⚠️ 以下所有内容总结都来自于 大语言模型的能力,如有错误,仅供参考,谨慎使用
🔴 请注意:千万不要用于严肃的学术场景,只能用于论文阅读前的初筛!
💗 如果您觉得我们的项目对您有帮助 ChatPaperFree ,还请您给我们一些鼓励!⭐️ HuggingFace免费体验
2025-04-30 更新
MASR: Self-Reflective Reasoning through Multimodal Hierarchical Attention Focusing for Agent-based Video Understanding
Authors:Shiwen Cao, Zhaoxing Zhang, Junming Jiao, Juyi Qiao, Guowen Song, Rong Shen, Xiangbing Meng
Even in the era of rapid advances in large models, video understanding remains a highly challenging task. Compared to texts or images, videos commonly contain more information with redundancy, requiring large models to properly allocate attention at a global level for comprehensive and accurate understanding. To address this, we propose a Multimodal hierarchical Attention focusing Self-reflective Reasoning (MASR) framework for agent-based video understanding. The key innovation lies in its ability to detect and prioritize segments of videos that are highly relevant to the query. Firstly, MASR realizes Multimodal Coarse-to-fine Relevance Sensing (MCRS) which enhances the correlation between the acquired contextual information and the query. Secondly, MASR employs Dilated Temporal Expansion (DTE) to mitigate the risk of missing crucial details when extracting semantic information from the focused frames selected through MCRS. By iteratively applying MCRS and DTE in the self-reflective reasoning process, MASR is able to adaptively adjust the attention to extract highly query-relevant context and therefore improve the response accuracy. In the EgoSchema dataset, MASR achieves a remarkable 5% performance gain over previous leading approaches. In the Next-QA and IntentQA datasets, it outperforms the state-of-the-art standards by 0.2% and 0.3% respectively. In the Video-MME dataset that contains long-term videos, MASR also performs better than other agent-based methods.
即使在大型模型迅速发展的时代,视频理解仍然是一项极具挑战性的任务。相比于文本或图像,视频通常包含更多冗余信息,需要大型模型在全局层面适当地分配注意力,以实现全面而准确的视频理解。为了解决这个问题,我们提出了一个面向基于代理的视频理解的多模态层次化注意力聚焦自我反思推理(MASR)框架。其主要创新之处在于能够检测和优先处理与查询高度相关的视频片段。首先,MASR实现了多模态从粗到细的相关性感知(MCRS),增强了获取的背景信息与查询之间的相关性。其次,MASR采用膨胀时间扩展(DTE)技术,以缓解在通过MCRS选择的聚焦帧中提取语义信息时遗漏关键细节的风险。通过自我反思推理过程中迭代应用MCRS和DTE,MASR能够自适应地调整注意力以提取高度相关的上下文信息,从而提高响应准确性。在EgoSchema数据集上,MASR相较于之前领先的方法取得了5%的性能提升。在Next-QA和IntentQA数据集上,其性能分别超出当前最佳标准0.2%和0.3%。对于包含长期视频的视频MME数据集,MASR也表现出比其他基于代理的方法更好的性能。
