⚠️ 以下所有内容总结都来自于 大语言模型的能力,如有错误,仅供参考,谨慎使用
🔴 请注意:千万不要用于严肃的学术场景,只能用于论文阅读前的初筛!
💗 如果您觉得我们的项目对您有帮助 ChatPaperFree ,还请您给我们一些鼓励!⭐️ HuggingFace免费体验
2025-04-30 更新
Co-Training with Active Contrastive Learning and Meta-Pseudo-Labeling on 2D Projections for Deep Semi-Supervised Learning
Authors:David Aparco-Cardenas, Jancarlo F. Gomes, Alexandre X. Falcão, Pedro J. de Rezende
A major challenge that prevents the training of DL models is the limited availability of accurately labeled data. This shortcoming is highlighted in areas where data annotation becomes a time-consuming and error-prone task. In this regard, SSL tackles this challenge by capitalizing on scarce labeled and abundant unlabeled data; however, SoTA methods typically depend on pre-trained features and large validation sets to learn effective representations for classification tasks. In addition, the reduced set of labeled data is often randomly sampled, neglecting the selection of more informative samples. Here, we present active-DeepFA, a method that effectively combines CL, teacher-student-based meta-pseudo-labeling and AL to train non-pretrained CNN architectures for image classification in scenarios of scarcity of labeled and abundance of unlabeled data. It integrates DeepFA into a co-training setup that implements two cooperative networks to mitigate confirmation bias from pseudo-labels. The method starts with a reduced set of labeled samples by