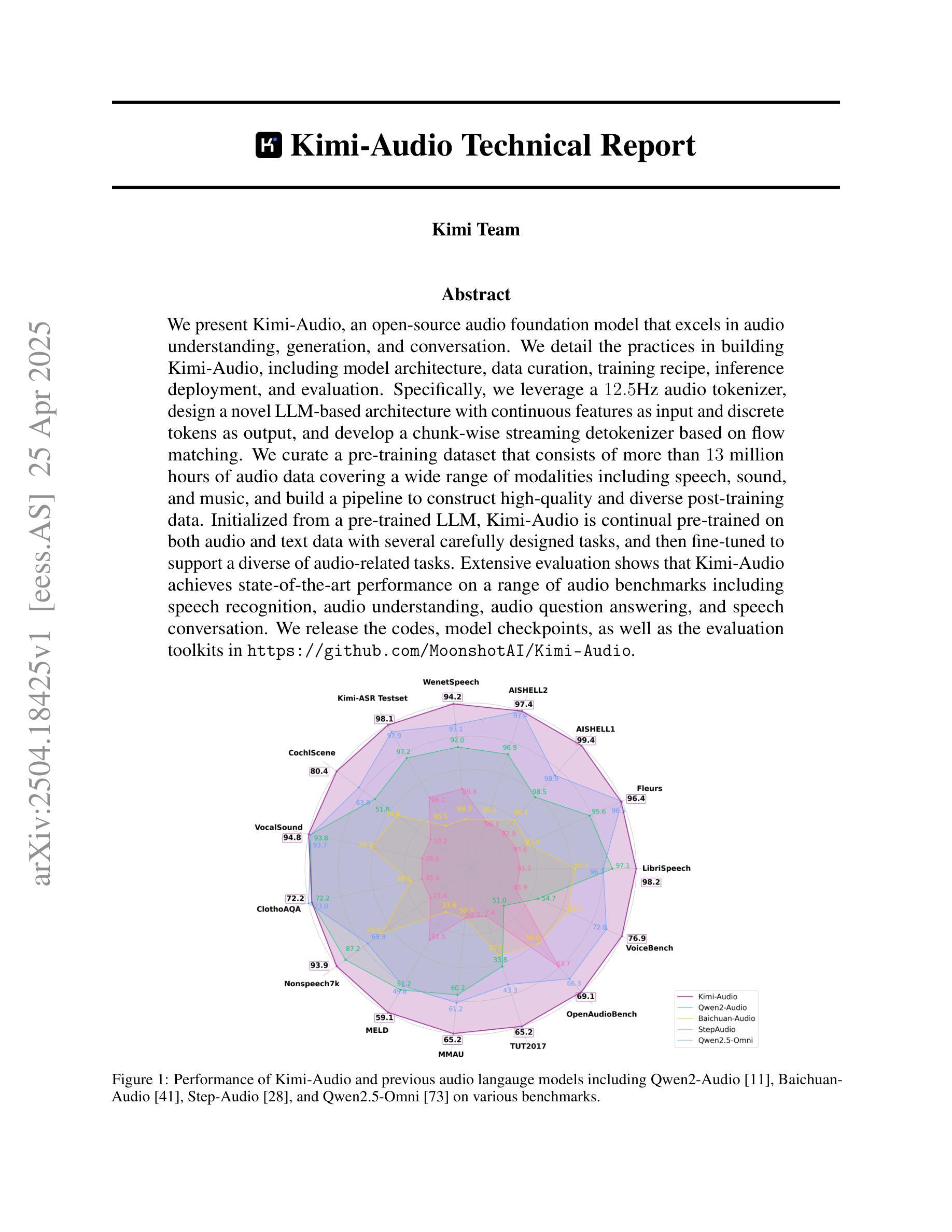
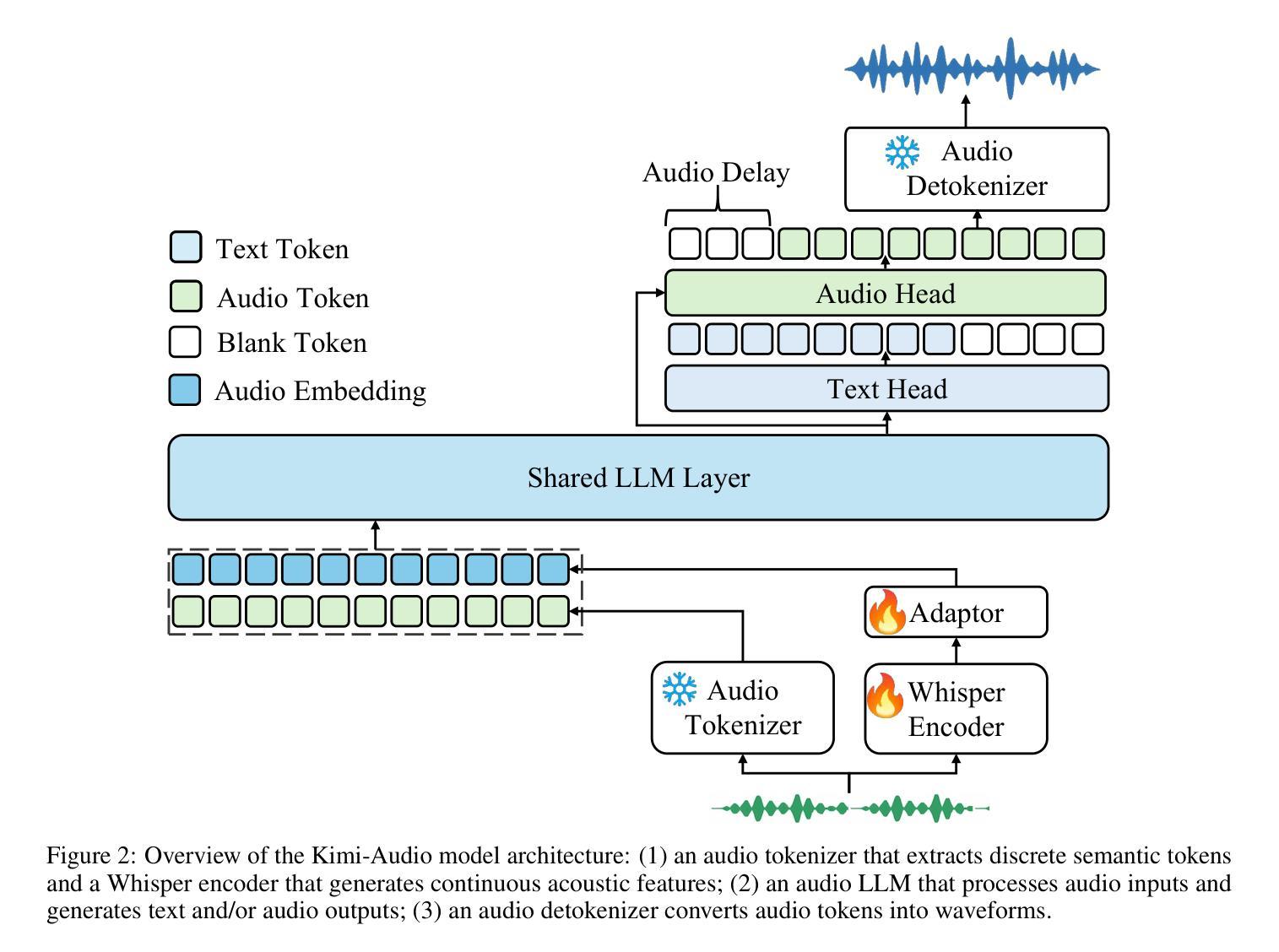
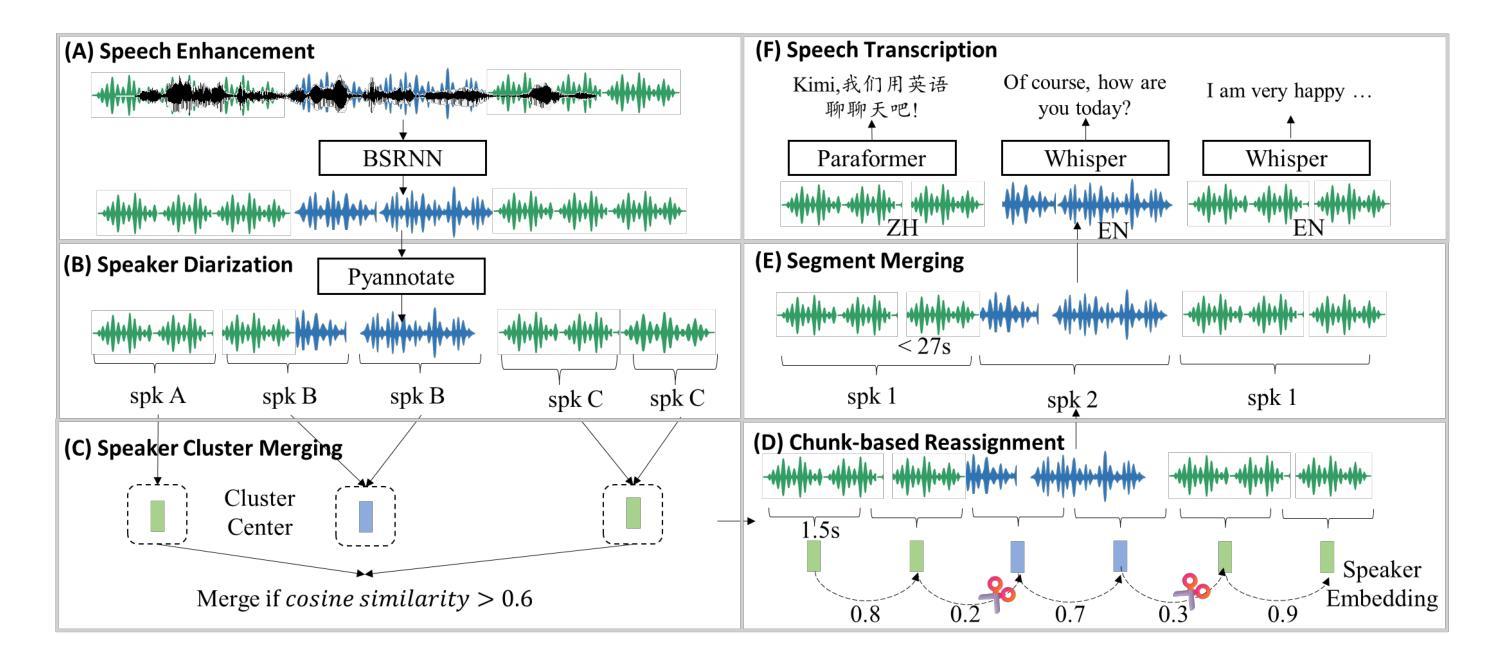
EmoDubber: Towards High Quality and Emotion Controllable Movie Dubbing
Authors:Gaoxiang Cong, Jiadong Pan, Liang Li, Yuankai Qi, Yuxin Peng, Anton van den Hengel, Jian Yang, Qingming Huang
Given a piece of text, a video clip, and a reference audio, the movie dubbing task aims to generate speech that aligns with the video while cloning the desired voice. The existing methods have two primary deficiencies: (1) They struggle to simultaneously hold audio-visual sync and achieve clear pronunciation; (2) They lack the capacity to express user-defined emotions. To address these problems, we propose EmoDubber, an emotion-controllable dubbing architecture that allows users to specify emotion type and emotional intensity while satisfying high-quality lip sync and pronunciation. Specifically, we first design Lip-related Prosody Aligning (LPA), which focuses on learning the inherent consistency between lip motion and prosody variation by duration level contrastive learning to incorporate reasonable alignment. Then, we design Pronunciation Enhancing (PE) strategy to fuse the video-level phoneme sequences by efficient conformer to improve speech intelligibility. Next, the speaker identity adapting module aims to decode acoustics prior and inject the speaker style embedding. After that, the proposed Flow-based User Emotion Controlling (FUEC) is used to synthesize waveform by flow matching prediction network conditioned on acoustics prior. In this process, the FUEC determines the gradient direction and guidance scale based on the user’s emotion instructions by the positive and negative guidance mechanism, which focuses on amplifying the desired emotion while suppressing others. Extensive experimental results on three benchmark datasets demonstrate favorable performance compared to several state-of-the-art methods.