论文及项目相关链接
Summary
本文介绍了视频理解的挑战性以及应对这一挑战的Multimodal层次化注意力聚焦自我反思推理(MASR)框架。MASR能够实现多模态粗到细的相关性感知,增强获取上下文信息与查询之间的相关性。此外,它通过膨胀时间扩展(DTE)缓解在提取选定关键帧语义信息时遗漏重要细节的风险。在多个数据集上的实验表明,MASR相较于其他前沿方法取得了显著的性能提升。
Key Takeaways
- 视频理解面临挑战,因为视频包含比文本或图像更多的信息,并存在冗余。
- MASR框架通过检测并优先处理与查询高度相关的视频片段来解决这一挑战。
- MASR实现了多模态粗到细的相关性感知(MCRS),增强了上下文信息与查询之间的相关性。
- MASR采用膨胀时间扩展(DTE)以缓解在提取选定关键帧语义信息时可能遗漏重要细节的风险。
- 通过自我反思推理过程迭代应用MCRS和DTE,MASR能够自适应地调整注意力,以提取与查询高度相关的上下文信息。
- 在EgoSchema数据集上,MASR相较于其他前沿方法实现了5%的性能提升。
点此查看论文截图
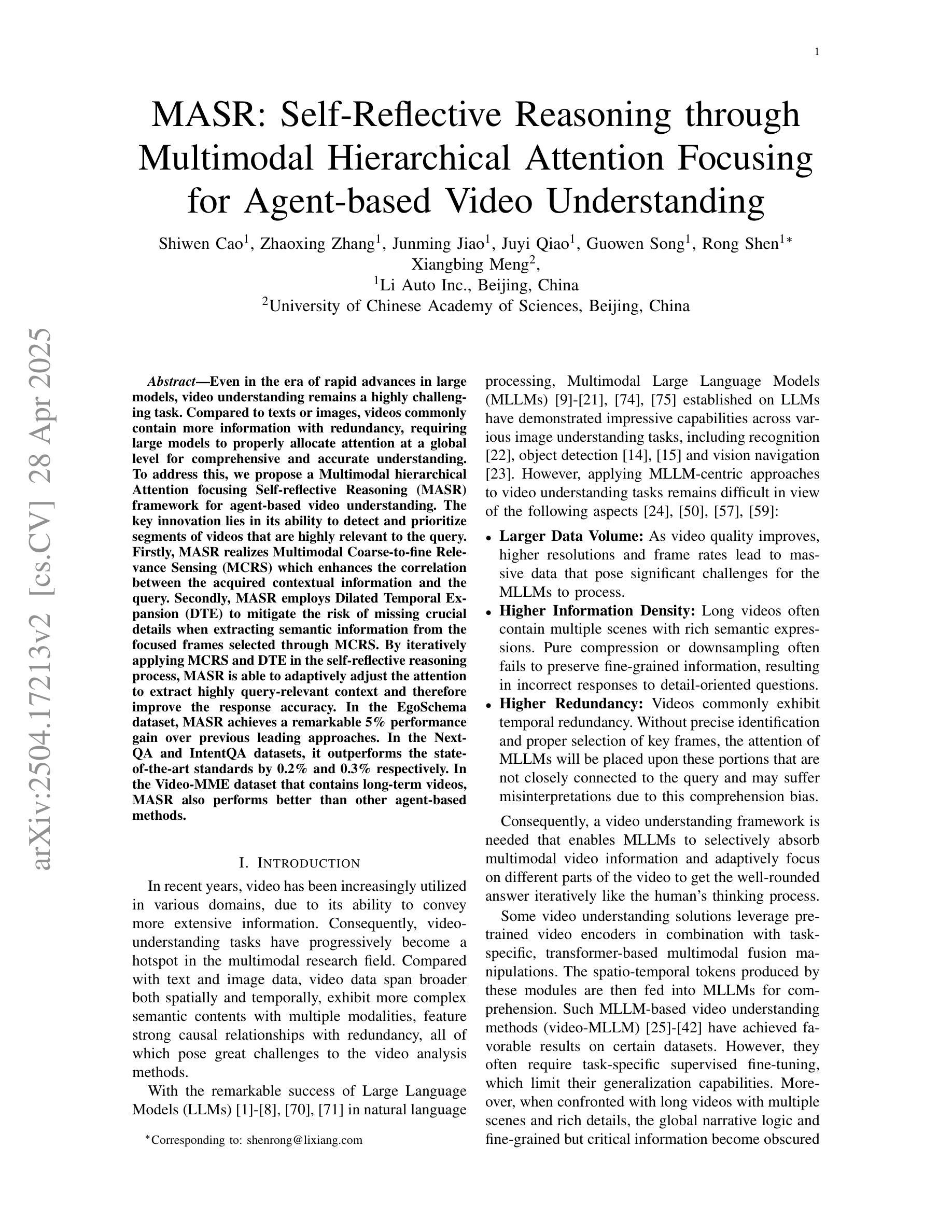
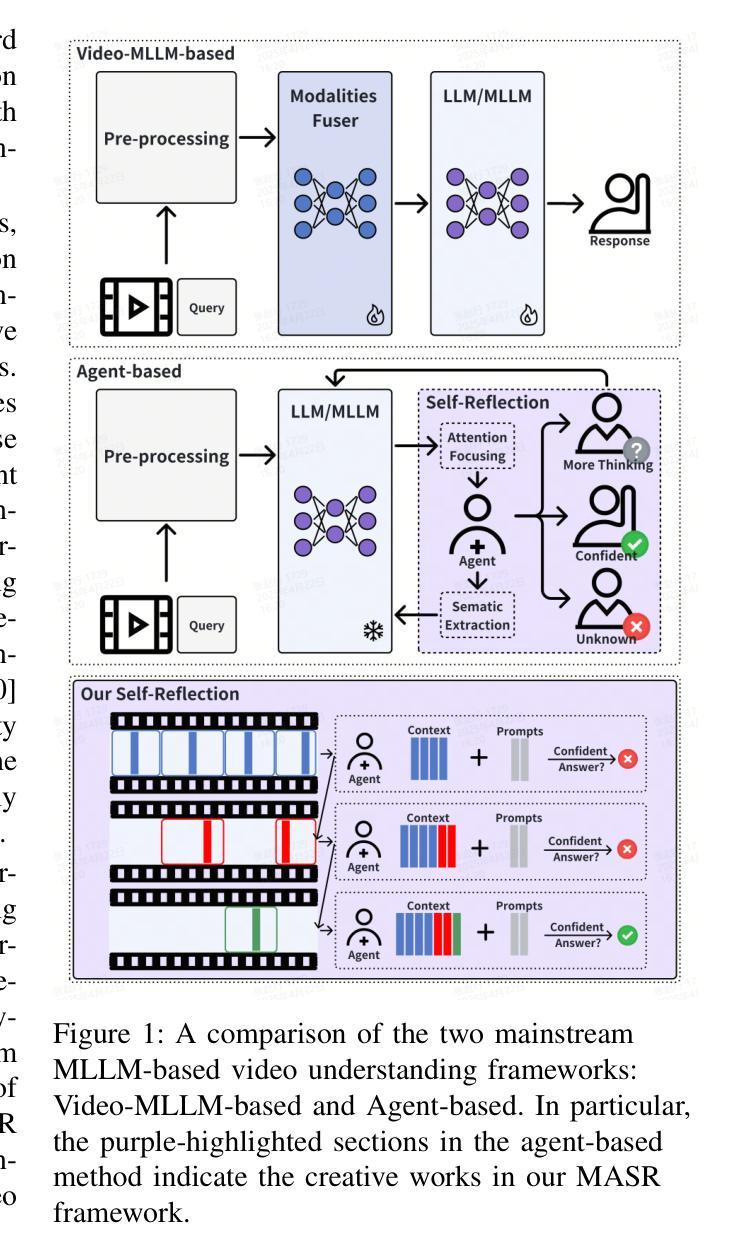
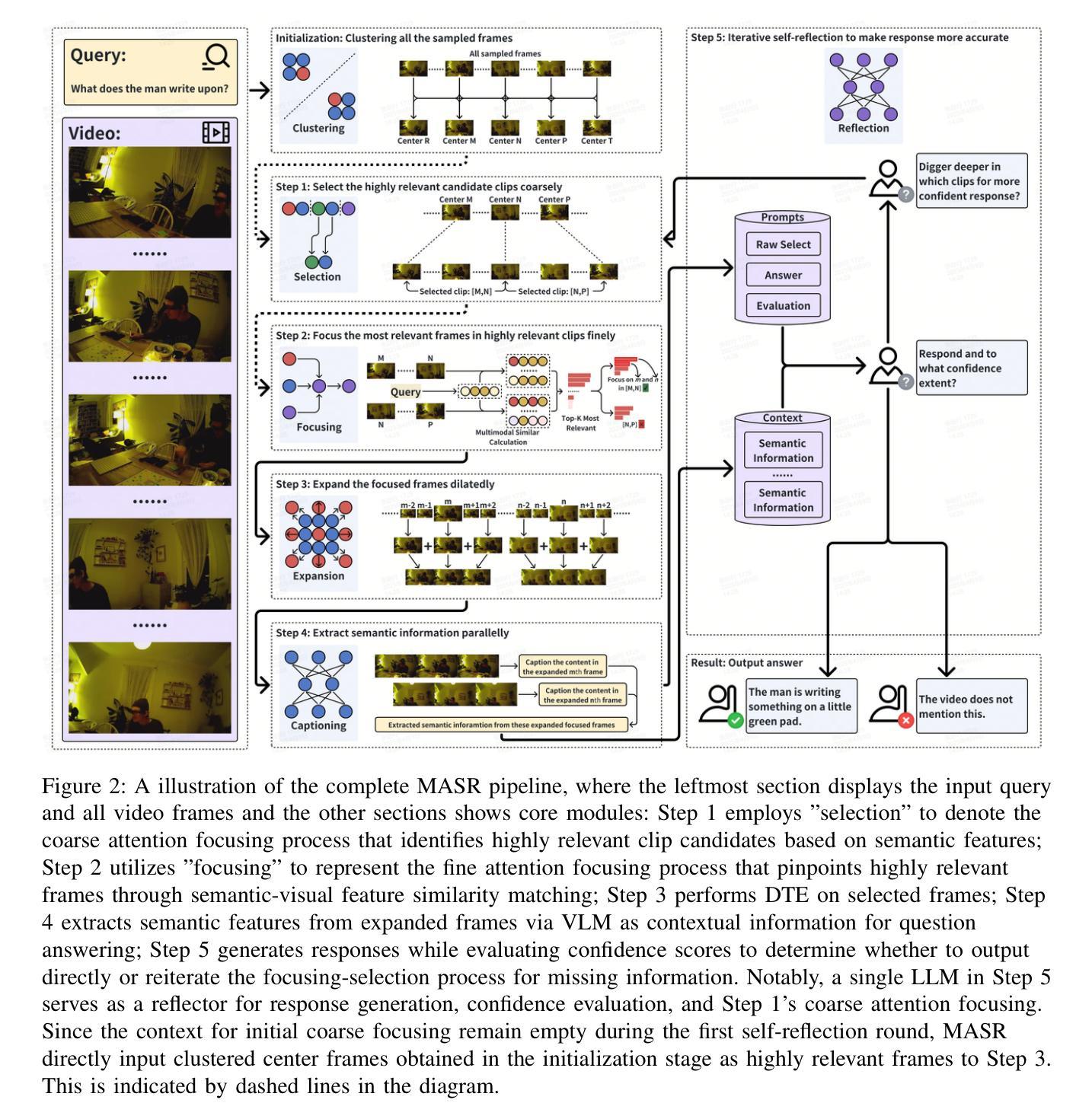
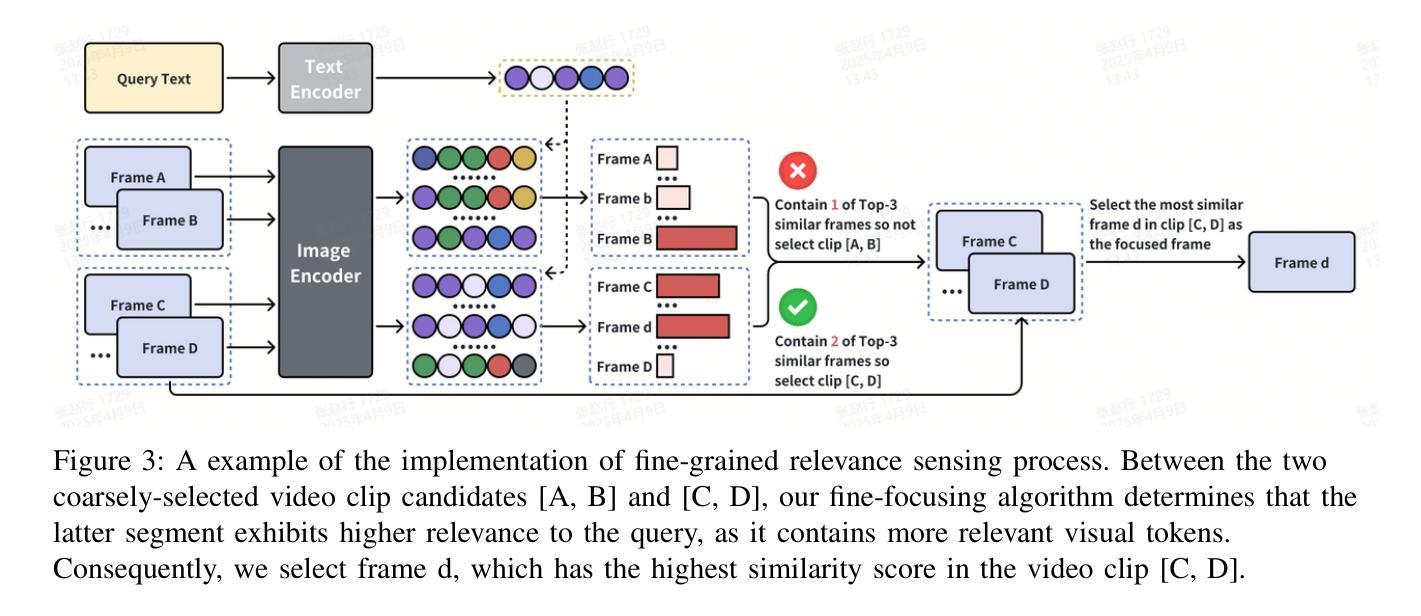
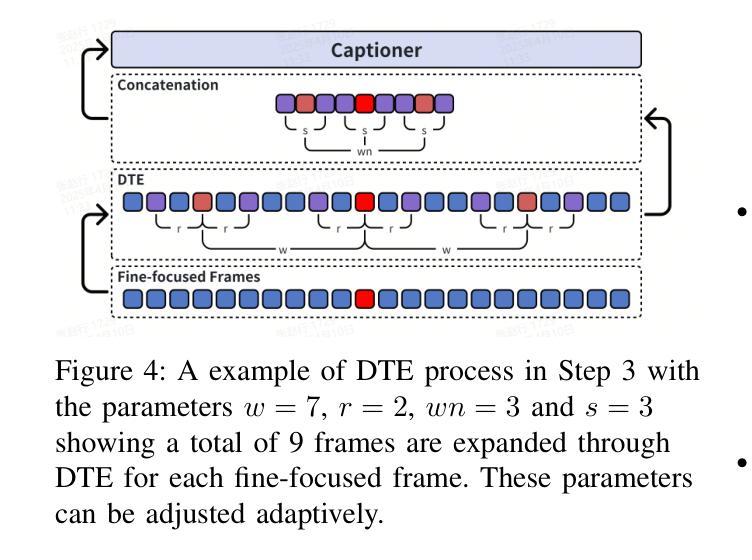
Video-XL-Pro: Reconstructive Token Compression for Extremely Long Video Understanding
Authors:Xiangrui Liu, Yan Shu, Zheng Liu, Ao Li, Yang Tian, Bo Zhao