warming up the networks with supervised CL. Afterward and at regular epoch intervals, label propagation is performed on the 2D projections of the networks’ deep features. Next, the most reliable pseudo-labels are exchanged between networks in a cross-training fashion, while the most meaningful samples are annotated and added into the labeled set. The networks independently minimize an objective loss function comprising supervised contrastive, supervised and semi-supervised loss components, enhancing the representations towards image classification. Our approach is evaluated on three challenging biological image datasets using only 5% of labeled samples, improving baselines and outperforming six other SoTA methods. In addition, it reduces annotation effort by achieving comparable results to those of its counterparts with only 3% of labeled data.
深度学习模型训练面临的一个主要挑战是准确标注数据的有限可用性。在数据标注成为耗时且易出错的任务的领域,这一缺陷尤为突出。在这方面,SSL通过利用稀缺的标签数据和大量的无标签数据来解决这一挑战;然而,最新技术通常依赖于预训练特征和大型验证集来学习分类任务的有效表示。此外,减少的有标签数据集通常是随机采样的,忽视了更有信息量的样本的选择。在这里,我们提出了active-DeepFA方法,它有效地结合了对比学习、基于教师-学生的元伪标签和主动学习,用于在标记数据稀缺、无标签数据丰富的情况下训练非预训练的CNN架构进行图像分类。它将DeepFA集成到协同训练设置中,实现两个合作网络,以减轻伪标签的确认偏见。该方法首先从一组减少的有标签样本开始,通过监督对比学习预热网络。之后,在定期的epoch间隔上,对网络深度特征的2D投影执行标签传播。然后,最可靠的伪标签在网络之间进行交叉训练方式的交换,同时最有意义的样本被标注并添加到有标签集合中。网络独立地最小化目标损失函数,包括监督对比、监督和半监督损失成分,以增强图像分类的表示。我们的方法仅在三个具有挑战性的生物图像数据集上使用5%的有标签样本进行评估,提高了基线性能并超越了其他六种最新技术。此外,通过仅使用3%的有标签数据,它实现了与同类方法相当的结果,减少了标注工作量。
