⚠️ 以下所有内容总结都来自于 大语言模型的能力,如有错误,仅供参考,谨慎使用
🔴 请注意:千万不要用于严肃的学术场景,只能用于论文阅读前的初筛!
💗 如果您觉得我们的项目对您有帮助 ChatPaperFree ,还请您给我们一些鼓励!⭐️ HuggingFace免费体验
2025-04-30 更新
Towards Robust Dialogue Breakdown Detection: Addressing Disruptors in Large Language Models with Self-Guided Reasoning
Authors:Abdellah Ghassel, Xianzhi Li, Xiaodan Zhu
Large language models (LLMs) are rapidly changing various domains. However, their capabilities in handling conversational breakdowns still require an in-depth exploration. This paper addresses the challenge of detecting and mitigating dialogue breakdowns within LLM-driven conversational systems. While powerful models from OpenAI and Anthropic excel in many dialogue tasks, they can still produce incoherent or contradictory responses, commonly referred to as breakdowns, which undermine user trust. To tackle this, we propose an approach that combines specialized fine-tuning with advanced prompting strategies, including few-shot learning, chain-of-thought reasoning, and analogical prompting. In particular, we fine-tune a small 8B model and demonstrate its robust classification and calibration capabilities in English and Japanese dialogue. We also validate its generalization on the BETOLD dataset, achieving a 7% accuracy improvement over its base model. Furthermore, we