给定一段文本、一个视频片段和参考音频,电影配音任务旨在生成与视频对齐的语音,同时克隆所需的语音。现有方法存在两个主要缺陷:(1)它们难以同时保持视听同步和清晰的发音;(2)它们缺乏表达用户定义情绪的能力。为了解决这些问题,我们提出了EmoDubber,一种情感可控的配音架构,允许用户指定情绪类型和情绪强度,同时满足高质量的唇部同步和发音。具体来说,我们首先设计了一个唇相关韵律对齐(LPA)模块,该模块通过时长级别对比学习来关注唇动和韵律变化之间的内在一致性,以实现合理对齐。然后,我们设计了发音增强(PE)策略,通过高效卷积神经网络融合视频级别的音素序列,以提高语音清晰度。接下来,声音主体适应模块旨在解码声音先验并注入说话者风格嵌入。之后,我们采用基于流的用户情绪控制(FUEC)方法,通过以声音先验为条件的流匹配预测网络来合成波形。在此过程中,FUEC通过正负引导机制根据用户的情绪指令确定梯度方向和引导尺度,侧重于放大所需情绪的同时抑制其他情绪。在三个基准数据集上的广泛实验结果表明,与几种最新方法相比,我们的方法表现出优越的性能。
论文及项目相关链接
PDF Accepted to CVPR 2025
摘要
该文本介绍了电影配音任务的目标,旨在生成与视频对齐的语音,同时克隆所需的语音。现有方法存在两个主要缺陷:一是难以同时保持视听同步和清晰的发音;二是缺乏表达用户定义情感的能力。为解决这些问题,提出了EmoDubber情感可控配音架构,允许用户指定情感类型和情感强度,同时满足高质量唇同步和发音。通过设计唇相关韵律对齐(LPA)和发音增强(PE)策略,实现了语音与视频对齐并提高了语音清晰度。此外,通过用户情绪控制流(FUEC)合成波形,根据用户的情绪指令确定梯度方向和指导规模。实验结果证明了该方法的优越性。
