⚠️ 以下所有内容总结都来自于 大语言模型的能力,如有错误,仅供参考,谨慎使用
🔴 请注意:千万不要用于严肃的学术场景,只能用于论文阅读前的初筛!
💗 如果您觉得我们的项目对您有帮助 ChatPaperFree ,还请您给我们一些鼓励!⭐️ HuggingFace免费体验
2025-04-30 更新
DeeCLIP: A Robust and Generalizable Transformer-Based Framework for Detecting AI-Generated Images
Authors:Mamadou Keita, Wassim Hamidouche, Hessen Bougueffa Eutamene, Abdelmalik Taleb-Ahmed, Abdenour Hadid
This paper introduces DeeCLIP, a novel framework for detecting AI-generated images using CLIP-ViT and fusion learning. Despite significant advancements in generative models capable of creating highly photorealistic images, existing detection methods often struggle to generalize across different models and are highly sensitive to minor perturbations. To address these challenges, DeeCLIP incorporates DeeFuser, a fusion module that combines high-level and low-level features, improving robustness against degradations such as compression and blurring. Additionally, we apply triplet loss to refine the embedding space, enhancing the model’s ability to distinguish between real and synthetic content. To further enable lightweight adaptation while preserving pre-trained knowledge, we adopt parameter-efficient fine-tuning using low-rank adaptation (LoRA) within the CLIP-ViT backbone. This approach supports effective zero-shot learning without sacrificing generalization. Trained exclusively on 4-class ProGAN data, DeeCLIP achieves an average accuracy of 89.00% on 19 test subsets composed of generative adversarial network (GAN) and diffusion models. Despite having fewer trainable parameters, DeeCLIP outperforms existing methods, demonstrating superior robustness against various generative models and real-world distortions. The code is publicly available at https://github.com/Mamadou-Keita/DeeCLIP for research purposes.