introduce a real-time deployment architecture that selectively escalates suspicious responses to more resource-intensive frontier models only when breakdowns are detected, significantly cutting operational expenses and energy consumption. Experimental results show our method surpasses prior state-of-the-art specialized classifiers while also narrowing performance gaps between smaller open-source models and large proprietary ones. Our approach offers a scalable solution for robust conversational AI in high-impact domains by combining efficiency, interpretability, and reliability.
大型语言模型(LLM)正在迅速改变各个领域。然而,它们在处理对话中断方面的能力仍然需要深入探索。本文旨在解决在LLM驱动的对话系统中检测和缓解对话中断的挑战。虽然来自OpenAI和Anthropic的强大模型在许多对话任务中表现出色,但它们仍然可能产生不连贯或矛盾的回应,这些回应通常被称为中断,破坏了用户的信任。为了解决这个问题,我们提出了一种结合专门的微调与先进的提示策略的方法,包括小样本学习、链式思维推理和类比提示。特别是,我们对一个小型的8B模型进行了微调,并展示了它在英语和日语对话中的稳健分类和校准能力。我们在BETOLD数据集上验证了其泛化性,相较于基础模型实现了7%的准确率提升。此外,我们引入了一种实时部署架构,该架构仅在检测到中断时选择将可疑的响应升级到资源密集的前沿模型,从而大幅降低了运营成本并减少了能源消耗。实验结果表明,我们的方法超越了现有的最先进的分类器,同时缩小了开源小型模型和大型专有模型之间的性能差距。通过结合效率、可解释性和可靠性,我们的方法为高影响力领域提供稳健的会话AI的可扩展解决方案。
论文及项目相关链接
Summary
大型语言模型(LLMs)在多个领域具有广泛的应用前景,但在处理对话中断方面仍存在挑战。本文提出一种结合特殊微调与先进提示策略的方法,以检测和缓解LLM驱动对话系统中的对话中断问题。通过微调小型8B模型并展示其在英语和日语对话中的稳健分类和校准能力,同时引入实时部署架构,只在检测到对话中断时选择性地调用更多资源密集型前沿模型,实现了成本节约和能源消耗的降低。实验结果表明,该方法超越了现有的专业分类器,并缩小了开源小型模型与大型专有模型之间的性能差距,为高效、可解释和可靠的对话AI提供了可伸缩的解决方案。