论文及项目相关链接
PDF Submitted to Journal of the Brazilian Computer Society (JBCS) [https://journals-sol.sbc.org.br]
摘要
在深度学习任务中,缺乏准确标注的数据是主要挑战之一。特别是在数据标注耗时且易出错的领域,半监督学习(SSL)通过利用有限的有标签数据和大量的无标签数据来解决这一问题。然而,当前最前沿的方法通常依赖于预训练特征和大型验证集来学习有效的表示来进行分类任务。此外,有限的有标签数据集通常是随机采样的,忽略了更具信息量的样本的选择。本文提出了active-DeepFA方法,它有效地结合了对比学习(CL)、基于教师-学生的元伪标签和主动学习(AL),用于训练图像分类场景中标注数据稀缺、无标注数据丰富的非预训练卷积神经网络(CNN)架构。它将DeepFA集成到协同训练设置中,实现两个合作网络,以减轻伪标签的确证偏见。该方法首先用有限的有标签样本对网络进行预热,然后进行监督对比学习。随后在定期的epoch间隔上,对网络深层特征的二维投影执行标签传播。最可靠的伪标签以跨训练的方式在网络之间进行交换,同时最有意义的样本被标注并添加到有标签集中。网络独立地最小化包含监督对比、监督和半监督损失组件的目标损失函数,以改善图像分类的表示。我们的方法在仅使用5%有标签样本的三个具有挑战性的生物图像数据集上进行了评估,提高了基线并超越了其他六种最前沿方法。此外,它仅在3%的有标签数据上取得了与同行相当的结果,减少了标注工作。
关键见解
- 深度学习中有限的有标签数据是主要的挑战之一,尤其是在数据标注耗时且易出错的领域。
- 半监督学习利用有限的有标签数据和大量的无标签数据来解决这一挑战。
- 当前最前沿的方法通常依赖于预训练特征和大型验证集来进行分类任务。
- active-DeepFA方法结合了对比学习、基于教师-学生的元伪标签和主动学习来优化图像分类的训练过程。
- DeepFA被集成到一个协同训练设置中,实现两个合作网络来减轻伪标签的确证偏见。
- 该方法通过最小化包含多种损失组件的目标损失函数来增强图像分类的表示能力。
点此查看论文截图
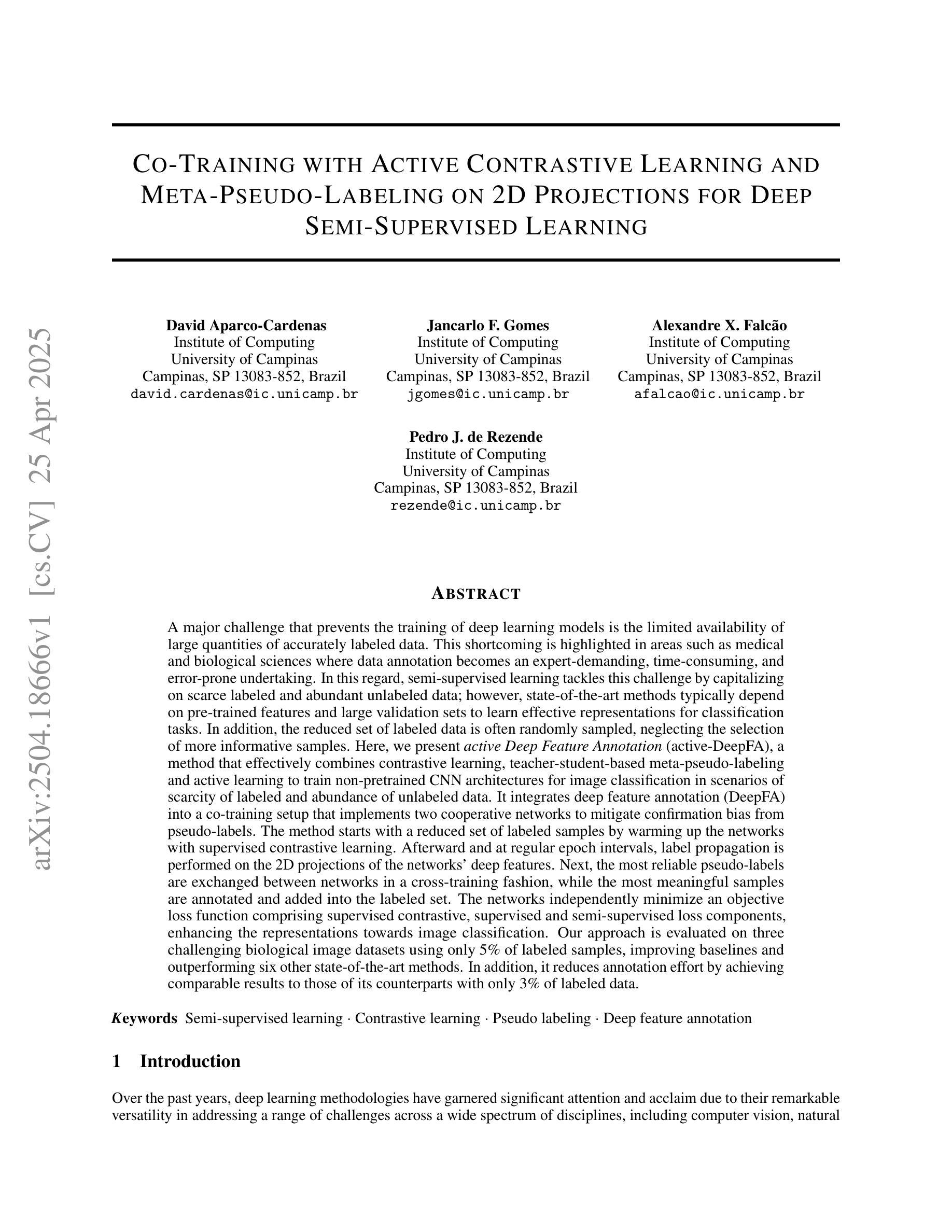
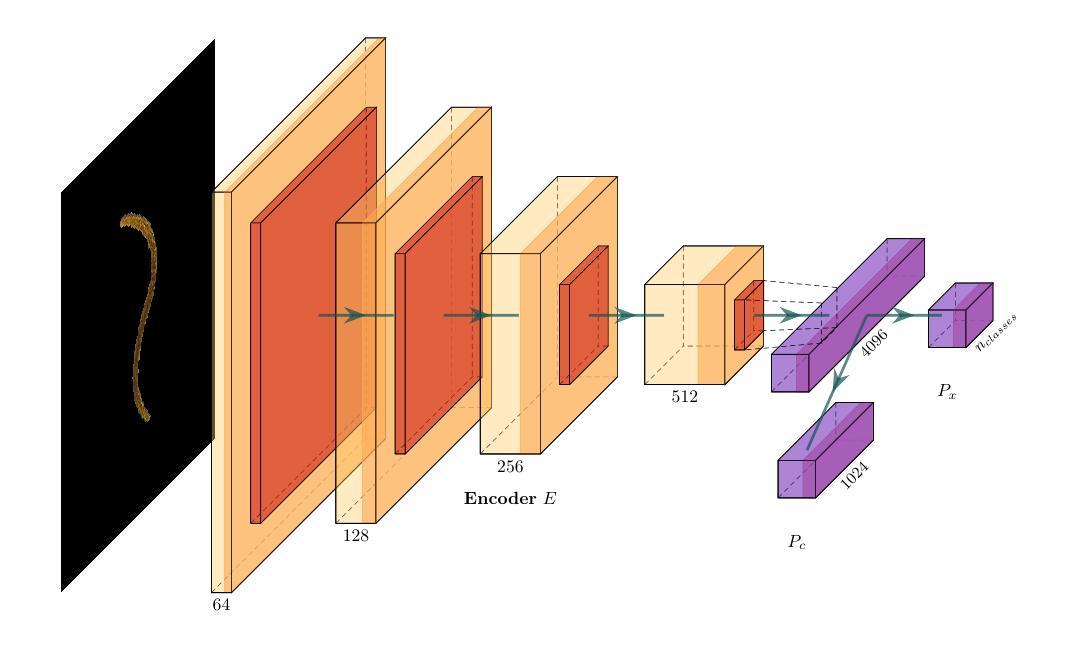
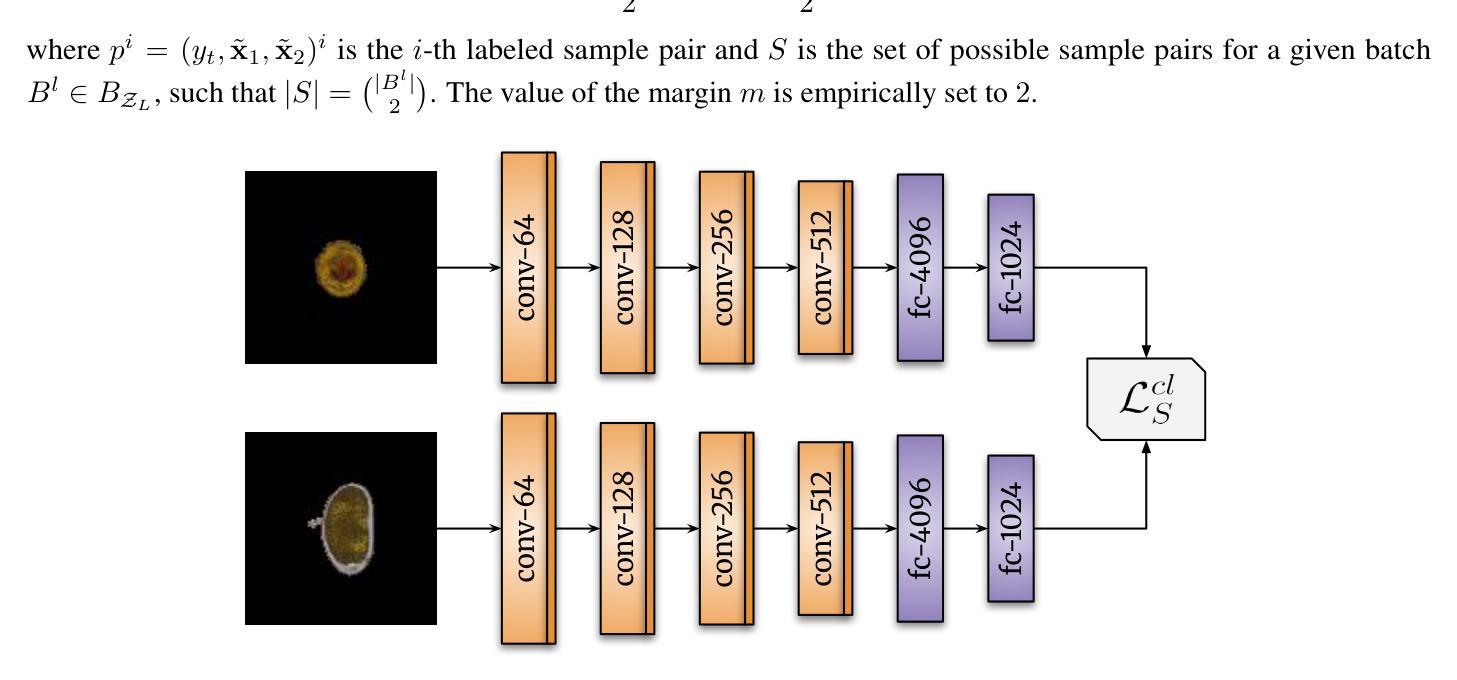
NCL-CIR: Noise-aware Contrastive Learning for Composed Image Retrieval
Authors:Peng Gao, Yujian Lee, Zailong Chen, Hui zhang, Xubo Liu, Yiyang Hu, Guquang Jing