关键见解
- 电影配音任务旨在生成与视频对齐的语音,同时克隆所需的语音。
- 现有方法在视听同步和清晰发音方面存在困难。
- 现有方法缺乏表达用户定义情感的能力。
- 提出了EmoDubber情感可控配音架构,可让用户指定情感类型和强度。
- 通过唇相关韵律对齐(LPA)和发音增强(PE)策略实现语音与视频对齐并提升语音清晰度。
- 通过用户情绪控制流(FUEC)合成波形,基于用户情绪指令进行梯度方向和指导规模的确定。
点此查看论文截图
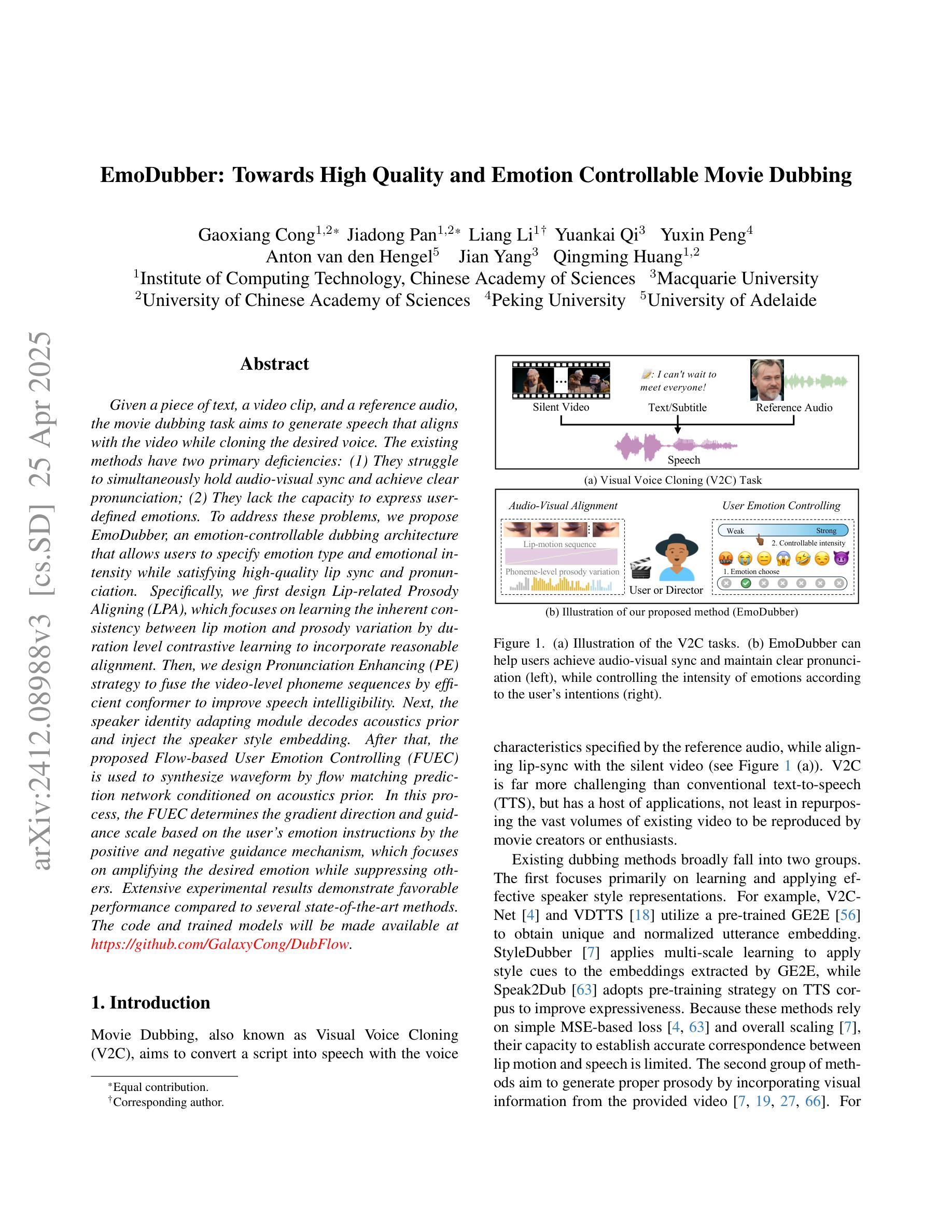
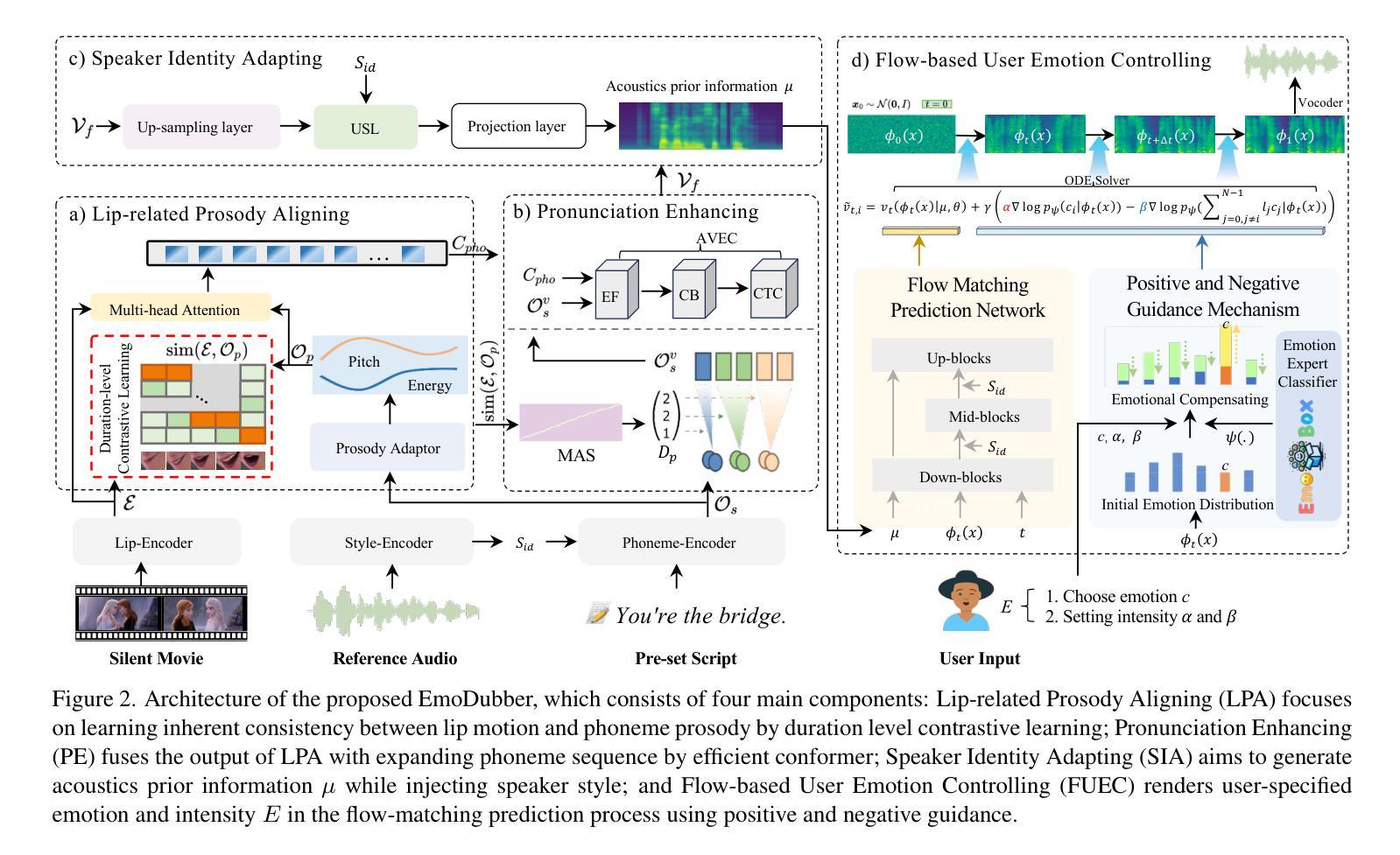
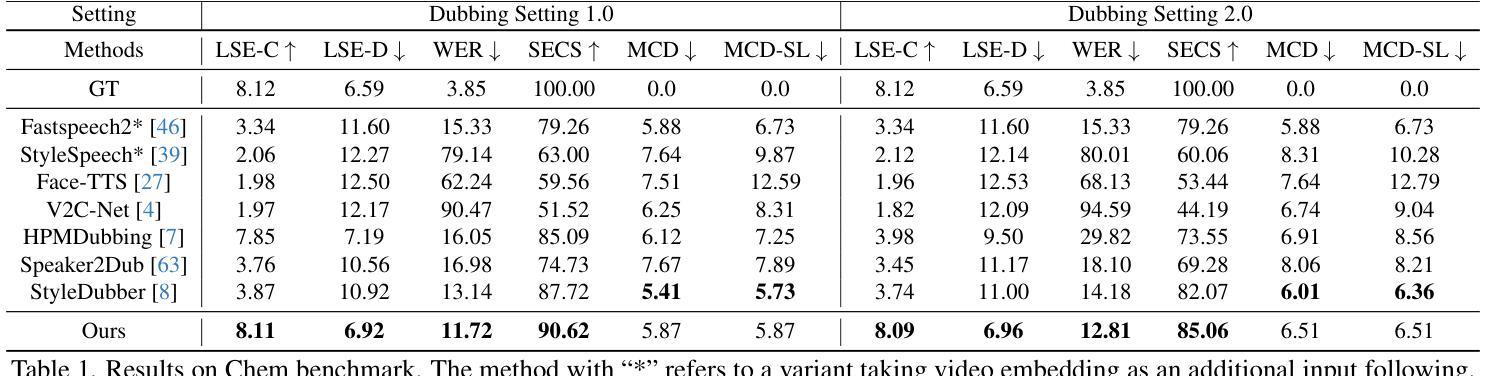