Key Takeaways
- 大型语言模型(LLMs)在对话系统中的表现仍需深入研究,特别是在处理对话中断方面。
- 论文提出了一种结合特殊微调与先进提示策略的方法,旨在检测和缓解对话中断问题。
- 通过微调小型8B模型,展示其在英语和日语对话中的稳健分类和校准能力。
- 引入实时部署架构,实现成本节约和能源消耗的降低。
- 方法超越了现有的专业分类器,并缩小了不同模型之间的性能差距。
- 论文提出的解决方案旨在为高效、可解释和可靠的对话AI提供可伸缩的解决方案。
点此查看论文截图
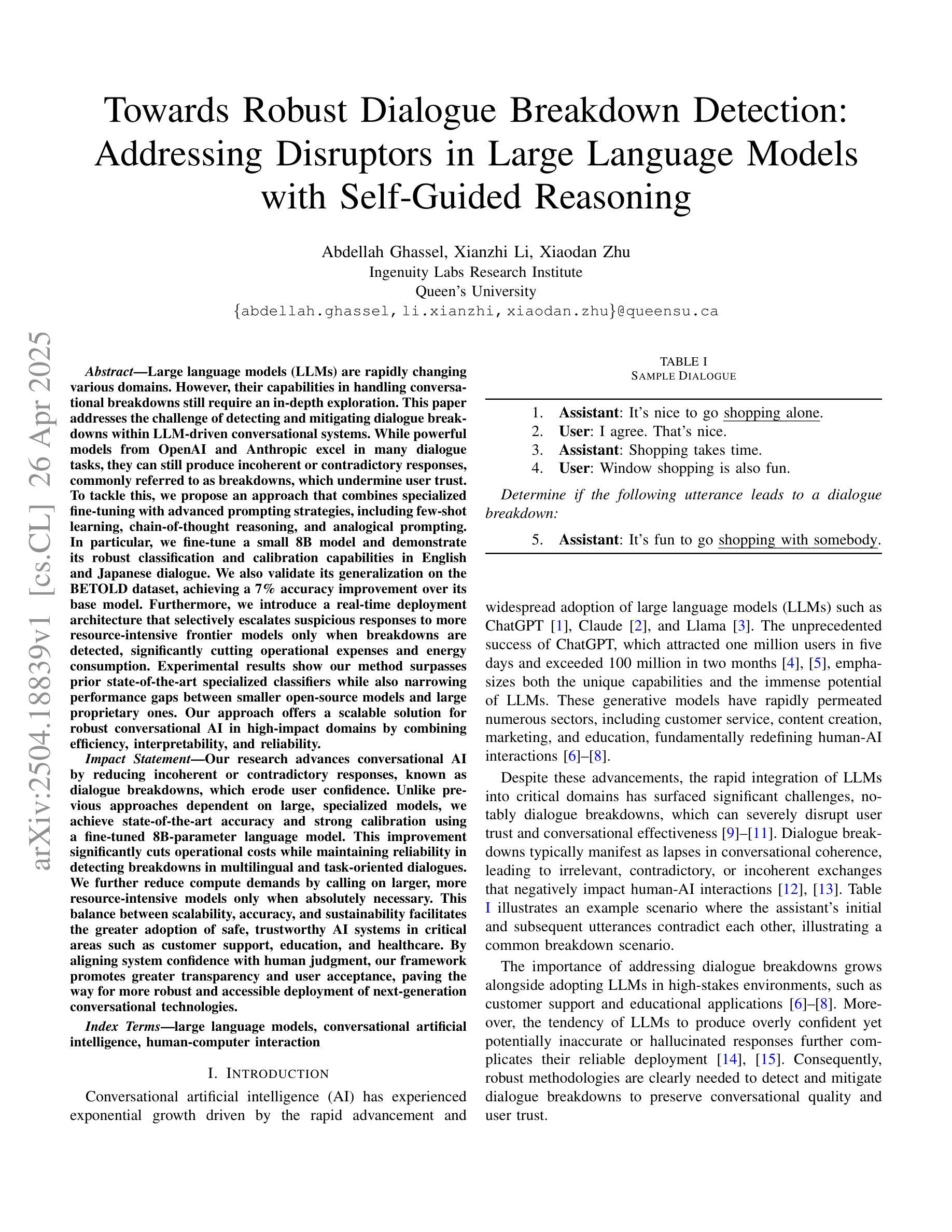
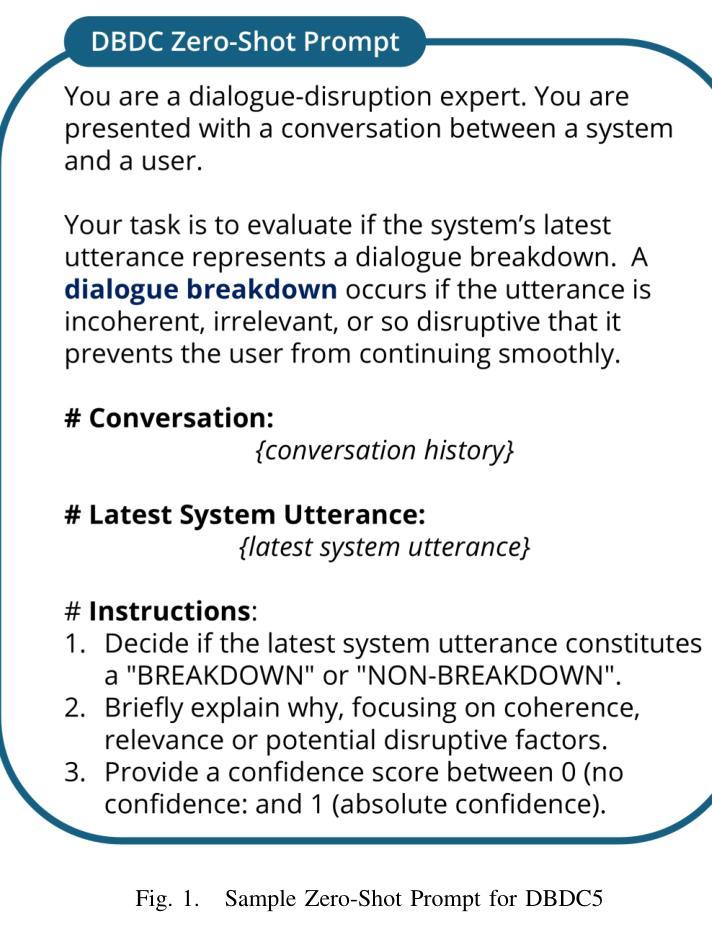
Molecular Similarity in Machine Learning of Energies in Chemical Reaction Networks
Authors:Stefan Gugler, Markus Reiher
Machine learning has emerged as a powerful tool for predicting molecular properties in chemical reaction networks with reduced computational cost. However, accurately predicting energies of transition state (TS) structures remains a challenge due to their distinct electronic characteristics compared to stable intermediates. In this work, we investigate the limitations of structural descriptors in capturing electronic differences between minima and TS structures. We explore $\Delta$-machine learning approaches to predict correlation energy corrections using both Hartree-Fock (HF) and density functional theory (DFT) as reference methods. Our results demonstrate that learning the energy difference between DFT and coupled cluster methods outperforms direct learning and HF-based $\Delta$-learning. We also assess the effectiveness