Despite advanced token compression techniques, existing multimodal large language models (MLLMs) still struggle with hour-long video understanding. In this work, we propose Video-XL-Pro, an efficient method for extremely long video understanding, built upon Reconstructive Compression of Tokens (ReCoT), a learnable module that leverages self-supervised learning to generate comprehensive and compact video tokens. ReCoT introduces two key components: (i) Dynamic Token Synthesizer (DTS): DTS generates pseudo-video tokens from static image tokens by learning intra-token relationships, which are then used in masked video modeling. (ii) Semantic-Guided Masking (SGM): SGM adaptively masks redundant visual tokens to facilitate more effective reconstructive learning. To improve training efficiency in MLLMs fine-tuning, we introduce a video-specific dataset pruning strategy and design a simple yet Query-aware Selector that enables the model to precisely locate query-relevant video tokens. With only 3B parameters, Video-XL-Pro outperforms most 7B models trained on larger datasets across multiple long video understanding benchmarks. Moreover, it can process over 8K frames on a single A100 GPU while maintaining high-quality performance.
尽管有先进的令牌压缩技术,现有的多模态大型语言模型(MLLMs)在理解长达数小时的视频时仍然面临挑战。在这项工作中,我们提出了Video-XL-Pro,这是一种基于重建令牌压缩(ReCoT)的高效超长视频理解方法。ReCoT是一个可学习的模块,它利用自监督学习生成全面且紧凑的视频令牌。ReCoT引入了两个关键组件:(i)动态令牌合成器(DTS):DTS通过学习令牌内部关系从静态图像令牌生成伪视频令牌,然后用于遮挡视频建模。(ii)语义引导遮挡(SGM):SGM自适应地遮挡冗余的视觉令牌,以促进更有效的重建学习。为了提高MLLMs微调中的训练效率,我们引入了一种针对视频特定的数据集修剪策略,并设计了一个简单但查询感知的选择器,使模型能够精确定位与查询相关的视频令牌。Video-XL-Pro仅使用3B参数,就在多个长视频理解基准测试中优于在更大数据集上训练的7B模型。此外,它可以在单个A100 GPU上处理超过8K帧,同时保持高质量的性能。
论文及项目相关链接
Summary
本文提出了Video-XL-Pro模型,用于长时间视频理解。该模型基于重建令牌压缩技术(ReCoT),利用自监督学习生成全面且紧凑的视频令牌。主要创新点包括动态令牌合成器(DTS)和语义引导遮蔽(SGM)。DTS通过学习令牌间的内在联系生成伪视频令牌,SGM则自适应地遮蔽冗余的视觉令牌以优化重建学习。此外,为提高多模态大型语言模型(MLLMs)的训练效率,本文还提出了视频特定数据集修剪策略和查询感知选择器。Video-XL-Pro在多个长时间视频理解基准测试中表现优异,仅使用3B参数就超越了大多数使用更大数据集的7B模型,同时能在单个A100 GPU上处理超过8K帧,保持高性能。
Key Takeaways
- Video-XL-Pro是一个用于长时间视频理解的模型,基于重建令牌压缩技术(ReCoT)。
- 该模型包括两个关键组件:动态令牌合成器(DTS)和语义引导遮蔽(SGM),分别用于生成伪视频令牌和自适应遮蔽冗余视觉令牌。
- 通过使用自监督学习,Video-XL-Pro能够生成全面且紧凑的视频令牌,提高了视频理解的效果。
- 该模型通过视频特定数据集修剪策略和查询感知选择器提高了训练效率。
- Video-XL-Pro在多个长时间视频理解基准测试中表现出优异的性能。
- 仅使用3B参数的Video-XL-Pro超越了大多数使用更大数据集的7B模型。