本文介绍了DeeCLIP,这是一个使用CLIP-ViT和融合学习检测AI生成图像的新型框架。尽管生成模型在创建高度逼真的图像方面取得了重大进展,但现有的检测方法在跨不同模型推广时经常遇到困难,并且对微小的扰动非常敏感。为了应对这些挑战,DeeCLIP结合了DeeFuser,一个融合模块,该模块结合了高级和低级特征,提高了对抗压缩、模糊等降质的稳健性。此外,我们应用三元组损失来优化嵌入空间,提高模型区分真实和合成内容的能力。为了进一步实现在保留预训练知识的同时进行轻量级适配,我们在CLIP-ViT主干中采用基于低秩适配(LoRA)的参数高效微调方法。这种方法支持有效的零样本学习,而不会牺牲泛化能力。DeeCLIP仅使用4类ProGAN数据进行训练,在由生成对抗网络(GAN)和扩散模型组成的19个测试子集上取得了平均89.00%的准确率。尽管可训练参数较少,DeeCLIP仍优于现有方法,显示出对各种生成模型和现实世界失真的卓越稳健性。代码已公开发布在https://github.com/Mamadou-Keita/DeeCLIP,仅供研究使用。
论文及项目相关链接
Summary
DeeCLIP框架利用CLIP-ViT和融合学习技术检测AI生成的图像。为提高模型对各类生成模型及真实世界失真的稳健性,引入DeeFuser融合模块并结合三元损失优化嵌入空间。通过采用参数有效的微调方法,DeeCLIP支持零样本学习并维持泛化能力。在特定数据集上训练的DeeCLIP模型在测试集上平均准确度达89%,表现优于现有方法。
Key Takeaways
- DeeCLIP是一个用于检测AI生成图像的新框架,基于CLIP-ViT和融合学习技术。
- 引入DeeFuser融合模块,结合高低层次特征,提高模型对压缩、模糊等降质的稳健性。
- 应用三元损失优化嵌入空间,增强模型区分真实和合成内容的能力。
- 采用参数有效的微调方法,支持零样本学习同时保持泛化能力。
- DeeCLIP在特定数据集上训练,对由生成对抗网络(GAN)和扩散模型组成的测试集平均准确度达89%。
- DeeCLIP表现优于现有方法,对各种生成模型和真实世界失真具有更强的稳健性。
点此查看论文截图
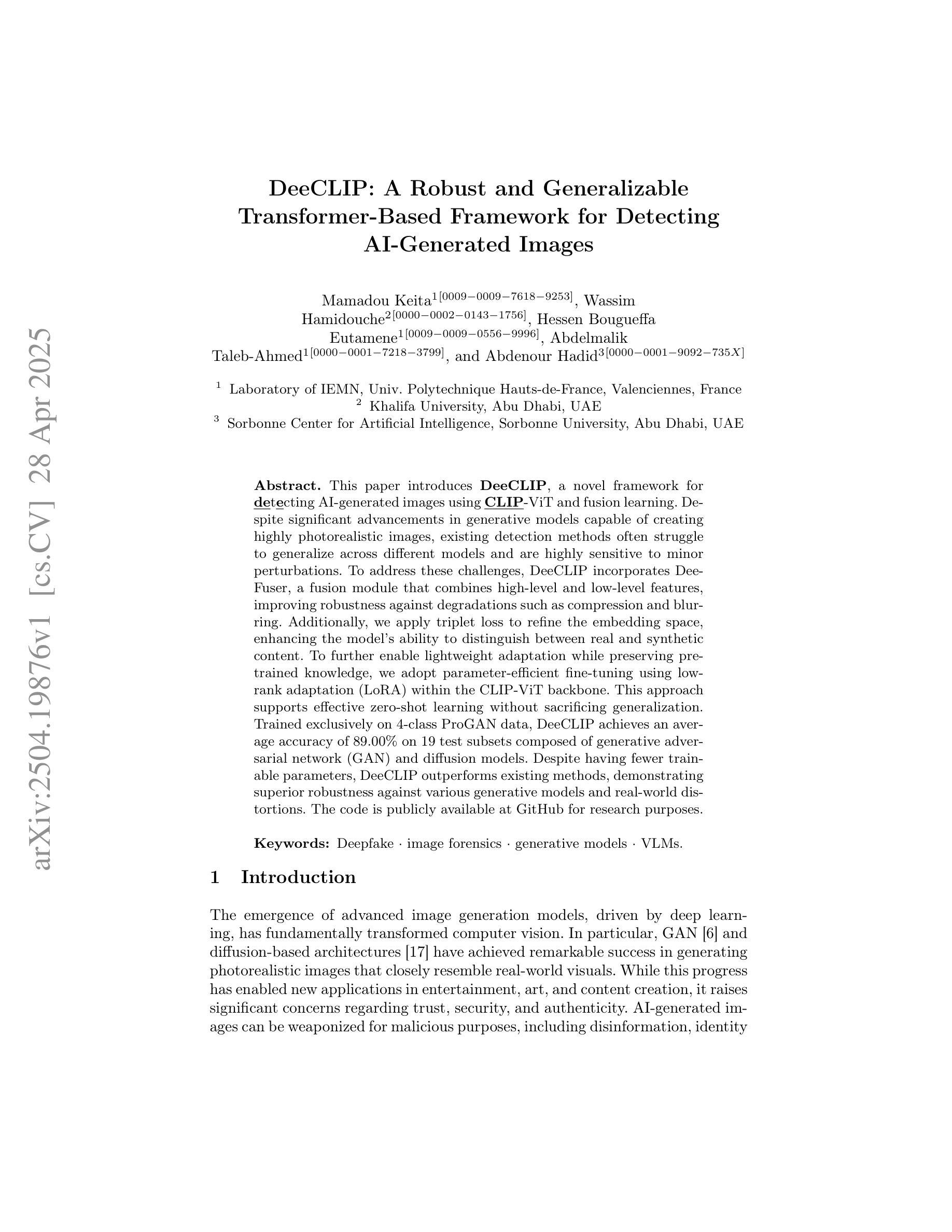
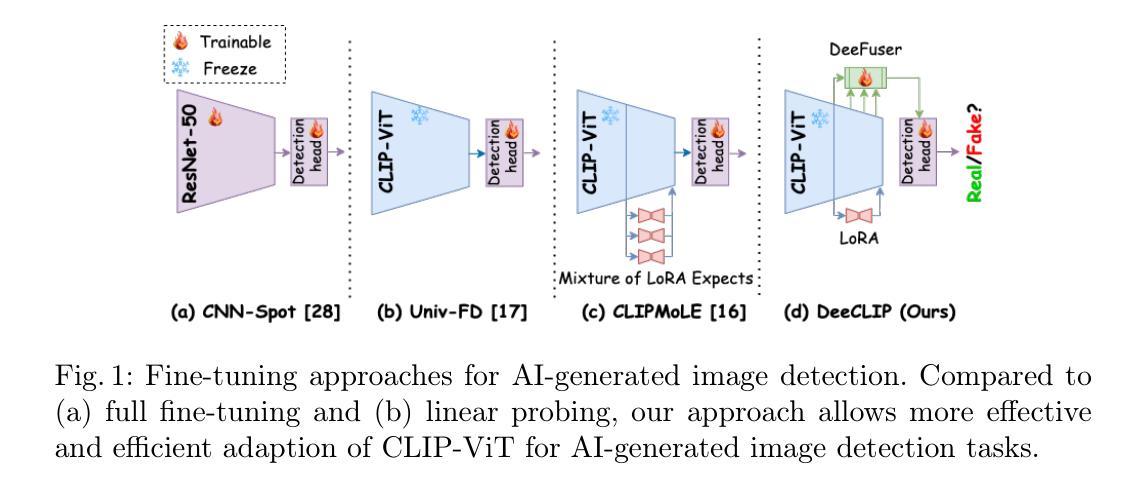
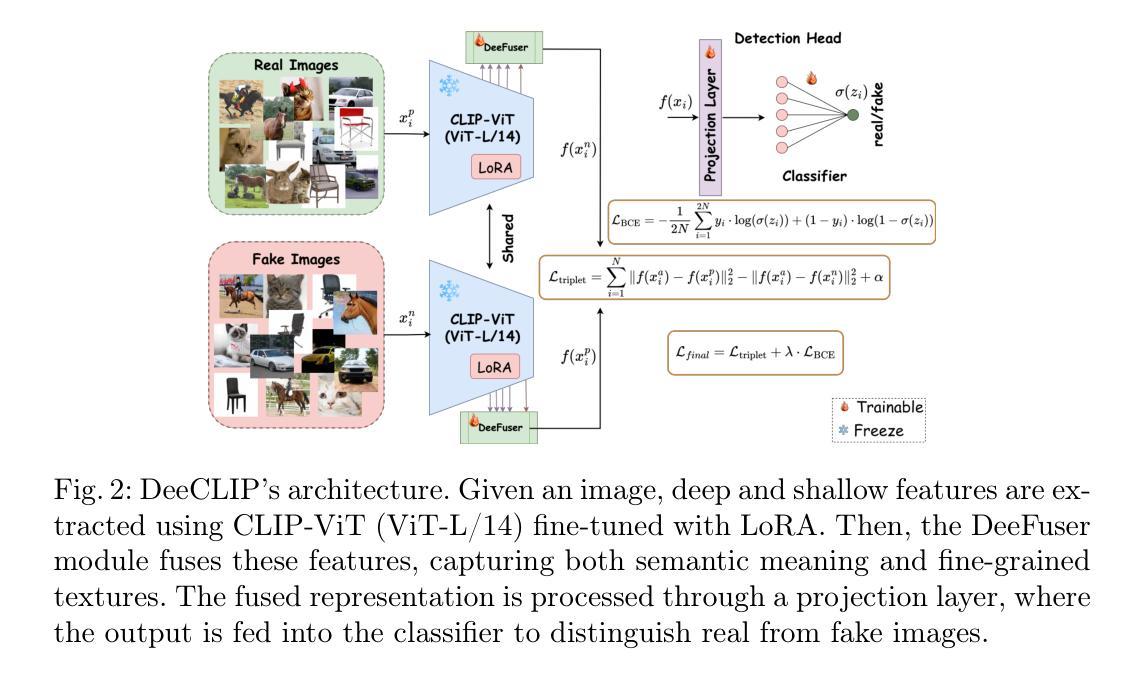
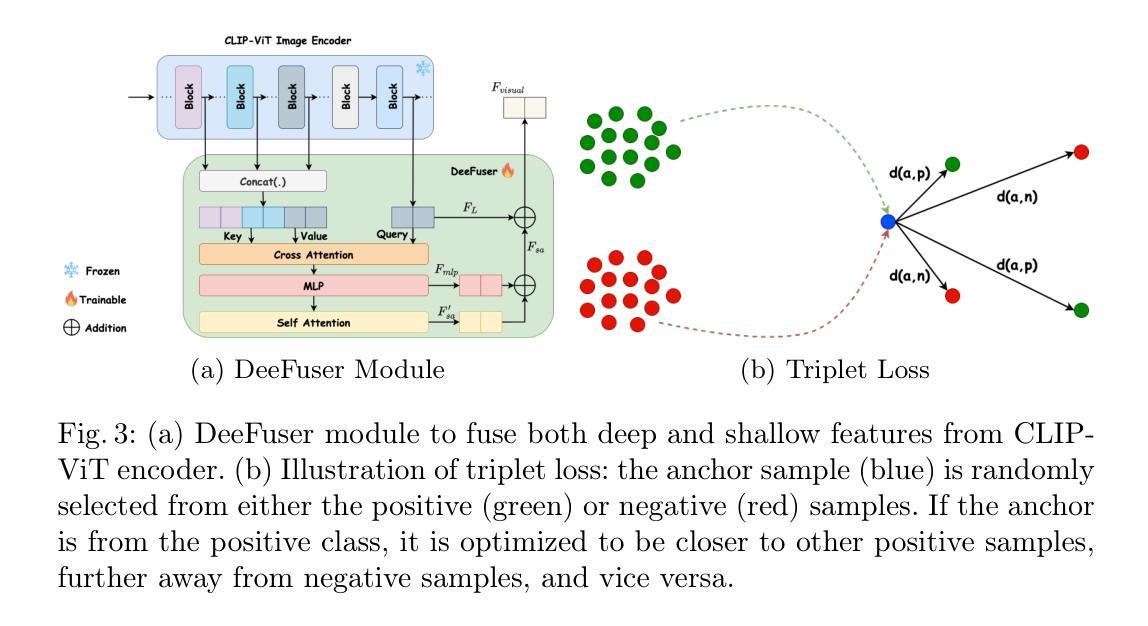