of combining electronic descriptors with structural ones but find that simple electronic features do not significantly enhance the prediction of TS energies. These findings highlight the need for more sophisticated descriptors or integrated approaches to accurately predict the electronic energies of TS structures within chemical reaction networks.
机器学习已经成为预测化学反应网络中分子属性的一种强大工具,且计算成本较低。然而,由于过渡态结构与稳定中间体的电子特性不同,准确预测过渡态的能量仍然是一个挑战。在这项工作中,我们研究了结构描述符在捕获最小值和过渡态结构之间电子差异方面的局限性。我们探索了使用Hartree-Fock(HF)和密度泛函理论(DFT)作为参考方法来预测相关能量校正的Δ机器学习的方法。我们的结果表明,学习DFT与耦合群方法之间的能量差异优于直接学习和基于HF的Δ学习。我们还评估了将电子描述符与结构描述符相结合的有效性,但发现简单的电子特征并没有显著增强对过渡态能量的预测。这些发现强调需要更复杂的描述符或综合方法来准确预测化学反应网络中过渡态结构的电子能量。
论文及项目相关链接
PDF 32 pages, 7 figures
Summary
机器学习已成为预测化学反应网络中分子属性的强大工具,但准确预测过渡态结构的能量仍具有挑战性。本文探讨了结构描述符在捕捉最小态和过渡态结构之间的电子差异方面的局限性。本文探索了使用Hartree-Fock(HF)和密度泛函理论(DFT)作为参考方法的Δ机器学习方法来预测关联能量校正。结果表明,学习DFT与耦合簇方法之间的能量差异优于直接学习和HF基础的Δ学习。评估了将电子描述符与结构描述符相结合的效果,但发现简单的电子特征并未显着提高过渡态能量的预测。这强调了需要更复杂的描述符或综合方法来准确预测化学反应网络中过渡态结构的电子能量。
Key Takeaways
- 机器学习在预测化学反应网络中分子属性方面表现出强大的能力,但在准确预测过渡态结构能量方面仍存在挑战。
- 过渡态结构具有独特的电子特性,与稳定中间体不同,这使得准确预测变得困难。
- 探讨了结构描述符在捕捉最小态和过渡态结构之间电子差异的局限性。
- 使用Hartree-Fock(HF)和密度泛函理论(DFT)作为参考方法的Δ机器学习方法被探索,以预测关联能量校正。
- 学习DFT与耦合簇方法之间的能量差异表现最佳。
- 结合电子描述符和结构描述符的效果有限,简单电子特征并不能显著提高过渡态能量的预测准确性。
点此查看论文截图
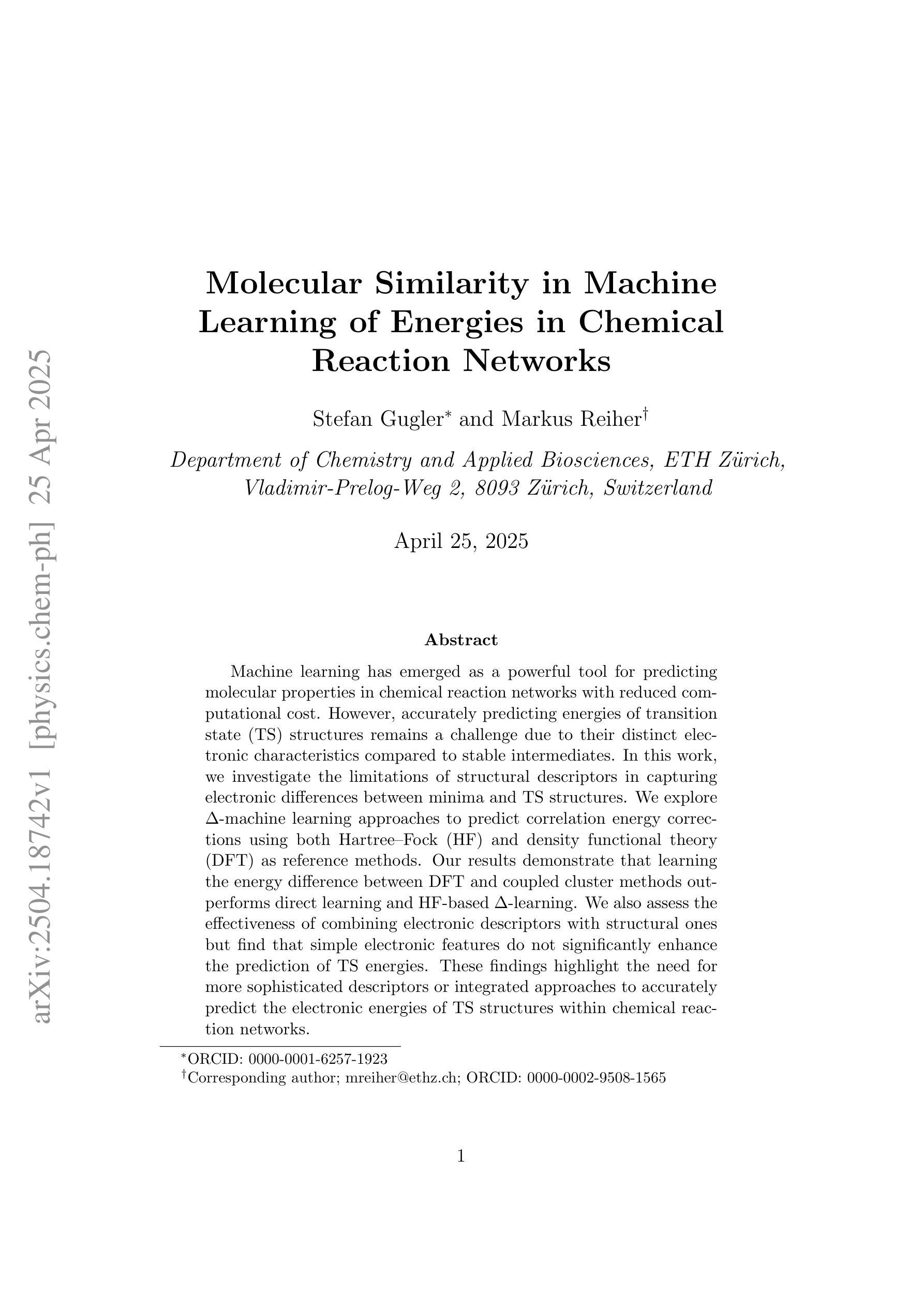