点此查看论文截图
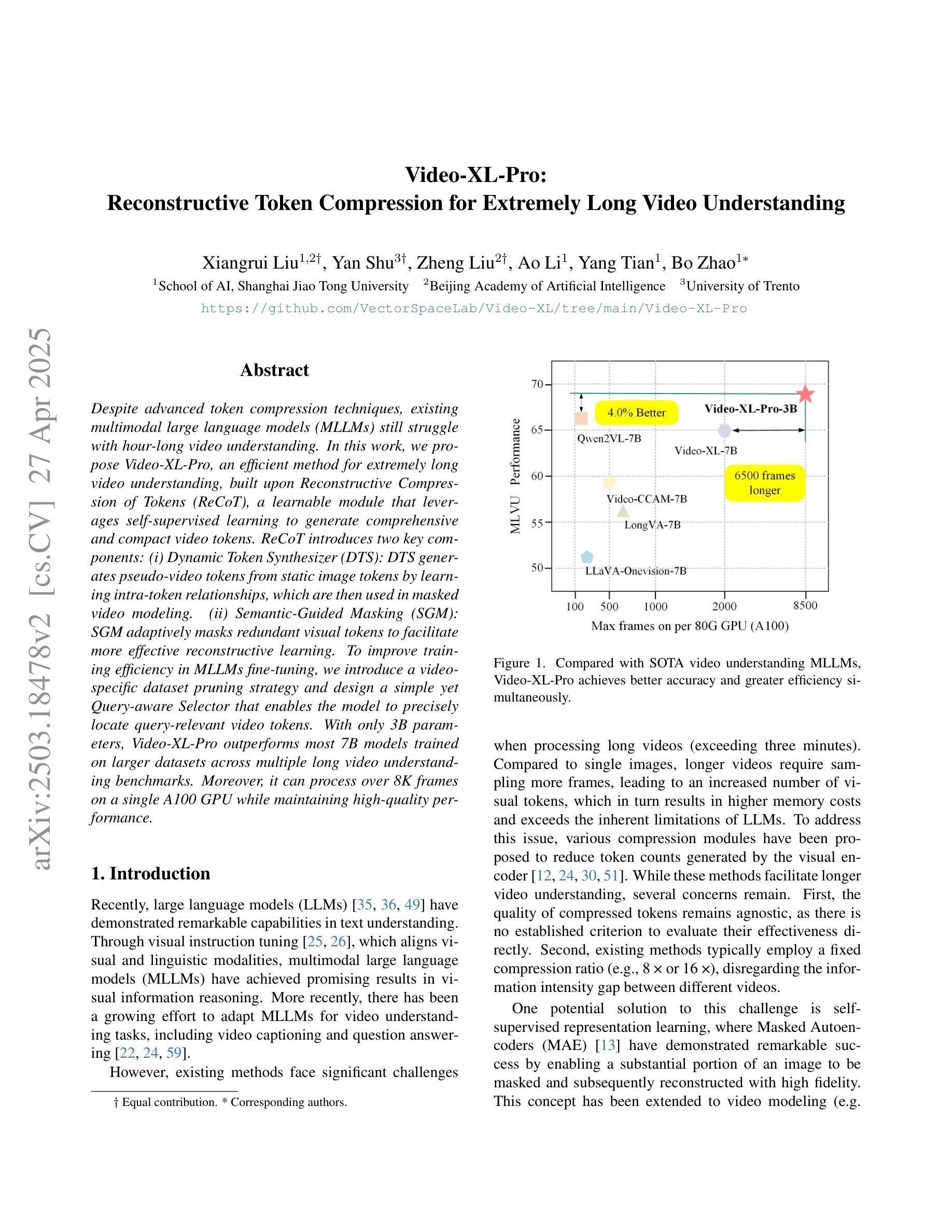
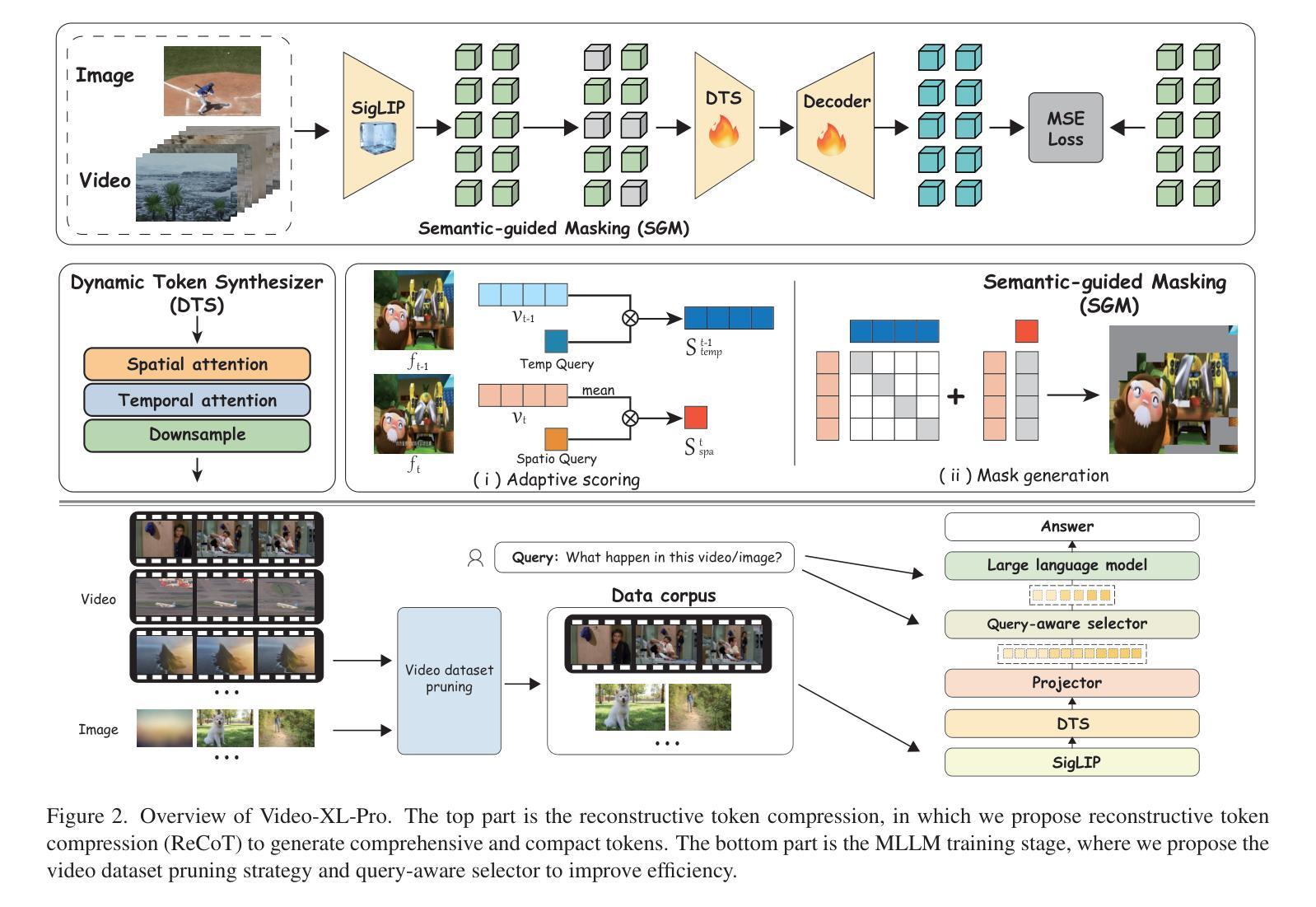
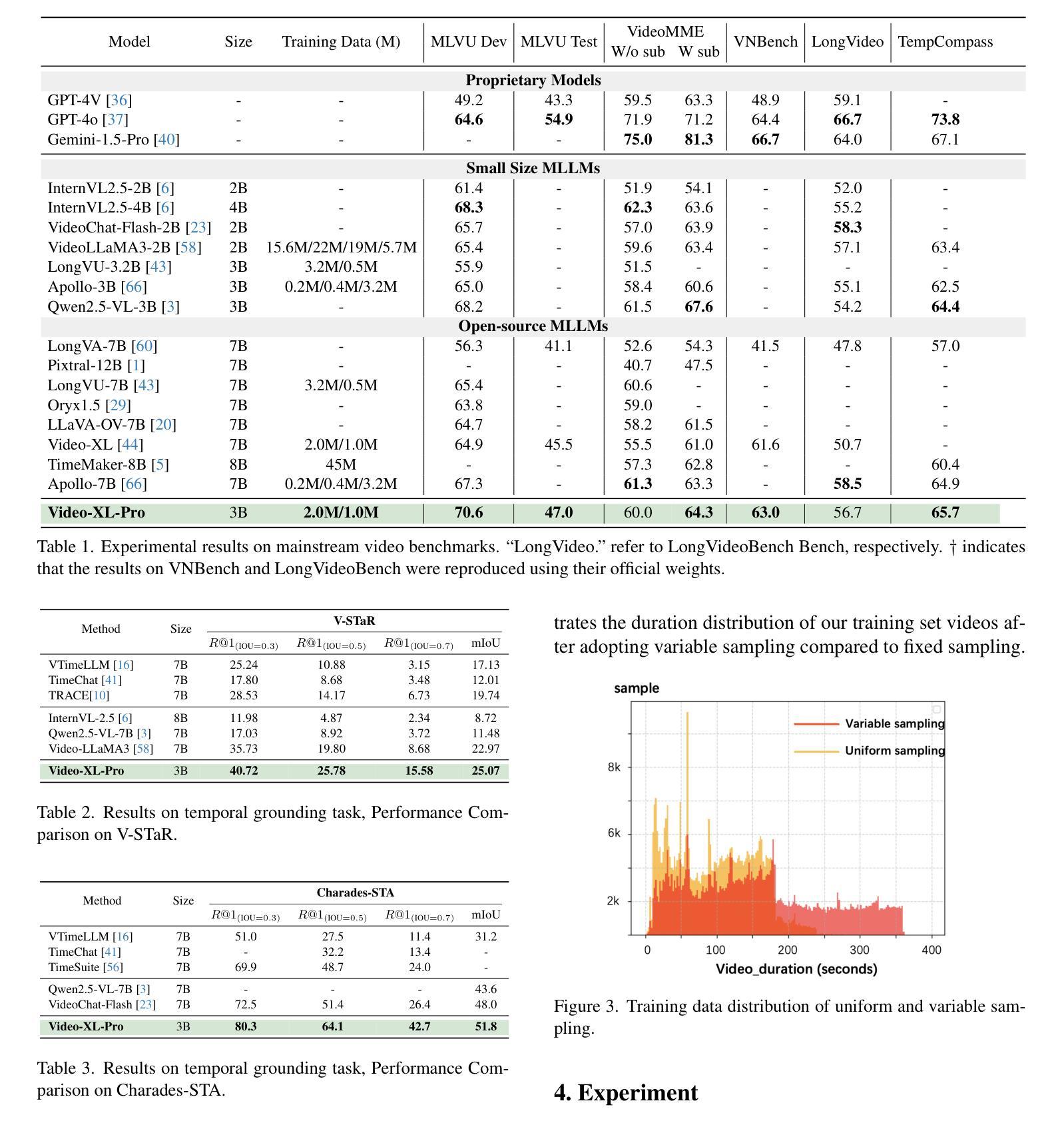
Optimizing GPT for Video Understanding: Zero-Shot Performance and Prompt Engineering
Authors:Mark Beliaev, Victor Yang, Madhura Raju, Jiachen Sun, Xinghai Hu
In this study, we tackle industry challenges in video content classification by exploring and optimizing GPT-based models for zero-shot classification across seven critical categories of video quality. We contribute a novel approach to improving GPT’s performance through prompt optimization and policy refinement, demonstrating that simplifying complex policies significantly reduces false negatives. Additionally, we introduce a new decomposition-aggregation-based prompt engineering technique, which outperforms traditional single-prompt methods. These experiments, conducted on real industry problems, show that thoughtful prompt design can substantially enhance GPT’s performance without additional finetuning, offering an effective and scalable solution for improving video classification.