Composed Image Retrieval (CIR) seeks to find a target image using a multi-modal query, which combines an image with modification text to pinpoint the target. While recent CIR methods have shown promise, they mainly focus on exploring relationships between the query pairs (image and text) through data augmentation or model design. These methods often assume perfect alignment between queries and target images, an idealized scenario rarely encountered in practice. In reality, pairs are often partially or completely mismatched due to issues like inaccurate modification texts, low-quality target images, and annotation errors. Ignoring these mismatches leads to numerous False Positive Pair (FFPs) denoted as noise pairs in the dataset, causing the model to overfit and ultimately reducing its performance. To address this problem, we propose the Noise-aware Contrastive Learning for CIR (NCL-CIR), comprising two key components: the Weight Compensation Block (WCB) and the Noise-pair Filter Block (NFB). The WCB coupled with diverse weight maps can ensure more stable token representations of multi-modal queries and target images. Meanwhile, the NFB, in conjunction with the Gaussian Mixture Model (GMM) predicts noise pairs by evaluating loss distributions, and generates soft labels correspondingly, allowing for the design of the soft-label based Noise Contrastive Estimation (NCE) loss function. Consequently, the overall architecture helps to mitigate the influence of mismatched and partially matched samples, with experimental results demonstrating that NCL-CIR achieves exceptional performance on the benchmark datasets.
图像检索(CIR)旨在使用多模态查询找到目标图像,该查询结合了图像和修改文本以精确定位目标。虽然最近的CIR方法显示出了一定的潜力,但它们主要集中在通过数据增强或模型设计探索查询对(图像和文本)之间的关系。这些方法通常假设查询和目标图像之间的完美对齐,这在实践中很少遇到。实际上,由于不准确的修改文本、低质量的目标图像和注释错误等问题,配对经常会部分或完全不匹配。忽略这些不匹配会导致大量称为数据集噪声对的误报配对(FFPs),导致模型过度拟合,并最终降低其性能。为了解决此问题,我们提出了用于CIR的噪声感知对比学习(NCL-CIR),它包括两个关键组件:权重补偿块(WCB)和噪声对过滤器块(NFB)。WCB与各种权重图相结合,可以确保多模态查询和目标图像的令牌表示更加稳定。同时,NFB结合高斯混合模型(GMM)通过评估损失分布来预测噪声对,并相应生成软标签,从而设计出基于软标签的噪声对比估计(NCE)损失函数。因此,整体架构有助于减轻不匹配和部分匹配样本的影响,实验结果表明,NCL-CIR在基准数据集上取得了出色的性能。
论文及项目相关链接
PDF Has been accepted by ICASSP2025
Summary
该文本介绍了组成图像检索(CIR)中使用噪声感知对比学习(NCL-CIR)的重要性及其两大关键组件:权重补偿块(WCB)和噪声对过滤器块(NFB)。NCL-CIR能有效解决现实场景中查询与目标的图像之间存在的部分或完全不匹配问题,通过减轻噪声对模型的影响,提高模型的性能。
Key Takeaways
- Composed Image Retrieval (CIR) 使用多模态查询(结合图像和修改文本)来定位目标图像。
- 现有CIR方法主要探索查询对之间的关系,假设查询和目标图像完美对齐,但这在现实场景中很少发生。
- 现实中的不匹配问题,如不准确修改文本、低质量目标图像和注释错误,会导致数据集中的噪声对(False Positive Pair, FFPs)。