在这项研究中,我们应对视频内容分类行业的挑战,通过探索和优化基于GPT的模型,进行零样本分类,涵盖七大关键视频质量类别。我们提出了一种改进GPT性能的新方法,通过提示优化和政策细化,证明简化复杂政策可以显著降低误报。此外,我们引入了一种基于分解聚合的提示工程技术,它优于传统的单一提示方法。这些在真实行业问题上进行的实验表明,有策略的提示设计可以显著提高GPT的性能,无需额外的微调,为改进视频分类提供了一种有效且可扩展的解决方案。
论文及项目相关链接
PDF 9 pages
Summary:本研究通过探索和优化基于GPT的模型,针对视频内容分类行业的挑战,提出了零样本分类的新方法,涉及七种关键视频质量类别。研究通过优化提示和精炼策略来提升GPT的性能,发现简化复杂策略能显著降低误报。此外,引入基于分解聚合的提示工程技术,表现优于传统单一提示方法。在真实行业问题上的实验表明,精心设计的提示能显著提高GPT的性能,无需额外微调,为改进视频分类提供了有效且可扩展的解决方案。
Key Takeaways:
- 研究针对视频内容分类行业的挑战,采用GPT模型进行零样本分类。
- 研究通过优化提示和精炼策略提升GPT性能。
- 简化复杂策略能显著降低误报。
- 引入基于分解聚合的提示工程技术,表现优于传统方法。
- 精心设计的提示能提高GPT性能,无需额外微调。
- 新方法针对七种关键视频质量类别进行分类。
点此查看论文截图
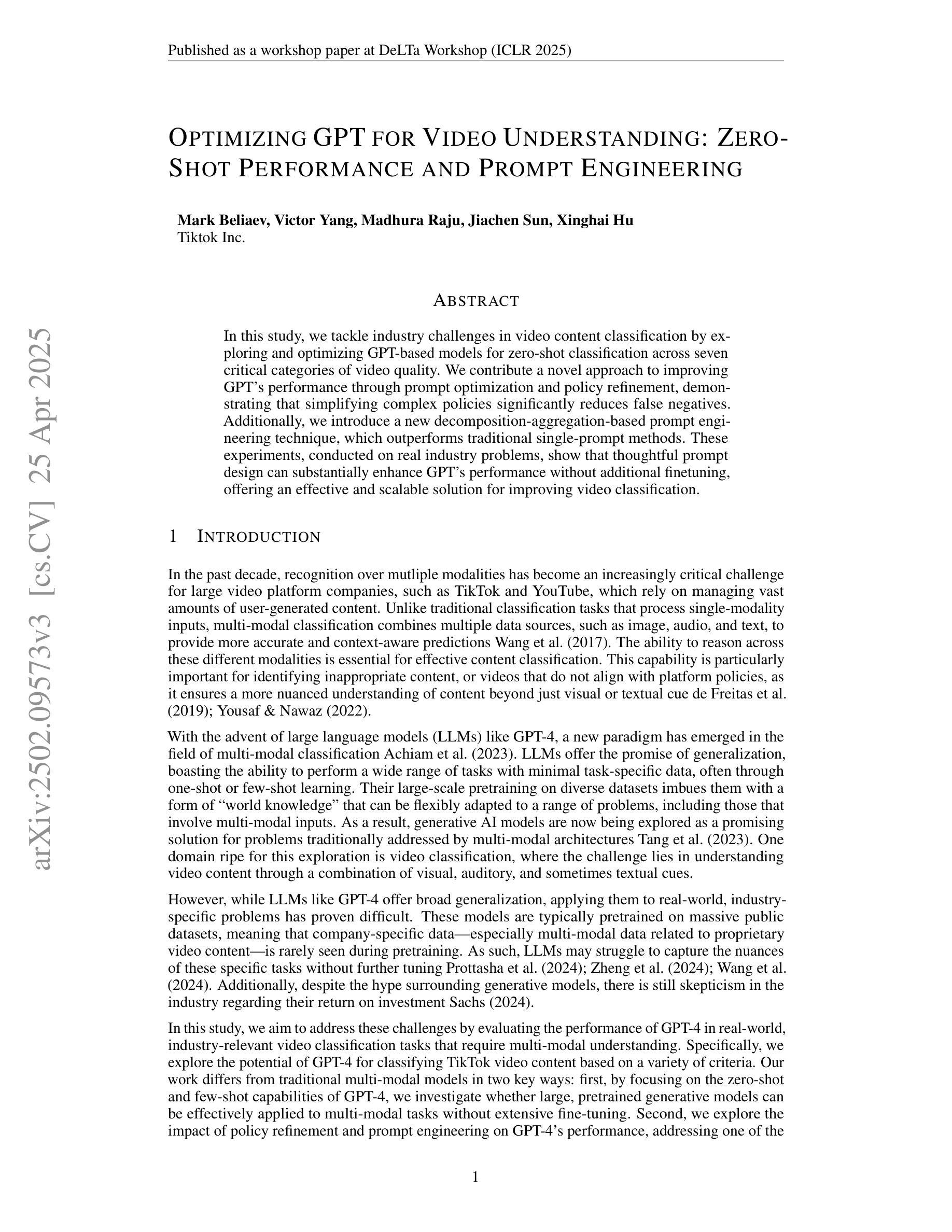
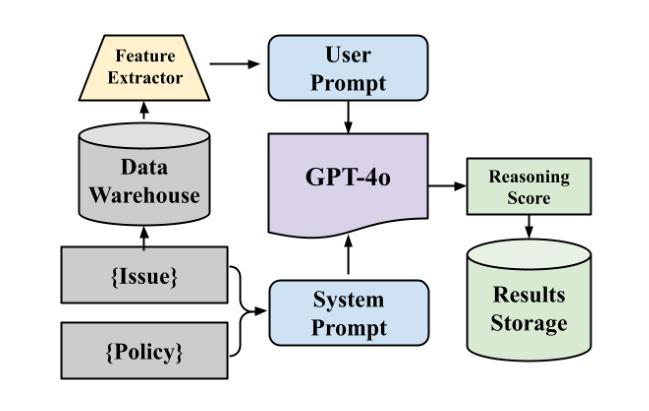
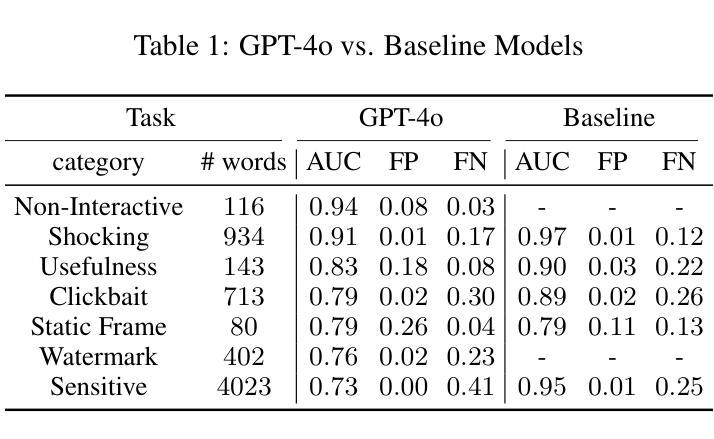
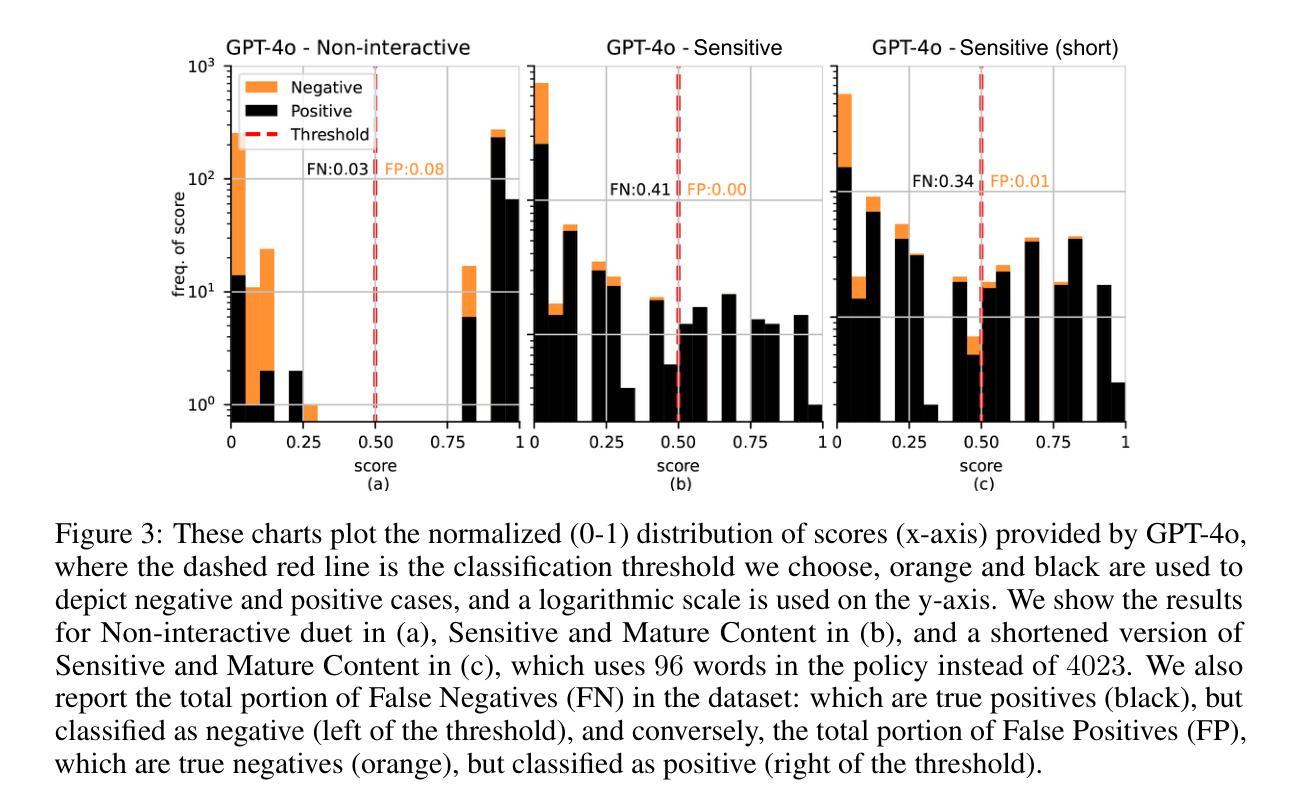
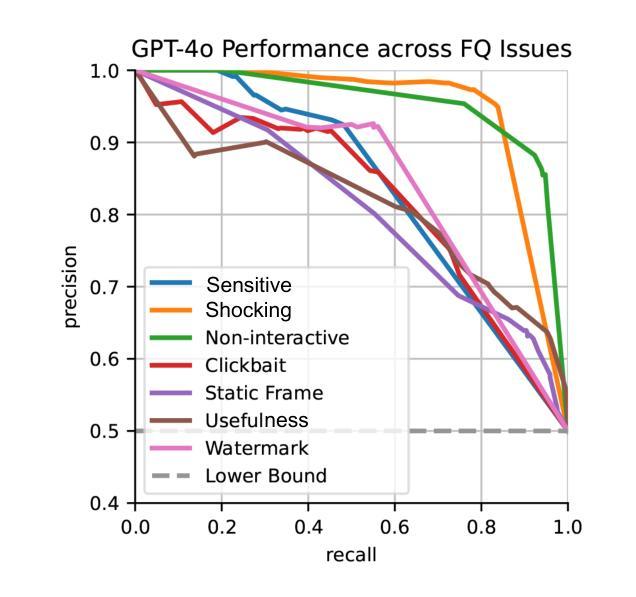