- 噪声感知对比学习(NCL-CIR)旨在解决这一问题,其包含两个关键组件:Weight Compensation Block (WCB) 和 Noise-pair Filter Block (NFB)。
- WCB 结合不同的权重图,确保多模态查询和目标图像的令牌表示更加稳定。
- NFB 结合高斯混合模型(GMM)预测噪声对,并据此生成软标签,用于设计基于软标签的噪声对比估计(NCE)损失函数。
点此查看论文截图
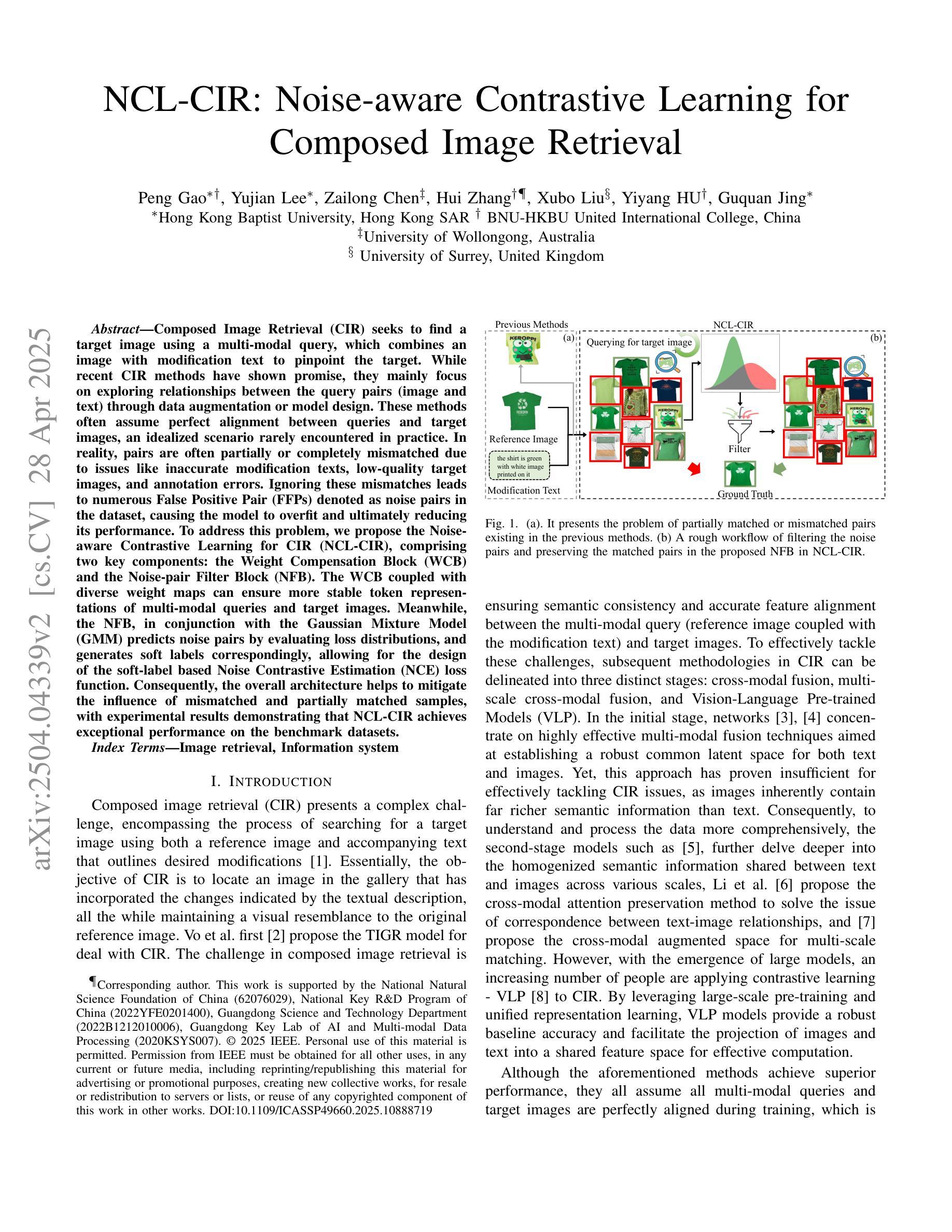
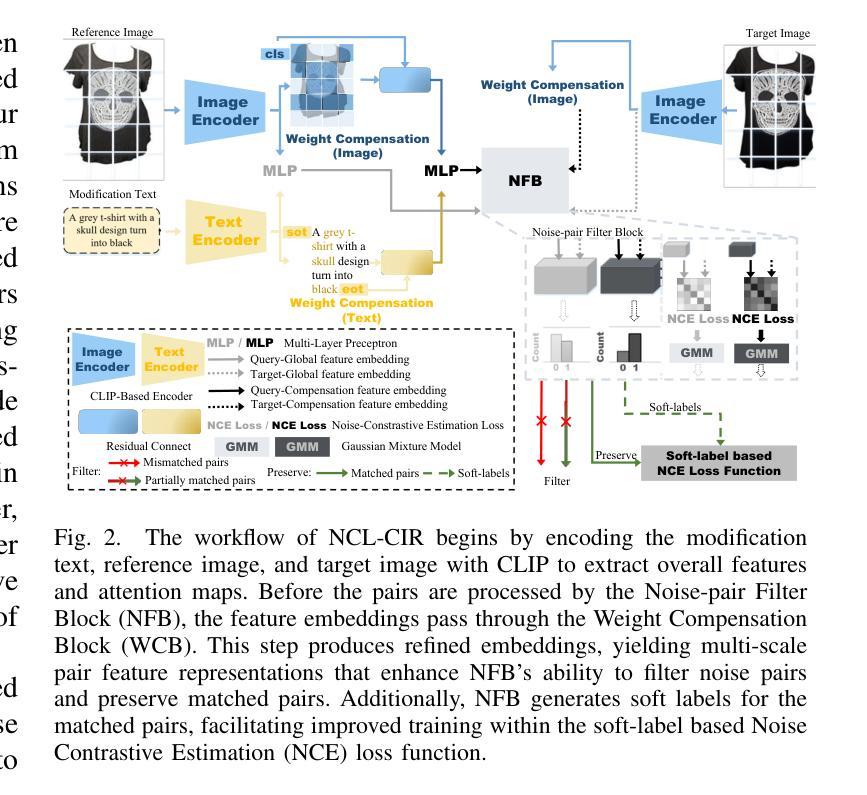
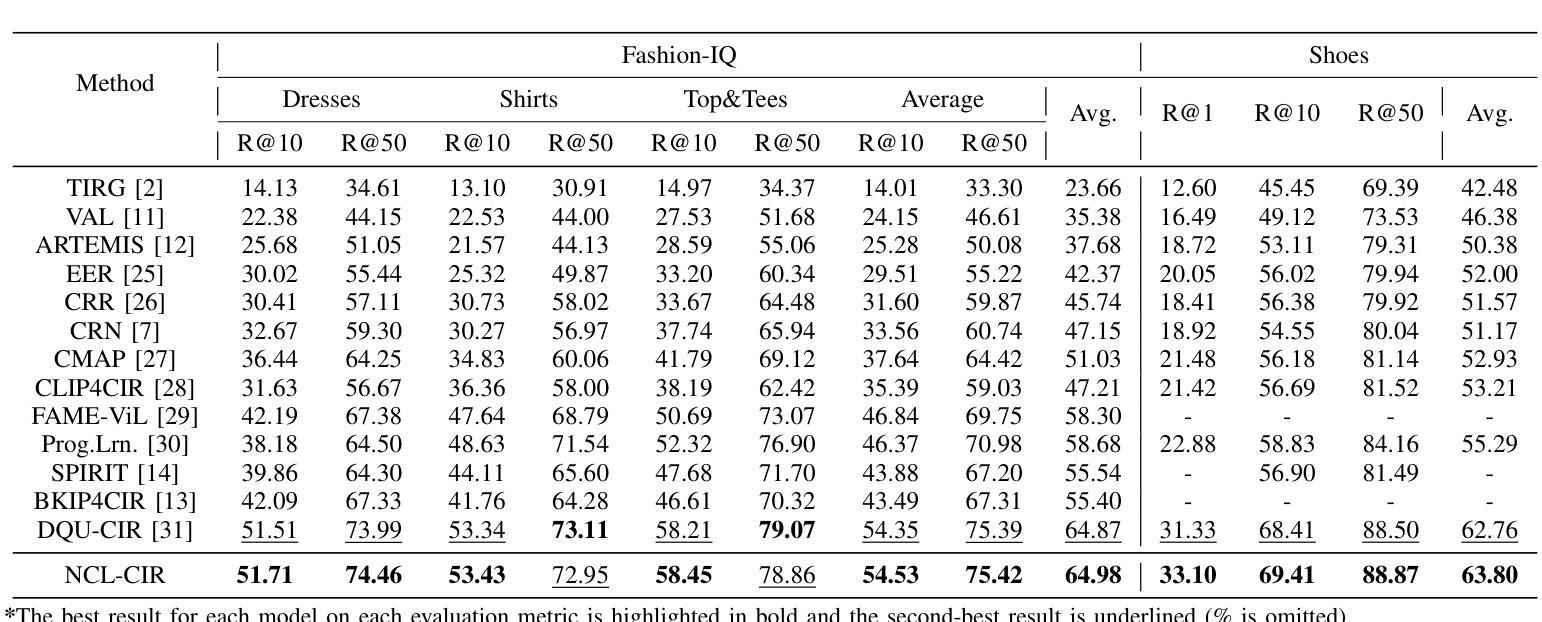
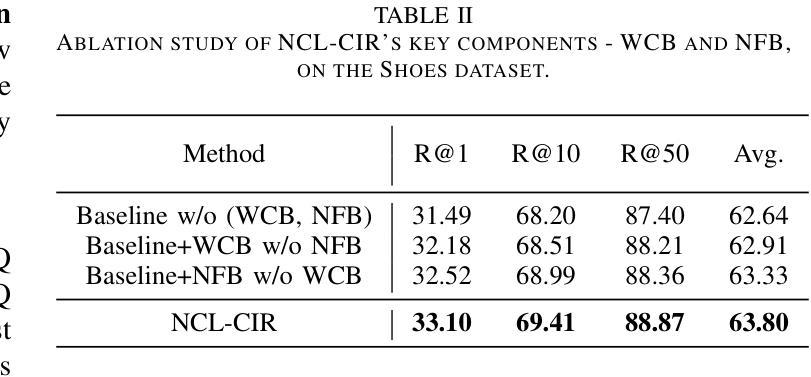